Notification: View the latest site access restrictions, updates, and resources related to the coronavirus (COVID-19) »

Combined 'SuperLab' Demonstrates Unique Hybrid Power Plant
Two national laboratories connected by high-speed network bring renewables and nuclear together.
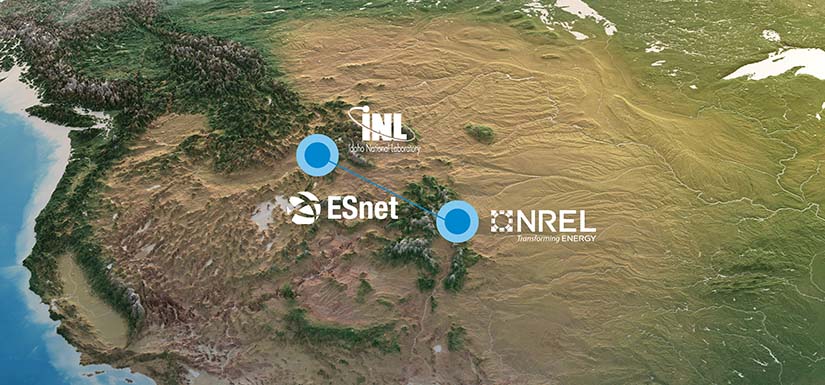
For a 60-minute period in January 2023, a power plant like no other existed in the U.S. Mountain West. It contained a solar array, lithium-ion battery, hydrogen electrolyzers, and a nuclear reactor, all coordinating with each other to provide reliable power. Even more unusual, the plant combined real and simulated technologies hundreds of miles apart.
This unique power plant was part of a national research and development project to remotely connect energy assets in real time using the Department of Energy's (DOE's) Energy Sciences Network (ESnet). By linking capabilities at the National Renewable Energy Laboratory (NREL) and the Idaho National Laboratory (INL), the researchers created a collaborative "SuperLab," which allowed them to study energy systems currently not in existence. In this case, they demonstrated that renewable and nuclear energy, combined within a hybrid system, can complement each other well to support the grid.
"Integrating nuclear assets deployed at INL and connecting them with renewable energy assets at NREL showcases the power of energy hybridization technology and underscores the importance of connectivity in achieving sustainable energy solutions," said Rob Hovsapian, ARIES research lead in hybrid energy systems at NREL. "Innovation without implementation is merely an idea, but at-scale validation is the bridge that makes ideas a reality. The Advanced Research on Integrated Energy Systems (ARIES) platform at NREL is the engine that powers this evolution, connecting multiple assets and de-risking complex energy systems for faster adoption of novel clean energy technologies."
A Virtual Hybrid Plant
The SuperLab demonstration successfully linked energy grid and power production simulations from two laboratories:
- At NREL (Golden, Colorado), the ARIES platform provided a solar array, battery storage system, hydrogen fuel electrolyzer, and a controllable grid interface. Digital real-time simulators enabled the researchers to connect the models and responses on both NREL and INL sides.
- At INL (Idaho Falls, Idaho), researchers readied simulations of a small modular nuclear reactor and high-temperature electrolysis in the Human Systems Simulation Laboratory (HSSL).

Left: INL's Human Systems Simulation Laboratory. Photo from INL . Right: NREL's ARIES platform. Photo by NREL . These two capabilities were connected via ESnet6 OSCARS circuits creating a virtual hybrid renewable-nuclear plant.
ESnet-operated fiber-optic cabling provided high-speed, low-latency, and low-jitter data connections between the two laboratories. This connection synchronized simulations and control signals, providing "virtual proximity" of the assets.
This SuperLab demonstration followed months of preparation by several dozen researchers at INL, NREL, and ESnet. The demonstration was attended by over 60 energy experts, including representatives from other national laboratories and DOE representatives from the Office of Science, the Office of Nuclear Energy, the Office of Electricity, and the Office of Energy Efficiency and Renewable Energy.
Text version
Renewable-Nuclear Hybrid: A Complementary Pairing
The SuperLab demonstration showed that nuclear power and renewables could be used in combination for the electric grid. Nuclear reactors operate best in a steady state as a source of baseload power but cannot respond quickly to changes in demand. Wind and solar power can provide intermittent power but are not always dispatchable. Together, they provide stable power during abrupt changes in demand or weather conditions. And for an extra-functional design, the researchers added hydrogen electrolyzers and thermal batteries to store excess power.
"A hybrid plant that incorporates both nuclear and renewable assets allows us to leverage the unique benefits offered by each of these clean energy technologies," said Shannon Bragg-Sitton, Integrated Energy & Storage Systems Division director at INL. "It ensures that grid demands are met reliably and affordably at all times while taking advantage of the heat provided by a nuclear thermal generator to produce clean hydrogen and support the decarbonization of industry."
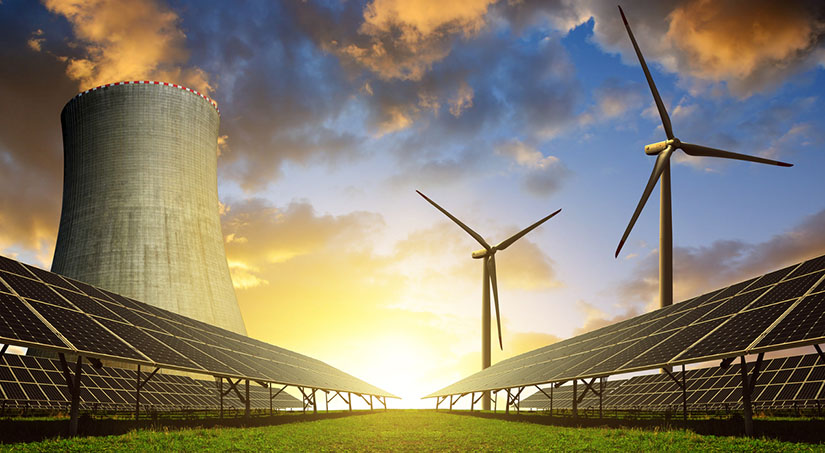
During the demonstration, the researchers found that their hybrid plant performed as desired. First, they simulated a sudden loss in solar power from a passing cloud, and the nuclear reactor stepped in to support grid demand. Then, when they simulated a storm knocking out neighborhood power lines, the nuclear reactor ramped down its power to the grid and redirected it to increasing hydrogen production and storage. These scenarios provide developers a baseline and high-quality operational data for how hybrid renewables-nuclear designs might operate together for a reliable power grid.
"Demonstrating integrated use of diverse generation assets in a controlled experimental facility allows us to better understand how these systems can mutually support a varying energy demand before major investments are decided," Bragg-Sitton said. "These demonstrations can emulate performance under both expected and off-nominal conditions to gain confidence in their operations. Through this SuperLab, each laboratory in the DOE complex brings unique expertise to the challenge of clean energy systems of the future."
Not the First SuperLab
The January demonstration was not just an achievement for hybrid power plants, as it also made strides for the network backbone supporting SuperLab: ESnet. This demonstration was one of several, with more ahead, to connect megawatts of power hardware using ESnet. In 2017, eight laboratories connected for the first demo using virtual private network connections, shown in the video below. It was a successful proof of concept, but varying latency made it difficult to cosimulate power signals requiring millisecond sensitivity.
The ESnet team reduced latency variance, bringing it down from 11.5 milliseconds to 0.02 milliseconds, which was useful in 2021 when another SuperLab was assembled to support a remote Alaskan city with its microgrid controls, shown in the video below.
During the January demonstration, the researchers were treated to another improvement when ESnet6 was unveiled, which features higher data capacity, real-time data visualization, and new automation and cybersecurity tools. It is the latest improvement to an extraordinary scientific platform that has steadily grown since 1986.
"ESnet is proud to support the ARIES project," said Eli Dart, Science Engagement Group lead at ESnet. "These demonstrations effectively use the OSCARS virtual circuit capabilities of ESnet6. ARIES requires low jitter and deterministic behavior, which OSCARS can provide over dedicated point-to-point connections. Together, ESnet and the ARIES team worked on both demonstrations, and we look forward to continuing this collaboration in the future."
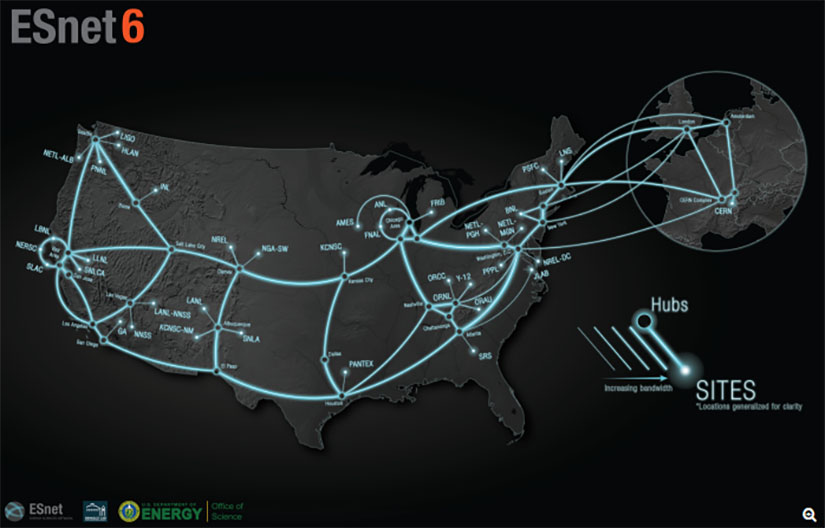
From its headquarters at Lawrence Berkeley National Laboratory, ESnet branches and converges at laboratories around the nation, reaching many powerful research nodes that include several in Europe. It is really a continental laboratory, with NREL and INL's experiment as one small (but no less super) link; meanwhile, other researchers using ESnet are synchronizing genetic data and simulating quantum materials. ESnet brings their physical capabilities closer together so that laboratories can share their supercomputers, particle reactors, and wind turbines, regardless of their geographic location.
"As ARIES scales up to thousands of devices and many more laboratories, we are excited to be a part of this team," Dart said. "The goal is to ensure that the U.S. has a power grid capable of reliably integrating the modern energy technologies we need for the 21st century. Codesign with the SuperLab program allows ESnet to ensure our advanced network supports the success of power grid research. Bringing together the complementary expertise of the national labs is what we do best—everyone brings their best game, and we all work together."
The renewable-nuclear hybrid demo also provided something in return to ESnet: a blueprint for how to build similar experiments. The next experiment is already in the works since NREL, INL, and other laboratories have already validated and established their SuperLab connections.
More Power, More Devices, and a Major Outage
In the spirit of resilience and with an interest in pushing the limits, the next SuperLab demonstration will simulate a national-scale disaster across eight national laboratories. The researchers plan to study how a major outage from a hurricane or cyberattack would play out on a distributed energy system. The scale of this experiment will be much greater than anything before; while the 2017 demo ran 80 devices, the upcoming demo, scheduled for late 2023, will aim for 10,000 devices.
Once the demonstration is complete, it will be a milestone for the SuperLab concept: The research community will have the capability to emulate national-scale scenarios on real power hardware, helping to reduce risk for future energy transition strategies. And that is just the start of what can be achieved when all U.S. research capabilities are combined.
"By connecting multiple labs through ESnet by 2024, we'll unlock the full potential of our national laboratories, their research assets, cutting-edge technologies, and talented scientists," Hovsapian said. "This SuperLab 2.0 infrastructure will prepare us to address large-scale emergent challenges to meet the nation's clean energy goals and to reinforce the energy security needs of every community."
Learn more about NREL's ARIES platform for power system emulation or contacting Rob Hovsapian or Steve Hammond .
Numbers, Facts and Trends Shaping Your World
Read our research on:
Full Topic List
Regions & Countries
- Publications
- Our Methods
- Short Reads
- Tools & Resources
Read Our Research On:
Majority of Americans support more nuclear power in the country
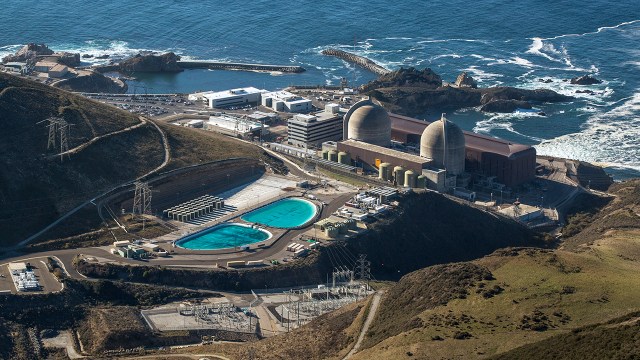
A majority of U.S. adults remain supportive of expanding nuclear power in the country, according to a Pew Research Center survey from May . Overall, 56% say they favor more nuclear power plants to generate electricity. This share is statistically unchanged from last year.
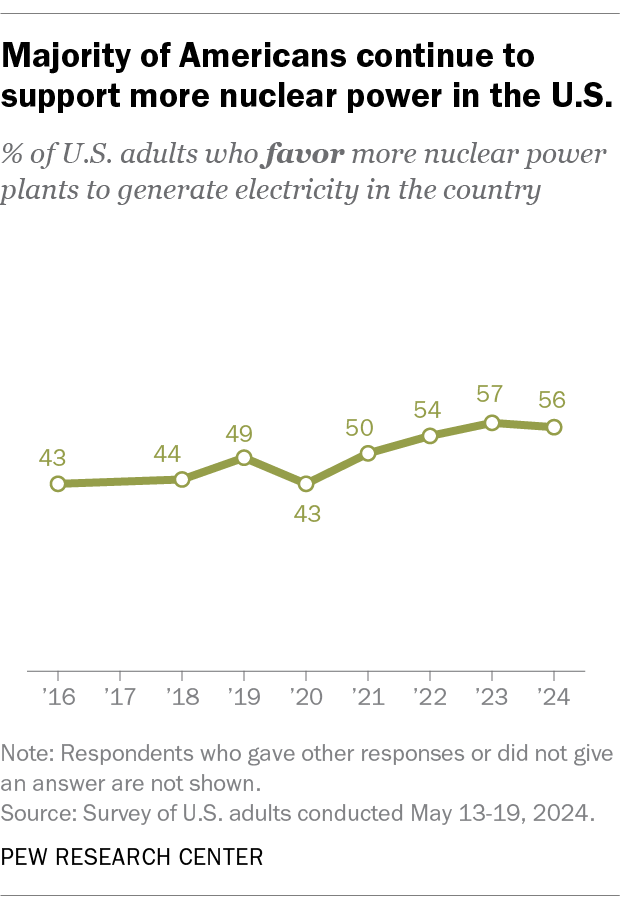
But the future of large-scale nuclear power in America is uncertain. While Congress recently passed a bipartisan act intended to ease the nuclear energy industry’s financial and regulatory challenges, reactor shutdowns continue to gradually outpace new construction.
Americans remain more likely to favor expanding solar power (78%) and wind power (72%) than nuclear power. Yet while support for solar and wind power has declined by double digits since 2020 – largely driven by drops in Republican support – the share who favor nuclear power has grown by 13 percentage points over that span.
When asked about the federal government’s role in encouraging the production of nuclear energy, Americans are somewhat split. On balance, more say the government should encourage (41%) than discourage (22%) this. But 36% say the government should not exert influence either way, according to a March 2023 Center survey .
To measure public attitudes toward the use of nuclear power in the United States, we analyzed data from Pew Research Center surveys. Most of the data comes from our survey of 8,638 U.S. adults conducted May 13-19, 2024.
Everyone who took part in the survey is a member of the Center’s American Trends Panel (ATP), an online survey panel that is recruited through national, random sampling of residential addresses. This way, nearly all U.S. adults have a chance of selection. The survey is weighted to be representative of the U.S. adult population by gender, race, ethnicity, partisan affiliation, education and other categories. Read more about the ATP’s methodology .
Here are the survey questions used for this analysis , along with responses, and its methodology .
Links to related Center surveys, including their questions and methodologies, can be found throughout the post.
In addition, we tracked the number of U.S. nuclear power reactors over time by analyzing data from the International Atomic Energy Agency’s (IAEA) Power Reactor Information System . The IAEA classifies a reactor as “operational” from the date of its first electrical grid connection to the date of its permanent shutdown. Reactors that face temporary outages are still categorized as operational. Annual totals exclude reactors that closed that year.
Views by gender
Attitudes on nuclear power production have long differed by gender.
In the May survey, men remain far more likely than women to favor more nuclear power plants to generate electricity in the United States (70% vs. 44%). This pattern holds true among adults in both political parties.
Views on nuclear energy differ by gender globally, too, according to a Center survey conducted from fall 2019 to spring 2020 . In 18 of the 20 places surveyed around the world (including the U.S.), men were more likely than women to favor using more nuclear power as a source of domestic energy.
Views by party

Republicans are more likely than Democrats to favor expanding nuclear power to generate electricity in the U.S. Two-thirds of Republicans and Republican-leaning independents say they support this, compared with about half of Democrats and Democratic leaners.
Republicans have supported nuclear power in greater shares than Democrats each time this question has been asked since 2016.
The partisan gap in support for nuclear power (18 points) is smaller than those for other types of energy, including fossil fuel sources such as coal mining (48 points) and offshore oil and gas drilling (47 points).
Still, Americans in both parties now see nuclear power more positively than they did earlier this decade. While Democrats remain divided on the topic (49% support, 49% oppose), the share who favor expanding the energy source is up 12 points since 2020. Republican support has grown by 14 points over this period.
While younger Republicans generally tend to be more supportive of increasing domestic renewable energy sources than their older peers, the pattern reverses when it comes to nuclear energy. For example, Republicans under 30 are much more likely than those ages 65 and older to favor more solar panel farms in the U.S. (80% vs. 54%); there’s a similar gap over expanding wind power. But when it comes to expanding nuclear power, Republicans under 30 are 11 points less likely than the oldest Republicans to express support (61% vs. 72%).
A look at U.S. nuclear power reactors
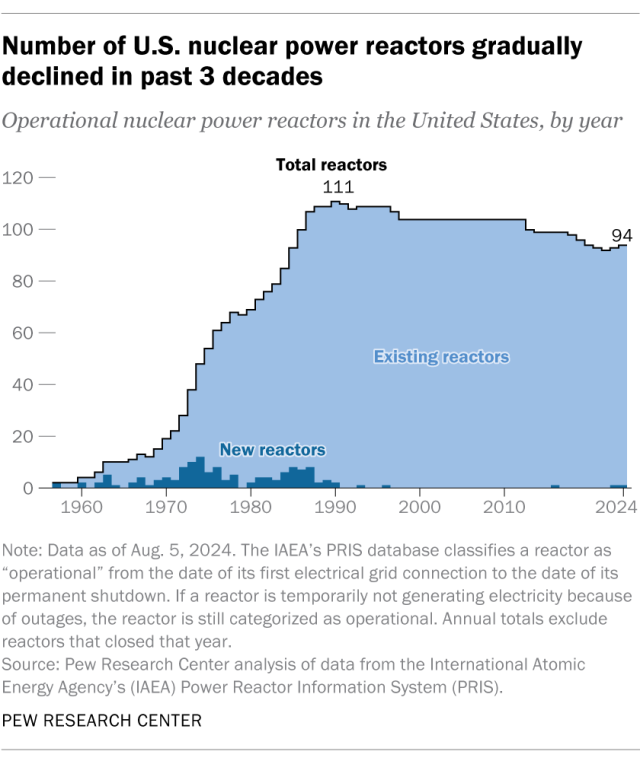
The U.S. currently has 94 nuclear power reactors, including one that just began operating in Georgia this spring. Reactors collectively generated 18.6% of all U.S. electricity in 2023 , according to the U.S. Energy Information Administration.
About half of the United States’ nuclear power reactors (48) are in the South, while nearly a quarter (22) are in the Midwest. There are 18 reactors in the Northeast and six in the West, according to data from the International Atomic Energy Agency (IAEA).
The number of U.S. reactors has steadily fallen since peaking at 111 in 1990. Nine Mile Point-1, located in Scriba, New York, is the oldest U.S. nuclear power reactor still in operation. It first connected to the power grid in November 1969. Most of the 94 current reactors began operations in the 1970s (41) or 1980s (44), according to IAEA data. (The IAEA classifies reactors as “operational” from their first electrical grid connection to their date of permanent shutdown.)
Within the last decade, just three new reactors joined the power fleet. Three times as many shut down over the same timespan.
One of the many reasons nuclear power projects have dwindled in recent decades may be the perceived dangers following nuclear accidents in the U.S. and abroad. For example, the 2011 Fukushima Daiichi accident led the Japanese government to greatly decrease its reliance on nuclear power and prompted other countries to rethink their nuclear energy plans . High construction costs and radioactive waste storage issues are also oft-cited hurdles to nuclear energy advancement.
Still, many advocates say that nuclear power is key to reducing emissions from electricity generation. There’s been a recent flurry of interest in reviving decommissioned nuclear power sites, including the infamous Three Mile Island plant and the Palisades plant , the latter of which shuttered in 2022. Last year, California announced it would delay the retirement of its one remaining nuclear power plant until 2030. And just this summer, construction began on a new plant in Wyoming. It’s set to house an advanced sodium-cooled fast reactor, pending approval from the Nuclear Regulatory Commission .
Note: Here are the questions used for the analysis , along with responses, and its methodology . This is an update of a post first published March 23, 2022.
- Climate, Energy & Environment
- Partisanship & Issues
- Political Issues
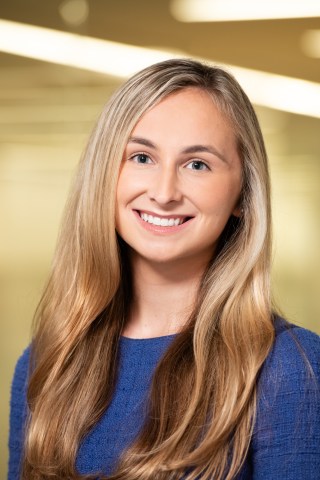
Rebecca Leppert is a copy editor at Pew Research Center .
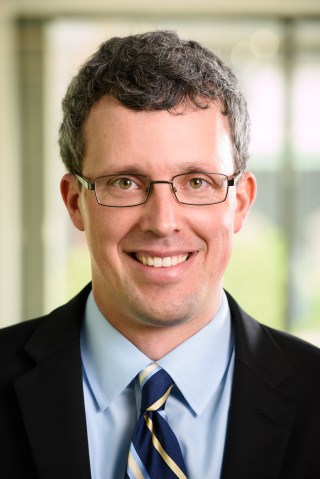
Brian Kennedy is a senior researcher focusing on science and society research at Pew Research Center .
Americans’ Extreme Weather Policy Views and Personal Experiences
U.s. adults under 30 have different foreign policy priorities than older adults, about 3 in 10 americans would seriously consider buying an electric vehicle, how americans view national, local and personal energy choices, electric vehicle charging infrastructure in the u.s., most popular.
901 E St. NW, Suite 300 Washington, DC 20004 USA (+1) 202-419-4300 | Main (+1) 202-857-8562 | Fax (+1) 202-419-4372 | Media Inquiries
Research Topics
- Email Newsletters
ABOUT PEW RESEARCH CENTER Pew Research Center is a nonpartisan fact tank that informs the public about the issues, attitudes and trends shaping the world. It conducts public opinion polling, demographic research, media content analysis and other empirical social science research. Pew Research Center does not take policy positions. It is a subsidiary of The Pew Charitable Trusts .
© 2024 Pew Research Center
REVIEW article
Solar photovoltaic energy optimization and challenges.
- 1 Department of Electrical Engineering, Mehran University of Engineering and Technology, Jamshoro, Pakistan
- 2 Processes, Energy, Environment and Electrical Systems, University of Gabes, Gabes, Tunisia
- 3 Faculty of Electrical and Control Engineering, Gdańsk University of Technology, Gdańsk, Poland
- 4 Department of Materials Technologies, Silesian University of Technology, Gliwice, Poland
The study paper focuses on solar energy optimization approaches, as well as the obstacles and concerns that come with them. This study discusses the most current advancements in solar power generation devices in order to provide a reference for decision-makers in the field of solar plant construction throughout the world. These technologies are divided into three groups: photovoltaic, thermal, and hybrid (thermal/photovoltaic). As a result, this article begins by outlining the approach that will be employed to undertake this research. Following that, solar energy production methods are researched and their sub-classifications are described in order to establish their resource needs and features. Following that, a detailed conversation is held. Each technology’s environmental and economic performance will be evaluated. Furthermore, a statistical analysis is conducted to emphasize the efficiency and performance of each solar technology, as well as to identify their global rankings in terms of power output. Finally, research trends in the development of solar power plants are presented. The credibility of the Photovoltaic system, types and limitations is the discussion under study system makes use of sun’s energy to generate electricity with the help of varied procedural systems; stand-alone, hybrid or grid charged. Based on this research, it is possible to infer that the primary goals of optimization approaches are to reduce investment, operation and maintenance costs, and emissions in order to improve system dependability. This paper also includes a brief overview of several solar energy optimization problems and issues.
1 Introduction
Global warming is an element in climate change and explicitly refers to the influence of greenhouse gases on the overall surface temperature of the Earth. When describing extreme weather events produced by greenhouse gases; the label “global warming” is appropriate. When characterizing other long-term changes to the planet’s weather patterns, however, climate change is the most appropriate phrase. Opponents of climate change and global warming have noted out how the Earth’s temperature patterns have fluctuated for generations, and that current climatic changes are not as severe as stated, nor are they only the consequence of human activity.
The Earth’s atmosphere is made up of several gases that work as a layer, trapping heat from the sun and blocking it from escaping back into space. Human actions have contributed to rising global temperatures, according to 97% of active climate experts throughout the world. According to climate experts, such negativity arises from a fear of confronting the scale of the harm caused by human actions to the environment. Little fluctuations in the Earth’s orbit around the Sun enable the ice sheets to develop and disappear. Solar radiation levels fluctuate. Such changes have a wide range of consequences in space, the Earth’s atmosphere, and on the Earth’s surface ( Mohamed et al., 2014 ). Upshot changes in solar activity, according to current scientific opinion, have only a little role in the Earth’s temperature. The warming induced by increasing levels of man-made greenhouse gas emissions is several times more than any other factors: Recent changes in solar activity are to blame. In fact, solar energy is a lot more beneficial for human beings. As the modern technological world is getting updated day by day. There is dire need to find a credible energy source in order to ensure a promising ground. In terms of solar energy, the sun is the most major source which can turn into feasible means if it is used to produce photovoltaic energy. Photovoltaic energy can be produced with the help of solar energy and is converted into electricity with the aid of solar photovoltaic panels.
Many activities rely on solar energy. Pumping water is mostly used in agriculture. PV panels and electric batteries are utilized to power the electro-pumps, allowing the irrigation system to be completely self-sufficient. In the construction sector, solar energy is used for air conditioning, water heating, lighting, and refrigeration systems. Desalination of water is another key application of solar energy. Solar energy is utilized to extract low-salt water from saltwater in this technique. Telecommunications is another key sector that makes use of solar energy. Satellites’ electrical demands are met by solar panels installed on their spinning limbs. Solar energy is occasionally utilized as a backup power source for established telecommunications networks. Hydrogen generation and consumption by electrolysis of water is one of the most promising ways to achieving carbon neutrality by 2050. Figure 1 shows the typical Photovoltaic system. Solar energy has shown to be the most cost-effective and environmentally friendly option for electrolysis procedures. For power generation, three primary technologies are used, namely thermal, photovoltaic, and hybrid thermal photovoltaic. Numerous nations have already implemented similar systems in their electrical grids, including the United States, Spain, Morocco, India, China, and. Furthermore, in order to select the most appropriate technology for a specific country, a thorough examination and knowledge of the many solar technologies and their underlying challenges is required to assist responsible institutions in making decisions. As a result, a comprehensive assessment of all solar technologies for energy generation is required. As a result, the purpose of this study is to cover several research gaps in the literature, such as the absence of statistical analysis of existing solar power plants throughout the world. Moreover, decision-makers will be able to implement the most appropriate solar power technology for a specific geographic region. The second gap in the literature concerns a recent comprehensive study of solar energy technology for power generation. The third significant research gap is an in-depth comparison of the performance of the three primary solar technologies and their modifications, which, to the best of the authors’ knowledge, has yet to be addressed in any contemporary study.
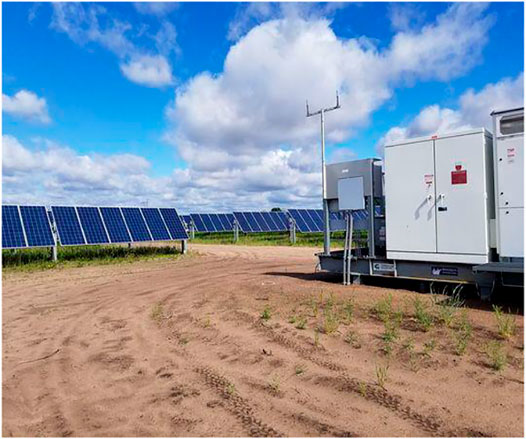
FIGURE 1 . Photovoltaic system (Flickr).
As a result, the following are the primary additions and innovations of the present article:
• A new summary of the three primary solar methods for generating power.
• Updated solar technology economic and environmental assessments.
• Audit of linear Fresnel reflectors, parabolic trough technology, Parabolic dish collectors, Heliostat field collectors, photovoltaic, and concentrated photovoltaic solar power plants.
• PV-CSP and PVT/CPVT are two hybrid systems for generating thermal solar electricity ( Getie et al., 2020 ).
The layout of this paper is as follows. Section methodology introduces the adopted methodology in this review paper. Section Technologies Overview for Generating Thermal Power describes the three main solar technologies for electricity production. The discussion and comparison of these technologies alongside future trends and evaluation of their environmental and economic aspects are conducted in section Discussions. Section Optimization Method discuses PV Base Hybrid System, PV Based Grid System and PV Based Standalone System. Section Utilization of Solar Photovoltaic Energy discusses application. Section Optimization Issues and Challenges highlight limitations, while Section Conclusion , provides the conclusion of this paper ( Bishoyi and Sudhakar, 2017 ).
2 Methodology
An accurate literature study was undertaken to assess the most recent relevant research and their conclusions in order to investigate solar technologies for power production. These latter have been investigated based on the accepted solar technologies, their working principle, their capabilities, and the environmental difficulties linked with them. Moreover, an analysis of the operating solar-powered power plants has been created. Finally, a comparison of all technologies is offered in terms of their advantages, efficiency, and resource needs. The paper covers an exact literature study to assess the most recent relevant research and their conclusions in directive to solar energy technology for electricity generation built on the solar techniques employed, their operating principles, and their performance. A list of all operational solar-powered power plants has also been established. A comparison of technologies in terms of their advantages, productivity, and reserve needs is presented as well. The review of literature is divided into three parts, the first of which was to gather the most recent information on the four essential technologies used in thermal solar stations ( Zhang et al., 2013 ) as follows:
• Parabolic trough collector (PTC) ( Ouagued et al., 2018 ).
• Linear Fresnel Reflector (LRF) ( Ghodbane et al., 2016 ).
• Heliostat field collector (HFC) ( Eddhibi et al., 2017 ).
• Parabolic dish collector (PDC) ( Chen et al., 2018 ).
Second part splits research into two areas based on the major technology used in solar power plants:
• Fundamentally as Photovoltaic (PV) ( Lokar and Virtic, 2020 ).
• Concentrated photovoltaic (CPV) ( Aqachmar et al., 2020 ).
Third part, the technologies used in hybrid thermal photovoltaic systems are investigated. Including:
• Photovoltaic thermal (PVT) ( Aqachmar et al., 2020 ).
• Concentrated photovoltaic thermal (CPVT) ( Bamisile et al., 2020 ).
Next section shows a detailed literature review in order to shed light on different optimization methods in terms of solar photo- voltaic energy. Furthermore, an overview on utilization of photovoltaic energy is presented. In the last step the cons of optimization methods are discussed in terms of challenges and issues to get a better understanding of debilitated points of this whole phenomenon.
2.1 Technologies Overview for Generating Thermal Power
DNI (Direct Normal Irradiance) is used to turn sunlight into electricity, solar thermal power uses the second principle of thermodynamics. This transition necessitates the use of two heat sources: a cold and a hot source. The heat transfer fluid (HTF) is employed as the hot source and water as the cold source in CSP power plants. Entropy is increased as a result of the natural heat exchange between water and HTF. After that, the HTF is heated using one of four different technologies: parabolic trough collectors, Fresnel reflectors, parabolic dish collectors, or solar power tower ( Alsaffar, 2015 ).
2.1.1 Plants Using PTC Technology
A solar field, a power block, and thermal energy storage (TES) are all parts of the PTC power plant. In the solar field, solar collectors with parabolic troughs and tubes filled with a heat transfer fluid (HTF) are employed. By way of it passes through the tubes, a reflected beam of solar light heats the HTF. The power block must be efficient and trustworthy because it is the core of the PTC system. As a result, Rankine or Hirn cycles are the most often used power blocks ( Zhar et al., 2021 ; Aqachmar et al., 2019 ). PTC power plants are already operational in 98 countries, with 43% in Spain, 7% in India and 17% in the United States. The capacity of these PTC plants between 0.15 MW in France to 2,474.5 MW in Spain ( Solar paces, 2019 ; Boukelia et al., 2017 ). As indicated in Table 1 , the LCOE of PTC power plants ranges from 0.07 to 0.23 USD/KWh, and is heavily impacted by the plant’s position (DNI), size (Capacity in MegaWatts), and TES time. This table covers a number of LCOE optimization studies were carried out for a number of PTC plants in various countries ( Boukelia, et al., 2017 ; Dowling et al., 2017 ; Aly et al., 2019 ; Achkari and El Fadar, 2020 ).
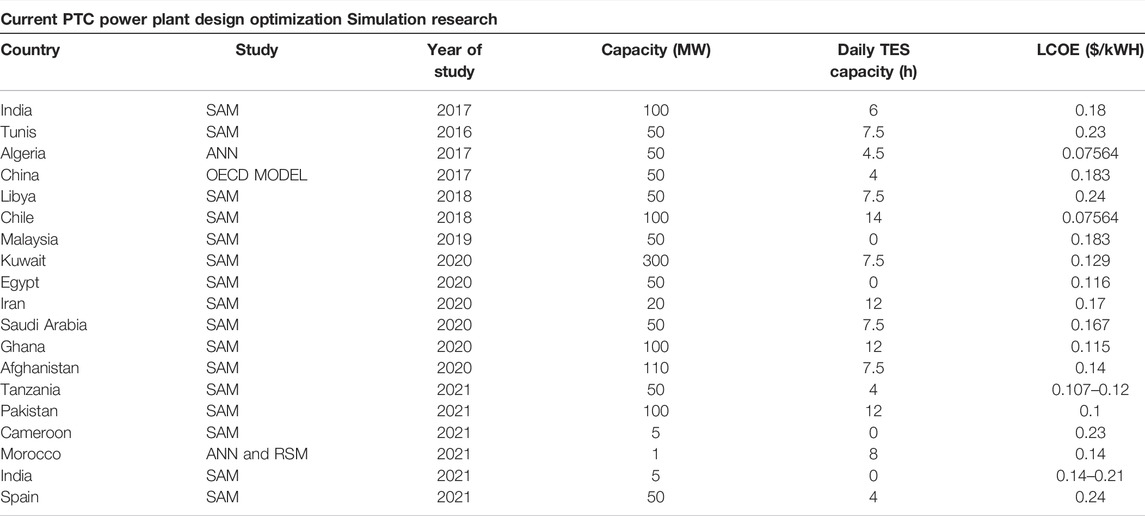
TABLE 1 . Current PTC power plant design optimization simulation research ( Zineb et al., 2021 ).
2.1.2 Heliostat Field Collector or Solar Power Tower
Large mirrors reflect the sun’s energy onto a receiver at the top of a tower in heliostat field collector power plants. Ceramics or any other physical substance that is stable at high temperatures is used to construct the receiver. The heat is subsequently transferred to the HTF, which, in turn, is used to generate electricity, when it reaches a particular temperature, activates the steam generating system. The focused solar radiation must reach the receiver at a rate of 200–1,000 kW/m 2 ( Simsek et al., 2018 ) to produce the required temperature for the procedure. In general, water, melted salt, sodium liquid or air, can be used as the HTF in SPT technology. Economic and technological research on SPT power plants have grown in popularity in recent years. This would include viability and optimization research, which necessitate an assessment of the power plant’s three components: the heliostat field (tower altitude, land-use factor, as well as the quantity, length, and width of separate mirrors (heliostats)), heat energy storing (area for storage, storage extent, storing capacity), and power generation, vessels, temperature levels), ( Chen et al., 2018 ; Simsek et al., 2018 ; Collado and Guallar, 2019 ; Zhuang et al., 2019 ; Awan et al., 2020a ; Agyekum and Velkin, 2020 ; Hakimi et al., 2020 ), and electricity cycle (thermal cycle, fluid transmit, effectiveness, boiler stress, etc.). Awan et al. (2020b) obtained a 35.6% gain when compared to the initial design, full load storage period (TES) required for multi-objective optimization, tower elevation, and SM resulted in a 35.6% increase in energy competence and a 16.9% drop in LCOE. Zhuang et al. (2019) showed a cost-benefit analysis of 100 MW SPT power stations in China utilizing various melted salts and anticipated that the LCOE in China will fall from 0.23 $/kWh in 2017 to 0.10 $/kWh by 2050. In a recent study from Chile looked at the impact of solar extinction on LCOE ( Marzo et al., 2021 ).
2.1.3 Solar Thermal Power Plant With a Linear Fresnel Solar
An absorber, a steam generation system (SGS), a tracking system, and an instrumentation system all employ a collection of Fresnel reflectors built of linear mirrors ( Ghodbane et al., 2019 ). LFR flat mirrors reflect the sun’s straight normal irradiance (or ray radiation) towards absorber surface ( Islam et al., 2018 ). As a result of the strong sun radiation, the water vaporizes. The steam turbine is spun by the evaporated water, which subsequently generator to produce electricity by rotating, thanks to the high pressure. As demonstrated in the study of a 120 MW LFR power plant in the El-Oued region ( Alotaibi et al., 2020 ) (LCOE 14 0, 0382$/kWh; avoided CO 2 14,420, 67 tCO 2 /year) and in India ( Bishoyi and Sudhakar, 2017 ) for countries with significant water stress, Fresnel reflector-based power production technology is a very promising and low-cost technology. However, when compared to other technologies, particularly PTC ( Bellos, 2019 ), in the solar industry, LFR power plants undergo from considerable optical losses. Sanda et al. (2019) gave a thorough review of thermal modeling and visual simulation tools for LFR power plant design. The prices of 50 MW LFR power plants are compared to PTC and SPC power plants in Table 2 with equivalent capacity in India’s diverse climatic zones ( Kumar et al., 2021 ). Several researches on various aspects of LFR power plants, the thermal energy storage system, for example, have been installed to improve the plant’s efficiency. By segregating the TES system from the rest of the system, into numerous modules ( Tascioni et al., 2020 ), established a fresh optimization strategy. This resulted in a 13% improvement in TES efficiency and a 30% reduction in solar field thermal loss. Lopez et al. (2020) were also able to improve the economic and energetic performance of an Iranian power plant by adopting phase change material (PCM) as the storage system.
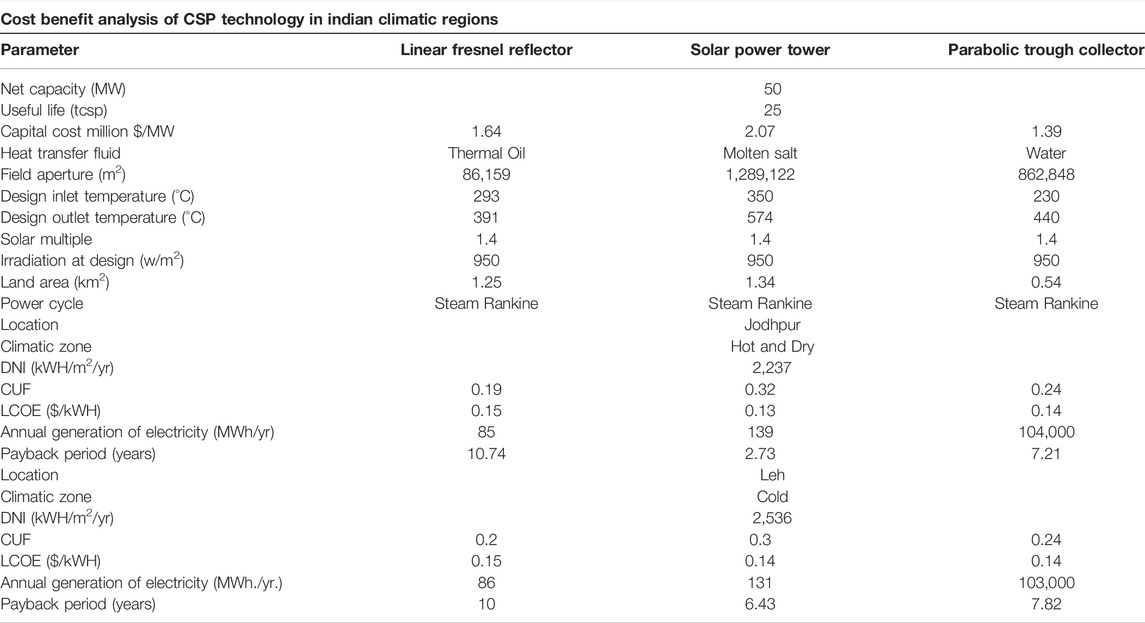
TABLE 2 . Cost-benefit analysis of CSP technology in Indian climatic regions ( Zineb et al., 2021 ).
2.1.4 Power Plants for Parabolic Dish Collectors
The parabolic dish collector (PDC) is a technique that directs solar energy beams gathered by a dish-shaped concentrator to a receiver at its focal point. Flat and cavity receivers are the two types of receivers. To track the direct normal irradiation, the concentrator uses a two-axis tracker. For optimal use of the obtained focused heat, at the focus point, an electrical generator with a Stirling-Brayton mechanism is placed ( Islam et al., 2019 ). When the ratio of concentrations surpasses 3000 ( Islam et al., 2018 ; Lopez et al., 2020 ), the pressure and temperature in the receiver might reach dangerous levels 200 bar and 700–750°C, respectively.
2.2 Photovoltaic Solar Energy Technologies Are Used to Generate Solar Power
2.2.1 pv technology.
PV technology is frequently used because to its simplicity in power generation. As seen in Figure 2 according to this, China (36%) leads the world in PV installed capacity, followed by the United States (13%), and Japan (11%) ( IEA, 2020 ). China is generating more than 175.01 GigaWatts of PV power, with the United States and Japan 62.2 GigaWatts, 55.5 GigaWatts coming in second and third, respectively.
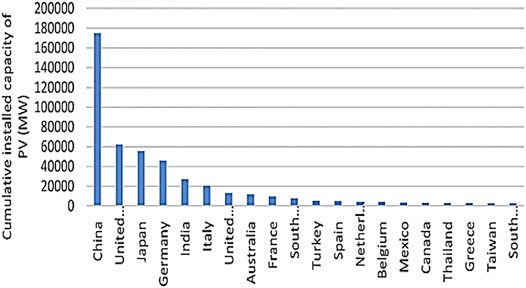
FIGURE 2 . Installed capacity of PV (MW) ( Zineb et al., 2021 ).
2.2.2 Concentrated PV Technology
Concentrated PV (CPV) cells were developed as a result of scientific advancements in the optical instruments field. The CPV focuses sunbeams onto PV cells with the help of utilizing an optical concentrator, such as a curved mirror or a lens. The solar cells’ efficiency improves as a result of the additional photons focused with the concentrator. Adding a concentrator to a cell, according to the literature, enhances the current generated by the cell and improves the efficiency of the cell operating voltage ( Gonzalez-Longatt, 2005 ; Luque and Hegedus, 2011 ). Appropriate concentration technology selection is critical because the performance of the CPV’s optics has a direct and significant impact on the CPV’s efficiency. As a result, Table 2 provides a detailed summary of various concentration schemes. Two (or more) concentration systems are sometimes combined to improve the efficiency of CPV systems. Figure 3 shows the total electricity generated by different countries utilizing CPV. CPV’s installed power ranges up till 2021 from 114 kW in Japan to 67.68 MW in China region. Concerned nations’ typical CPV output is around 22.43 MW. Moreover, China has half (50%) of the mounted CPV volume, tracked the United States having 21%. High concentrated PV (HCPV) accounts for 81% of installed CPV power plants, while low concentrated PV (LCPV) accounts for 19%. As a result, HCPV generates 93% of the produced electricity, whereas LCPV generates just 7%.
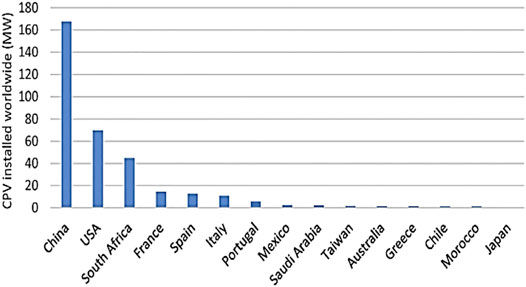
FIGURE 3 . Total Installed CPV worldwide ( Zineb et al., 2021 ).
2.3 Hybrid Solar Thermal Power Generating Technology
Decoupled Photovoltaic and connected PV-CPV/CSP are the two types of hybrid solar power systems. These are systems that combine the two technologies on one worksite. Photovoltaic thermal collector (PVT) or concentrated photovoltaic thermal collector (CPVT) systems are dense or coupled hybrid solar systems that combine CPV/PV and CSP into a sole system are the second type of hybrid solar systems.
2.3.1 Hybrid PV-CSP Technology
PV-CSP hybrid expertise is relatively different topic which has piqued the curiosity of scientists all over the world. By expanding hybrid power plants on large scale, this is especially promising, according to the IEA’s solar thermal energy technology roadmap. The fascinating properties of such a combo, that can enhance system stability, enhance energy quality, cut LCOE, limit heat losses, and increase efficiency of power plant, driving this decision. The strategy for hybrid PV-CSP power plants on a large scale includes a battery energy storage system i-e (BESS) plants in recent research. The expected drop in battery costs may make this option more realistic in future. Several modeling researches dedicated to hybrid decoupled PV/CSP systems are included in Table 3 ( Gaga et al., 2017 ; Ju et al., 2017 ; Moukhtar et al., 2021 ). Conventional PV-thermal hybrid solar systems. A standard photovoltaic module is combined by a thermal accumulator in the PVT solar system to harvest sun energy. Several investigations have been conducted into this ( Hissouf et al., 2020 ).
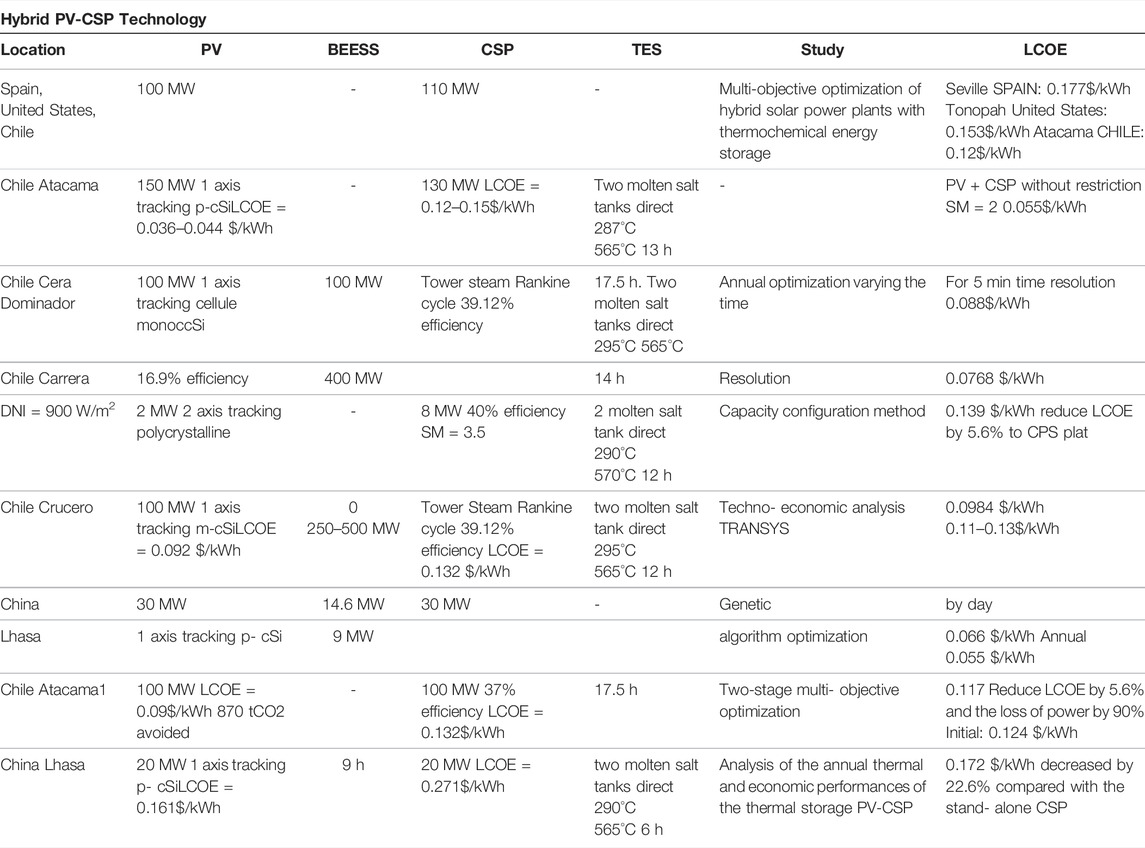
TABLE 3 . Hybrid PV-CSP technology ( Zineb et al., 2021 ).
PV-thermal hybrid solar systems with concentrated photovoltaic. CPV technology, despite its high conversion ratio, is not without flaws, has serious flaw: excessive PV cell heating. A cooling technique is essential to avoid this. In this case, incorporating a thermal procedure achieve dual goals of freezing the CPV cells while also producing valuable thermal heat. The development of hybrid CPV-thermal technology was sparked by this.
2.3.2 Discussion of Thermal Methods for Energy Generation
Thermal technology for energy generation may be split into four groups, according to the review: solar power tower, parabolic dish collector, parabolic trough collector, linear Fresnel reflector, and the parabolic dip is the most commonly used solar thermal technique, having 64 percent of all CSP installation units in operation. Following that is tower technology, which accounts for 31% of all CSP technology installed globally. On either side, because PDC and LFR are two separate entities, they are relatively new technologies with considerable obstacles to overcome, their contribution to the energy mix are fairly limited. Because the performance of each technology is influenced by a variety of elements such as geographic location and HTF used, selecting one technology over another for a given place should be based on these features. The conclusions of this study revealed the barriers to widespread CSP implementation. PDC, for example, is expensive; PTC has low conversion efficiency; and LFR devices have a restricted operating temperature. Furthermore, the significant both the SGS and the cooling process make use of water is a serious issue with CSP facilities. The focus on resolving these difficulties will improve CSP plant acceptability in the background of the global energy alteration.
2.3.3 Photovoltaic Technology Power Generation Discussion
Photovoltaic technologies for power generation are the focus of the present research. Depending on whether or not a concentrator is used, photovoltaic technology may be divided into two groups. Attempting to provide a simple summary of mounted CPV and PV systems across the world, regardless of the fact that CPV has a higher efficiency than PV, the accumulative capacity of CPV mounted globally is small, according to the statistics presented. The comparatively great prices of Concentrators and trackers for CPV explain this result ( Aqachmar et al., 2020 ) directed a viability evaluation of CPV large scale facilities and provided unique policy solutions for addressing the problem of high CPV device pricing. In addition ( Laarabi et al., 2021b ), looked at the soiling problem. In both Morocco and India, the authors reviewed a large amount of literature on PV soiling measurement methodologies, impacts, and cleaning approaches, further showed that soiling is a much localized process, with the position of the plants having a substantial influence on soiling.
2.4 Discussion of Hybrid Technologies to Produce Electricity
According to the findings of this study, hybrid photovoltaic thermal technology may be classed as either traditional PVT or concentrated PVT. The hybrid PV or CPV achieves a dual goal of cooling the PV cells, so increasing electrical output, and providing usable thermal heat for thermoelectric generators. It is worth noting that hybrid solar technologies, whether coupled or decoupled, are still in their infancy and will require more development before being utilized in large-scale power facilities. This covers, among other things, optimizing optical concentrators, water usage, and investment costs. Next section will cover solar photovoltaic energy system types and solar energy optimization method, issues and challenges ( Laarabi et al., 2021b ).
2.5 Types of Solar Photovoltaic Energy System
Figure 4 shows types of the solar photovoltaic systems which includes the most common configuration - a grid-connected PV system, which is used when customers want can reduce their energy costs, and the grid is accessible for using when the array PV is not generating electricity. A “Utility-Interactive PV System or Grid Tied PV System” is a PV-array without the need of a storage system; it is directly connected to the grid. Solar panels that generate part, if not all, of their power demands during the day while staying linked to the local electrical grid at night are included in these Connected Grid PV Systems. In most connected grid PV schemes, extra or surplus electricity is stored in batteries or sent back into the electrical grid. Solar energy can be utilized to meet some or all energy needs for those with a connected grid PV arrangement in their houses and buildings. Moreover, since this type of PV system is indefinitely linked to the grid, there is no need to calculate solar energy consumption or solar panel sizing, enabling for a variety of options, including a system as limited as 1.0 kiloWh on the tower to dramatically lessen your electricity bills, and a much bigger ground assembled array large enough just to totally eliminate your electricity costs completely. Hybrid PV systems are similar to stand-alone ones. The goal of a hybrid power system is to generate as much energy as possible from renewable sources while meeting load demand. An? AC or DC distribution system, a storing system, filters, converters, and a load management or supervisory system are all possible components of a hybrid system, in addition to energy sources. All of these elements related in a different ways. Depending on the system size, renewable energy sources can be linked to the DC bus. HPS systems can generate power ranging from just few watts for personal usage to very few megawatts for modest community electrification systems. As a result, DC loads are frequently supplied by hybrid systems used for extremely low-power applications. Commonly linked with more than 100 kW of power as well as an AC bus are intended to be a component of the system of massive interconnected networks. Furthermost hybrid systems like a UPS system, can serve as a backup power supply during a blackout due to their ability to store energy ( Georgescu-Roegen, 1979 ; Furkan and Mehmet Emin, 2010 ; Raturi, 2019 ). The word “hybrid” in the solar field refers to a system that employs a combination of solar and batteries and may interact with the power grid. The most cost-effective hybrid system employs a basic hybrid inverter, which includes a solar inverter and a battery inverter/charger, as well as smart controls that determine the most efficient practice of your available energy. PV system, stand-alone are suitable for sequestered rural areas and uses in which other sources of power are troublesome/nonexistent for powering lighting, applications, as well as additional equipment. This is frequently additional cost efficient for installing a solo stand-alone PV system to having local energy provider spread the power cables and lines directly towards the house as part of a grid-connected PV arrangement. A simple PV system is a self-contained solar expertise which produces electricity throughout the day to charge batteries for usage whenever the sun energy is absent at night. Rechargeable batteries are used to storing the electrical energy generated by panels (PV) or an arrangement in a stand-alone small-scale PV system ( Farh et al., 2018 ). The battery allows an independent photovoltaic system to run when the solar panel itself does not generate enough energy, because the size of the battery is proportional to the power previously consumed. The two main types of batteries used to store solar energy are deep cycle batteries and shallow cycle batteries. Batteries are necessary component on which every standalone self sufficient solar power system relies. It also transforms electric energy into chemical energy which then is stored for usage anytime the solar array is not generating electricity. The PV system provides direct electricity to the load during daylight hours, with any extra energy being stored in batteries for later use.
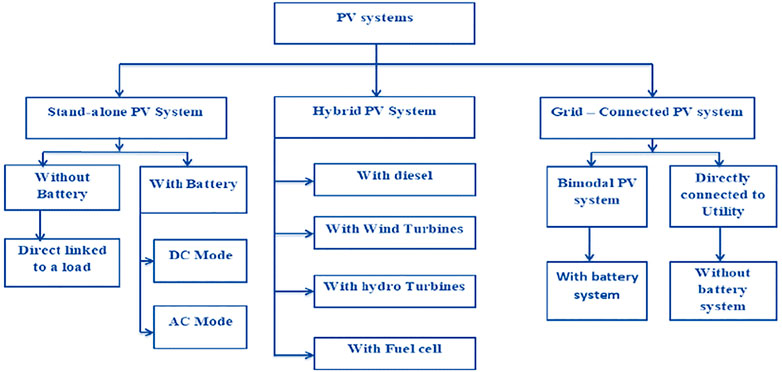
FIGURE 4 . Types of solar photovoltaic systems ( Assadeg et al., 2019 ).
3 Overview of Solar Energy Optimization Method
Solar energy systems emit no noise and produce no pollutants during operation and maintenance. Photovoltaic cell technologies have less environmental dangers than other forms of electric energy sources ( Otero et al., 1998 ). Chemicals used in the manufacture of PV cells, on the other hand, might be discharged into the air, surface water, and groundwater in the production plant, installation site, and disposal or recycling facility. The solar collector storage system may provide energy at temperatures greater than the ambient outside air. A significant quantity of CO 2 is emitted by a PV power plant based on single crystalline silicon technology. There was no pressing necessity for optimizing the energy balance of the production process in the so far very modest PV sector. The analysis of the affecting solar energy system optimization, as well as operational characteristics, is critical aspects in improving power conversion efficiency. The climate has a considerable influence on the solar energy’s reliability systems. As a consequence, optimization tactics are crucial in boosting the solar system’s reliability and efficacy. To accomplish so, strategies for tackling challenging PV system optimization difficulties must be developed.
3.1 Optimization Method for PV Base Hybrid System
3.1.1 hybrid renewable energy system.
Wind turbines, photovoltaic, mini hydro, and/or anything else fossil-fuel-powered producers are all examples of hybrid power systems. Small systems that can power a single home to big systems that can power a colony or an island, these systems come in a variety of sizes. Many isolated locations, especially those in developing countries in which the grid operator is economically and technically non-viable, will benefit from hybrid power systems. In 1978, the first rural hybrid energy system systems, which included solar panels and diesel generators, were built in the United States. Until an electric grid was connected to the hamlet, the power generated by the system was used to power the communal laundry machine, refrigerator, stitching machine, lighting and water drives. Photovoltaic (PV), Micro hydropower (MHP) and tiny wind power bases are routinely used to provide electricity to clients in remote locations, with or without energy storage systems. Varied energy sources have different properties in terms of production, like as seasonal river flows, strong sunlight during the day rather than at night, and high wind speeds in the summer. Commercial PV or wind systems that operate they do not create power 24 h a day, 365 days a year. When PV and wind are combined, the battery bank capacity and fuel requirements (if a conventional generator is utilized as a backup) are reduced, among other benefits. However, in order for a hybrid PV-Wind system to work, the area must have a high potential for both solar and wind energy. Environmental conditions, PV capacity, wind generator capacity, storage device capacity, generating location, and other factors all have a significant impact on the hybrid PV/wind-diesel system’s operation, maintenance, and cost ( Prakash and Khatod, 2016 ).
Extra energy is stored in battery banks, which are then used to power the devices, load when the hybrid system is underpowered. The inverter (DC/AC) must convert to fulfill consumer load demand, the voltage is converted from DC to AC. The battery charger’s output terminal, the storage battery, and the input terminal of the (DC/AC) converter are all linked in equivalent. Because fluctuations in solar radiation and wind velocity have a significant impact on energy generation, hybrid systems must be carefully designed to ensure a consistent power supply to clients in changing climatic conditions. Similarly, to keep system costs low, a detailed design should be conducted.
3.1.2 Photovoltaic System
Solar photovoltaic is the world’s third-largest renewable energy source by installed capacity, after hydro and wind power. Solar panels transform the sun’s solar radiation directly into useful electrical energy ( Figure 5 ). California and the Agua Caliente Solar Project are the world’s largest standalone PV generating installations. The aggregate capacity of both power plants is more than 250 MWP. However, due to the high cost of solar panels, its use is limited to less than 1% of total global energy production. PV energy arrangements are supposed to be unique of the most economical alternatives to encounter rural needs of energy.
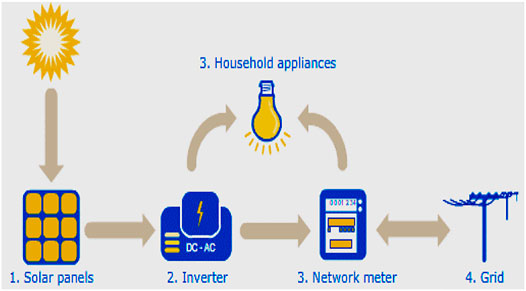
FIGURE 5 . Residential grid-tied solar Photovoltaic system diagram ( Wikimedia Commons, 2018 ).
For small communities of up to 100 homes, the economic feasibility has been built a hybrid PV system for decentralized power generation. The ideal mix can be determined using the hybrid PV system optimization approach based on the charge of energy produced, that is justified further by distance angle, tilt, and azimuth angle from the nearest power line. A PV hybrid system’s performance is measured in terms of electricity generation dependability across a wide range of load circumstances. The load and insolation were calculated using statistical methods. The output power of a PV panel is calculated using the equation below.
Where E stands for energy that is in (kWh). r shows solar panel yield which is in (percent). A stands for total covered panel area (m 2 ).
PR stands for performance ratio, a constant for losses (ranges lies between 0.5 and 0.9, showing default value = 0.75). H stands for solar radiation yearly average on slanted panels, and r is the solar panel return, which is computed by dividing one solar panel’s electrical power which is in kWp by its area.
3.1.3 Hydro System
Over the last four decades, global hydroelectric power output has gradually increased by an average of 3% every year. In 2011, hydropower from over 160 nations generated around 16% of global electricity. Water wheels are the forerunners of current turbines, which are used to transform hydraulic power into mechanical power, which is then converted into electrical power using a generator ( Li, 2021 ). Hydroelectric power, unlike solar and wind power that is fluctuating and constantly changing, is subject to a protracted seasonal cycle. The flow of water in rivers and streams fluctuates slowly as the seasons change.
3.1.4 Wind System
The area must have a high potential for wind energy throughout the year in order to operate a hybrid wind energy system successfully and affordably. Wind energy is currently captured utilizing a variety of small and large wind turbines of varying sizes and designs. It is one of the most rapidly increasing sources of alternative energy. It has a longer operational life than solar power and can generate electricity even on gloomy days and at night.
As a result, both wind and solar power systems require energy storage systems to store extra energy and use it when demand exceeds supply ( Zhang and Toudert, 2018 ; Zheng et al., 2018 ; Motahhir et al., 2020 ). The reassuring option, on the other hand, is that people can produce enough energy to satisfy their regular needs by setting up small solar or wind farms. Figure 6 shows the height to wind speed relation.
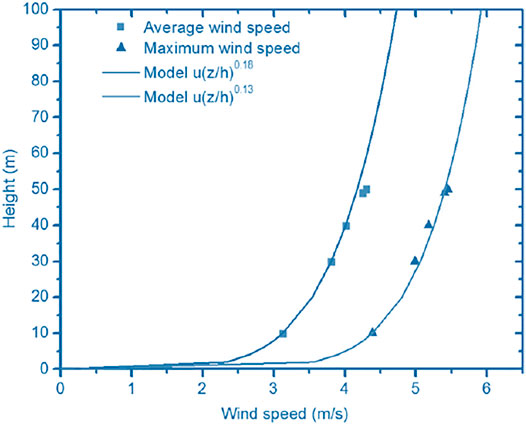
FIGURE 6 . Relation between Wind system and height ( Liu and Janajreh, 2012 ).
The generating capacity of a wind turbine is one of the most important factors to consider decisive criteria in selecting a certain kind of wind turbine for the chosen location is as an important component of a hybrid wind generators. Whenever feasible, turbine with best regular generating capacity recommended. A simplified technique for predicting yearly wind percentage was provided grounded based on the findings of an 8-year simulation using Wind statistics from five different places, hour by hour. Weibull wind speed distribution on a monthly basis data as input, other model factors such as the energy-to-load ratio, battery-to-load ratio, and others are used. Weibull devised the following equation to compute wind speed:
β = shape factor v = wind speed .
3.2 Optimization method for PV Based Grid System
The efficacy of grid-connected solar power is heavily dependent on the site’s solar irradiation, ambient temperature, load demand, and other factors in geographic area of installation. Identifying the best location and size for solar PV installation is a critical answer for improving radial distribution system performance. The design of the PV system to interface artificial intelligence techniques are used in the radial distribution network necessitates power system network analysis and mathematical modeling. The load flow analysis is performed in MATLAB simulation as shown in Figure 7 in conjunction with the SPSO to identify the placement and capacity of solar PV that will link to the Bahir Dar distribution network. By allowing for fluctuations in power demand over time, PV on-grid system integration is crucial for enhancing network capacity and system dependability. The utility grid is connected to an on-grid solar power system. The primary advantage of such a system is that electricity may be obtained from the utility grid, and when that power is unavailable, the PV system can step in. These on-grid systems might include or exclude battery storage. Batteries, a charge controller, solar modules, and inverters are used in these systems to improve the on-grid electrical system’s stability and offer long-term utilities service for a wide range of loads Electronic converters with high power play an important role in connecting a solar system to the grid by converting DC to AC and power conditioning ( Zakaria et al., 2020 ). A proposal is made for the current status of solar optimization study in a power system. This research looks on modeling approaches, restriction criteria, and optimization techniques. Because it is clean, ecologically friendly, and provides reliable power, the PV module system is full of potential. The effects of stand-alone and grid-installed solar generating on power system link, as well as their link to mandate answer, were researched. For calculating the location and size of solar generators, optimization approaches like as the genomic algorithm and swarm optimization were both introduced around the same time ( Getie et al., 2020 ) used the evolutionary algorithm and a geographical information system to integrate solar power with radial feeders. The genetic algorithm which was used to approximate the magnitude and point of penetration, and geographical information is utilized as data to decide where to install solar panels. As the multi objective function for photovoltaic integration, this study solely analyses real power loss and voltage profile.
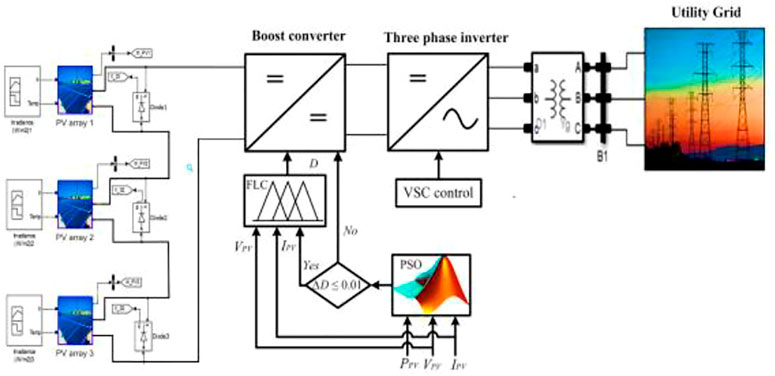
FIGURE 7 . PV based hybrid system MATLAB schematics ( Gonzalez-Longatt, 2005 ).
3.3 Optimization method for PV Based Stand Alone System
A stand-alone PV system should always be able to provide power to the load or energy consumption. The suggested technique, based on using hourly energy analysis, a curl (statistics) for battery bank (power, Wh), PV- array (space, m 2 ) proportions that satisfy the demand at all periods may be created. Since the solar power supply capacity varies, the battery store should be huge enough to provide enough power regardless of the number of cycles (discharge/charge) that the battery has to go concluded. This means that the solar grid will be backed up at sunset to meet the charging until more solar energy is available to start another charging cycle. Solar radiation, array size of PV, and storage volume are used to determine the efficiency of a PV system that is not connected to the grid. As a consequence, the scale of freestanding PV systems is essential to their dependability. Instinctive, analytical sizing methods and numerical are three types of sizing methods. Because it works with intuitive knowledge (without the use of cognitive processes), the first category of algorithms is highly imprecise and unreliable, and the risk of the end result being inaccurate is fairly high. The second is more precise, but accurate modeling requires series of solar radiation for a long term. There are ways in the third category that utilizes equations to describe the size of PV arrangement displaying as a result of dependability that is the focus of this study: boosting the output power of PV modules while lowering the system life cycle cost. Since most of the solar energy arrives in a straight line, solar panels or solar installations that point directly at the sun accumulate more energy by being perpendicular to the straight line between the panel and the sun. During the day, solar panels should face the earth’s equator (southern northern hemisphere or northern southern hemisphere) to capture as much solar energy as possible. The challenges and limitations of autonomous solutions to optimize the size of photovoltaic installations are highlighted to solve the problems of inaccurate parameter assumptions and poor demand performance evaluation of photovoltaic systems, which often lead to high material and installation costs. Along the same route, a new adaptation method was also proposed to improve the ability of photovoltaic generators to provide power to remote areas with pumping storage. Their research results show that zero power outages can be achieved at low energy costs, but the system does not use all the solar energy available in the area. Photovoltaic systems analysis refers to the concept of daily battery status to improve reliability while minimizing the possibility of power outages, excess energy, and cost constraints. However, priority must be given to strict compliance with the load profile. Another optimization strategy involves three steps. The first step is to calculate the photovoltaic power generation capacity connected to the grid with the help of 1-year solar energy data. It is believed that peak sunlight, ambient temperature, and cable and dust losses will affect the output energy of photovoltaic networks.
The quantity of stored energy, PV array output energy, load energy demand, battery efficiency, and inverter efficiency are used to compute the daily status of the battery storage in the second stage. In the third step, the chance of load loss is assessed, and the system cost is approximated using the costs of the PV array, batteries, and other components ( Lund and Mathiesen, 2009 ). On the other hand, the system cost equation is only partially derived and needs to be solved intuitively. The system simulation can be adapted to reduce the battery when the size of the solar photovoltaic device is not limited (very large). Since the solar photovoltaic device is huge, the system simulation can be repeated after determining the minimum battery size, but this time it is the smallest solar photovoltaic device. The size of the battery makes the battery sensitive to deep discharge when using this method and the size of the photovoltaic installation makes the system too large to be economically feasible. Therefore, the capacity of the battery pack can be increased, and each size can be simulated to find the smallest array. You can repeat this process to create a curve of the battery and PV array size pair, as shown in the results and discussion section. The accuracy of this method is determined by the simulation data, which includes both environmental parameters (such as sunlight exposure and ambient temperature) and loads. Therefore, hourly data obtained from reliable models such as those used in this study can guarantee availability and synchronization of exposure to solar radiation. To develop energy balances over time while taking into account real energy requirements for an energy efficient house as well as real radiation and ambient temperature data, a PV single system with dependencies on ambient temperature and other important factors was used in conjunction with inverter converter performance data. To ensure continuous functioning, the simulations first established a direct relationship between the area of the PV array and the capacity of the battery bank. One extreme of the connection is a high area of PV panels paired with a small battery capacity, resulting in low PV array effectiveness and a low battery consumption index. A small PV array, on the other hand, is the polar opposite. The tilt angle optimization approach was developed as a new optimization tool. Because of climatic and environmental conditions that fluctuate throughout the year, such as seasonal fluctuations, this optimization approach is focused at calculating effective tilt angles at various periods of the year. When compared to tracking systems, this strategy enhances collection efficiency while incurring no additional costs. Averaging the values of the solar geometry parameters for each mean solar day was used to do the calculations. The incident solar irradiances on a tilted surface, which include direct, diffuse, and reflected solar irradiances, may be expressed as a function of the global irradiance I on a horizontal surface by
The notion of the optimal periodical inclination angle was used to carry out this optimization, which allows for maximum incidence on the panels while simultaneously maximizing the use of the household’s actual energy usage. The inclination angle should be changed seven times per year, according to the computation of solar radiation for sloping surfaces using different solar geometry factors and incoming solar radiation, although total apparent power overestimates the load demand by 18%. On the other hand, these adjustments result in higher panel output and PV station reliability. The analysis of random load fluctuation demonstrates that the load profile must be followed notwithstanding the constraints. A power shortfall in the plant might occur from even slight increases in load demand.
3.3.1 Utilization of Solar Photovoltaic Energy
Photovoltaic systems power entire towns in distant places of the world. In the United States and Europe, a few utility companies operate “solar farms” to generate power ( Majidi et al., 2017 ). Photovoltaic cells have other industrial applications as well. These are often low-power applications in regions where regular electricity sources are cumbersome. Some emergency roadside phones use solar cells to charge their batteries. Some of the common applications are listed below.
3.3.2 Duffel Bags
The thin-film solar panels mounted on the outside of the backpack generate up to 4 W of power, which is enough to charge mobile phones, cameras and other electrical appliances while walking. External solar cells can also be added to briefcases and handbags. Students, hikers, and campers who need to keep their electronics charged while travelling or when they arrive at their destination would appreciate these backpacks.
3.3.3 Paint
Rather of utilizing standard silicon solar cells, polymers soaked in a solvent form “paint” or coat that may be put to any surface, include buildings, workplaces, and vehicles. It is low-cost and versatile. Instead of bulky solar photovoltaic panels, a solar paint employs thin-film nanoparticles as solar conductors rather than silicon. When small solar cells are placed to the surfaces of structure which confront the sun, they silently create pure, green power.
3.3.4 Solar Transportation
Photovoltaic (PV)-powered transportation is a novel technique to make the most of the sun’s energy. Solar energy can be used to power trains, subways, buses, airplanes, vehicles and even roads, and solar transportation is rapidly becoming a leading choice for renewable energy. A solar-powered aircraft has just completed a round-the-world voyage over the Pacific Ocean, capturing huge waves in unforgettable images. On the other hand, solar buses are helping China reduce its carbon footprint while ensuring efficient public transportation in densely populated areas such as Beijing. In the end, solar cars began to appear in racing competitions around the world, especially in Australia, where the solar spirit model aroused great interest. With these and other improvements, there is no doubt that solar energy is transforming the global transportation sector.
3.3.5 Refrigerators for Vaccines
As the entire world is experiencing the effects of the Corona Virus. Vaccine refrigerators are now required in all hospitals and clinics. There is no guarantee of 24-h electricity in developing countries, there is often no electrical infrastructure. Solar-powered vaccination coolers have been developed by private companies for use by healthcare workers in rural areas may provide crucial medication to individuals in need, according to Charlie Gay, Office of Energy Efficiency and Renewable Energy, Solar Energy Technologies Office. This technical solution has been saving lives for more than four decades.
3.3.6 Cell Phone Charger
After a few hours of ultraviolet radiation, the mobile phone USB charger can fully charge the mobile phone. These tablet-sized solar panels can power GPS trackers, tablets and even computers. They can be attached to backpacks and used to extract solar energy while walking, making them ideal for leisure activities ( Ming et al., 2017 ).
3.3.7 Solar Textile
Solar garments are a sort of solar textile that can be used for a variety of purposes. To generate useable solar power, solar cells are weaved into textile strands. According to Hicks, one variation, developed with faster than light (FTL) Solar, might remain erected like a camp to supply both electricity and shelter. Considering military service, safety missions, respite efforts, leisure activities, medical centers, and even makeshift housing as options. Solar fabric is the ideal answer for everywhere that need flexible and convenient solar power. According to Gay, roofs are one of hundreds of places somewhere solar panels create energy. We expect to see many more sites where solar technology is used to offer unconstrained, low-cost electricity as costs decrease and energy output rises.
3.3.8 Solar Water Pumps
Solar water pumps are used to promote water for irrigation, gardening, household use, drinking and other related purposes. These devices are suitable for areas where there is no electricity or limited power supply. The precisely crafted modules of the system are impact resistant and can withstand harsh weather conditions such as storms, rain, and dust.
3.3.9 Solar Tents
The solar tent is just a larger solar backpack. The built-in photovoltaic cells in the tent store solar energy throughout the day and are then used to illuminate the tent at night, as well as small electrical appliances such as charging or power electronics and radiators. The United States military uses a variant that can generate up to 2 kW of electricity during day.
3.3.10 Solar Buildings Technologies
Passive cooling and heating systems rely on the building’s design to satisfy specified thermal demand objectives with little or no mechanical support. Active heating systems use mechanical aid to provide hot water for space heating, while passive heating and cooling systems rely on the building’s architecture to satisfy set thermal demand objectives. Solar building technique is widely used in Pakistan ( Li and Zheng, 2019 ). However, there are no construction laws in place in the country that allow solar building systems installation.
3.3.11 Street Lights
Solar energy is increasingly being used to power streetlights around the world. The sun charges the batteries throughout the day, which power the light-emitting diodes (LEDs) that illuminate the streets at night. Smart sensors are being installed in streetlights in San Diego, which might direct motorists to open parking spots and assist first responders in an emergency. The combination of internet-connected sensors and solar-powered lamps saves both time and money ( Indra Gandhi et al., 2018 ).
3.3.12 Solar Ovens
Solar ovens, sometimes called solar cookers, use the sun’s energy to prepare food. Solar cookers either are parabolic or square shapes covered with a reflecting substance that focuses the amount of solar radiation into the box, warming the food equally. To assist focus the sun’s beams, the top lid is commonly made of glass. They are healthy to live with and are commonly employed in developing countries to reduce air pollution produced by fuel burning.
3.4 Optimization Issues
Solar energy confronts significant obstacles that might stymie its rapid expansion. These impediments can be characterized in terms of technology, politics, economics, and dependability. The adjustment of these issues, on the other hand, reduces the drawbacks and improves the solar energy system’s reliability. As a result, greater solar energy optimization can help to alleviate production uncertainty. PV power technology is being heavily invested in to improve efficiency and economic feasibility.
3.4.1 Extra Investment
Inverters and storage batteries must be purchased separately from PV cells. For use on the power grid, inverters convert direct current to alternating current. Storage batteries are important in on-grid connections for giving continuous power of electric power. On the other side, this higher spending could provide a solution to the PV cells’ intermittent problems ( Dong et al., 2019 ).
3.4.2 Issues With Intermittency
Solar energy and photovoltaic cells, like all other renewable energy sources, are prone to outages. It implies that it is not always available for power conversion, such as at night or when the weather is gloomy or damp. As a result, PV cells are unlikely to meet all of an electric power system’s demands.
3.4.3 Easily Broken
Solar PV has no upkeep or operating costs, it is vulnerable to damage due to its fragility. To protect your investment, there is a solution in the form of additional insurance.
3.4.4 Expensive
PV system market costs remain exorbitant and beyond of reach for many households. The higher production costs of non-conventional energy sources, combined with the availability of cheaper fossil fuel alternatives, entice customers and generate market rivalry for non-renewable technology ( Lagouir et al., 2019 ). The lack of economic models to support renewable energy technology prohibits small-scale PV systems from being scaled up to large-scale or commercial facilities. Subsidies are distributed more effectively to traditional fuel sources, giving them an unfair advantage over nonconventional sources. Governments must stimulate the market for PV technologies in order to reap the most advantage from renewable applications in the government market, government-driven market, and loan and cash market. PV plant input requirements, like as land and water, impede the installation of PV capacity.
3.4.5 Low Productivity Level in Future
In the near future, the technology will face another severe threat when the panels put during the early stages of the energy boom reach the end of their anticipated lifetime and are finally thrown in landfills. When the life duration of these panels reaches roughly 25 years, as indicated by the manufacturer, their productivity begins to decline. Recycling solar panels is a logical alternative for addressing the predicted worldwide PV waste, since retired PV panels may be reconditioned and redeployed. Recycling not only provides an effective method of recovering valuable elements from solar waste, but it also contributes to a better environment by using less energy to recover raw materials. The research and development work concerning solar PV recycling has already begun in countries such as Japan, the United States, India, Australia, and Europe.
3.4.6 Lack of Trained Professionals
The scarcity of trained personnel to teach, operate, and maintain non-conventional energy infrastructure, particularly in rural regions, has a detrimental impact on people’s desire to adopt these technologies. Geographic location is also important, because PV systems are only practical in certain places, and they face competition from alternative technologies that are better suited to the specific topography. Adopters are often concerned about systems failing during the rainy season and avoid purchasing PV systems owing to a lack of understanding. The absence of information between the supply and adopter sides further impedes the technology’s uptake. Because the generation and consuming sites are far apart, poor grid connection raises transportation costs and transmission losses. As a result, the majority of investors are hesitant to invest in technology.
3.4.7 Environmental Disadvantages
Due to various preliminary case studies, there is little understanding of the environmental and economic advantages of PV recycling technology. Although element recovery is advantageous, the energy required to collect valuable metals from discarded PV panels is greater than that required to gather, dismantle, and retrieve the modules. Although recycling operations are not viable, the cost of recycling PV panels is minimal ( Maulik and Das, 2018 ) According to research, the profit earned from selling the recycled material of copper indium gallium selenite solar panels (CIGS) is greater than the recovery price. However, the earned amount for c-Si and p-Si solar modules is less than the cost of solar panels. Despite the financial benefits of reselling recovered items and the environmental benefits of recycling, businesses prefer landfill disposal to recycling due to the lower initial cost of dumping.
4 Optimization Challenges
Table 4 shows the summary of different approaches which are used for optimization techniques and method. Non-conventional energy sources have been an important aspect of study and development among scholars since the early 20th century. Despite the remarkable technologies that have evolved in recent decades, the majority of developing countries have delayed the shift to renewable energy sources. Fossil fuels have boosted CO 2 emissions and contributed to global warming. Because of numerous types of hurdles, the majority of nations have exhibited reluctance to adopt renewable technologies. Based on the existing market, policy implementation is critical, which includes energy auctions, integration of PV technologies with non-conventional energy sources, and timely completion of PV projects. By allowing users to purchase or lease a portion of the shared PV system, community-shared solar projects aid in the creation of financial arrangements and the alleviation of financial constraints. These business models, in turn, contribute to the development of a PV system in the residential market, the stabilization of power prices, and the reduction of power bills. To reap the full benefits of an energy transition, policies must be strengthened through mobilizing financial investment, economic diversification, and information exchange. PV system market costs remain exorbitant and beyond of reach for many households. The lack of economic models to support renewable energy technology prohibits small-scale PV systems from being scaled up to large-scale or commercial facilities.
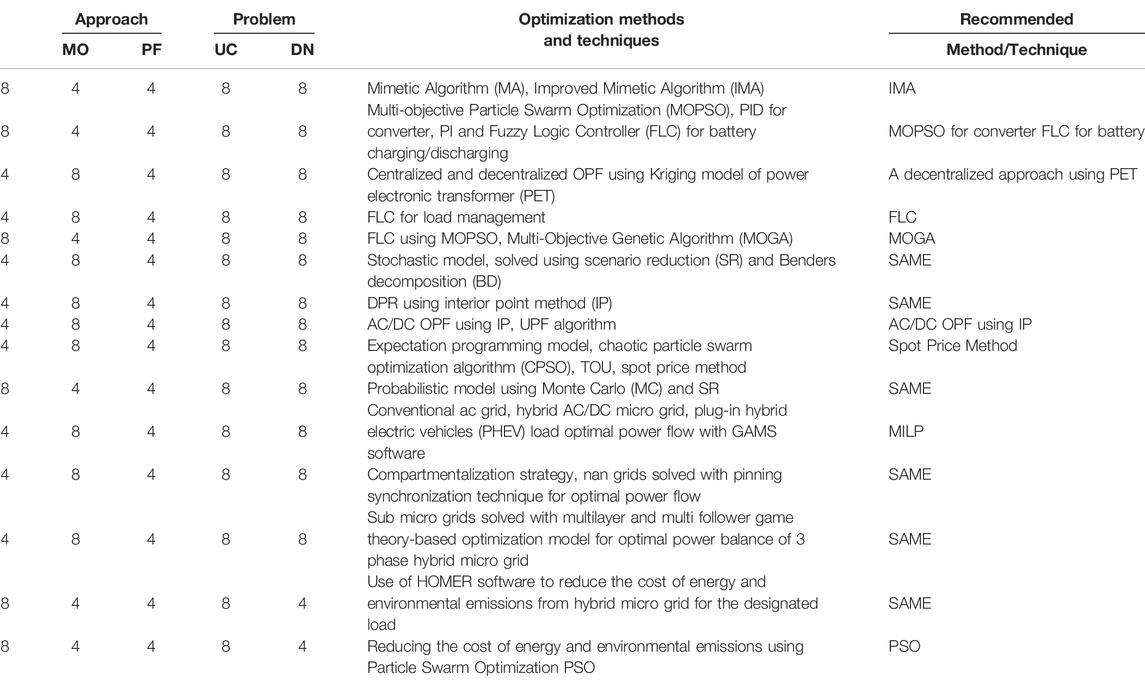
TABLE 4 . Summary of approach optimization technique and methods ( Li et al., 2016 ).
4.1 RES Optimization Challenges
To enhance efficiency, there is a significant investment in renewable energy technologies. The cost of producing renewable energy continues to plummet in 2017, according to an IRENA (2018) . The development and deployment of renewable energy technologies necessitate policies and expenditures that have not been thoroughly assessed. As a result, power quality is a metric that assesses a system’s capacity to offer users with continuous access to their electronic devices. Any irregularity or breakdown in the power grid that obstructs or interrupts electrical equipment’s operation indicates a lack of power quality or reliability. Renewable energy sources will save costs in both transmission and production. The most prevalent disadvantage of employing RESs is that they represent a constant challenge because to their fluctuating existence, which is entirely dependent on climate fluctuation and may result in load refusal in some places ( Maulik and Das, 2018 ). The power production of RESs has substantially grown as a consequence of the deployment of various optimization technologies in response to growing energy demand and improved performance. The entire power producing capacity has increased by about 9% since 2016 ( Zakaria et al., 2020 ). According to the reference, renewable energy accounted for 70% of net additions to global power capacity in 2017 ( Raturi, 2019 ). The key causes for this were the improved cost competitiveness of solar PV panels and wind turbine technology, as well as the availability of performance optimization technologies. Furthermore, the increased usage of renewable energy raises awareness of the need of energy efficiency and quality in power generation and distribution. The biggest disadvantage of adopting renewable resources is that they are intermittent nonetheless, one of the key advantages is the system reliability demonstrated by the operational parameters. Power generated from renewable sources will soon be less expensive than electricity generated from fossil fuels. General, the development of RESs has resolved various difficulties-optimization-in reducing energy costs, reducing costs, net and other optimizations related to costs, such as reducing life cycle costs (LCC). The optimal size and capacity of the trees is determined by providing the required reliability in terms of power supply, operating costs, and grid power and greenhouse gas emissions.
4.2 Energy Optimization Challenges
The uncertainty of how much of the sun’s rays it will get is an issue for solar PV because the weather might change at any time. As a result, determining how much energy to store for future use would be challenging. While power is still required, sunlight is rare during the night. Solar energy has significant obstacles that might limit its fast expansion. Technology, politics, economics, and reliability are the four areas that these hurdles fall into. On the other side, addressing these problems decreases the disadvantages and improves the solar energy system’s dependability. The researchers are also given information on the most recent developments in intelligent optimization in solar energy applications, as well as important research topics. Since the goal of optimization is to maximize benefits while reducing costs, it is critical to understand the advantages and disadvantages of the systems under consideration. In this setting, academics have begun to explore and propose strategies and models to maximize advantages while minimizing drawbacks. To overcome difficulties related to the design, operation, and process of renewable systems, several researches combine traditional optimization techniques with newer heuristic approaches ( Zhao T. et al., 2017 ; Zhao Zy. et al., 2017 ; Allam et al., 2018 ; Eajal et al., 2017 ). PV systems require precise and reliable performance data in order to precisely assess power output and capacity in current operating circumstances. The formulation of effective operational and control choices is aided by this dependable data. On the other hand, by examining the numerous aspects that impact performance and exploring potential ways to increase the power plant’s performance, the optimization and efficiency of a solar system may be improved. PV cells have a number of problems, including a halt in power output when the panel is not exposed to sunlight and a poor efficiency. This might result in the system’s original investment criteria not being met. As a result, solar energy storage devices have been proposed as a means of compensating for the lack of light and smoothing out power output. This technology is dependent on batteries, which are frequently bulky, huge, and heavy, take up a lot of space, and require maintenance or even replacement on a regular basis ( Li et al., 2016 ).
5 Conclusion
For policymakers all throughout the world, this document presented an in-depth review and relative analysis of solar technology for clean power generation.
According to the research results, there are two types of technologies: complex technologies, such as PTC, PV and STP, with a total installed capacity of 7,828.5 MW and an efficiency of 10–16%, LCEO is $0.1–0.24/kwh, which has broad prospects in terms of environmental impact and technical efficiency. There are also technologies that, although having a 390 MW installed capacity, look to be promising in terms of environmental implications and technological efficiency. Furthermore, CPVT and CPV, they have yet to be utilized in large-scale power facilities since they are still in the early stages of development. Nonetheless, Scientists from several nations are leading the charge in CPV and CPVT research. The use of solar energy to improve energy efficiency has been a concern due to the dynamic nature of solar energy, solar PV material, design, and challenging computation of optimization difficulties. As a result, this review looks into solar energy optimization in depth. The optimization techniques have shown excellent results in solar PV applications in terms of size, power production and capacity demand. Additionally, the enhancements to reduce operational expenses and power damages while also increasing peak power integration and controllability. The paper also looked at the primary roadblocks to solar PV optimization, emphasizing the importance of modern computers and objective function ( Qiu et al., 2019 ).
Author Contributions
AS, and AH, suggested the idea of this work, wrote the manuscript and made final improvement, whereas SC and PM provided help with alignment of the paper, proof reading, editing, improvement of the article. AI and MM provided the financial assistance.
Conflict of Interest
The authors declare that the research was conducted in the absence of any commercial or financial relationships that could be construed as a potential conflict of interest.
Publisher’s Note
All claims expressed in this article are solely those of the authors and do not necessarily represent those of their affiliated organizations, or those of the publisher, the editors and the reviewers. Any product that may be evaluated in this article, or claim that may be made by its manufacturer, is not guaranteed or endorsed by the publisher.
Achkari, O., and El Fadar, A. (2020). Latest Developments on TES and CSP Technologies - Energy and Environmental Issues, Applications and Research Trends. Appl. Therm. Eng. 167, 114806. doi:10.1016/j.applthermaleng.2019.114806
CrossRef Full Text | Google Scholar
Agyekum, E. B., and Velkin, V. I. (2020). Optimization and Techno-Economic Assessment of Concentrated Solar Power (CSP) in South-Western Africa: A Case Study on Ghana. Sustain. Energy Technol. Assessments 40, 100763. doi:10.1016/j.seta.2020.100763
Allam, M. A., Hamad, A. A., Kazerani, M., and El-Saadany, E. F. (2018). A Novel Dynamic Power Routing Scheme to Maximize Loadability of Islanded Hybrid AC/DC Microgrids under Unbalanced AC Loading. IEEE Trans. Smart Grid 9, 5798–5809. doi:10.1109/tsg.2017.2697360
Alotaibi, S., Alotaibi, F., and Ibrahim, O. M. (2020). Solar-assisted Steam Power Plant Retrofitted with Regenerative System Using Parabolic Trough Solar Collectors. Energy Rep. 6, 1 24–33. doi:10.1016/j.egyr.2019.12.019
Alsaffar, A. (2015). An Overview of Location Planning of Solar Generation. Available at: https://www.nrel.gov/docs/fy14osti/60240.pdf .
Google Scholar
Aly, A., Bernardos, A., Fernandez-Peruchena, C. M., Jensen, S. S., and Pedersen, A. B. (2019). Is Concentrated Solar Power (CSP) a Feasible Option for Sub-saharan Africa?: Investigating the Technoeconomic Feasibility of CSP in Tanzania. Renew. Energy 135, 12 24–40. doi:10.1016/j.renene.2018.09.065
Aqachmar, Z., Allouhi, A., Jamil, A., Gagouch, B., and Kousksou, T. (2019). Parabolic Trough Solar Thermal Power Plant Noor I in Morocco. Energy 178. doi:10.1016/j.energy.2019.04.160
Aqachmar, Z., Bouhal, T., and Lahrech, K. (2020). Energetic, Economic, and Environmental (3 E) Performances of High Concentrated Photovoltaic Large Scale Installations: Focus on Spatial Analysis of Morocco. Int. J. Hydrogen Energy 45, 10840–10861. doi:10.1016/j.ijhydene.2020.01.210
Assadeg, J., Sopian, K., and Fudholi, A. (2019). Performance of Grid-Connected Solar Photovoltaic Power Plants in the Middle East and North Africa. Int. J. Electr. Comput. Eng. (IJECE) 9, 3375. doi:10.11591/ijece.v9i5.pp3375-3383
Awan, A. B., Chandra Mouli, K. V. V., and Zubair, M. (2020a). Performance Enhancement of Solar Tower Power Plant: a Multi-Objective Optimization Approach. Energy Convers. Manag. 225, 113378. doi:10.1016/j.enconman.2020.113378
Awan, A. B., Zubair, M., and Chandra Mouli, K. V. V. (2020b). Design, Optimization and Performance Comparison of Solar Tower and Photovoltaic Power Plants. Energy 199, 117450. doi:10.1016/j.energy.2020.117450
Bamisile, O., Huang, Q., Dagbasi, M., and Adebayo, V. (2020). Thermo-environ Study of a Concentrated Photovoltaic Thermal System Integrated with Kalina Cycle for Multigeneration and Hydrogen Production. Int. J. Hydrogen Energy 45 (51), 267 16–32. doi:10.1016/j.ijhydene.2020.07.029
Bellos, E. (2019). Progress in the design and the applications of linear Fresnel reflectors e a critical review. Therm. Sci. Eng. Prog. 10, 1 12–37. doi:10.1016/j.tsep.2019.01.014
Bishoyi, D., and Sudhakar, K. (2017). Modeling and Performance Simulation of 100 MW LFR Based Solar Thermal Power Plant in Udaipur India. Resource-Efficient Technol. 3 (4), 365–377. doi:10.1016/j.reffit.2017.02.002
Boukelia, T. E., Arslan, O., and Mecibah, M. S. (2017). Potential Assessment of a Parabolic Trough Solar Thermal Power Plant Considering Hourly Analysis: ANN-Based Approach. Renew. Energy 105, 3 24–33. doi:10.1016/j.renene.2016.12.081
Chen, R., Rao, Z., and Liao, S. (2018). Determination of Key Parameters for Sizing the Heliostat Field and Thermal Energy Storage in Solar Tower Power Plants. Energy Convers. Manag. 177, 3 85–94. doi:10.1016/j.enconman.2018.09.065
Collado, F. J., and Guallar, J. (2019). Quick Design of Regular Heliostat Fields for Commercial Solar Tower Power Plants. Energy 178, 1 15–25. doi:10.1016/j.energy.2019.04.117
Dong, L., Zhang, T., Pu, T., Chen, N., and Sun, Y. (2019). A Decentralized Optimal Operation of AC/DC Hybrid Microgrids Equipped with Power Electronic Transformer. IEEE Access 7, 157946–157959. doi:10.1109/access.2019.2949378
Dowling, A. W., Zheng, T., and Zavala, V. M. (2017). Economic Assessment of Concentrated Solar Power Technologies: a Review. Renew. Sustain. energy Rev. 72, 1019–1032. doi:10.1016/j.rser.2017.01.006
Eajal, A. A., El-Saadany, E. F., and Ponnambalam, K. (2017). “Optimal Power Flow for Converter-Dominated AC/DC Hybrid Microgrids,” in Proceedings of the 2017 IEEE International Conference on Industrial Technology (ICIT) (Toronto, Canada: IEEE ), 603–608. doi:10.1109/icit.2017.7915427
Eddhibi, F., Ben Amara, M., Balghouthi, M., and Guizani, A. (2017). Design and Analysis of a Heliostat Field Layout with Reduced Shading Effect in Southern Tunisia. Int. J. Hydrogen Energy 42 (48), 289 73–96. doi:10.1016/j.ijhydene.2017.07.217
Farh, H. M. H., Eltamaly, A. M., and Othman,, M. F. (2018). Hybrid PSO-FLC for Dynamic Global Peak Extraction of the Partially Shaded Photovoltaic System. PLOS ONE 13, e0206171. doi:10.1371/journal.pone.0206171
PubMed Abstract | CrossRef Full Text | Google Scholar
Furkan, D., and Mehmet Emin, M. (2010). Critical Factors That Affecting Efficiency of Solar Cells. Smart Grid and Renewable Energy 1 (1), 47–50. doi:10.4236/sgre.2010.11007
Gaga, A., Benssassi, H., Errahimi, F., and Sbai, N. E. (2017). Battery State of Charge Estimation Using an Adaptive Unscented Kalman Filter for Photovoltaics Applications. Int. Rev. Autom. Control 10 (4), 3 49–58. doi:10.15866/ireaco.v10i4.11393
Georgescu-Roegen, N. (1979). Energy Analysis and Economic Valuation. South. Econ. J. 45, 1023–1058. doi:10.2307/1056953
Getie, E. M., Gessesse, B. B., and Workneh, T. G. (2020). Photovoltaic Generation Integration with Radial Feeders Using GA and GIS. Int. J. Photoenergy 2020, 8854711. doi:10.1155/2020/8854711
Ghodbane, M., Boumeddane, B., and Said, N. (2016). A Linear Fresnel Reflector as a Solar System for Heating Water: Theoretical and Experimental Study. Case Stud. Therm. Eng. 8, 176–186. doi:10.1016/j.csite.2016.06
Ghodbane, M., Boumeddane, B., Said, Z., and Bellos, E. (2019). A Numerical Simulation of a Linear Fresnel Solar Reflector Directedto Produce Steam for the Power Plant. J. Clean. Prod. 231, 494e508. doi:10.1016/j.jclepro.2019.05.201
Gonzalez-Longatt, F. M. (2005). Model of Photovoltaic Module in Matlab. Ii Cibelec 2005, 1–5.
Hakimi, M., Baniasadi, E., and Afshari, E. (2020). Thermo-economic Analysis of Photovoltaic, Central Tower Receiver and Parabolic Trough Power Plants for Herat City in Afghanistan. Renew. Energy 150, 8 40–53. doi:10.1016/j.renene.2020.01.009
Hissouf, M., Feddaoui, M., Najim, M., and Charef, A. (2020). Numerical Study of a Covered Photovoltaic-Thermal Collector (PVT) Enhancement Using Nanofluids. Sol. Energy 199, 1 15–27. doi:10.1016/j.solener.2020.01.083
IEA (2020). International Energy Agency. Available at: https://www.iea.org/ .
Indra Gandhi, V., Logesh, R., Subramaniyaswamy, V., Vijayakumar, V., Siarry, P., and Uden, L. (2018). Multi-Objective Optimization and Energy Management in Renewable Based AC/DC Microgrid. Comput. Electr. Eng. 70, 179–198.
IRENA (2018). Global Energy Transformation: A Roadmap to 2050 . International Renewable energy agency . Available at: https://www.irena.org/-/media/Files/IRENA/Agency/Publication/2018/Jul/IRENA_Renewable_Energy_Statistics_2018.pdf .
Islam, M. T., Huda, N., Abdullah, A. B., and Saidur, R. (2018). A Comprehensive Review of State-Of-The-Art Concentrating Solar Power (CSP) Technologies: Current Status and Research Trends. Renew. Sustain. Energy Rev. 91, 987–1018. doi:10.1016/j.rser.2018.04.097
Islam, M. T., Huda, N., and Saidur, R. (2019). Current Energy Mix and Techno-Economic Analysis of Concentrating Solar Power (CSP) Technologies in Malaysia. Renew. Energy 140, 789–806. doi:10.1016/j.renene.2019.03.107
Ju, X., Xu, C., Hu, Y., Han, X., Wei, G., and Du, X. (2017). A Review on the Development of Photovoltaic/concentrated Solar Power (PVCSP) Hybrid Systems. Sol. Energy Mater Sol. Cells 161 (12), 3 05–27. doi:10.1016/j.solmat.2016.12.004
Kumar, S., Agarwal, A., and Kumar, A. (2021). Financial Viability Assessment of Concentrated Solar Power Technologies under Indian Climatic Conditions. Sustain. Energy Technol. Assessments 43, 100928. doi:10.1016/j.seta.2020.100928
Laarabi, B., El Baqqal, Y., Dahrouch, A., Barhdadi, A., Deep Pizzi, S., Corbo, L., et al. (2021a). Fintech and SMEs Sustainable Business Models: Reflections and Considerations for a Circular Economy. J. Clean. Prod. 281, 125217.
Laarabi, B., El Baqqal, Y., Rajasekar, N., and Barhdadi, A. (2021b). Updated Review on Soiling of Solar Photovoltaic Systems Morocco and India Contributions. J. Clean. Prod. 311, 127608. doi:10.1016/j.jclepro.2021.127608
Lagouir, M., Badri, A., and Sayouti, Y. (2019). “An Optimal Energy Management System of Islanded Hybrid AC/DC Microgrid,” in Proceedings of the 2019 5th International Conference on Optimization and Applications (ICOA) (Kenitra, Morocco: IEEE ), 1–6.
Li, P., Hua, H., Di, K., and Zhou, J. (2016). “Optimal Operation of AC/DC Hybrid Microgrid under Spot Price Mechanism,” in Proceedings of the 2016 IEEE Power and Energy Society General Meeting (PESGM) (Boston, MA, USA: IEEE ), 1–5. doi:10.1109/pesgm.2016.7741670
Li, P., and Zheng, M. (2019). Multi-objective Optimal Operation of Hybrid AC/DC Microgrid Considering Source-Network-Load Coordination. J. Mod. Power Syst. Clean. Energy 7, 1229–1240. doi:10.1007/s40565-019-0536-3
Li, y. N. (2021). Renewable Power Generation Subsidies in china: An Economic Feasibility Analysis and Policy Recommendations . University of Tokyo . doctoral dissertation.
Liu, S., and Janajreh, I. (2012). “Wind Energy Assessment: Masdar City Case Study,” in 2012 8th International Symposium on Mechatronics and its Applications ( Springer ), 1–6. doi:10.1109/ISMA.2012.6215162
Lokar, J., and Virtic, P. (2020). The Potential for Integration of Hydrogen for Complete Energy Self-Sufficiency in Residential Buildings with Photovoltaic and Battery Storage Systems. Int. J. Hydrogen Energy 45, 345 66–78. doi:10.1016/j.ijhydene.2020.04.170
Lopez, O., Banos, A., and Arenas, A. (2020). On the Thermal Performance of Flat and Cavity Receivers for a Parabolic Dish Concentrator and Low/medium Temperatures. Sol. Energy 199, 91123. doi:10.1016/j.solener.2019.07.056
Lund, H., and Mathiesen, B. V. (2009). Energy System Analysis of 100% Renewable Energy Systems—The Case of Denmark in Years 2030 and 2050. Energy 34 (5), 524–531.
Luque, A., and Hegedus, S. (2011). Handbook of Photovoltaic Science and Engineering . John Wiley & Sons .
Majidi, M., Nojavan, S., Nourani Esfetanaj, N., Najafi-Ghalelou, A., and Zare, K. (2017). A Multi-Objective Model for Optimal Operation of a battery/PV/fuel Cell/grid Hybrid Energy System Using Weighted Sum Technique and Fuzzy Satisfying Approach Considering Responsible Load Management. Sol. Energy 144, 79–89. doi:10.1016/j.solener.2017.01.009
Marzo, A., Salmon, A., Polo, J., Ballestrín, J., Soto, G., Quiñones, G., et al. (2021). Solar Extinction Map in Chile for Applications in Solar Power Tower Plants, Comparison with Other Places from Sunbelt and Impact on LCOE. Renew. Energy 170, 197–211. doi:10.1016/j.renene.2021.01.126
Maulik, A., and Das, D. (2018). “Multi-Objective Optimal Dispatch of AC-DC Hybrid Microgrid,” in Proceedings of the 2018 IEEE PES Asia-Pacific Power and Energy Engineering Conference (APPEEC) (Kota Kinabalu, Malaysia: IEEE ), 82–87. doi:10.1109/appeec.2018.8566354
Ming, M., Wang, R., Zha, Y., and Zhang, T. (2017). Multi-objective Optimization of Hybrid Renewable Energy System Using an Enhanced Multi-Objective Evolutionary Algorithm. Energies 10 (5), 674. doi:10.3390/en10050674
Mohamed, A. F., Elarini, M. M., and Othman, A. M. (2014). A New Technique Based on Artificial Bee Colony Algorithm for Optimal Sizing of Stand-Alone Photovoltaic System. J. Adv. Res. 5 (3), 397–408. doi:10.1016/j.jare.2013.06.010
Motahhir, S., El Hammoumi, A., and El Ghzizal, A. (2020). The Most Used MPPT Algorithms: Review and the Suitable Low-Cost Embedded Board for Each Algorithm. J. Clean. Prod. 246, 118983. doi:10.1016/j.jclepro.2019.118983
Moukhtar, I., El Dein, A. Z., Elbaset, A. A., and Mitani, Y. (2021). “Penetration Characteristics of Hybrid CSP and PV Solar Plants Economic,” in Power Systems ( Springer Science and Business Media Deutschland GmbH ), 99–111. doi:10.1007/978-3-030-61307-5_5
Otero, A. F., Cidras, J., and Garrido, C. (1998). “Genetic Algorithm Based Method for Grounding Grid Design,” in 1998 IEEE International Conference on Evolutionary Computation Proceedings ( IEEE World Congress on Computational Intelligence, Cat. No. 98TH8360 ), 120–123.
Ouagued, M., Khellaf, A., and Loukarfi, L. (2018). Performance Analyses of CueCl Hydrogen Production Integrated Solar Parabolic Trough Collector System under Algerian Climate. Int. J. Hydrogen Energy 43 (6), 34 51–65. doi:10.1016/j.ijhydene.2017.11.040
Prakash, P., and Khatod, D. K. (2016). Optimal Sizing and Siting Techniques for Distributed Generation in Distribution Systems: A Review. Renew. Sustain. energy Rev. 57, 111–130. doi:10.1016/j.rser.2015.12.099
Qiu, H., Gu, W., Xu, Y., and Zhao, B. (2019). Multi-Time-Scale Rolling Optimal Dispatch for AC/DC Hybrid Microgrids with Day-Ahead Distributionally Robust Scheduling. IEEE Trans. Sustain. Energy 10 (4), 1653–1663. doi:10.1109/TSTE.2018.2868548
Raturi, A. K. (2019). Renewables 2019 Global Status Report. Available at: https://www.ren21.net/wp-content/uploads/2019/05/gsr_2019_full_report_en.pdf .
Sanda, A., Moya, S. L., and Valenzuela, L. (2019). Modeling and Simulation Tools for Direct Steam Generation in Parabolic-Trough Solar Collectors: a Review. Renew. Sustain. energy Rev. 113, 109226. doi:10.1016/j.rser.2019.06.033
Simsek, Y., Mata-Torres, C., Escobar, R., and Cardemil, J. M. (2018). Incentives and Financial Conditions Effect Analysis on Levelized Cost of Electricity (LCOE) and Government Cost for Concentrated Solar Power (CSP) Projects in Chile. AIP Conf. Proc. 2033. doi:10.1063/1.5067134
Solar paces (2019). Solar Paces NREL. Available at: https://solarpaces.nrel.gov/ .
Tascioni, R., Arteconi, A., Del Zotto, L., and Cioccolanti, L. (2020). Fuzzy Logic Energy Management Strategy of a Multiple Latent Heat Thermal Storage in a Small-Scale Concentrated Solar Power Plant. Energies 13 (11), 23–28. doi:10.3390/en13112733
Wikimedia Commons (2018). How Solar Power Works. Available at: https://commons.wikimedia.org/wiki/File:How_Solar_Power_Works.png .
Zakaria, A., Ismail, F. B., Lipu, M. S. H., and Hannan, M. A. (2020). Uncertainty Models for Stochastic Optimization in Renewable Energy Applications. Renew. Energy 145, 1543–1571. doi:10.1016/j.renene.2019.07.081
Zhang, H. L., Baeyens, J., Degreve, J., and Cac eres, G. (2013). Concentrated Solar Power Plants: Review and Design Methodology. Renew. Sustain. energy Rev. 22, 466e81. doi:10.1016/j.rser.2013.01.032
Zhang, H., and Toudert, J. (2018). Optical Management for Efficiency Enhancement in Hybrid Organic-Inorganic Lead Halide Perovskite Solar Cells. Sci. Technol. Adv. Mater. 19 (1), 411–424. doi:10.1080/14686996.2018.1458578
Zhao T, T., Xiao, J., Hai, K. L., and Wang, P. (2017). “Two-Stage Stochastic Optimization for Hybrid AC/DC Microgrid Embedded Energy Hub,” in Proceedings of the 2017 IEEE Conference on Energy Internet and Energy System Integration (EI2) (Beijing, China: IEEE ), 1–6. doi:10.1109/ei2.2017.8245648
Zhao Zy, Z. Y., Chen, Y. L., and Thomson, J. D. (2017). Levelized Cost of Energy Modeling for Concentrated Solar Power Projects: a China Study. Energy 120, 1 17–27. doi:10.1016/j.energy.2016.12.122
Zhar, R., Allouhi, A., Ghodbane, M., Jamil, A., and Lahrech, K. (2021). Parametric Analysis and Multi-Objective Optimization of a Combined Organic Rankine Cycle and Vapor Compression Cycle. Sustain. Energy Technol. Assessments 47, 101401. doi:10.1016/j.seta.2021.101401
Zheng, Y., Jenkins, B. M., Kornbluth, K., and Træholt, C. (2018). Optimization under Uncertainty of a Biomass-Integrated Renewable Energy Microgrid with Energy Storage. Renew. energy 123, 204–217. doi:10.1016/j.renene.2018.01.120
Zhuang, X., Xu, X., Liu, W., and Xu, W. (2019). LCOE Analysis of Tower Concentrating Solar Power Plants Using Different Molten-Salts for Thermal Energy Storage in China. Energies 12 (7), 1394. doi:10.3390/en12071394
Zineb, A., Hicham, B., Khadija, L., and Abdelfettah, B. (2021). Solar Technologies for Electricity Production: An Updated Review. nternational J. Hydrogen Energy 46 (60), 30790–30817. ISSN 0360-3199. doi:10.1016/j.ijhydene.2021.06.190
FPC Flat plate collector
CPV Concentrated photovoltaic
GaAs Gallium Arsenide
CPC Compound parabolic collector
GIS Geographic information system
HCPV High Concentrated photovoltaic
MWh Megawatt hour
LCPV Low Concentrated photovoltaic
CIGS Copper indium gallium selenide solar cells
LFR Linear Fresnel reflector
IEA International Energy Agency
HTF Heat transfer fluid
HFC Heliostat Field Collector
CdTe Cadmium telluride
BESS Battery Energy Storage System
MHP Micro hydropower
CSP Concentrated solar power
PTSTPP Parabolic Trough Solar Thermal Power Plant
PV Photovoltaic
ANN Artificial neural network
PVT Photovoltaic thermal collectors
CPVT Concentrated photovoltaic thermal collectors
SAM Solar advisor model
SBS Spectral beam splitting
SCR Solar Central Receiver
SGS Steam Generation System
TES Thermal energy storage
LCOE Levelized cost of electricity ($/kWh)
NREL National renewable energy laboratory
STP Standard temperature and pressure
PDC Parabolic dish collector
Keywords: photovoltaic, hybrid system, stand-alone system, grid system, energy, solar energy, renewable, clean energy optimization methods
Citation: Soomar AM, Hakeem A, Messaoudi M, Musznicki P, Iqbal A and Czapp S (2022) Solar Photovoltaic Energy Optimization and Challenges. Front. Energy Res. 10:879985. doi: 10.3389/fenrg.2022.879985
Received: 20 February 2022; Accepted: 24 March 2022; Published: 30 May 2022.
Reviewed by:
Copyright © 2022 Soomar, Hakeem, Messaoudi, Musznicki, Iqbal and Czapp. This is an open-access article distributed under the terms of the Creative Commons Attribution License (CC BY). The use, distribution or reproduction in other forums is permitted, provided the original author(s) and the copyright owner(s) are credited and that the original publication in this journal is cited, in accordance with accepted academic practice. No use, distribution or reproduction is permitted which does not comply with these terms.
*Correspondence: Arsalan Muhammad Soomar, [email protected] , [email protected]
Disclaimer: All claims expressed in this article are solely those of the authors and do not necessarily represent those of their affiliated organizations, or those of the publisher, the editors and the reviewers. Any product that may be evaluated in this article or claim that may be made by its manufacturer is not guaranteed or endorsed by the publisher.
Thank you for visiting nature.com. You are using a browser version with limited support for CSS. To obtain the best experience, we recommend you use a more up to date browser (or turn off compatibility mode in Internet Explorer). In the meantime, to ensure continued support, we are displaying the site without styles and JavaScript.
- View all journals
- Explore content
- About the journal
- Publish with us
- Sign up for alerts
- Open access
- Published: 26 November 2020
Performance evaluation of a large-scale thermal power plant based on the best industrial practices
- Yousef S. H. Najjar 1 &
- Amer Abu-Shamleh ORCID: orcid.org/0000-0002-2553-731X 1
Scientific Reports volume 10 , Article number: 20661 ( 2020 ) Cite this article
17k Accesses
7 Citations
Metrics details
- Diesel fuel
- Natural gas
The aim of this study is to assess and evaluate the performance of a large-scale thermal power plant (TPP). The performance rating was conducted in compliance with the statistical principles. The data for this analysis were obtained for a TPP with an installed capacity of 375 MW during a span of 8 years (2010–2017). Four parameters were used to evaluate the performance of the TPP including the availability, the reliability, the capacity factor, and the thermal efficiency. These parameters were calculated using a set of equations and then compared to the international best practices and target values. The results indicate that approximately 91% of the expected capacity was available throughout the studied period against the industry best practice of 95%. However, the average TPP’s reliability was found to be approximately 95% against the target value of 99.9%. Furthermore, the average capacity factor throughout the studied period is 70% as against the international value of 40–80%. Moreover, the thermal efficiency of the TPP is 40% against the target value of 49%. Due to the outage hours and malfunctions, the power losses throughout the studied period reached 846 MW. Overall, the analysis indicates that the studied TPP is not within the scope of the best industrial practices.
Similar content being viewed by others
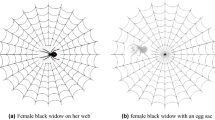
Greenhouse gases emission reduction for electric power generation sector by efficient dispatching of thermal plants integrated with renewable systems
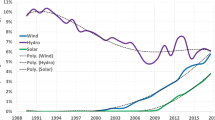
Techno-economic performances of future concentrating solar power plants in Australia
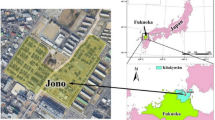
Analysis of the potential application of a residential composite energy storage system based on a double-layer optimization model
Introduction.
The gas turbine (GT), also known as the combustion turbine, is a rotary motor that removes energy from a hot gas flow generated in a stream of compressed air by combustion of gas or fuel oil. The GT has a radial or axial flow air compressor mechanically connected with an upstream turbine and combustion chamber. Energy is released by the mixing and ignition of compressed air into the combustion chamber (combustor) 1 . Energy is generated in shaft power by GTs and used to power generators and other machinery. GTs have been recognized as prime movers for reliable baseload applications and they are being increasingly used world wide 2 , 3 .
GTs have earned a privileged position among other electrical generation technologies due to its high efficiency and reliability, particularly when incorporated with combined cycle 4 . GTs are also known for their flexibility and regular availability 4 . However, the performance of the GT is influenced by both the efficiency of components and the turbine inlet temperature 5 , 6 . In addition, the operation of GTs and combined-cycle operations is predominantly affected by the long-term operation. In addition, the output of the gas-turbine engine has relatively poor performance at part-load, and power output ( \({P}_{\mathrm{out}}\) ) deteriorates during hot seasons 7 , 8 . Najjar et al. 9 studied the performance diagnostics and the degradation of the GT cycle using actual data obtained from a combined-cycle power plant throughout 2 years of operation. It has been concluded that the degradation of the GT increases with temperature and load over time. In this context, the performance ratings of thermal power plants (TPPs) including reliability, availability, capacity factor, and efficiency, are expected to flocculate with the operation time.
CCGT is one type of power plant that directs the exhaust gas of the GT over a heat exchanger which generates steam at various levels of pressure 10 . The performance analysis of the combined-cycle gas turbine (CCGT) focuses mainly on evaluating the power efficiency of the plant 11 . The efficiency of a plant has a definite economic significance because heat inputs are the energy that is to be purchased at high temperatures, and net energy production is the return on the energy that is purchased. The GT running at lower inlet temperatures of the turbine produces low performance thus, lower efficiency 12 . The lower efficiency of the GT means that low \({P}_{\mathrm{out}}\) is produced. Several factors affecting the efficiency of TPPs in general, these include age, fuel type, capacity factor, and heat sink system 13 .
Sabouhi et al. 14 , investigated the reliability of a CCGT power plant using a developed model. The analysis involved modeling both GT and steam turbine power plants from an engineering system perspective, which provided the necessary data to estimate the reliability of the CCGT. Overall, the results point out to the most important components that help in selecting convenient strategies for the CCGT power plants 15 . On the other hand, Kolawole et al. 16 studied the availability, reliability, and capacity factor of a power generating plant using its historical data. It has been concluded that plant unavailability, the grid constraints, and gas restriction prevented the power plant from running at the maximum continuous rating. However, they suggested that in order to enhance the power supply, there is a demand for better maintenance, adequate gas supply, and the examination of the distribution and transmission units.
Lamfon et al. 17 studied the performance of a GT with a capacity of 23.7 MW operated at an ambient temperature between 30 and 45 °C. They reported an 11% improvement in the net \({P}_{\mathrm{out}}\) when the inlet of the GT engine is provided with cold air. However, an increase of 11% in the net \({P}_{\mathrm{out}}\) is also reported at an ambient temperature of 30 °C. This was based on the International Standard Organization (ISO) rated condition along with a 2% increase in \(\eta\) with a 2% decrease in specific fuel consumption. However, Ameri et al. 18 investigated the variation of ambient temperature on the \({P}_{\mathrm{out}}\) . It has been pointed out that if the ambient temperature drops from 34.2 °C to the ISO-rated condition an average increase in the \({P}_{\mathrm{out}}\) by as much as 11.3% can be achieved. It has also been reported that the \({P}_{\mathrm{out}}\) efficiency decreased by 0.74% when the ambient air temperature increased by 1 °C. In another similar study, Mohanty et al. 19 concluded that the net \({P}_{\mathrm{out}}\) decrease by 10% when the inlet air temperature increases by 30% from the ISO-rated conditions. Nevertheless, for small-scale GTs, this incline in the net \({P}_{\mathrm{out}}\) can be much greater. In addition, the study showed that raising the ambient temperature by 1 °C would result in about a 1% drop in the rated capacity of the GT.
Given the important role of energy in economic development in a country and the anticipated substantial future requirements, it is a key choice to conserve energy and make productive usage. The aim of this paper is to report on an evaluation method to assess the performance of a TPP in terms of availability, reliability, capacity factor, and thermal efficiency, for a period of 8 years (from 2010 to 2017). The proposed evaluation methodology will help in the performance diagnostics of similar power plants. Moreover, this evaluation is useful for linking maintenance to the overall performance of the power plant, thus, helping in maintenance scheduling.
Power plant description
The plant is situated in the northern part of Jordan, 70 km from the capital Amman. The region where the plant is located has a warm climate with average ambient temperature and relative humidity of 18 °C and 48% respectively. The plant is located within a rural region approximately 832 m above sea level.
The power plant consists of two simple-cycle GTs with a rated capacity of 30 MW each and a 297 MW CCGT comprised of two GTs which are nominally rated at 100 MW each and one ST with a rated capacity of 97 MW. The plant utilizes two types of fuels namely, diesel oil and natural gas and uses dry cooling fans for cooling. Figure 1 shows a schematic diagram of a CCGT.
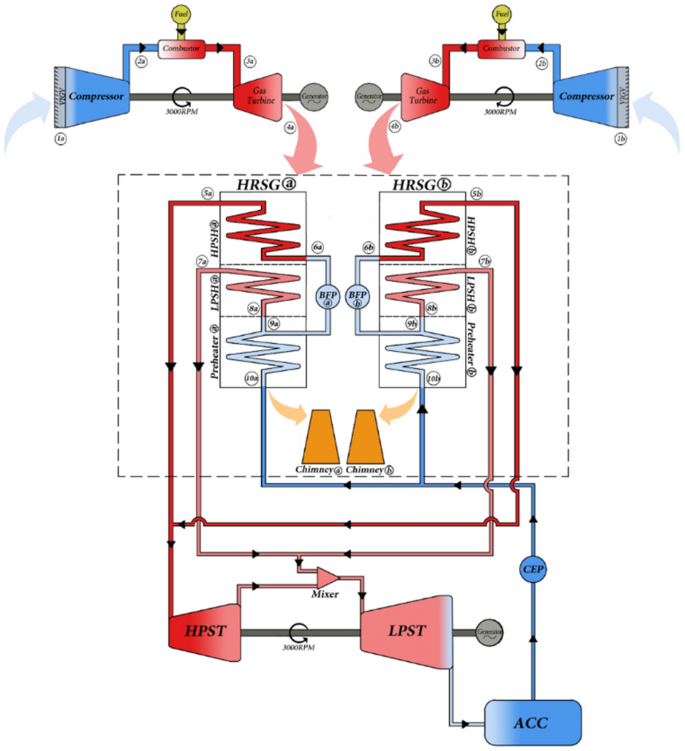
A schematic of process flow throughout a CCGT. Reprinted from Sustainable Energy Technologies and Assessments, 37, Najjar, Y. S. H., Alalul, O. F. A. & Abu-Shamleh, Degradation analysis of a combined cycle heat recovery steam generator under full and part load conditions. Sustainable Energy Technologies and Assessments, 100,587, 2020, with permission from Elsevier.
Data collection
The data of the power plant were obtained for a period of 8 years (2010–2017) from CEGCO annual reports 20 , 21 . Throughout the studied period, several major malfunctions occurred to the power plant. Though minor failures were also observed during the extended period, the major failures were observed for the years 2010, 2012, and 2013. These malfunctions affected the overall performance of the power plant and caused some of the generation units to be out of service for several days. The total number of days the malfunctioned units were out of service throughout the studied period is 206. Table 1 summarizes the major failures the power plant was exposed to during the period of the study.
The specific data that was used to evaluate the power plant performance includes, total installed capacity (MW), power output (MW), generated electrical energy ( \({E}_{g}\) ) (MWh), running hours (h), heat supply (MW), and heat rate (HR) (kJ/kWh). Table 2 shows the data of the power plant throughout the studied period.
Performance analysis
The performance evaluation was carried out based on statistical principles. The evaluation was carried out based on four key parameters namely, availability, reliability, capacity factor, and thermal efficiency. These parameters are calculated based on the data of the power plant and then compared to the best industrial practices and target values.
The forced outage factor (FOF) and the planned outage factor (POF) were both used to calculate the annual running hours of the TPP by subtracting the number of hours per year from both. FOF is defined as the shutdown of the plant as a result of undesired occurrences, whereas POF is defined as the prescheduled shutdown as for routine maintenance 22 . Equations 1 – 9 were used to calculate the identified 4 parameters. The calculated FOF and POF are presented in Table 3 .
The running hours of the power plant per each year can be calculated using the following equation:
where \(FOH\) is the forced outage hours. and \(POH\) is the planned outage hours.
The forced outage factor can be calculated as follows:
The planned outage factor can be calculated as follows:
The power output of the plant in MW was obtained as follows:
The availability of the power plant is calculated using the following equation:
The reliability of the power plant is calculated using the following equation:
The capacity factor of the power plant is calculated using the following equation:
The thermal efficiency ( \({\eta }_{th}\) ) of the power plant throughout the studied period is calculated as follows:
Finally, the HR of the power plant can be calculated as follows:

Results and discussion
The calculated values for each rating parameter across the studied period are shown in Table 4 . These values are fluctuated based on the performance of the TPP during the years. Ideally, the total generated power of the TPP during the 8 years can be calculated by multiplying the total installed capacity by the number of the years. In this case, the total generated power in MW should be 2856 MW, however, the actual generated power is less than that, due to the TPP being operated at part load conditions, along with other technical reasons including outage hours and malfunctions. The actual power generated throughout the studied period is approximately 1991.6 MW, meaning that 864.4 MW was lost. Overall, this operating loss does not mean that the plant is not achieving its purpose as most of the power plants are expected to have such huge losses during the years. In this context, the performance analysis is used to decide whether these losses are significant or not. Figure 2 illustrates the actual power generated to the installed generated power.
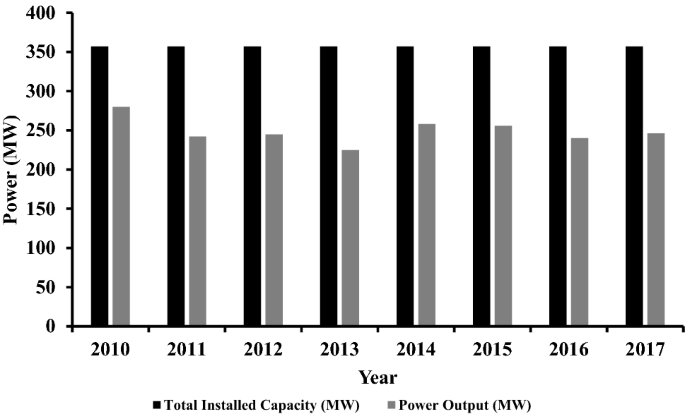
The generated power by the TPP throughout (2010–2017).
The availability factor of a power plant is the amount of time that it is able to produce electricity over a certain period, divided by the amount of time in the period 23 . The availability factor depends on the operation of the power plant, the fuel type, and the design of the plant. In this study, the availability of the studied TPP varies from 82.3 to 97.7%. Considering that the best industrial practices designate that GTs have a relatively high availability factor of 95% 24 , the TPP, on average, is not within the target value. Figure 3 shows the variation of the availability factor over the studied period.
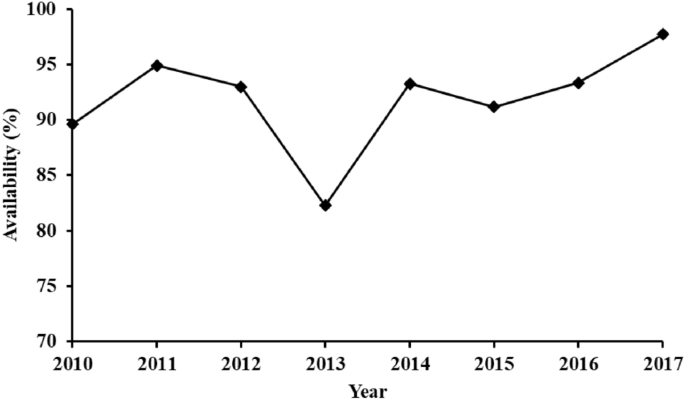
The availability factor of the TPP throughout (2010–2017).
The reliability analysis is an important step in the evaluation of CCGTs and plays a significant role in the operation of the plant in terms of maintenance scheduling 25 . However, the reliability factor of a power plant is mainly dependent upon the FOH and can be calculated by dividing the FOH by the actual time of operation. Over the studied period, the reliability of the TPP ranged between approximately 82.4% and 99.8% as shown in Fig. 4 . The lowest value has been obtained for the year 2013 due to one GT being out of service for a relatively large amount of time as mentioned in Table 1 . Nevertheless, the TPP’s average reliability was found to be 95.4%. Since there is no mentioning of the best industrial range of reliability for CCGTs in the literature, this percentage was compared to the starting reliability value of 99.9% 26 . This means that the reliability of the power plant is not within the best industrial practices. The high average percentage obtained can be attributed to the annual percentage of hours due to forced outage and the planned outage is around 4%.
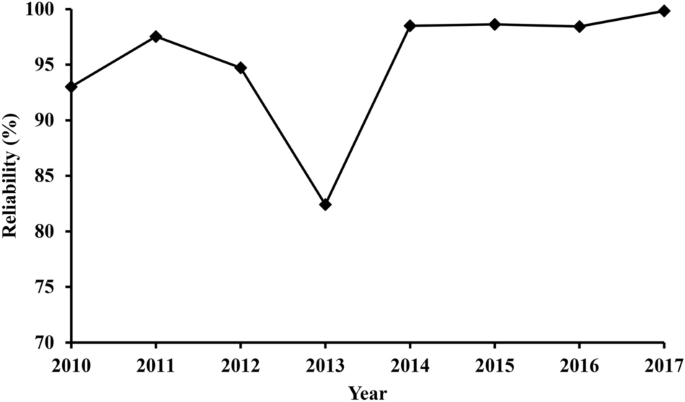
The reliability factor of the TPP throughout (2010–2017).
The capacity factor of a power plant is essentially a measure of its overall utilization 27 . For a power plant, the capacity factor can be calculated by dividing the actual electricity produced by its maximum possible electricity output throughout a certain period of time 28 . The average capacity factor of the TPP over the studied period is 70% with a minimum value of 65.6% obtained for 2013 and a maximum value of 76% for the year 2010. The flocculation of the capacity factor can be attributed to the age of the plant, outages, operation and maintenance, and weather conditions. Based on the international values, the average capacity factor of a power plant within a specific period should not be less than 65% 26 , meaning the power plant is within the normal ranges. Figure 5 shows the graph of the capacity factor throughout the studied years.
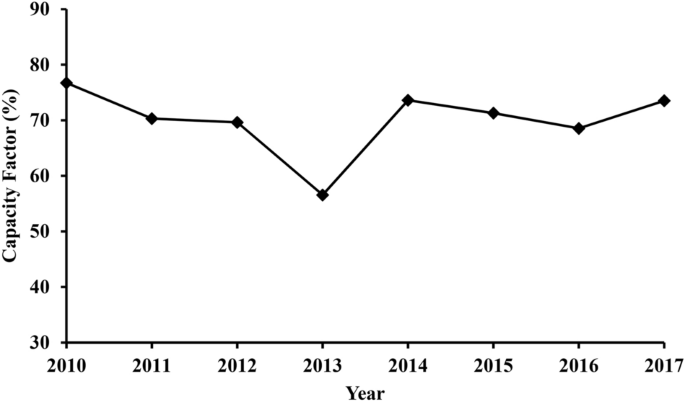
The capacity factor of the TPP throughout (2010–2017).
The thermal efficiency of the power plant is defined as the power output of the plant divided by the heat supplied. The thermal efficiency mainly depends on the heat value of the fuel used and the temperature. As the inlet temperature of the turbine increases, the thermal efficiency increases 29 . The thermal efficiency of the studied TPP throughout (2010–2017) is shown in Fig. 6 . The average thermal efficiency of the TPP is approximately 39.9%. The maximum obtained value is approximately 40.8% for both 2012 and 2016, while the minimum value is approximately 39.8% for the year 2013. Although the large malfunctions that occurred in 2013 do not directly affect the thermal efficiency, the cut in the fuel supply as a result does. Since the power plant is composed of two units (simple and combined), the obtained efficiency was compared to the target value of 49%. This value is the average between the approximate thermal efficiency of simple cycle gas power plants which is in the range of 35–42% 30 , and that average value for CCGTs which is 60% 31 .
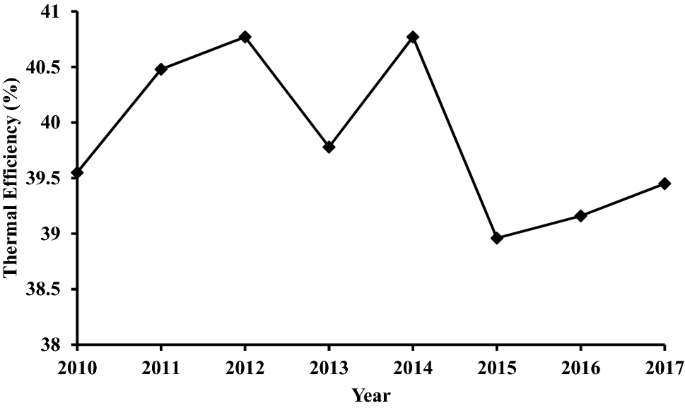
The thermal efficiency of the TPP throughout (2010–2017).
GTs are designed for standard air conditions. Nevertheless, the operating times are much greater at off-design conditions than at site conditions. When a GT operates at site environmental conditions different from the ISO, a distinction can be found between the actual power produced by a GT and the design-rated power marked on the GT. A comprehensive study and recording of operating data have shown that the ambient temperature is related to direct degradation of the GT capacity 32 . The GT loses 1% of its \({\eta }_{th}\) and 1.47 MW of gross capacity for each 1 °C increase in the ambient temperature above the ISO limit.
The GT's power generation output is dependent on the turbine inlet temperature. The temperature of the turbine inlet plays an important role in the performance of a cycle system. The efficiency of the component and the working temperature of the turbine affect the GT performance. The overall efficiency of the CCGT is mainly dependent on the compressor pressure ratio and turbine inlet temperature 33 . The key performance parameters are summarized and shown in Table 5 . These parameters are compared to the industry's best practices and target values.
Conclusions
In this study, a large-scale TPP was evaluated based on statistical principles. The evaluation was based on collected data from an actual power plant for a period of 8 years. The main performance parameters that were studied include availability, reliability, capacity factor, and thermal efficiency. These parameters were compared to the best industrial practices and target values. The average availability and reliability of the TPP throughout the studied period were found to be approximately 91% and 95% respectively. In addition, the average capacity factor and thermal efficiency over the studied period were found to be 70% and 40% respectively. The power losses out of the total generated electrical power over the studied period reached 864.4 MW. Several major malfunctions were reported over the studied period which caused the TPP to be out of service for a number of days. These malfunctions are linked to the overall performance of the TPP and the evaluated parameters. Overall, the analysis indicates that the plant is not within the context of the best industrial practices. Moreover, the evaluation methodology followed in this research can be useful in building maintenance schedules for such plants.
Recommendations
The CCGT efficiency and reliability can be improved considerably. The following are suggested ways to improve the performance of such a plant:
Increasing the inlet temperature of the turbine, taking into consideration the building material of the turbine can withstand high temperatures or by replacing the current parts with others that can withstand high temperatures. For 56 °C, the work output rises by around 10%, and the overall efficiency also increases by 1.5% 34 .
Suitable maintenance and cleaning of the inlet filters of the compressors. Dirty and poorly maintained filters cause significant efficiency loss as the compressor blades suffer clogging and damage. In addition, the pressure drop can be caused by dirty filters. Thus, Regular part checks and cleaning will boost plant performance and reliability.
Abbreviations
Combined-cycle gas turbine
Generated electrical energy (MWh)
Forced outage factor (%)
Forced outage hour (h)
Gas turbine
Heat rate (kJ/kWh)
International Organization for Standardization
Planned outage factor (%)
Planned outage hour (h)
Power output (MW)
Thermal power plant
Thermal efficiency (%)
Air compressor inlet
Combustor outlet
Gas turbine inlet
Gas turbine outlet
Inlet of high-pressure heat recovery steam generator
Outlet of high-pressure heat recovery steam generator
Inlet of low-pressure heat recovery steam generator
Outlet of low-pressure heat recovery steam generator
Inlet of heat recovery steam generator preheater
Outlet of heat recovery steam generator preheater
Ukwamba, S. I., Orhorhoro, E. K. & Omonoji, A. A. Performance evaluation of a simple gas turbine power plant using vapour absorption chiller. IOSR J. Mech. Civ. Eng. 15 , 13–18 (2018).
Google Scholar
Najjar, Y. S. H., Akyurt, M., Al-Rabghi, O. M. & Alp, T. Cogeneration with gas turbine engines. Heat Recov. Syst. CHP 13 , 471–480 (1993).
Article CAS Google Scholar
Najjar, Y. S. H. & Zaamout, M. S. Comparative performance of closed cycle gas turbine engine with heat recovery using different gases. Heat Recov. Syst. CHP 12 , 489–495 (1992).
Mohamed, O. & Khalil, A. Progress in modeling and control of gas turbine power generation systems: a survey. Energies 13 , 2358 (2020).
Article Google Scholar
Basrawi, F., Yamada, T., Nakanishi, K. & Naing, S. Effect of ambient temperature on the performance of micro gas turbine with cogeneration system in cold region. Appl. Therm. Eng. 31 , 1058–1067 (2011).
Najjar, Y. S. H., Abubaker, A. M. & El-Khalil, A. F. S. Novel inlet air cooling with gas turbine engines using cascaded waste-heat recovery for green sustainable energy. Energy 93 , 770–785 (2015).
Najjar, Y. S. H. & Abubaker, A. M. Indirect evaporative combined inlet air cooling with gas turbines for green power technology. Int. J. Refrig 59 , 235–250 (2015).
Najjar, Y. S. & Zaamout, M. S. Enhancing gas-turbine engine performance by means of the evaporative regenerative cycle. J. Inst. Energy 69 , 2–8 (1996).
CAS Google Scholar
Najjar, Y. S. H., Alalul, O. F. A. & Abu-Shamleh, A. Degradation analysis of a heavy-duty gas turbine engine under full and part load conditions. Int. J. Energy Res. 44 , 4529–4542 (2020).
Najjar, Y. S. H., Alalul, O. F. A. & Abu-Shamleh, A. Steam turbine bottoming cycle deterioration under different load conditions. Therm. Sci. Eng. Prog. 20 , 100733 (2020).
Orhorhoro, E. K., Polytechnic, D. S., Orhorhoro, O. W. & Polytechnic, D. S. Simulation of air inlet cooling system of a gas turbine power. ELK Asia Pac. J. Appl. Therm. Eng. 1 , 2394-0433 (2016).
Akyurt, M., Lamfon, N. J., Najjar, Y. S. H., Habeebullah, M. H. & Alp, T. Y. Modeling of waste heat recovery by looped water-in-steel heat pipes. Int. J. Heat Fluid Flow 16 , 263–271 (1995).
Aziz, N. L. A. A., Yap, K. S. & Bunyamin, M. A. A hybrid fuzzy logic and extreme learning machine for improving efficiency of circulating water systems in power generation plant. in IOP Conference Series: Earth and Environmental Science , Vol. 16 (2013).
Sabouhi, H., Abbaspour, A., Fotuhi-Firuzabad, M. & Dehghanian, P. Reliability modeling and availability analysis of combined cycle power plants. Int. J. Electr. Power Energy Syst. 79 , 108–119 (2016).
Ibrahim, T. K. et al. The optimum performance of the combined cycle power plant: a comprehensive review. Renew. Sustain. Energy Rev. 79 , 459–474 (2017).
Kolawole, A., Agboola, O. O., Ikubanni, P. P., Raji, O. G. & Okechukwu Osueke, C. Reliability and power loss analysis: a case study of a power plant in Nigeria. Cogent Eng. 6 , 1–13 (2019).
Lamfon, N. J., Najjar, Y. S. H. & Akyurt, M. Modeling and simulation of combined gas turbine engine and heat pipe system for waste heat recovery and utilization. Energy Convers. Manag. 39 , 81–86 (1998).
Ameri, M. & Hejazi, S. H. The study of capacity enhancement of the Chabahar gas turbine installation using an absorption chiller. Appl. Therm. Eng. 24 , 59–68 (2004).
Mohanty, B. & Paloso, G. Enhancing gas turbine performance by intake air cooling using an absorption chiller. Heat Recov. Syst. CHP 15 , 41–50 (1995).
Central Electricity Generating Company (CEGCO). Annual Report 2017 . (2017).
Central Electricity Generating Company (CEGCO). Annual Report 2013 . (2013).
Graf, R. F. O. in Modern Dictionary of Electronics , 7th edn. (ed. Graf, R. F.) 514–533 (Newnes, 1999). https://doi.org/10.1016/B978-0-08-051198-6.50020-5
Institute of Electrical and Electronics Engineers. Std 762-2006—IEEE Standard Definitions for Use in Reporting Electric Generating Unit Reliability, Availability, and Productivity (2006).
Oyedepo, S. O., Fagbenle, R. O., Adefila, S. S. & Adavbiele, S. A. Performance evaluation and economic analysis of a gas turbine power plant in Nigeria. Energy Convers. Manag. 79 , 431–440 (2014).
Dev, N., Kachhwaha, S. S. & Attri, R. Development of reliability index for combined cycle power plant using graph theoretic approach. Ain Shams Eng. J. 5 , 193–203 (2014).
Oyedepo, S. O., Fagbenle, R. O. & Adefila, S. S. Assessment of performance indices of selected gas turbine power plants in Nigeria. Energy Sci. Eng. 3 , 239–256 (2015).
Morales Pedraza, J. Chapter 4—Current Status and Perspective in the Use of Coal for Electricity Generation in the North America Region. In Conventional Energy in North America (ed. Morales Pedraza, J.) 211–257 (Elsevier, Amsderdam, 2019). https://doi.org/10.1016/B978-0-12-814889-1.00004-8 .
Chapter Google Scholar
Neill, S. P. & Hashemi, M. R. Chapter 1—Introduction. In Fundamentals of Ocean Renewable Energy (eds Neill, S. P. & Hashemi, M. R.) 1–30 (Academic Press, London, 2018). https://doi.org/10.1016/B978-0-12-810448-4.00001-X .
Alfellag, M. A. A. Parametric investigation of a modified gas turbine power plant. Therm. Sci. Eng. Prog. 3 , 141–149 (2017).
Willnow, K. Energy Efficiency Technologies ANNEX III Technical Report for Thermal Power Plants . WEC Knowledge Network (2013).
da Matto, H. A. et al. Combined cycle performance evaluation and dynamic response simulation. J. Aerosp. Technol. Manag. 8 , 491–497 (2016).
Najjar, Y. S. H., Alalul, O. F. A. & Abu-Shamleh, A. Degradation analysis of a combined cycle heat recovery steam generator under full and part load conditions. Sustain. Energy Technol. Assess. 37 , 100587 (2020).
Najjar, Y. S. A cryogenic gas turbine engine using hydrogen for waste heat recovery and regasification of LNG. Int. J. Hydrog. Energy 16 , 129–134 (1991).
William, O. E. Performance analysis of gas turbine power (a case study of Delta III GT9 Transcorp Gas Turbine Power Plant, Ughelli, Nigeria). Global Scientfic Journals 44–55 (2018).
Download references
Acknowledgements
The authors received no specific funding for this work.
Author information
Authors and affiliations.
Mechanical Engineering Department, Jordan University of Science and Technology, Irbid, Jordan
Yousef S. H. Najjar & Amer Abu-Shamleh
You can also search for this author in PubMed Google Scholar
Contributions
Yousef S. H. Najjar: Supervision. Amer Abu-Shamleh: Writing—original draft, methodology, investigation, validation, Writing—review & editing.
Corresponding author
Correspondence to Amer Abu-Shamleh .
Ethics declarations
Competing interests.
The authors declare no competing interests.
Additional information
Publisher's note.
Springer Nature remains neutral with regard to jurisdictional claims in published maps and institutional affiliations.
Rights and permissions
Open Access This article is licensed under a Creative Commons Attribution 4.0 International License, which permits use, sharing, adaptation, distribution and reproduction in any medium or format, as long as you give appropriate credit to the original author(s) and the source, provide a link to the Creative Commons licence, and indicate if changes were made. The images or other third party material in this article are included in the article's Creative Commons licence, unless indicated otherwise in a credit line to the material. If material is not included in the article's Creative Commons licence and your intended use is not permitted by statutory regulation or exceeds the permitted use, you will need to obtain permission directly from the copyright holder. To view a copy of this licence, visit http://creativecommons.org/licenses/by/4.0/ .
Reprints and permissions
About this article
Cite this article.
Najjar, Y.S.H., Abu-Shamleh, A. Performance evaluation of a large-scale thermal power plant based on the best industrial practices. Sci Rep 10 , 20661 (2020). https://doi.org/10.1038/s41598-020-77802-8
Download citation
Received : 10 April 2020
Accepted : 10 November 2020
Published : 26 November 2020
DOI : https://doi.org/10.1038/s41598-020-77802-8
Share this article
Anyone you share the following link with will be able to read this content:
Sorry, a shareable link is not currently available for this article.
Provided by the Springer Nature SharedIt content-sharing initiative
By submitting a comment you agree to abide by our Terms and Community Guidelines . If you find something abusive or that does not comply with our terms or guidelines please flag it as inappropriate.
Quick links
- Explore articles by subject
- Guide to authors
- Editorial policies
Sign up for the Nature Briefing newsletter — what matters in science, free to your inbox daily.

Information
- Author Services
Initiatives
You are accessing a machine-readable page. In order to be human-readable, please install an RSS reader.
All articles published by MDPI are made immediately available worldwide under an open access license. No special permission is required to reuse all or part of the article published by MDPI, including figures and tables. For articles published under an open access Creative Common CC BY license, any part of the article may be reused without permission provided that the original article is clearly cited. For more information, please refer to https://www.mdpi.com/openaccess .
Feature papers represent the most advanced research with significant potential for high impact in the field. A Feature Paper should be a substantial original Article that involves several techniques or approaches, provides an outlook for future research directions and describes possible research applications.
Feature papers are submitted upon individual invitation or recommendation by the scientific editors and must receive positive feedback from the reviewers.
Editor’s Choice articles are based on recommendations by the scientific editors of MDPI journals from around the world. Editors select a small number of articles recently published in the journal that they believe will be particularly interesting to readers, or important in the respective research area. The aim is to provide a snapshot of some of the most exciting work published in the various research areas of the journal.
Original Submission Date Received: .
- Active Journals
- Find a Journal
- Proceedings Series
- For Authors
- For Reviewers
- For Editors
- For Librarians
- For Publishers
- For Societies
- For Conference Organizers
- Open Access Policy
- Institutional Open Access Program
- Special Issues Guidelines
- Editorial Process
- Research and Publication Ethics
- Article Processing Charges
- Testimonials
- Preprints.org
- SciProfiles
- Encyclopedia
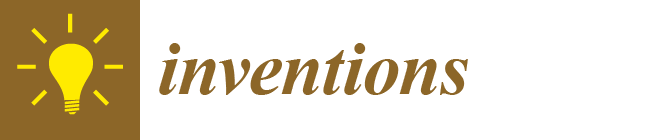
Article Menu

- Subscribe SciFeed
- Recommended Articles
- Google Scholar
- on Google Scholar
- Table of Contents
Find support for a specific problem in the support section of our website.
Please let us know what you think of our products and services.
Visit our dedicated information section to learn more about MDPI.
JSmol Viewer
Research and development of the combined cycle power plants working on supercritical carbon dioxide.
1. Introduction
1.1. promising methods to increase the ccgt plant efficiency, 1.2. replacing the water stream circuit with carbon dioxide for ccgt plants, 1.3. current state of research on carbon dioxide cycles, 1.4. approaches to energy cycle optimisation, 2. materials and methods, 2.1. research object, 2.2. modeling method, 2.3. validation of the modelling, 3. results and discussion, 3.1. optimisation of the co 2 brayton cycle with recompression, 3.2. optimisation of the basic version of the co 2 brayton cycle, 4. conclusions.
- The thermal scheme and mathematical model for the gas turbine combined cycle working on CO 2 have been developed. The optimal values of the key thermodynamic parameters have been identified for the case of gas turbine unit GTE-160. It has been established, that at a temperature of 517 °C, the efficiency of the carbon dioxide Brayton cycle with recompression could be equal to 43.41% and achieve inlet and outlet turbine pressures of 24.0 and 8.5 MPa, respectively, and at the recompression percentage of 37.5%.
- The maximum net power generation of 55.1 MW is achieved with a zero-recompression ratio with a net efficiency of 37.98% at a pressure of 30 MPa. This happens due to the fact that the temperature of carbon dioxide at the inlet to the waste heat boiler decreases, therefore, the heat supply to the highly efficient cycle increases.
- When optimizing the secondary circuit, it was found that during the operation of the main Brayton cycle, the highest efficiency of the combined cycle is observed at pressures at the inlet and outlet of the turbine equal to 32 MPa and 8 MPa, at which the efficiency reaches 19.6%.
- Based on the mathematical simulation, it was found that replacing the conventional steam power plant, which operates in combination with GTE-160 gas turbine plant and uses carbon dioxide Brayton cycles, with recompression and base version provides a 1.2% increase in the net efficiency of the combined power plant. Such an increase in efficiency can be explained by a high average integral temperature of the heat supply in the Brayton cycle, the carbon dioxide temperature upstream of HE1 being about 311 °C, whereas, in the conventional design, this value is about 100 °C (the temperature downstream of the atmospheric deaerator).
Author Contributions
Institutional review board statement, informed consent statement, data availability statement, conflicts of interest.
- World Energy Balances—International Energy Agency. Available online: https://www.iea.org/data-and-statistics/data-product/world-energy-balances (accessed on 1 August 2022).
- Uvarov, A.; Antonova, A.; Vorobjev, A. The Analysis of Initial Parameters of Steam in the Combined-Cycle Plant with High Temperature Gas Turbine. MATEC Web Conf. 2015 , 37 , 01062. [ Google Scholar ] [ CrossRef ]
- Rogalev, A.; Komarov, I.; Kindra, V.; Osipov, S. Methods for Competitiveness Improvement of High-Temperature Steam Turbine Power Plants. Inventions 2022 , 7 , 44. [ Google Scholar ] [ CrossRef ]
- Vélez, F.; Segovia, J.J.; Martín, M.C.; Antolín, G.; Chejne, F.; Quijano, A. A technical, economical and market review of organic Rankine cycles for the conversion of low-grade heat for power generation. Renew. Sustain. Energy Rev. 2012 , 16 , 4175–4189. [ Google Scholar ]
- Rogalev, N.; Kindra, V.; Komarov, I.; Osipov, S.; Zlyvko, O.; Lvov, D. Comparative Analysis of Low-Grade Heat Utilization Methods for Thermal Power Plants with Back-Pressure Steam Turbines. Energies 2021 , 14 , 8519. [ Google Scholar ] [ CrossRef ]
- Rogalev, A.; Rogalev, N.; Kindra, V.; Zlyvko, O.; Vegera, A. A Study of Low-Potential Heat Utilization Methods for Oxy-Fuel Combustion Power Cycles. Energies 2021 , 14 , 3364. [ Google Scholar ] [ CrossRef ]
- Cao, Y.; Mihardjo, L.W.; Dahari, M.; Tlili, I. Waste heat from a biomass fueled gas turbine for power generation via an ORC or compressor inlet cooling via an absorption refrigeration cycle: A thermoeconomic comparison. Appl. Therm. Eng. 2021 , 182 , 116117. [ Google Scholar ] [ CrossRef ]
- Kindra, V.; Rogalev, N.; Osipov, S.; Zlyvko, O.; Naumov, V. Research and Development of Trinary Power Cycles. Inventions 2022 , 7 , 56. [ Google Scholar ] [ CrossRef ]
- Braimakis, K.; Karellas, S. Energetic Optimization of Regenerative Organic Rankine Cycle (ORC) Configurations. Energy Convers. Manag. 2018 , 159 , 353–370. [ Google Scholar ] [ CrossRef ]
- Sun, W.; Yue, X.; Wang, Y. Exergy Efficiency Analysis of ORC (Organic Rankine Cycle) and ORC-Based Combined Cycles Driven by Low-Temperature Waste Heat. Energy Convers. Manag. 2017 , 135 , 63–73. [ Google Scholar ] [ CrossRef ]
- Shi, L.; Shu, G.; Tian, H.; Deng, S. A Review of Modified Organic Rankine Cycles (ORCs) for Internal Combustion Engine Waste Heat Recovery (ICE-WHR). Renew. Sustain. Energy Rev. 2018 , 92 , 95–110. [ Google Scholar ] [ CrossRef ]
- Xu, J.; Liu, C.; Sun, E.; Xie, J.; Li, M.; Yang, Y.; Liu, J. Perspective of S−CO2 Power Cycles. Energy 2019 , 186 , 115831. [ Google Scholar ] [ CrossRef ]
- Song, J.; Li, X.; Ren, X.; Gu, C. Performance Analysis and Parametric Optimization of Supercritical Carbon Dioxide (S-CO2) Cycle with Bottoming Organic Rankine Cycle (ORC). Energy 2018 , 143 , 406–416. [ Google Scholar ] [ CrossRef ]
- Song, J.; Li, X.-S.; Ren, X.-D.; Gu, C.-W. Performance improvement of a preheating supercritical CO2 (S-CO2) cycle based system for engine waste heat recovery. Energy Convers. Manag. 2018 , 161 , 225–233. [ Google Scholar ] [ CrossRef ]
- Dostal, V.; Hejzlar, P.; Driscoll, M.J. Supercritical Carbon Dioxide Cycle for Next Generation Nuclear Reactors. Ph.D. Thesis, MIT Center for Advanced Nuclear Energy Systems (CANES), Cambridge, MA, USA, 2004. [ Google Scholar ]
- Angelino, G. Real Gas Effects in Carbon Dioxide Cycles. In Gas Turbine Conference and Products Show ; American Society of Mechanical Engineers: Cleveland, OH, USA, 1969; p. V001T01A071. [ Google Scholar ]
- Reyes-Belmonte, M.A.; Sebastián, A.; Romero, M.; González-Aguilar, J. Optimization of a recompression supercritical carbon dioxide cycle for an innovative central receiver solar power plant. Energy 2016 , 112 , 17–27. [ Google Scholar ] [ CrossRef ]
- Rogalev, A.; Kindra, V.; Komarov, I.; Osipov, S.; Zlyvko, O. Structural and Parametric Optimization of S-CO 2 Thermal Power Plants with a Pulverized Coal-Fired Boiler Operating in Russia. Energies 2021 , 14 , 7136. [ Google Scholar ] [ CrossRef ]
- Liu, Y.; Wang, Y.; Huang, D. Supercritical CO 2 Brayton Cycle: A State-of-the-Art Review. Energy 2019 , 189 , 115900. [ Google Scholar ]
- Cheng, W.-L.; Huang, W.-X.; Nian, Y.-L. Global Parameter Optimization and Criterion Formula of Supercritical Carbon Dioxide Brayton Cycle with Recompression. Energy Convers. Manag. 2017 , 150 , 669–677. [ Google Scholar ] [ CrossRef ]
- Thanganadar, D.; Asfand, F.; Patchigolla, K. Thermal Performance and Economic Analysis of Supercritical Carbon Dioxide Cycles in Combined Cycle Power Plant. Appl. Energy 2019 , 255 , 113836. [ Google Scholar ] [ CrossRef ]
- Invernizzi, C.M.; Iora, P. The exploitation of the physical exergy of liquid natural gas by closed power thermodynamic cycles. An overview. Energy 2016 , 105 , 2–15. [ Google Scholar ] [ CrossRef ]
- Angelino, G.; Invernizzi, C.M. Carbon dioxide power cycles using liquid natural gas as heat sink. Appl. Therm. Eng. 2009 , 29 , 2935–2941. [ Google Scholar ] [ CrossRef ]
- Qiang, W.; Yanzhong, L.; Xi, C. Exergy analysis of liquefied natural gas cold energy recovering cycles. Int. J. Energy Res. 2005 , 29 , 65–78. [ Google Scholar ] [ CrossRef ]
- Bellos, E.; Tzivanidis, C. Parametric Analysis of a Polygeneration System with CO 2 Working Fluid. Appl. Sci. 2021 , 11 , 3215. [ Google Scholar ] [ CrossRef ]
- Li, X.; Huang, H.; Zhao, W. A supercritical or transcritical Rankine cycle with ejector using low-grade heat. Energy Convers. Manag. 2014 , 78 , 551–558. [ Google Scholar ] [ CrossRef ]
- Walraven, D.; Laenen, B.; D’haeseleer, W. Optimum configuration of shell-and-tube heat exchangers for the use in low-temperature organic Rankine cycles. Energy Convers. Manag. 2014 , 83 , 177–187. [ Google Scholar ] [ CrossRef ]
- Martelli, A.; Martelli, E.; Pierobon, L. Thermodynamic and technoeconomic optimization of Organic Rankine Cycle systems. In Organic Rankine cycle (ORC) Power Systems ; Woodhead Publishing: Cambridge, UK, 2017; pp. 173–249. [ Google Scholar ]
- Biegler, L.; Cuthrell, J. Improved infeasible path optimization for sequential modular simulators—II: The optimization algorithm. Comput. Chem. Eng. 1985 , 9 , 257–267. [ Google Scholar ] [ CrossRef ]
- Shcherbatov, I.; Agibalov, V.; Dolgsuhev, A.; Belov, M. Subsystem for building a digital twin of the main and auxiliary equipment of thermal scheme of thermal power plant. In Society 5.0: Human-Centered Society Challenges and Solutions ; Springer: Cham, Swizterland, 2022; pp. 239–249. [ Google Scholar ]
- Sarkar, J.; Bhattacharyya, S. Optimization of recompression S-CO 2 power cycle with reheating. Energy Convers. Manag. 2009 , 50 , 1939–1945. [ Google Scholar ] [ CrossRef ]
Click here to enlarge figure
Parameter | Value |
---|---|
GT flue gas massflow, kg/s | 509 |
GT inlet temperature, °C | 1200 |
GT inlet pressure, MPa | 12 |
GT pressure ratio | 10.9 |
Heater the first cycle hot end temperature difference, °C | 20 |
Heater the second cycle hot end temperature difference, °C | 10 |
Regenerator temperature difference, °C | 10 |
Turbine outlet temperature (GTE-160), °C | 537 |
Exhaust gas mass flow (GTE-160), kg/s | 509 |
Electrical net efficiency (GTE-160), % | 34.4 |
The cycle minimal temperature, °C | 30 |
Cooler circulation water pressure, bar | 1.3 |
Turbine specific internal efficiency, % | 90 |
Compressor specific internal efficiency, % | 85 |
Mechanical efficiency, % | 99 |
Power generator efficiency, % | 99 |
Power motor efficiency, % | 99 |
Heat transportation efficiency, % | 99 |
Tmin, °C | Tmax, °C | Pmax, Bar | rcopt | Xopt | ηth, % [ ] | ηth, % (This Work) | Delt |
---|---|---|---|---|---|---|---|
50 | 550 | 200 | 2.39 | 0.1837 | 36.71 | 36.93 | 0.599 |
50 | 550 | 300 | 2.8 | 0.254 | 38.93 | 39.021 | 0.234 |
32 | 550 | 200 | 2.64 | 0.3337 | 41.18 | 41.5 | 0.777 |
32 | 550 | 300 | 3.86 | 0.3549 | 43.32 | 43.17 | 0.346 |
Optimized Cycle | Initial Temperature, °C | Initial Pressure, MPa | Share of Recompression, % | Final Pressure, MPa | Net Efficiency, % |
---|---|---|---|---|---|
Recompression cycle | 517 | 30 | 0 | 8.5 | 37.91 |
Basic cycle version | 311 | 32 | - | 8.0 | 21.98 |
MDPI stays neutral with regard to jurisdictional claims in published maps and institutional affiliations. |
Share and Cite
Rogalev, A.; Rogalev, N.; Kindra, V.; Komarov, I.; Zlyvko, O. Research and Development of the Combined Cycle Power Plants Working on Supercritical Carbon Dioxide. Inventions 2022 , 7 , 76. https://doi.org/10.3390/inventions7030076
Rogalev A, Rogalev N, Kindra V, Komarov I, Zlyvko O. Research and Development of the Combined Cycle Power Plants Working on Supercritical Carbon Dioxide. Inventions . 2022; 7(3):76. https://doi.org/10.3390/inventions7030076
Rogalev, Andrey, Nikolay Rogalev, Vladimir Kindra, Ivan Komarov, and Olga Zlyvko. 2022. "Research and Development of the Combined Cycle Power Plants Working on Supercritical Carbon Dioxide" Inventions 7, no. 3: 76. https://doi.org/10.3390/inventions7030076
Article Metrics
Article access statistics, further information, mdpi initiatives, follow mdpi.
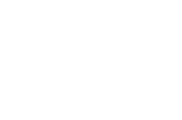
Subscribe to receive issue release notifications and newsletters from MDPI journals
- Search Menu
- Sign in through your institution
- Advanced Articles
- Editor's Choice
- Author Guidelines
- Publish with us
- Submission Site
- Open Access
- Self-Archiving Policy
- About Clean Energy
- About the National Institute of Clean and Low-Carbon Energy
- Editorial Board
- Instructions for Reviewers
- Advertising & Corporate Services
- Journals Career Network
- Journals on Oxford Academic
- Books on Oxford Academic

Article Contents
Introduction, 1 methods and challenges addressed, 2 ai techniques, 3 application of ai in the power sector, 4 conclusions, 5 future scope, authors’ contributions, conflict of interest statement, data availability.
- < Previous
Applications of artificial intelligence in power system operation, control and planning: a review

- Article contents
- Figures & tables
- Supplementary Data
Utkarsh Pandey, Anshumaan Pathak, Adesh Kumar, Surajit Mondal, Applications of artificial intelligence in power system operation, control and planning: a review, Clean Energy , Volume 7, Issue 6, December 2023, Pages 1199–1218, https://doi.org/10.1093/ce/zkad061
- Permissions Icon Permissions
As different artificial intelligence (AI) techniques continue to evolve, power systems are undergoing significant technological changes with the primary goal of reducing computational time, decreasing utility and consumer costs and ensuring the reliable operation of an electrical power system. AI techniques compute large amounts of data at a faster speed than numerical optimization methods with higher processing speeds. With these features, AI techniques can further automate and increase the performance of power systems. This paper presents a comprehensive overview of diverse AI techniques that can be applied in power system operation, control and planning, aiming to facilitate their various applications. We explained how AI can be used to resolve system frequency changes, maintain the voltage profile to minimize transmission losses, reduce the fault rate and minimize reactive current in distributed systems to increase the power factor and improve the voltage profile.

The demand for advanced research and technology has steadily increased in the sector of electric grids [ 1 ]. Automation and intelligent technology have become widely used in response to development demands over time. Traditional research methods are quickly becoming insufficient to enable data scientists and researchers to keep up with any global challenges that artificial intelligence (AI) may be able to assist us in solving and uncovering important insights among the billions of pieces of data scattered throughout power systems. AI can handle large amounts of data and utilize them to make power system operations, control and planning more efficient. The use of AI technology in power systems has been investigated and debated in related areas and has resulted in more study material and certain outcomes, which are reviewed in this paper. The demand for advanced research and technology has constantly risen in the sector of electricity grids. The application of AI technology to the automation of power system control can improve the efficiency of electrical automation management, mitigate the risk of accidents and ensure smooth operation of the power system over an extended period [ 2 ]. Evaluating the use of AI technology in power systems requires a comprehensive analysis of existing research in the field of artificial intelligence and its related industries.
The power system is a network consisting of three components: generation, distribution and transmission. In the power system, energy sources (such as coal, sunlight, wind, nuclear reactions and diesel) are transformed into electrical energy [ 3 ]. There are different power systems, such as solar power systems, wind power systems, thermal power plants [ 4 ], nuclear power plants, geothermal power plants, etc. All power systems have different structures and equipment for the generation of electricity [ 5 ]. The basic structure of a power system includes:
(i) generating substation;
(ii) transmission substation;
(iii) sub-transmission substation;
(iv) distribution substation.
In power system problem-solving, conventional approaches such as practical numerical optimization methods (e.g. lambda iteration and Newton–Rapson methods) have been used. Optimization problems are non-linear and, with the various constraints included, these optimization problems become slow and complex. So, several AI techniques are discussed here to solve many optimization issues with less computation time. Furthermore, experiments were conducted to identify which backpropagation algorithm would give the most efficient and reliable network training [ 6 ]. The systematic approach was introduced [ 7 ] for developing a recurrent neural network (RNN) that could effectively predict the dynamic behaviour of a pilot-scale entrained-flow gasifier. The RNN was trained using a data set consisting of input and output data collected from a dynamic read-only memory (ROM) system established in a previous study. To evaluate the accuracy of the RNN, comparisons were made with computational fluid dynamics models and experimental data obtained from the pilot-scale gasifier. The findings demonstrated that the RNN surpassed the performance of the dynamic ROM model, showing strong predictive capabilities to capture the transient behaviour of the gasifier. Additionally, this was achieved while significantly reducing the computational resources required. Power systems are one of the main study topics for the advanced development of AI. The use of AI algorithms in power plants has been under continual investigation since the advent of the expert system technique [ 8 ]. However, in most situations, issues such as long cycle times, complex computation and difficulty in learning arise with classic AI methods. In recent years, efficiency has increased significantly with the continued advancement of AI algorithms. The multi-source model of heterogeneous large data has gradually developed, the data volume continues to grow, and new possibilities and problems are being created with the use of AI in power systems. AI encompasses several technologies such as expert systems, pattern recognition, genetic algorithms (GAs) and neural networks. By incorporating AI into the automation of power system control, it has the potential to enhance the efficiency of electrical automation management, mitigate the risk of accidents and ensure long-term smooth operation of the power system. Machine learning (ML) has also found extensive applications in predicting the properties of rechargeable battery materials, particularly electrolyte and electrode materials, as well as the development of novel materials, according to relevant research. The breadth of ML applications will grow steadily as ML technology advances and new unique issues emerge in the research of rechargeable battery material [ 9 ]. Although ML has shown considerable potential in modelling complex systems, its implementation introduces new challenges. These challenges include difficulties associated with accessing relevant and reliable data sets, and addressing inaccuracies in model predictions needs to be addressed before ML can be widely deployed. To effectively implement ML on a large scale, it is crucial to have compelling evidence of its effectiveness in diverse areas such as manufacturing processes, energy generation, storage and management. Furthermore, the availability of commercial software and a skilled workforce specialized in the relevant domains is essential [ 10 ]. ML has proven to be beneficial in creating data-driven models that accurately correlate material properties with catalytic performance, including activity, selectivity and stability. As a result, there have been advancements in the development of effective design and screening criteria for solid-state catalysts with desired properties [ 11 ]. Nevertheless, there are still difficulties in applying existing ML algorithms to accurately predict catalyst performance or devise strategies for designing high-performance catalysts. The review emphasizes recent advancements in ML applied to solid heterogeneous catalysis, as well as the limitations and constraints faced by ML in this field, and also discusses some of the prospects for using ML effectively in the design of solid heterogeneous catalysts. Successful uses of ML in short-term hydrothermal scheduling will strengthen the link between real operations and issue formulation, and prepare the hydropower sector for autonomy by identifying the need for and availability of autonomous systems now and in the future. In this research, a review of the state of the art of ML applications for the hydroelectric sector was offered [ 12 ]. Digital technologies have a significant impact on energy market services and the safety of residents and energy consumers, particularly in smart homes. Sustainable smart home networks can improve energy efficiency, utilize local renewable energy, decarbonize heating and cooling systems, and promote responsible electric vehicle charging [ 13 ]. The next decade is crucial for achieving ambitious global CO 2 reduction targets and the decarbonization of buildings is a major challenge. Water-efficient development and resilient homes are essential for coping with impacts of climate change. Research on sustainability and energy efficiency is vital to improve the quality of life in the face of climate change [ 14 ]. Several important aspects were highlighted [ 15 ] with respect to the current state and prospects of smart homes. It was recognized that despite the increasing prevalence of smart homes and the growing familiarity with them, there were still significant obstacles that researchers must address to achieve widespread adoption [ 16 ].
One of the technical hurdles highlighted is the diversity of manufacturers and devices, each with varying charging systems, frequencies and communication methods [ 17 ]. This fragmentation can hinder interoperability and compatibility between devices and systems. The greater acceptance of smart home technologies poses a significant challenge. This review highlights the crucial task of convincing consumers about the safety and reliability of these technologies. An approach [ 18 ] was presented to develop prediction models that were capable of identifying faults and malfunctions in power equipment, demonstrating their effectiveness in predicting the progression of degradation phenomena. The challenges were discussed [ 19 ] associated with predicting the technical condition index of the equipment and determining the probability of its current state having defects. This research contributes significantly to the advancement of predictive analytics tools in the industry, enabling proactive maintenance of equipment. ML and data-driven approaches exhibit significant promise in the field of predictive analysis within power systems, especially in the context of smart grids. These methods can efficiently analyse the vast amounts of data collected from smart meters and other devices in real time, facilitating optimized energy flow in an increasingly renewable-energy-focused landscape [ 20 ]. They offer advantages such as improved accuracy, cost reduction and improved efficiency. However, certain challenges must be overcome, such as ensuring the availability of high-quality data and managing the potential risk of information overload [ 21 ].
The articles selected for review are based on different parameters and selection criteria. The shortlist is based on parameters such as duration, analysis, comparison and applications, as listed in Table 1 . The challenges investigated for power system operations, control and planning in the article are as follows and a diagram visualizing the domains of the power sector along with the AI techniques used and their application is presented as Fig. 1 . Power system operation [ 22 ] includes the total power requirement that must reliably meet the real-time generation, including transmission losses. The problems involved in this task are economic load dispatch (ELD), power flow, unit commitment and generator maintenance schedule.
Selection criteria for shortlisted research papers
Parameters . | Selection criteria . | Elimination criteria . |
---|---|---|
Duration | Research articles published between 2007 and 2021 | Research articles published before 2007 |
Analysis | The research includes various artificial intelligence techniques and applications in power systems | The research includes various operators and modifications in artificial intelligence techniques in power systems |
Comparison | Research concentrates on variations in artificial intelligence power system approaches | Research is focused on several metaheuristics variations. Genetic algorithms were part of some studies |
Applications | Multimedia research, operational management and wireless networks are included | Research includes engineering, data mining, software and astronomical applications |
Study | Mathematical foundations and experimental results are part of the research | Patents, cases and publications are included in the research |
Parameters . | Selection criteria . | Elimination criteria . |
---|---|---|
Duration | Research articles published between 2007 and 2021 | Research articles published before 2007 |
Analysis | The research includes various artificial intelligence techniques and applications in power systems | The research includes various operators and modifications in artificial intelligence techniques in power systems |
Comparison | Research concentrates on variations in artificial intelligence power system approaches | Research is focused on several metaheuristics variations. Genetic algorithms were part of some studies |
Applications | Multimedia research, operational management and wireless networks are included | Research includes engineering, data mining, software and astronomical applications |
Study | Mathematical foundations and experimental results are part of the research | Patents, cases and publications are included in the research |

Visual depiction of power sector domains, their application and AI techniques used
The complex and large design of the power system is presented [ 23 ] and interferences in the power system are a problem. When a large interference occurs, control tasks are needed to find the disturbed area, control the impact caused and bring the process to normal form. Heuristic solutions are non-linear and hence are not designed to deal with fast-occurring disturbances. Therefore, many control optimization techniques such as voltage control (VC), power system stability control and load frequency control are discussed to address this problem.
Power system planning has an arrangement of a power system that is complex and large with many parts such as flexible alternating current transmission system (FACTS) devices and distribution systems. The major goal of least-cost planning is to optimize the components required to deliver enough power at a minimal cost. Many factors such as FACTS placement and demand are given importance in the expansion of power system planning. Reactive power optimization, distribution system planning (DSP) [ 24 ] and capacitor placement are the optimization problems considered in this task [ 25 ].
2.1 Artificial neural network
In AI, a set of inputs is transformed into an output using a network of neurons. A neuron produces a single power by simply operating its input in the same way as a processor [ 26 ]. The working group of neurons and the pattern of their connections may be utilized to build computers with real-world issues in model recognition and pattern categorization. As the human brain processes are replicated, input signals are processed using mathematical operations utilizing artificial neurons.
The network consists of neurons organized in layers and connected to ensure information input–output flows [ 27 ]. By using what is known as the activation function, in layer ‘i’, each neuron is linked to the ‘i+1’ layer of all neurons. The input signals for a specific neuron originate from all neurons in the prior layer and their excitation power changes to govern the degree of signal reaching each neuron [ 28 ].
In several scientific disciplines, such as medical diagnosis, voice, pattern recognition, etc., artificial neural networks (ANNs) are utilized. The ANN is a computing system partly based on biological neural networks, expressed by linked nodes (artificial neurons), correctly structured in layers that are found in human or animal brains. All artificial neurons are linked and are able, employing their connections (synapses) to send signals, generally real values, which result in an output computed according to the original input, depending on the sizes allocated to all neurons [ 29 ].
ANNs are recognized as data-mining approaches capable of modelling several independent characteristics with dependent functions in non-linear functions. ANNs may predict a future value of a dependent variable after training with a comparable sample, replicating the learning process of a human brain [ 30 ].
In turn, a difference in signal strength affects the activation of the neuron and, as a result, signals that are transmitted to other neurons as shown in Fig. 2 .

Simplified diagram of the artificial neural network
Input layer—distribute other units but does not process the data.
Hidden layer—the ability to map the non-linear problems is provided through hidden layers.
Output later—the output units encode the value to be assigned to this instance.
2.2 Adaptive neuro-fuzzy interference system
The adaptive neuro-fuzzy interference system (ANFIS) creates an input/output data set whose membership function parameters are modified with the minimum square method type or the backpropagation algorithm by itself, using a fuzzy interference system [ 31 ]. This modification has helped the fuzzy system learn from the data it models. By applying hybrid learning, ANFIS utilizes a systematic approach to determine the optimal distribution of membership functions, enabling effective mapping of the relationship between input and output data [ 32 ]. The ANFIS architecture combines ANNs with fuzzy logic, making the modelling process more structured and less dependent on expert knowledge. This inference system is constructed using five layers in its basic form. Each ANFIS layer has several nodes defined in the layer specification using the node function. The current layer inputs from the preceding layer nodes are collected. The structure of the ANFIS is shown in Fig. 3 [ 33 ].

Simplified diagram of an artificial neuro-fuzzy interference system
The fuzzy-inference method involves organizing empirical information in a professional manner, which presents challenges in quantifying it through membership functions (MFs) and fuzzy rule bases [ 34 ]. Additionally, neural networks possess learning capabilities. From top to bottom, they are very adaptable in their system set-up and have great parallel processing and fault tolerance. The theories for neural network neuro-fuzzy systems are actively explored in several areas [ 35 ].
The utilization of a neuro-fuzzy system, which emulates human learning and decision-making abilities, can lead to varying model performances compared with traditional mathematical approaches. The process of rule generation and grouping in a neuro-fuzzy inference system, adapted to the specific model, can be approached through a grid-based methodology, known as the ANFIS.
2.3 Fuzzy logic
To identify the fuzzy set from which the value comes and the degree of membership within that set, fuzzy logic systems base their choices on input in terms of variables generated from the member functions [ 36 ]. The variables are then combined with IF–THEN language requirements (fuzzy logic rules) and a fluid implication is used to answer each rule [ 37 ]. The response of each rule is weighted according to the confidence or degree of the inputs of each rule and the central part of answers is computed to provide a suitable output and achieve the compositional rule of deference. Now, the design of fuzzy logic systems is not a systematic approach. The easiest way is to subjectively define member functions and rules with a human-operated system or an existing controller and then test the design for the right output. If the design fails the testing, the MFs and/or rules should be changed. Recent investigation directions involve the creation of fuzzy logic systems that can learn from experience.
Currently, only published findings can create and modify fuzzy control rules based on experience [ 38 ]. Among them can be Scharf’s self-organizing robotic control system [ 39 ] by using a performance matrix to change the rule matrix and alter the rules that constitute the management strategy. Another intriguing example is the Sugeno fuzzy vehicle, which can be trained to turn and park itself. Instead of a membership function, the effect of a rule is viewed as a linear equation of the process state variables. Through optimization of least-squares performance indices using a weighted linear regression system, the challenge is simplified to a parameter estimate. Although these approaches provide promising outcomes, they are subjective, somewhat heuristic and depend on trial and error for the choice of MFs. Thus, the ability to learn neural networks can offer a more promising approach to fuzzy logic systems [ 40 ]. As shown in Fig. 4 , the fuzzy logic system consists of four parts: knowledge base, fuzzification, inference and defuzzification. On the basis of the fuzzy constants provided, the process converts given inputs to the fuzzification stage. Based on the knowledge base, the inference is made. Then, in the defuzzification stage, every fuzzy output is mapped to complex output MFs [ 41 , 42 ].

Fuzzy logic system
2.4 Ant colony optimization
Ants exhibit a behaviour in which they remember and follow a specific path between their colony and a food source. They achieve this by leaving pheromone trails during their food search [ 43 ]. When other ants come across these pheromone trails, they start to follow them. Because the increased presence of the chemical on the path has the effect of attracting more ants to follow it, the ants will emphasize the pheromone trail. To find the best solution to the problem studied, ant colony optimization (ACO) builds multiple iterative solutions. The objectives of [ 44 ] were to evaluate the features of the search area for problems and to use this knowledge to address the solution process. The solution–construction process is a sequential decision-making process due to parametric stochastic decisions. An ACO algorithm depends on a sequence of learning of the parameters used in decision-making to reach a global policy that provides optimum solutions for a particular situation [ 45 ]. The parameters of the learning object are considered pheromones and are called variables of the pheromones.
An ACO method contains a stochastic local search technique to organize the routing pathways that artificial ants can determine. These ants co-operate together through indirect information exchange to create the best and shortest route. The concept of the ACO is taken from the food search characteristic of the true colony in an intelligent optimization algorithm and how the ants work together in this difficult job. It can be expected that the ACO finds the quickest route from nest to food according to the biological study of the ants. The ant pheromone distribution technique is termed staggered, in which information is shared with other ants indirectly. Pheromone updates are the basis of the ACO algorithm. These pheromone updates depend on the pheromone and the number of ants that work best. Natural ants can determine the quickest route based on their best knowledge and a strong pheromone trace. The shortest path is inversely proportional to the amount of pheromone and length of the path using an ACO method. The following is a step-by-step explanation of the algorithm replicating these properties [ 46 ]. The pseudocode for ACO is shown in Table 2 .
Pseudocode of the ant colony optimization algorithm
Steps . |
---|
Start: |
Set pheromone pathways and parameters in motion; |
Generate a random m ant (solution) population; |
Choose the optimal position according to the target function for every individual ant; |
Get the finest search ant; |
Restore the trail of the pheromone; |
Check if the end is true; |
End; |
Steps . |
---|
Start: |
Set pheromone pathways and parameters in motion; |
Generate a random m ant (solution) population; |
Choose the optimal position according to the target function for every individual ant; |
Get the finest search ant; |
Restore the trail of the pheromone; |
Check if the end is true; |
End; |
Set pheromone pathways: The algorithm starts by setting the initial pheromone pathways in the search space of the problem. These pathways act as a guide for ants to navigate and find solutions.
Generate a random ant population: Next, the algorithm generates a population of random solutions (ants) to start searching for the optimal solution.
Choose the optimal position: Each ant then uses a combination of pheromone information and heuristics to determine the next step (position) to take. The objective is to find the position that maximizes the target function.
Get the finest search ant: After all ants have completed their search, the algorithm selects the best ant, i.e. the one with the highest value of the target function.
Restore the trail of pheromone: The pheromone trail of the best ant is then updated to reinforce its path, encouraging other ants to follow it.
Check the end condition: The algorithm repeats the above steps until a stopping criterion is met.
End: The algorithm concludes when it satisfies the stopping condition and provides the best solution discovered.
2.5 Artificial bee colony optimization
The artificial bee colony (ABC) optimization imitates bee behaviour. A colony of bees is made up of onlookers, scouts and worker bees [ 47 ]. Artificial bees are flown in this system in a multidimensional search room and, depending on the experience they have gained and based on their next partner experience, the used bees pick their food sources and bees to change positions. Scout bees pick their food sources at random without any experience. Each food source avoids the probable solution to the problem under discussion [ 48 ]. The number of bees employed is as high as the food sources, each being a site, which is currently being used or as many solutions as individuals [ 49 ]. This procedure is continued until the ABC optimization meets a stop criterion.
ABC_Optimization (n, m, k)
population <- initialize (n, m, k)
global_best <- assign_random_food_source(population[m])
while! stop_criteria_met ()
for bee in population
fitness <- calculate_fitness (bee. food_source)
if fitness > global_best. fitness
global_best <- bee. food_source
for the bee in population
bee. update_food_source (global_best, bee. next_partner)
update_food_source (global_best, next_partner)
prob <- random_probability ()
if prob < experience
food_source <- global_best
else if prob < experience + next_partner. experience
food_source <- next_partner. food_source
food_source <- random_food_source ()
Initialization phase:
Initialize the x i j solution population in the j domain parameter. The exact description may be used for that purpose:
where x m a x j is the upper bound of the parameter j and x m i n j is the lower bound of the parameter j.
Worker bee phase:
Each worker bee uses a formula to identify and assess a food source v i j representative of a location such as a food source in her x i j memory. Each worker offers information about their food source to onlookers who select a food source website based on information collected from their bees while they wait at the hive according to Equation (2) :
If x k is a randomly picked solution, j is a parameter randomly selected and β i j is a random integer within the [–a, a] range. A greedy selection between v i and x i is applied after the production of a new solution v i .
Onlooker bee phase:
There is a reference previously to the proportion of the amount of a food source to its location in the solution. Onlookers are positioned at food sources using a selection strategy based on fitness, such as the way of selecting the roulettes wheel. New solutions x i based on pi are picked to assess the new solutions v i and new solutions v i for spectators are created. The hired bees between v i and x i receive a greedy selection.
Scout bee phase:
Former workers who lost their resources start scouting randomly for food supplies. Every colony of bees has scout bees. The scouts have little instruction when looking for food. They mostly focus on finding food. Artificial bees can find the available answers rapidly. ABC decides that the artificial scout is the bee whose food supply has been lost or whose profitability has fallen below a specific level of profitability. The control parameter that decides the class is the withdrawal criterion or the ‘limit’. After a predefined number of attempts, a worker bee leaves an unimproved solution that is a source of food. The number of tests necessary to release the answer is determined by ‘limits’.
2.6 Particle swarm optimization
Particle swarm optimization (PSO) is a population-based evolutionary computational technique that is employed to address stochastic troubleshooting. It belongs to the category of swarm intelligence and is founded on social and psychological principles. PSO provides valuable insights into engineering applications and contributes to their development [ 50 ]. Social impact and social learning make cognitive consistency possible for the person. People may resolve issues by talking to people and by changing their ideas, attitudes and behaviour; they can usually be portrayed as people moving in a socio-cognitive space towards one another. But PSO has certain inconveniences such as global convergence; unlike some other optimization algorithms, PSO does not have a guarantee of global convergence, which means that it may not find the true optimal solution. To address this drawback, a novel PSO and a chaotic PSO are designed to tackle energy-system optimization issues efficiently. The analysis of the problem of unit commitment within the regulated system leads to the examination of UCP (uniform customs and practice for documentary credits) inside the deregulated market. The overall profit, execution time and convergence criteria are compared between various approaches.
One element is the current velocity of the particle v ( t ) . Another is the optimum position Y ∗ ( t ) to approach the particle. The third factor is that the community or sub-community is optimally informed by Y ∗ ∗ ( t ) [ 51 ]. In each iteration step, the particle speed is modified to Y ∗ ( t ) and Y ∗ ∗ ( t ) . Meanwhile, the random weight is independently allocated to the V i , Y ∗ ( t ) and Y ∗ ∗ ( t ) . The speed and position are updated following Equations (3) and (4):
In the given equation, v k +1( i , j ) represents the velocity of the particle in the i -th particle and j -th dimension at iteration k + 1.
The weight factor ω determines the extent to which the previous velocity influences the new velocity.
v k ( i , j ) denotes the velocity of the particle in the i -th particle and the j -th dimension at iteration k .
C 1 and C 2 are the learning parameters that determine the influence of the personal best and global best solutions, respectively.
r and 1 and r and 2 are randomly generated numbers within the range of [0,1].
P bes t k ( i , j ) represents the personal best position of the i -th particle in the j -th dimension achieved thus far.
Y k ( I , j ) represents the current position of the i -th particle in the j -th dimension.
G b es t k signifies the global best position discovered by all particles up to the present iteration.
The flow chart for PSO is shown in Fig. 5 .

Flow chart of particle swarm optimization
2.7 Regression model
The research model [ 52 , 53 ] can be defined using Equation (5) :
where Y represents the dependent variable; this refers to the indication of respondent i ’s willingness to adopt smart home technology and their level of flexibility in terms of demand for technology j. β refers to the intercept. X 1 ij ,..., Xnij are dichotomous predictors included in the model. εij represents the random error term.
Building on Equation (5) , the level 2 model can be formulated as follows:
In Equations (5) and (6) , u 0 j ,..., u 1 j represent the random effects. W 1 j and W 2 j correspond to grand-mean centred and uncentred variables, respectively.
These equations are utilized in research to describe the relationships between the dependent variable, predictors and random effects. Equation (5) serves as the core model equation, capturing the influence of the predictors on the dependent variable while accounting for random error. Equations (5) and (6) extend the model by specifying the relationships and random effects associated with the intercept and predictor coefficients at the level 2 analysis. Collectively, these equations offer a comprehensive framework to analyse the variables that impact the acceptance of smart home technology and the adaptability of demand within the specific research context.
2.8 Regression and classification problems using AI
The RNN is a variation of the neural network frequently employed in the power systems domain to address regression and classification problems that involve sequential data. Unlike direct neural network models, the structure and operating principle of the RNN differ significantly [ 54 ]. In an RNN, the input data are fed to the model sequentially at each time step ( t ), as shown in the signal propagation diagram. At each step, the current state ( output ) is calculated by considering the current input data and the previously computed state. This iterative process continues for a fixed number of steps ( n ) until the desired output (predicted value) is achieved or until all input data ( input ) have been processed [ 55 ].
The propagation of signals in the RNN model is illustrated by the values assigned to each hidden state (hidden). These hidden states are calculated using the previous hidden state ( hidden − 1) and current input data ( input ) [ 56 ]. hiddent = (〈 w hidden , hiddent – 1〉 + 〈 w input , input 〉) Here, σ () represents the activation function (such as the sigmoid function, hyperbolic tangent or rectified linear unit (ReLU)), while w hidden and w input are the weights for the hidden and input states, respectively.
The output value at each calculation step output is obtained by taking the dot product of the weights associated with the output state and the values of the hidden state, similar to a regression equation: output = 〈w output , hiddent 〉 [ 57 ].
During training, the initial stage involves calculating the output signal, after which the error function is calculated to determine the discrepancy. For regression problems, it is common to utilize the square root of the standard deviation between the output of the RNN and the values from the response space ( y t ):
Applying the chain rule, the gradient of the error functional is calculated. The weight coefficients ( w ij ) are adjusted in a manner that reduces the functional, following the direction of decreasing values, until it reaches the minimum value or the training iterations reach the predetermined limit. It is important to note that the weights associated with the hidden state of the RNN ( w hidden ) remain unchanged after propagating the error from each output ( output ). Conversely, the coefficients w output and w input change at each step of the gradient [ 58 ].
3.1 Operation of the power system
ELD is the process of assigning the generation output to the generation unit to supply the system load fully and economically. The whole engaged generating unit produces total electricity costs to minimize energy. The main concern of the ELD problem is to reduce the overall fuel cost. This is achieved by generating electricity in a way that optimizes the use of resources and reduces the overall cost of power production in the electricity system. Multiple generators provide enough total output to meet the consumer requirements in a typical power system. The production costs of each generating unit in the electricity system are different, as the producing units are not the same distance from the loading unit. Over the years, several AI approaches have been created to address this challenge. After simulation, authors concluded that using the genetic algorithm technique to solve the ELD problem can result in a lower overall cost of electricity production, but may also result in higher emissions. However, choosing a solution with a higher cost may result in minimum emissions. Transmission losses are usually neglected when they are small. However, for long-distance transmission in large and interconnected networks, transmission losses become significant and have an impact on the optimal distribution of power generation. It is possible to operate the same multitasking system with a better voltage profile and with evolutionary calculation technology, the cost and emission value of the best compromise.
The proposed results of the simulation of the ANN emphasize that the results are indiscernible from conventional methods, although the time used by neural networks is less than conventional methods. The number of generators increases the prediction error because there is a lot of input and output data to be learned. To monitor the performance, neural networks have been modelled. The authors have performed the simulations with many generation units having ramp rate limits and prohibited operating zones as constraints and the resultant performance is compared with ANN, GA and ACO techniques, but the ABC technique gives better outputs with fast convergence. The greedy selection procedure and the timely abandonment of the used food sources contained in ABC give it this potential. The basic operations of ABC optimization prevent solutions from stopping and make the algorithm more exploitative.
3.1.2 Generator maintenance scheduling
Generator maintenance scheduling (GMS) is a complicated combinatorial optimization issue for a power provider. Mathematical approaches include traditional ways to tackle the GMS issue. To evaluate the needed objective function, a mathematical model approach employs a trial-and-error procedure. Mathematical approaches even fail to provide viable answers as in some circumstances the operator needs to rely on certain assumptions and models that may not accurately reflect real-world conditions. In some cases, operators may need to be involved to provide additional input and expertise to make informed decisions about maintenance schedules. In addition, there may be unpredictable factors, such as equipment failures or changes in demand, that cannot be accurately accounted for by mathematical models alone. Maintenance is a preventive outage program for generating units within a certain time horizon in a power system. In the event of a range of various specification generating units in the energy system and several limitations to produce a sustainable and practical solution, maintenance planning becomes a difficult challenge. The maintenance planning of the generators is done for time horizons of different lengths. Short-term maintenance plans from 1 hour to 1 day are crucial to the daily operations, engagement and operational planning of power plants. Medium-term planning is necessary for resource management between 1 day and 1 year. Long-term planning from 1 to 2 years is crucial for future planning. An examination is being conducted to resolve some AI methods, including simulated developments, neural networks and GAs. The application of the genetic algorithm through case research shows that suitable GA parameters are safeguarded, as well as issue coding and development functions. The use of integer encoding decreases the velocity of the genetic search method investigation. By using integer encoding, the algorithm needs to perform additional operations to convert solutions into integers, which can slow down the search process. Planning the generation of power remains a barrier to competent solution technology and a difficult optimization problem. The challenge in power generation planning lies in finding the optimal balance between cost and efficiency, while also considering factors such as environmental impact, reliability and security of supply. The answer to the difficulties in generation planning consists of finding the UC (unit commitment) at every point in the programming period for each generator in one power system. An electrical system must be defined in each planning interval for each power generator for the decisions and levels of output. The solution process must be addressed concurrently for binary decisions and continuous variables. Generation difficulties with scheduling are typically quite narrow and combined. Match swarm optimization approaches have been used to achieve viable schedules within a specified time. The study found that an optimization-based approach using PSO provided better results than a GA or an evolutionary strategy. Data from the actual power system were used to evaluate the performance of the different optimization techniques.
UC is properly scheduled for the ON/OFF status and the genuine generator power outputs of the system. To satisfy a high number of system limitations and decrease the overall fuel cost at every time interval, a spinning reserve is necessary (spinning reserve refers to the additional generation capacity that is available and running but not actively supplying power to the grid). UC meets the expected load requirements in advance. To implement UCs, medium-term load forecasting using ANN was used. The neural network structure was trained through learning and parameter learning. Total operational expenses under 24 hours were used for the assessment criterion. The study demonstrates the effectiveness of the proposed approach by comparing the performance of the ANN-based load forecasting model with traditional methods such as linear regression and time-series forecasting. The results show that the ANN-based load forecasting model significantly improves the accuracy of load forecasting and reduces the scheduling cost by reducing the number of units needed for scheduling. The study also highlights the importance of considering the uncertainty and variability of load demand in UC scheduling and suggests that ANN-based load forecasting models can be a useful tool for achieving more efficient and reliable scheduling in power systems. Locational marginal prices have been evaluated through a trained ANN. The findings show that the current technique gives a different UC mechanism. To develop unit commitment, the PSO technique is used. On implementation, with the increasing size, the execution time is also increasing. To accelerate the PSO, a convergence repair method is also implemented.
3.1.4 Optimal power flow
Optimal power flow (OPF) is a highly important technique to identify the optimum control parameter settings that enhance or decrease the intended target function, but also under a variety of limitations. An essential instrument to design and operate a power system is the issue of optimum power flow to identify the best parameter settings that can maximize or minimize the intended goal function within specific limitations. Voltage and reactive controls, also called OPD, are an OPF sub-problem that seeks to reduce overall transmission loss by resuming the reactive power flow. Optimal reactive power dispatch is a non-linear solution for the issue of blending integers since some control variables such as tap ratios for transformers, shunt capacitor outputs and reactors are distinct.
The alternate strategy for mitigating the problem of GA-ANN is set out in this article. A collection of ANN networks is trained offline in specified system quantities to work on a general OPF issue. To choose the appropriate ANN inputs, the k-mean clustering technique is utilized. When learning the functions correctly, ANNs can easily estimate the associated results with great precision.
The ANFIS develops the input/output data set fuzzy-inference system (FIS) that matches the membership (adjustment) parameters with a backpropagation or minimum square process type. This update allows you to learn from the fuzzy systems data IEEE 39 bus system implementations and simulated software from the power world are utilized for the formation of the ANFIS. The results indicate that the ANFIS offers solutions as accurate as conventional ones. It takes less time, though, and it works quickly. Some additional papers on the application of AI in the operation of power systems are presented in Table 3 .
Applications of artificial intelligence in the operation of a power system
Applications . | Reference . | Year . | Objective . | Technique(s) . |
---|---|---|---|---|
Economic load dispatch | Alquthami [ ] | 2020 | Get the best results for the lowest amount of money and in the shortest amount of time compared with previous techniques | Artificial bee colony optimization |
Sahay [ ] | 2018 | To reduce fuel costs, transmission costs, labour costs and maintenance costs | Genetic algorithm | |
Mishra [ ] | 2015 | Better compromised solutions, i.e. cost and emissions, between the two objectives | Genetic algorithm | |
Dixit [ ] | 2011 | The problem with a single equivalent objective function to address economic, emission and combination economic and emission dispatch problems | Artificial bee colony optimization | |
Daniel [ ] | 2018 | The period in which the load shipment is computed varies dynamically for each selected time interval | Artificial neural network | |
Ruiz-Abellón [ ] | 2019 | The objective is to minimize ELD losses using a GA-based optimum power flow system | Particle swarm optimization | |
Ali [ ] | 2020 | To start with, ELD is solved by using a genetic algorithm with prevailing constraints, but no line flow constraint | Genetic algorithm | |
Generator maintenance scheduling | Fu [ ] | 2020 | Performing preventive maintenance on components that are at risk helps distribution networks avoid failures by preventing them before they occur | FACTS devices |
Esmaili [ ] | 2014 | A multi-target framework for congestion management is presented in which three competing target functions are simultaneously optimized, total operating expenses, voltage and margins for transient stability | FACTS devices | |
Suresh [ ] | 2013 | For a power system, minimizing annual supply reserve ratio deviation and reducing loss of load probability | Particle swarm optimization | |
Lakshminarayanan [ ] | 2018 | The aims are to maximize and distribute reserved electricity equally over 52 weeks while fulfilling the numerous limitations | Genetic algorithm | |
Scalabrini Sampaio [ ] | 2019 | Data collection for the training and testing of an artificial neural network to anticipate and identify defects in future machinery | Artificial neural network | |
Power flow | Fikri [ ] | 2019 | Implementation of ANN in the absence of some problem data and, more importantly, in the absence of convergence of numerical methods with high precision | Artificial neural network |
Rahul [ ] | 2012 | The objective is to minimize transmission losses using a GA-based optimum power flow system for the IEEE 30-bus test power system | Genetic algorithm | |
Nakawiro [ ] | 2009 | Offline neural artificial networks replace the power flow in the OPF, which is a non-linear mixture of integral optimization and a network reduction goal | Genetic algorithm and artificial neural network | |
Sumpavakup [ ] | 2010 | This method has been used to identify the optimum solution for each producing unit and reduce the overall production cost | Artificial bee colony optimization | |
Abdellah [ ] | 2015 | To be optimum, the standard power flow program must be increased (OPF) | Adaptive neuro-fuzzy interference system | |
Unit commitment | Nemati [ ] | 2018 | Modern power systems such as microgrids must face a variety of strict hurdles due to the present energy revolution | Genetic algorithm and mixed-integer linear programming |
Alshareef [ ] | 2011 | In this study, the cost, emission and both cost and emission of the system are all minimized | Particle swarm optimization | |
Arora [ ] | 2016 | The use of neural network learning results on medium-term load forecasting is presented as a method for unit commitment | Artificial neural network | |
Liu [ ] | 2008 | In this research, a hybrid ANN technique is given to tackle combinational optimization issues in power systems, including unit commitment | Lagrangian relaxation and artificial neural network | |
Kumar [ ] | 2010 | To begin with, unit commitment is solved by using a genetic algorithm with prevailing constraints but no line flow constraint. In the second phase, using GA-based OPF, the number of violations in the lines is minimized for a committed schedule | Genetic algorithm |
Applications . | Reference . | Year . | Objective . | Technique(s) . |
---|---|---|---|---|
Economic load dispatch | Alquthami [ ] | 2020 | Get the best results for the lowest amount of money and in the shortest amount of time compared with previous techniques | Artificial bee colony optimization |
Sahay [ ] | 2018 | To reduce fuel costs, transmission costs, labour costs and maintenance costs | Genetic algorithm | |
Mishra [ ] | 2015 | Better compromised solutions, i.e. cost and emissions, between the two objectives | Genetic algorithm | |
Dixit [ ] | 2011 | The problem with a single equivalent objective function to address economic, emission and combination economic and emission dispatch problems | Artificial bee colony optimization | |
Daniel [ ] | 2018 | The period in which the load shipment is computed varies dynamically for each selected time interval | Artificial neural network | |
Ruiz-Abellón [ ] | 2019 | The objective is to minimize ELD losses using a GA-based optimum power flow system | Particle swarm optimization | |
Ali [ ] | 2020 | To start with, ELD is solved by using a genetic algorithm with prevailing constraints, but no line flow constraint | Genetic algorithm | |
Generator maintenance scheduling | Fu [ ] | 2020 | Performing preventive maintenance on components that are at risk helps distribution networks avoid failures by preventing them before they occur | FACTS devices |
Esmaili [ ] | 2014 | A multi-target framework for congestion management is presented in which three competing target functions are simultaneously optimized, total operating expenses, voltage and margins for transient stability | FACTS devices | |
Suresh [ ] | 2013 | For a power system, minimizing annual supply reserve ratio deviation and reducing loss of load probability | Particle swarm optimization | |
Lakshminarayanan [ ] | 2018 | The aims are to maximize and distribute reserved electricity equally over 52 weeks while fulfilling the numerous limitations | Genetic algorithm | |
Scalabrini Sampaio [ ] | 2019 | Data collection for the training and testing of an artificial neural network to anticipate and identify defects in future machinery | Artificial neural network | |
Power flow | Fikri [ ] | 2019 | Implementation of ANN in the absence of some problem data and, more importantly, in the absence of convergence of numerical methods with high precision | Artificial neural network |
Rahul [ ] | 2012 | The objective is to minimize transmission losses using a GA-based optimum power flow system for the IEEE 30-bus test power system | Genetic algorithm | |
Nakawiro [ ] | 2009 | Offline neural artificial networks replace the power flow in the OPF, which is a non-linear mixture of integral optimization and a network reduction goal | Genetic algorithm and artificial neural network | |
Sumpavakup [ ] | 2010 | This method has been used to identify the optimum solution for each producing unit and reduce the overall production cost | Artificial bee colony optimization | |
Abdellah [ ] | 2015 | To be optimum, the standard power flow program must be increased (OPF) | Adaptive neuro-fuzzy interference system | |
Unit commitment | Nemati [ ] | 2018 | Modern power systems such as microgrids must face a variety of strict hurdles due to the present energy revolution | Genetic algorithm and mixed-integer linear programming |
Alshareef [ ] | 2011 | In this study, the cost, emission and both cost and emission of the system are all minimized | Particle swarm optimization | |
Arora [ ] | 2016 | The use of neural network learning results on medium-term load forecasting is presented as a method for unit commitment | Artificial neural network | |
Liu [ ] | 2008 | In this research, a hybrid ANN technique is given to tackle combinational optimization issues in power systems, including unit commitment | Lagrangian relaxation and artificial neural network | |
Kumar [ ] | 2010 | To begin with, unit commitment is solved by using a genetic algorithm with prevailing constraints but no line flow constraint. In the second phase, using GA-based OPF, the number of violations in the lines is minimized for a committed schedule | Genetic algorithm |
3.2 Control of the power system
The main objective of a voltage controller power system is to maintain the voltage profile within a defined limit, thus minimizing transmission losses and avoiding cases of voltage instability [ 81 ]. The VC system consists of three levels of hierarchical control: AVR (automatic voltage regulator), tertiary voltage control (TerVC) and secondary voltage control (SecVC). AVR is aimed at controlling the voltage of buses that are equipped with reactive power sources (e.g. synchronous, sync, static var compensators and STATCOM (static synchronous compensator)). Actions are carried out locally at this control level. SecVC is used to monitor the voltage on a specific bus that controls a cargo bus.
In situations in which there is hardware present in the vicinity that modifies the reference point for the AVR, the control level typically operates at a slower pace compared with the AVR control level. SecVC is responsible for identifying VC regions and their correlation with individual load buses. To accommodate varying power system conditions, SecVC must demonstrate flexibility in adjusting the control regions to accommodate all grid conditions. On the other hand, TerVC determines the optimal reference value for voltage grids at each load bus. The objective is to minimize power loss, optimize reactive power and maintain a minimum load release or reservation. TerVC is usually updated every 30 minutes to 1 hour.
The backward error propagation algorithm trains the multilayered feedforward perception. The minimum singular value method analyses the static voltage collapse. The procedure uses a minimum voltage stability evaluation time once the network training is complete. For monitoring voltage collapse, complementary methodologies of neural networks and expert systems would be combined for use in the application [ 82 ].
GA is an iterative optimization technique with several solutions from the candidates (known as a population). In the case in which there is no knowledge of the problem field, then the GA starts to look for solutions from a random population. The appropriate coding (or display) must first be defined to solve the problem. A fitness function should also be defined so that every coding solution is given a figure of merit. If parents are not satisfied with the termination condition, for reproduction, they must be picked [ 83 ]. They are then joined to generate offspring through reproduction and, to refresh the population of candidate solutions, crossover and mutation operators are utilized. Typically, in a basic genetic algorithm, three operators are involved: selection, crossover and mutation. These operations are performed to generate new offspring, individuals and subsequent generations. The same process is repeated with the new generation until the desired criteria are met. The approach of this method is used to teach swarming at the beginning of PSO. In this case, 10 control variables are used for the ANN input. The neuron and its prejudice are 11. A hidden layer consists of this group of neurons and biases. Ten outputs/goals are available. These objectives are achieved by using the optimal value of PSO. The last outputs are the initializations in the time-varying non-linear particle swarm optimization (TVNL-PSO). The steps are as follows:
PSO is used to take the ANN input; the weight value is applied at random;
the ANN input and partition in a cached layer are weighed and then activated by the sigmoid binary function;
weighting of the output in the hidden layer and activation of the linear function;
to optimize the reactive power and VC by TVNL-PSO, the ANN output is transmitted as a starting initialization value.
3.2.2 Power system stability control
The stability of a power system is a feature that allows it to remain under a balance in normal operating conditions and retrieve an acceptable balance after a change. Margins of stability can be seen to decline throughout the world [ 84 ]. We highlight three of the many reasons for this:
The inhibition by economic and environmental constraints of further transmission or construction. Therefore, power systems must be operated with lower safety margins.
Restructuring of the electricity industry. The restructuring process reduces the margins of stability, as power systems do not co-operate effectively [ 85 ].
Increased complexity of power systems multiplies the compulsive properties. These include large, non-linear oscillations; frequency differences between weakly binding energy-system areas; interactions with saturated devices.
Fuzzy logic endeavours to address problems by emulating human reasoning, allowing optimal decision-making based on available information. It can also be employed to regulate the stability of un-modelled systems. To achieve improved performance, a fuzzy logic (FL) controller is combined with a PID (proportional–integral–derivative) controller. In this particular scenario, the fuzzy logic control adjusts the gains of the PID controller based on the power system.
A fuzzy logic controller primarily consists of four major parts: fuzzification, fuzzy rule base, fuzzy inference and defuzzification. FACTS have proven to be extremely promising for increasing performance under stable conditions. The most promising FACTS device is a unified power flow controller (UPFC). Three control factors can be adjusted: bus voltage, reaction line and phase angle between two buses. The power flow should be redistributed across lines while a stable state is preserved. It can also be utilized to increase damping when low frequencies are damped temporarily.
Power system stability control.
Load frequency control as defined by the controllable generator power output control in a prescribed area resolves system frequency changes, two-line loadings or interactions to maintain an interchange with other regions within the fixed limit or scheduled system frequency [ 86 ]. The traditional proportional–integral (PI) controller is the most widely used among different types of load frequency controllers. The PI controller can be easily implemented and provides a faster response, but its performance decreases when unwanted disturbances, such as load change dynamics, increase the difficulty within the system. In this paper, less computing is required for the non-linear autoregressive-moving average-L2 (NARMA-L2) control architecture. Plant output, reference and control signals are included. The controller is therefore taught to monitor the output of the reference model. The model network that updates controller settings predicts the effect of the change in plant performance. Some additional papers on the application of AI in the control of power systems are presented in Table 4 .
Applications of AI in control of a power system
Applications . | Reference . | Year . | Objective . | Technique(s) . |
---|---|---|---|---|
Voltage control | Kothakotla [ ] | 2021 | Integrated–proportional–derivative controller designed to control the isolated microgrid grid voltage | Genetic algorithm |
Wang [ ] | 2020 | A multi-agent grid control system driven by data using an ANN method | Artificial neural network | |
Zidani [ ] | 2018 | The voltage and frequency of an automated induction generator are being manipulated using a novel technique | Artificial neural network | |
Sumathi [ ] | 2015 | The backpropagation feeder for an artificial neural network has been designed to estimate the UPFC output variables for different loading conditions in a 24-bus Indian extra-high-voltage power system | Backpropagation feedforward artificial neural network | |
Kanata [ ] | 2018 | Improving the power system quality to measure the precise control variable value. Improved power system quality | Particle swarm optimization and hybrid artificial neural network | |
Abdalla [ ] | 2016 | Avoiding voltage violations in contingencies of power systems by adjusting coordinated PID controller parameters | Genetic algorithm | |
Chung [ ] | 2008 | This study provides control systems for coordinating numerous microgrid generators for grid-connected and autonomous modding, utilizing interfaces of inverter type | Particle swarm optimization | |
Power system stability control | Yousuf [ ] | 2021 | The electricity system automation ensures restoration, error diagnostic, management and network security | Fuzzy logic, genetic algorithm |
Aakula [ ] | 2020 | This article uses optimization, a heuristic-based swarm intelligence method, to obtain enough reactive energy to improve bus voltages | Particle swarm optimization | |
Karthikeyan [ ] | 2017 | In this paper, fuzzy-PID-based STATCOM is proposed to increase the stability of the energy system under failure conditions | Fuzzy logic | |
Sallama [ ] | 2014 | Here, stability is received in the shortest amount of time and with the least amount of disruption | Neuro-fuzzy system and particle swarm optimization | |
Chen [ ] | 2018 | To enhance the current communication network to meet low latency and high economic requirements, a perfect planning method is presented | Genetic algorithm | |
Torkzadeh [ ] | 2014 | The genetic algorithm, the GA-FLC (optimized fuzzy logic controller), is used to damp down low-frequency oscillations | Genetic algorithm and fuzzy logic | |
Dutta [ ] | 2017 | A common solution required for the power stabilizer to compress low-frequency oscillation (PSS) | Ant colony optimization | |
Nam [ ] | 2018 | A comparison of different existing simulation models showed that, compared with existing optimization models, the projected method showed superior results for power system stability control | Kringing models | |
Miraftabzadeh [ ] | 2021 | Advance machine learning can make work much easier in power system stability than conventional methods | Artificial neural network, genetic algorithm | |
Load frequency control | Safari [ ] | 2021 | A microgrid (MG) is proposed for load frequency control (LFC) on the island, just like the model eves in this work contributes to the LFC system | Particle swarm optimization-based artificial neural network |
Joshi [ ] | 2020 | For the first time, a novel control plan for the LFC of a hydro–hydro vitality framework has been developed based on joint efforts of the fuzzy logic control and PSO algorithm-built design of PID, resulting in an FLPSO-PID | Fuzzy logic with particle swarm optimization | |
Nguyen [ ] | 2018 | The suggested constrained particle swarm optimization technique compares ACO with an assessment of its efficiency in the thermal interconnection system | Ant colony optimization | |
Balamurugan [ ] | 2018 | Its primary goal is to balance the generation and demand of a power system | Fuzzy logic | |
Otani [ ] | 2017 | The control of a recurrent neural network is proposed for efficient use of the introduced storage battery | Artificial neural networks | |
Kuma [ ] | 2020 | The planned solar and wind power is being utilized to analyse load frequencies, mitigate frequency changes, guarantee stability in the GM power system, to respond to the unexpected surge in demand for charging power and PI controllers by non-renewable sources | Recurrent neural network | |
Arora [ ] | 2020 | A comparison of many existing simulation models showed that, compared with existing optimization models, the projected method showed superior results for smart grid control of frequency problems | Genetic algorithm, particle swarm optimization |
Applications . | Reference . | Year . | Objective . | Technique(s) . |
---|---|---|---|---|
Voltage control | Kothakotla [ ] | 2021 | Integrated–proportional–derivative controller designed to control the isolated microgrid grid voltage | Genetic algorithm |
Wang [ ] | 2020 | A multi-agent grid control system driven by data using an ANN method | Artificial neural network | |
Zidani [ ] | 2018 | The voltage and frequency of an automated induction generator are being manipulated using a novel technique | Artificial neural network | |
Sumathi [ ] | 2015 | The backpropagation feeder for an artificial neural network has been designed to estimate the UPFC output variables for different loading conditions in a 24-bus Indian extra-high-voltage power system | Backpropagation feedforward artificial neural network | |
Kanata [ ] | 2018 | Improving the power system quality to measure the precise control variable value. Improved power system quality | Particle swarm optimization and hybrid artificial neural network | |
Abdalla [ ] | 2016 | Avoiding voltage violations in contingencies of power systems by adjusting coordinated PID controller parameters | Genetic algorithm | |
Chung [ ] | 2008 | This study provides control systems for coordinating numerous microgrid generators for grid-connected and autonomous modding, utilizing interfaces of inverter type | Particle swarm optimization | |
Power system stability control | Yousuf [ ] | 2021 | The electricity system automation ensures restoration, error diagnostic, management and network security | Fuzzy logic, genetic algorithm |
Aakula [ ] | 2020 | This article uses optimization, a heuristic-based swarm intelligence method, to obtain enough reactive energy to improve bus voltages | Particle swarm optimization | |
Karthikeyan [ ] | 2017 | In this paper, fuzzy-PID-based STATCOM is proposed to increase the stability of the energy system under failure conditions | Fuzzy logic | |
Sallama [ ] | 2014 | Here, stability is received in the shortest amount of time and with the least amount of disruption | Neuro-fuzzy system and particle swarm optimization | |
Chen [ ] | 2018 | To enhance the current communication network to meet low latency and high economic requirements, a perfect planning method is presented | Genetic algorithm | |
Torkzadeh [ ] | 2014 | The genetic algorithm, the GA-FLC (optimized fuzzy logic controller), is used to damp down low-frequency oscillations | Genetic algorithm and fuzzy logic | |
Dutta [ ] | 2017 | A common solution required for the power stabilizer to compress low-frequency oscillation (PSS) | Ant colony optimization | |
Nam [ ] | 2018 | A comparison of different existing simulation models showed that, compared with existing optimization models, the projected method showed superior results for power system stability control | Kringing models | |
Miraftabzadeh [ ] | 2021 | Advance machine learning can make work much easier in power system stability than conventional methods | Artificial neural network, genetic algorithm | |
Load frequency control | Safari [ ] | 2021 | A microgrid (MG) is proposed for load frequency control (LFC) on the island, just like the model eves in this work contributes to the LFC system | Particle swarm optimization-based artificial neural network |
Joshi [ ] | 2020 | For the first time, a novel control plan for the LFC of a hydro–hydro vitality framework has been developed based on joint efforts of the fuzzy logic control and PSO algorithm-built design of PID, resulting in an FLPSO-PID | Fuzzy logic with particle swarm optimization | |
Nguyen [ ] | 2018 | The suggested constrained particle swarm optimization technique compares ACO with an assessment of its efficiency in the thermal interconnection system | Ant colony optimization | |
Balamurugan [ ] | 2018 | Its primary goal is to balance the generation and demand of a power system | Fuzzy logic | |
Otani [ ] | 2017 | The control of a recurrent neural network is proposed for efficient use of the introduced storage battery | Artificial neural networks | |
Kuma [ ] | 2020 | The planned solar and wind power is being utilized to analyse load frequencies, mitigate frequency changes, guarantee stability in the GM power system, to respond to the unexpected surge in demand for charging power and PI controllers by non-renewable sources | Recurrent neural network | |
Arora [ ] | 2020 | A comparison of many existing simulation models showed that, compared with existing optimization models, the projected method showed superior results for smart grid control of frequency problems | Genetic algorithm, particle swarm optimization |
3.3 Planning of power system
DSP plays a crucial role in enhancing reliability and minimizing costs for both utilities and consumers. Electric power distribution networks are a fundamental component of the electrical power system. In general, transport networks are denser and more complex than those that provide transformer stations [ 110 ]. Automating previously manual jobs increases with distribution networks becoming more complex. New tools are known as advanced automation functions that support the operation of such networks. These functions enable the network operator to effectively address issues that arise. Furthermore, the reconfiguration of distribution networks is essential to identifying optimal solutions that align with the operator’s requirements and constraints, ensuring a secure and economically optimized electricity supply.
The optimal design of a distribution network is not a fixed solution, but rather a process that involves considering various technically feasible options and using improvement tools to make the best decision based on factors such as demand, reliability of power transmission and network structure. All potential paths are initially identified with uploaded system data and then the energy-loss cost calculation applies for each identified path forward/backward sweeping load flow technique. For the distribution of power, the minimum energy-loss path is chosen. The optimal selection of the branch conductor of the radial system is done using optimization of PSO. In this case, parameters such as power loss, voltage profile and capital investment depreciation improve optimization. These parameters are used as optimization criteria to determine the optimal branch conductor that minimizes power loss, improves the voltage profile and reduces capital investment depreciation. The PSO algorithm iteratively updates the position of each particle in the search space based on its own experience and the experiences of its neighbours. The algorithm continues until a global optimum is found or a stopping criterion is met. The final solution produced by the PSO algorithm represents the optimal branch conductor that meets the optimization criteria [ 111 ]. The optimization of the PSO results in the optimal conductor and the best substation, the positioning of the optimal conductor is selected and then the optimal substation power distribution is achieved.
A multi-target algorithm was proposed that uses a fluid optimization technique to handle contradictory targets [ 112 ]. The plan formulation and the algorithm include a multi-target function that uses battery energy storage systems (BESSs) and traditional resources to select the best planning option. The microgrid BESS has been receptive to power management and improvement in power quality. The proposed algorithm is based on the fuse-based decision-making processes of the Mamdani-type FIS and Bellman–Zadeh approach.
In this paper [ 113 ], two algorithms, namely the mixed-integer linear program (MILP) and GA, are compared for the design of a radial distribution system feeder. The main objective is to minimize total investment and operational outages while maximizing system reliability. The study aims to evaluate and compare these two optimization techniques in terms of their optimality, complexity and time requirements. A unique aspect of the optimization model is the consideration of operational costs associated with failures, which are directly linked to the design of the system. The fault rate and defect cost at each loading point are updated based on the proposed configurations. It is crucial to determine which method produces superior results in terms of optimality, complexity and time efficiency.
The GA technique is used to build the algorithm for optimizing distribution networks. The fundamental concept is the growth of the genetic operator population (selection, crossover and mutation). These are used to generate a fresh population from the previous generation throughout each generation procedure. In GA, a single chromosome shows each person. This chromosome corresponds, according to the graph theory, to a radial distribution network configuration or a particular graph twist. The chromosome group is the population. Randomly, an initial group is created as a first step in the implementation of GA. Then the encoding is applied to each chromosome. In this study, only closed branches represent the network topology. A true coding strategy was used to match each gene to the edge of the coagulation tree [ 114 ].
3.3.2 Reactive power optimization
As the demand of electricity increases and new lines are built, the environment and the unforeseen power fluid in the lens are reduced, it is generated by the current scenario. Effect reactive compensation control improves voltage, reduces energy loss and improves system performance under stable and dynamic conditions in weak nodes [ 115 ]. Because the complexity of power systems is constantly increasing and the network components are constantly being loaded, abnormal operating conditions such as voltage can occur more often. Therefore, it is obvious that the power system needs adequate reactive power and VC.
In pursuit of intelligent theory development, a combination of fuzzy logic and ANNs is used to determine the control strategy for transformer taps and capacitors. However, due to the increasing complexity of control variables, rapid optimization becomes challenging [ 116 ]. To address this issue, a genetic algorithm is utilized, which tackles problems associated with incorporating regulatory time as penalty terms in the objective function and determining appropriate penalty factors that affect algorithm performance. When regulatory time is a constraint, the optimization objective focuses on minimizing the total energy loss during the dispatch period. GAs, inspired by natural selection mechanics and genetics, such as inheritance, mutation and recombination, are utilized (also referred to as crossover) to optimize the solution.
The PSO method can be used for handling FACTS devices in power systems. Various reactive power problem objectives and different solutions are addressed in the interconnected power system. Solutions and comparative analyses using the FACTS device, differential evolution (DE) and PSO algorithms are presented under various loading conditions [ 117 ]. The algorithm proposed [ 118 ] employs DE to minimize generator fuel costs on FACTS devices. Additionally, the authors discuss the hybridization of DE and PSO (DEPSO) to overcome the maximum load limit. The control of reactive flow is addressed using fuzzy sets and a fuzzy feature optimization technique is introduced to optimize reactive power. The utilization of fuzzy linear programming offers an effective approach to calculating reactive power, aiming to minimize active power loss and maximize the voltage stability margin. The paper [ 119 ] explores the combination of fuzzy and GA approaches for FACTS shunt controller placement and sizing. Lastly, the focus of [ 120 ] is on the integration of fuzzy systems with GM algorithms and the PSO algorithm to tackle the OPF problem and optimize control variables. In this paper, the authors [ 121 ] focus on fluid-based reactive and voltage controls to reduce actual loss of power.
3.3.3 Capacitor placement
There are some advantages if capacitors are placed optimally, including various factors, such as maximizing energy and reducing peak power loss through the introduction of a condenser in an electrical distribution system. In the paper, a novel adaptive modified firefly algorithm is presented to address the optimal capacitor placement problem in power systems. This optimization problem involves identifying the best positions and sizes of capacitors in a power system, in order to enhance voltage stability, minimize energy losses and improve the power factor. The proposed algorithm combines the firefly algorithm with adaptive parameter settings and introduces a unique crossover operator to enhance both convergence speed and solution quality. The authors conduct evaluations on a test system and compare the performance of the algorithm with other optimization methods. The results demonstrate the effectiveness of the proposed approach in finding optimal solutions and highlight its superiority in terms of solution quality and computational efficiency compared with other algorithms [ 122 ]. Losses occur due to reactive currents in the distribution system and are therefore minimized in the right places. Shunt capacitors are used, depending on their use. A capacitor is used to improve the voltage profile, reduce losses and increase the power factor [ 123 ].
Elbaz et al. [ 124 ] have been using ANN techniques to control both capacitor banks and voltage regulators. The ANN has many input connections and all inputs are combined to determine the output capacity. The purpose of the capacitor search algorithm was to reduce total active losses in the distribution system by utilizing the capacitor banking search to address the capacitor placement problem. The operation of the ant colony was proposed to address problems related to the installation of the capacitor. The fuzzy method uses variables such as angle, current and voltage, etc. A degree for a set and fuzzy variable is determined by MFs. This degree changes from zero to one that takes zero or one as opposed to the classical methods [ 125 ]. A fuzzy logic-based algorithm is developed to minimize line loss for the placement of condensers in a radial system. The fuzzy expert system identifies the capacitor candidate nodes by compromising the possible reduction in loss between the condenser system and the voltage level. This paper [ 126 ] presents a fluffy approach to identifying the appropriate places for capacitor placement. In the design of a seamless logic to determine the optimal placement of capacitors, two main objectives are taken into account. These objectives include (i) minimizing actual power loss and (ii) maintaining voltage within acceptable limits. Fuzzy member functions are used to model voltage and power loss indices for nodes in the transmission system [ 127 ]. The suitability of capacitor placement for each node in the distribution system is then determined. This is achieved using a set of rules within the FIS. Nodes can be fitted with capacitors of greatest appropriateness.
In [ 128 ], a method based on GAs is used to identify the optimal locations and sizes of capacitors in the distribution network. The capacitor sizes are considered discrete and known variables placed on the buses of the network. Hence, the maximum losses of the distribution system are reduced. GA technology is selected as the capacitor problem is more accurately addressed in the power grid. When the search area crosses for an optimal solution, the advantages of GA are multifunctional—that is, when a locally optimal solution is found to an engineering goal, GA adapts its search to find an optimal global solution, subject to predefined search restrictions [ 129 , 130 ]. The article shows the results of the study of the best size and location for a GA-connected system using bays in Saudi Arabia in West–East regions [ 131 , 132 ]. Two formulas are proposed for capacitor positioning: (i) cost balance condenser/loss principle and (ii) total system performance cost estimates, standard analysis and verification of annual benefits, power loss and operational tension results [ 133 , 134 ]. AI is applicable for various aspects of the power system [ 135 ]. Some additional papers on power system application of AI in planning are presented in Table 5 .
Applications of AI in the planning of a power system
Reference | Year | Objective | Technique(s) | |
Distribution system planning | Kahouli [ ] | 2021 | An ideal approach to increasing the safety of a distribution system and decrease power loss by optimizing the network reconfiguration problem | Genetic algorithm and particle swarm optimization |
Žarković [ ] | 2019 | Although the primary goal of a DSP is to minimize the total cost of ownership, it also aims to maximize system reliability | Mixed-integer linear programming and genetic algorithm | |
Ahmetovic [ ] | 2021 | It is proposed that the Bellman–Zadeh decision-making process shall use the proposed fuzzy-inference system type Mamdani to assess the Powerline feeder reliability as a planning criterion | Fuzzy logic | |
Suresh [ ] | 2017 | These studies are crucial to establishing the status of each node or bus and conditions in the distribution system and these artificial neural networks are efficient at describing the relationship between the raw data and these neural networks | Artificial neural network | |
Kumari [ ] | 2018 | This article offers the optimal energy distribution system for routes and optimal drives with the lowest energy-loss costs | Particle swarm optimization | |
Saha [ ] | 2021 | Solving the optimal problem of diesel generator allocation | Genetic algorithm | |
Hosseini [ ] | 2021 | Cyber-physical detection, stochastic and cyber security enhancement to detect and estimate damages | Artificial neural network | |
Lytras [ ] | 2019 | There are different methods suggested to optimize distribution system planning | Particle swarm optimization | |
Gandhar [ ] | 2020 | Using a proportional–integral (PI) controller and FACTS, the performance of the test system is assessed by the unified power flow controller (UPFC), which is usually used in traditional energy systems. To investigate the hybrid microgrid test system, this paper uses UPFC | Fuzzy logic | |
Tang [ ] | 2021 | Improving the minimum reactive system based on the harmonic analysis method | Artificial neural network, genetic algorithm | |
Harrye [ ] | 2014 | A new three-phase shift algorithm is presented that reduces the total reactive power of a converter | Artificial neural network | |
Sharma [ ] | 2012 | The method suggested reduces active power loss. All the control variables are bus generator tensions, tap locations and capacitor banks for shunt switchable | Particle swarm optimization | |
Wang [ ] | 2021 | To maximize the population’s ability to exploit a new space, the proposed algorithm employs a sequential optimization strategy | Genetic algorithm | |
Bhattacharyya [ ] | 2014 | FACTS devices, such as static var compensator and thyristor-controlled series compensator (TCSC), are placed at weak nodes in the power system by using fuzzy membership functions, while the TCSC is placed according to reactive power flow in lines in this proposed approach to FACTS | Fuzzy logic | |
Capacitor placement | Bharti [ ] | 2020 | A strategy to optimize the location of shunt capacitor banks in electricity distribution systems | Ant colony optimization, genetic algorithm |
Roy [ ] | 2020 | Reduced power loss through the optimal location of the condenser using AI techniques | Artificial neural network, fuzzy logic | |
Pimentel Filho [ ] | 2009 | The aim is to decrease overall losses by placing capacitor banks in distribution networks | Ant colony optimization | |
Isac [ ] | 2013 | The target function comprises energy loss, energy loss and condenser banks. The placement of the condenser sites is selected based on loss sensitivity factors | Fuzzy logic | |
Reddy [ ] | 2008 | A fuzzy and PSO method for placing condensers in the primary suppliers of the radial distribution systems was developed to reduce power losses and enhance the voltage profile | Fuzzy logic and particle swarm optimization | |
Shwehdi [ ] | 2018 | The article focuses on the performance between the stable and the transient states in the 380-kV transmission line West–East. To dynamically handle the condenser placement problem, the GA technique is explained and implemented | Genetic algorithm | |
Mahdavian [ ] | 2017 | The research aims to enhance the voltage profile and activity loss. Loss sensitivity and GA are utilized for the condenser placement and sizing | Fuzzy logic |
Reference | Year | Objective | Technique(s) | |
Distribution system planning | Kahouli [ ] | 2021 | An ideal approach to increasing the safety of a distribution system and decrease power loss by optimizing the network reconfiguration problem | Genetic algorithm and particle swarm optimization |
Žarković [ ] | 2019 | Although the primary goal of a DSP is to minimize the total cost of ownership, it also aims to maximize system reliability | Mixed-integer linear programming and genetic algorithm | |
Ahmetovic [ ] | 2021 | It is proposed that the Bellman–Zadeh decision-making process shall use the proposed fuzzy-inference system type Mamdani to assess the Powerline feeder reliability as a planning criterion | Fuzzy logic | |
Suresh [ ] | 2017 | These studies are crucial to establishing the status of each node or bus and conditions in the distribution system and these artificial neural networks are efficient at describing the relationship between the raw data and these neural networks | Artificial neural network | |
Kumari [ ] | 2018 | This article offers the optimal energy distribution system for routes and optimal drives with the lowest energy-loss costs | Particle swarm optimization | |
Saha [ ] | 2021 | Solving the optimal problem of diesel generator allocation | Genetic algorithm | |
Hosseini [ ] | 2021 | Cyber-physical detection, stochastic and cyber security enhancement to detect and estimate damages | Artificial neural network | |
Lytras [ ] | 2019 | There are different methods suggested to optimize distribution system planning | Particle swarm optimization | |
Gandhar [ ] | 2020 | Using a proportional–integral (PI) controller and FACTS, the performance of the test system is assessed by the unified power flow controller (UPFC), which is usually used in traditional energy systems. To investigate the hybrid microgrid test system, this paper uses UPFC | Fuzzy logic | |
Tang [ ] | 2021 | Improving the minimum reactive system based on the harmonic analysis method | Artificial neural network, genetic algorithm | |
Harrye [ ] | 2014 | A new three-phase shift algorithm is presented that reduces the total reactive power of a converter | Artificial neural network | |
Sharma [ ] | 2012 | The method suggested reduces active power loss. All the control variables are bus generator tensions, tap locations and capacitor banks for shunt switchable | Particle swarm optimization | |
Wang [ ] | 2021 | To maximize the population’s ability to exploit a new space, the proposed algorithm employs a sequential optimization strategy | Genetic algorithm | |
Bhattacharyya [ ] | 2014 | FACTS devices, such as static var compensator and thyristor-controlled series compensator (TCSC), are placed at weak nodes in the power system by using fuzzy membership functions, while the TCSC is placed according to reactive power flow in lines in this proposed approach to FACTS | Fuzzy logic | |
Capacitor placement | Bharti [ ] | 2020 | A strategy to optimize the location of shunt capacitor banks in electricity distribution systems | Ant colony optimization, genetic algorithm |
Roy [ ] | 2020 | Reduced power loss through the optimal location of the condenser using AI techniques | Artificial neural network, fuzzy logic | |
Pimentel Filho [ ] | 2009 | The aim is to decrease overall losses by placing capacitor banks in distribution networks | Ant colony optimization | |
Isac [ ] | 2013 | The target function comprises energy loss, energy loss and condenser banks. The placement of the condenser sites is selected based on loss sensitivity factors | Fuzzy logic | |
Reddy [ ] | 2008 | A fuzzy and PSO method for placing condensers in the primary suppliers of the radial distribution systems was developed to reduce power losses and enhance the voltage profile | Fuzzy logic and particle swarm optimization | |
Shwehdi [ ] | 2018 | The article focuses on the performance between the stable and the transient states in the 380-kV transmission line West–East. To dynamically handle the condenser placement problem, the GA technique is explained and implemented | Genetic algorithm | |
Mahdavian [ ] | 2017 | The research aims to enhance the voltage profile and activity loss. Loss sensitivity and GA are utilized for the condenser placement and sizing | Fuzzy logic |
Application of AI in various problems of the operation, control and planning of power systems has shown good performance over conventional methods. ABC algorithms function better than other AI techniques in the ELD problem, as shown in a comparison. The ABC algorithm has the highest-quality solutions, consistent convergence and exceptional computing efficiency. Compared with traditional mathematical models, both GA and PSO techniques are superior; the PSO technique is preferable since GA replaces humans. Unlike GA, PSO models alter humans through time and, in the following generation, all people survive. The fate of each person is continually adjusted based on the global ideal point thus far. The swarming effect in PSO allows the population of particles to move collectively in the search space, facilitating a more efficient exploration of the solution space and a faster convergence to optimum search areas compared with GA. PSO is particularly useful for optimization problems with many variables and when the solution space is complex and not well understood. Additionally, PSO requires minimal computational resources compared with GA as it does not require the evaluation and selection of multiple generations of offspring. In UC, with the increasing time, the execution time is also increasing, so the combination of many AI techniques can be progressive, as it can potentially increase the efficiency and accuracy of the optimization problem. The scalability of any heuristic optimization method is a major issue. In the load flow method, the ANFIS and ABC algorithms provide efficient and accurate solutions, and the implementation of ANNs is fast and can handle missing data effectively. In VC, rather than replacing conventional methods, the focus should be on enhancing their capabilities through the integration of AI techniques, modern control theory, fuzzy technology and ANNs, along with adaptive control and expert systems. This approach, which combines current research trends with practical experience, has great potential for practical applications. In load frequency control, the NARMA-L2 ANN network architecture provides the best output after some series of trials and improvements. Under fault conditions, it has been observed. The fuzzy-PID power output works so that the power systems are fast and stable. Due to the specialization, the fuzzy logic in condenser placement is better than other approaches. Fuzzy logic includes the relatively basic technique of calculating the necessary nodes in the distribution system to replace condensers with approximate reasoning. The results of the study indicate that the GA method can provide a globally optimal solution for reactive power optimization, particularly when an ample generation and population size is used. Furthermore, it was found that the use of the UPFC resulted in minimized losses compared with the static var compensator and thyristor-controlled series capacitor. In terms of reactive power planning, a fuzzy membership-based approach is employed in an interconnected power system to identify weak nodes and determine optimal parameter settings for FACTS devices. The feasibility of this strategy is validated through its solutions and compared with other global optimization approaches. The proposed technique is applied to a standard system under high load conditions, resulting in a stable system with reduced losses and cost savings. This approach has the potential to become a novel technology for effectively coordinating FACTS devices with other existing generators. Following the pandemic, it is expected that governments around the world will prioritize energy efficiency in buildings and smart homes. To support this, the development of open-source protocols and unified connectivity solutions is crucial. Smart home systems are focused on maximizing the energy efficiency of major household appliances, thereby contributing to overall energy balance. By constructing sustainable homes integrated with smart technologies and a combination of energy sources, significant cost savings and a reduction in carbon footprints can be achieved. While smart homes are becoming more common, there are still barriers to widespread adoption that researchers need to address. It emphasizes the potential of smart home companies and highlights technical challenges such as device compatibility. The review also discusses the importance of studying consumer attitudes and demands, and mentions the limitations of the survey methodology. Significant scientific results include an algorithm for a modern predictive analytics system, an approach to assess the condition index of the equipment and a method to determine the probability of defects using ML. The study validated the model using data from a hydroelectric power plant, demonstrating its accuracy. Future research can focus on refining the index calculation for equipment with multiple functional units and constructing predictive models for specific equipment classes. ML and data-driven techniques hold great promise in the field of power systems, especially in the context of smart grids. These methods can effectively analyse and process large volumes of data, resulting in improved accuracy and increased operational efficiency. However, some challenges need to be addressed, such as ensuring the quality of the data and interpreting the results in a meaningful way. Accurate forecasting plays a vital role in optimizing grid operations, integrating renewable energy sources and achieving cost-effective power generation. ML plays a vital role in transforming traditional grids into smart grids, improving reliability and safety. It also aids in demand-side management and enhances cybersecurity.
Evaluating energy costs and making improvements can lead to significant energy savings. Smart technologies have the potential to reduce electricity demand and environmental impact. Social acceptance of smart home systems needs to be promoted. Further research can expand sample sizes, include more diverse countries and explore smart meter readings. Future research should emphasize the importance of addressing technical, security and privacy concerns, and call for collaboration between stakeholders to enhance the smart home market. Although the developed approach offers several benefits, there is still an unresolved issue regarding the calculation of the technical condition index for equipment consisting of multiple functional units. Existing methods rely on assigning weights to each unit based on an expert evaluation to determine its importance. Additional research can focus on improving the methods for calculating the technical condition index for different types of power equipment and establishing predictive models to anticipate equipment defects in the event of functional unit failures. Future investigations should prioritize the development of more precise and dependable predictive models for power systems, taking into account the challenges related to data availability and interpretability.
Anshumaan Pathak and Utkarsh Pandey did the critical review. Surajit Mondal and Adesh Kumar supervised and reviewed the manuscript.
The authors declare that they have no known competing financial interests or personal relationships that could have appeared to influence the work reported in this paper.
The paper is a study and review article in which no specific data are referred to. No simulation software is used.
Sozontov A , Ivanova M , Gibadullin A. Implementation of artificial intelligence in the electric power industry . E3S Web of Conferences , 2019 , 114 : 01009 .
Google Scholar
Zhao X , Zhang X. Artificial intelligence applications in power system . In: Luo X (ed). Advances in Intelligent Systems Research—2016 2nd International Conference on Artificial Intelligence and Industrial Engineering (AIIE 2016). Dordrecht, Netherlands : Atlantis Press , 2016 , 158 – 161 .
Nath RP , Balaji VN. Artificial intelligence in power systems . IOSR Journal of Computer Engineering (IOSR-JCE) , 2014 . https://jcboseust.ac.in/electrical/images/notes/aitech_ug_ai_reactive_power_control.pdf ( 29 July 2023 , date last accessed).
Das S , Mukherjee M , Mondal S. Detailed energy audit of thermal power plant equipment . World Scientific News , 2015 , 22 : 70 – 90 .
Mondal S , Mondal AK , Sharma A , et al. . An overview of cleaning and prevention processes for enhancing efficiency of solar photovoltaic panels . Current Sci , 2018 , 115 : 1065 – 1077 .
Wasesa M , Tiara AR , Afrianto MA , et al. . SARIMA and artificial neural network models for forecasting electricity consumption of a microgrid based educational building . In: 2020 IEEE International Conference on Industrial Engineering and Engineering Management (IEEM), Singapore, 14–17 December 2020 , 210 – 214 .
Jang JS. ANFIS: adaptive-network-based fuzzy inference system . IEEE Trans Syst Man Cybern , 1993 , 23 : 665 – 685 .
Borges AF , Laurindo FJ , Spínola MM , et al. . The strategic use of artificial intelligence in the digital era: systematic literature review and future research directions . Int J Inf Manage , 2021 , 57 : 102225 .
Guan Y , Chaffart D , Liu G , et al. . Machine learning in solid heterogeneous catalysis: recent developments, challenges and perspectives . Chem Eng Sci , 2022 , 248 : 117224 .
Bordin C , Skjelbred HI , Kong J , et al. . Machine learning for hydropower scheduling: state of the art and future research directions . Procedia Comput Sci , 2020 , 176 : 1659 – 1668 .
Wang H , Ricardez-Sandoval LA. Dynamic optimization of a pilot-scale entrained-flow gasifier using artificial recurrent neural networks . Fuel , 2020 , 272 : 117731 .
Isnen M , Kurniawan S , Garcia-Palacios E. A-SEM: an adaptive smart energy management testbed for shiftable loads optimisation in the smart home . Measurement , 2020 , 152 : 107285 .
Bibri SE , Krogstie J. Environmentally data-driven smart sustainable cities: applied innovative solutions for energy efficiency, pollution reduction, and urban metabolism . Energy Informatics , 2020 , 3 : 1 – 59 .
Strielkowski W. Social Impacts of Smart Grids: The Future of Smart Grids and Energy Market Design . Amsterdam, Netherlands : Elsevier , 2019 .
Google Preview
Oliveira L , Mitchell V , May A. Smart home technology—comparing householder expectations at the point of installation with experiences 1 year later . Pers Ubiquitous Comput , 2020 , 24 : 613 – 626 .
Tao M , Zuo J , Liu Z , et al. . Multi-layer cloud architectural model and ontology-based security service framework for IoT-based smart homes . Future Gener Comput Syst , 2018 , 78 : 1040 – 1051 .
Shcherbatov IA , Turikov GN. Determination of power engineering equipment’s defects in predictive analytic system using machine learning algorithms . J Phys Conf Ser , 2020 , 1683 : 042056 .
Moleda M , Momot A , Mrozek D. Predictive maintenance of boiler feed water pumps using SCADA data . Sensors , 2020 , 20 : 571 .
Ren C , Xu Y. A fully data-driven method based on generative adversarial networks for power system dynamic security assessment with missing data . IEEE Trans Power Syst , 2019 , 34 : 5044 – 5052 .
Yu J , Guo Y , Sun H. Testbeds for integrated transmission and distribution networks: generation methodology and benchmarks . CSEE J Power Energy Syst , 2020 , 6 : 518 – 527 .
Gonen T. Electric Power Distribution Engineering . Boca Raton, FL, USA : CRC Press , 2015 .
Wood AJ , Wollenberg BF , Sheblé GB. Power Generation, Operation, and Control . Hoboken, NJ, USA : John Wiley & Sons , 2013 .
Kumar N , Mishra VM , Kumar A. Smart grid and nuclear power plant security by integrating cryptographic hardware chip . Nuclear Engineering and Technology , 2021 , 53 : 3327 – 3334 .
Kumar N , Mishra VM , Kumar A. Smart grid security by embedding s-box advanced encryption standard . Intelligent Automation &. Soft Comput , 2022 , 34 : 623 – 638 .
Xu G , Wang Z. Power system load flow distribution research based on adaptive neuro-fuzzy inference systems . In: 2012 Spring Congress on Engineering and Technology, Xi’an, China, 27–30 May 2012 , 1 – 4 .
Karaboga D , Akay B. A comparative study of artificial bee colony algorithm . Appl Math Comput , 2009 , 214 : 108 – 132 .
Nualhong D , Chusanapiputt S , Phomvuttisarn S , et al. . Diversity control approach to ant colony optimization for unit commitment problem . In: 2004 IEEE Region 10 Conference TENCON 2004, Chiang Mai, Thailand, 24 November 2004 , 488 – 491 .
Ho SL , Yang S , Ni G , et al. . A particle swarm optimization-based method for multiobjective design optimizations . IEEE Trans Magn , 2005 , 41 : 1756 – 1759 .
Ratnaweera A , Halgamuge SK , Watson HC. Self-organizing hierarchical particle swarm optimizer with time-varying acceleration coefficients . IEEE Trans Evol Comput , 2004 , 8 : 240 – 255 .
Kumar N , Nangia U , Sahay KB. Economic load dispatch using improved particle swarm optimization algorithms . In: 2014 6th IEEE Power India International Conference (PIICON), Delhi, India, 5–7 December 2014 , 1 – 6 .
Panta S , Premrudeepreechacharn S. Economic dispatch for power generation using artificial neural network ICPE’07 conference in Daegu, Korea . In: 2007 7th International Conference on Power Electronics, Daegu, South Korea, 22–26 October 2007 , 558 – 562 .
Rahmat NA , Musirin I. Differential evolution ant colony optimization (DEACO) technique in solving economic load dispatch problem . In: 2012 IEEE International Power Engineering and Optimization Conference, Melaka, Malaysia, 6–7 June 2012 , 263 – 268 .
Saxena A , Pandey SN , Srivastava L. Congestion management in open access—a review . International Journal of Science, Engineering and Technology Research , 2013 , 2 : 922 – 930 .
Emami H , Sadri JA. Congestion management of transmission lines in the market environment . International Research Journal of Applied and Basic Sciences , 2012 , 3 : 2572 – 2580 .
Ghosh K , Pandey U , Pathak A , et al. . Simulation of density based traffic control system using Proteus 7.1 professional . In: Mathur G , Bundele M , Tripathi A , Paprzycki M (eds). Proceedings of 3rd International Conference on Artificial Intelligence: Advances and Applications: ICAIAA 2022. Singapore: Springer Nature Singapore , 2023 , 493 – 504 .
Deb S , Goswami AK. Congestion management by generator rescheduling using artificial bee colony optimization technique . In: 2012 Annual IEEE India Conference (INDICON), Kochi, India, 7–9 December 2012 , 909 – 914 .
Choudekar P , Sinha SK , Siddiqui A. Transmission line efficiency improvement and congestion management under critical contingency condition by optimal placement of TCSC . In: 2016 7th India International Conference on Power Electronics (IICPE), Patiala, India, 17–19 November 2016 , 1 – 6 .
Wang X , McDonald JR. Modern Power System Planning . London, UK : McGraw-Hill , 1994 , 247 – 307 .
Dahal K , McDonald J. A review of generator maintenance scheduling using artificial intelligence techniques . In: Proceedings of the 32nd Universities Power Engineering Conference (UPEC ’97), Manchester, UK, 10–12 September 1997 , 787 – 790 .
Koay CA , Srinivasan D. Particle swarm optimization-based approach for generator maintenance scheduling . In: Proceedings of the 2003 IEEE Swarm Intelligence Symposium. SIS’03 (Cat. No. 03EX706), Indianapolis, IN, USA, 26 April 2003 , 167 – 173 .
Johnson RC , Happ HH , Wright WJ. Large scale hydro-thermal unit commitment: method and results . IEEE Trans Power Appar Syst , 1971 , PAS-90 : 1373 – 1384 .
Thum YM. Hierarchical linear models for multivariate outcomes . Journal of Educational and Behavioral Statistics , 1997 , 22 : 77 – 108 .
Reyes DM , de Souza RM , de Oliveira AL. A three-stage approach for modeling multiple time series applied to symbolic quartile data . Expert Syst Appl , 2022 , 187 : 115884 .
Almaghrebi A , Aljuheshi F , Rafaie M , et al. . Data-driven charging demand prediction at public charging stations using supervised machine learning regression methods . Energies , 2020 , 13 : 4231 .
Huang P , Copertaro B , Zhang X , et al. . A review of data centers as prosumers in district energy systems: renewable energy integration and waste heat reuse for district heating . Appl Energy , 2020 , 258 : 114109 .
Sun L , You F. Machine learning and data-driven techniques for the control of smart power generation systems: an uncertainty handling perspective . Engineering , 2021 , 7 : 1239 – 1247 .
Lisin E , Shuvalova D , Volkova I , et al. . Sustainable development of regional power systems and the consumption of electric energy . Sustainability , 2018 , 10 : 1111 .
Prakash S , Jain J , Hasnat S , et al. . Economic load dispatch with valve point loading effect using optimization techniques . In: Bansal P , Tushir M , Balas VE , Srivastava R (eds). Proceedings of International Conference on Artificial Intelligence and Applications: ICAIAA 2020, Singapore: Springer , 2021 , 407 – 416 .
Chowdhury AK , Mondal S , Mukherjee M , Biswas PK. Mega watt security assessment of power systems . International Letters of Chemistry, Physics and Astronomy, 2015 , 58 : 9 – 15 .
Dommel HW , Tinney WF. Optimal power flow solutions . IEEE Transactions on Power Apparatus and Systems, 1968 , PAS-87 : 1866 – 1876 .
Wakiru JM , Pintelon L , Muchiri P , et al. . A comparative analysis of maintenance strategies and data application in asset performance management for both developed and developing countries . International Journal of Quality & Reliability Management , 2022 , 39 : 961 – 983 .
Muñoz-Delgado G , Contreras J , Arroyo JM , et al. . Integrated transmission and distribution system expansion planning under uncertainty . IEEE Trans Smart Grid , 2021 , 12 : 4113 – 4125 .
Chowdhury AK , Mondal S , Alam SM , et al. . Voltage security assessment of power system . World Scientific News , 2015 , 21 : 36 – 50 .
Holen AT , Botnen A , Stoa P , et al. . Coupling between knowledge-based and algorithmic methods . Proc IEEE , 1992 , 80 : 745 – 757 .
Short MJ , Hui KC , Ekwue AO , et al. . Applications of artificial neural networks for NGC voltage collapse monitoring . International Conference On Large High Voltage Electric Systems, 1994 , 2 : 38 – 205 .
Haida T , Akimoto T. Voltage optimization using genetic algorithm . In: Proc. 3rd Symposium on Expert System Applications to Power Systems, Tokyo, Japan, 1991 , 375 – 380 .
Denny FI , Dismukes DE. Power System Operations and Electricity Markets . Boca Raton, FL, USA : CRC Press , 2017 .
Masiala M , Ghribi M , Kaddouri A. An adaptive fuzzy controller gain scheduling for power system load-frequency control . In: 2004 IEEE International Conference on Industrial Technology, IEEE ICIT’04, Vol. 3 , Hammamet, Tunisia, 8–10 December 2004 , 1515 – 1520 .
Alquthami T , Butt SE , Tahir MF , et al. . Short-term optimal scheduling of hydro-thermal power plants using artificial bee colony algorithm . Energy Rep , 2020 , 6 : 984 – 992 .
Sahay KB , Sonkar A , Kumar A. Economic load dispatch using genetic algorithm optimization technique . In: 2018 International Conference and Utility Exhibition on Green Energy for Sustainable Development (ICUE), Phuket, Thailand, 24–26 October 2018 , 1 – 5 .
Mishra SK , Mishra SK. A comparative study of solution of economic load dispatch problem in power systems in the environmental perspective . Procedia Comput Sci , 2015 , 48 : 96 – 100 .
Dixit GP , Dubey HM , Pandit M , et al. . Artificial bee colony optimization for combined economic load and emission dispatch . In: International Conference on Sustainable Energy and Intelligent Systems (SEISCON 2011), Chennai, India, 20–22 July 2011 , 340 – 345 .
Daniel L , Chaturvedi KT , Kolhe ML. Dynamic economic load dispatch using Levenberg Marquardt algorithm . Energy Procedia , 2018 , 144 : 95 – 103 .
Ruiz-Abellón MC , Fernández-Jiménez LA , Guillamón A , et al. . Integration of demand response and short-term forecasting for the management of prosumers’ demand and generation . Energies , 2020 , 13 : 11 .
Ali SS , Choi BJ. State-of-the-art artificial intelligence techniques for distributed smart grids: a review . Electronics , 2020 , 9 : 1030 .
Fu J , Nunez A , De Schutter B. A short-term preventive maintenance scheduling method for distribution networks with distributed generators and batteries . IEEE Trans Power Syst , 2020 , 36 : 2516 – 2531 .
Esmaili M , Shayanfar HA , Moslemi R. Locating series FACTS devices for multi-objective congestion management improving voltage and transient stability . Eur J Oper Res , 2014 , 236 : 763 – 773 .
Suresh K , Kumarappan N. Hybrid improved binary particle swarm optimization approach for generation maintenance scheduling problem . Swarm Evol Comput , 2013 , 9 : 69 – 89 .
Lakshminarayanan S , Kaur D. Optimal maintenance scheduling of generator units using discrete integer cuckoo search optimization algorithm . Swarm Evol Comput , 2018 , 42 : 89 – 98 .
Scalabrini Sampaio G , Vallim Filho ARDA , Santos da Silva L , et al. . Prediction of motor failure time using an artificial neural network . Sensors , 2019 , 19 : 4342 .
Fikri M , Cheddadi B , Sabri O. Predicting Moroccan real network’s power flow employing the artificial neural networks . In: 2019 Third International Conference on Intelligent Computing in Data Sciences (ICDS), Marrakech, Morocco, 28–30 October 2019 , 1 – 6 .
Rahul J , Sharma Y , Birla D. A new attempt to optimize optimal power flow-based transmission losses using genetic algorithm . In: 2012 Fourth International Conference on Computational Intelligence and Communication Networks, Mathura, India, 3–5 November 2012 , 566 – 570 .
Nakawiro W , Erlich I. A combined GA-ANN strategy for solving optimal power flow with voltage security constraint . In: 2009 Asia-Pacific Power and Energy Engineering Conference, Wuhan, China, 27–31 March 2009 , 1 – 4 .
Sumpavakup C , Srikun I , Chusanapiputt S. A solution to the optimal power flow using artificial bee colony algorithm . In: 2010 International Conference on Power System Technology, Zhejiang, China, 24–28 October 2010 , 1 – 5 .
Abdellah D , Djamel L. Power flow analysis using adaptive neuro-fuzzy inference systems . In: 2015 3rd International Renewable and Sustainable Energy Conference (IRSEC), Marrakech, Morocco, 10–13 December 2015 , 1 – 5 .
Nemati M , Braun M , Tenbohlen S. Optimization of unit commitment and economic dispatch in microgrids based on genetic algorithm and mixed integer linear programming . Appl Energy , 2018 , 210 : 944 – 963 .
Alshareef A. An application of artificial intelligent optimization techniques to dynamic unit commitment for the western area of Saudi Arabia . In: 2011 Third International Conference on Computational Intelligence, Communication Systems and Networks, Bali, Indonesia, 26–28 July 2011 , 17 – 21 .
Arora I , Kaur M. Unit commitment scheduling by employing artificial neural network-based load forecasting . In: 2016 7th India International Conference on Power Electronics (IICPE), Patiala, India, 17–19 November 2016 , 1 – 6 .
Liu Z , Li N , Zhang C. Unit commitment scheduling using a hybrid ANN and Lagrangian relaxation method . In: 2008 International Conference on Multimedia and Ubiquitous Engineering (mue 2008), Busan, South Korea, 24–25 April 2008 , 481 – 484 .
Kumar VS , Mohan MR. Solution to security constrained unit commitment problem using genetic algorithm . International Journal of Electrical Power & Energy Systems , 2010 , 32 : 117 – 125 .
Prakash S , Sinha SK. Application of artificial intelligence in load frequency control of interconnected power system . International Journal of Engineering, Science and Technology , 2011 , 3 : 264 – 275 .
Moshtagh J , Rafinia A. A new approach to high impedance fault location in three-phase underground distribution system using combination of fuzzy logic & wavelet analysis . In: 2012 11th International Conference on Environment and Electrical Engineering, Venice, Italy, 15–18 May 2012 , 90 – 97 .
Santoso NT , Tan OT. Neural-net based realtime control of capacitors installed on distribution systems . IEEE Trans. Power Delivery , 1990 , 5 : 266 – 272 .
Ng HN , Salama MMA , Chikhani AY. Capacitor allocation by approximate reasoning: fuzzy capacitor placement . IEEE Trans Power Deliv , 2000 , 15 : 393 – 398 .
Zhang H , Zhang L , Meng F. Reactive power optimization based on genetic algorithm . In: POWERCON’98. International Conference on Power System Technology. Proceedings, Vol. 2 , Beijing, China, 18–21 August 1998 , 1448 – 1453 .
Mamandur KRC , Chenoweth RD. Optimal control of reactive power flow for improvements in voltage profiles and for real power loss minimization . IEEE Transactions on Power Apparatus and Systems, 1981 , PAS-100 : 3185 – 3194 .
Kothakotla V , Kumar B. Analysis and design of robust PID controller for grid voltage control of islanded microgrid using genetic algorithm . In: 2021 5th International Conference on Intelligent Computing and Control Systems (ICICCS), Madurai, India, 6–8 May 2021 , 719 – 726 .
Wang S , Duan J , Shi D , et al. . A data-driven multi-agent autonomous voltage control framework using deep reinforcement learning . IEEE Trans Power Syst , 2020 , 35 : 4644 – 4654 .
Zidani Y , Zouggar S , Elbacha A. Steady-state analysis and voltage control of the self-excited induction generator using artificial neural network and an active filter . Iranian Journal of Science and Technology, Transactions of Electrical Engineering , 2018 , 42 : 41 – 48 .
Sumathi S. Artificial neural network application for voltage control and power flow control in power systems with UPFC . In: 2015 International Conference on Emerging Research in Electronics, Computer Science and Technology (ICERECT), Mandya, India, 17–19 December 2015 , 403 – 407 .
Kanata S , Sianipar GH , Maulidevi NU. Optimization of reactive power and voltage control in power system using hybrid artificial neural network and particle swarm optimization . In: 2018 2nd International Conference on Applied Electromagnetic Technology (AEMT), Lombok, Indonesia, 9–12 April 2018 , 67 – 72 .
Abdalla OH , Ghany AA , Fayek HH. Coordinated PID secondary voltage control of a power system based on genetic algorithm . In: 2016 Eighteenth International Middle East Power Systems Conference (MEPCON), Cairo, Egypt, 27–29 December 2016 , 214 – 219 .
Chung IY , Liu W , Cartes DA. Control parameter optimization for a microgrid system using particle swarm optimization . In: 2008 IEEE International Conference on Sustainable Energy Technologies, Singapore, 24–27 November 2008 , 837 – 842 .
Yousuf H , Zainal AY , Alshurideh M , et al. . Artificial intelligence models in power system analysis . In: Hassanien AE , Bhatnagar R , Darwish A (eds). Artificial Intelligence for Sustainable Development: Theory, Practice and Future Applications . Cham, Switzerland : Springer , 2021 , 231 – 242 .
Aakula JL , Khanduri A , Sharma A. Determining reactive power levels to improve bus voltages using PSO . In: 2020 IEEE 17th India Council International Conference (INDICON), New Delhi, India, 10–13 December 2020 , 1 – 7 .
Karthikeyan R , Pasam S , Sudheer S , et al. . Fuzzy fractional order PID based parallel cascade control system . In: Thampi S , Abraham A , Pal S , Rodriguez J (eds). Recent Advances in Intelligent Informatics. Advances in Intelligent Systems and Computing , Vol. 235 . Cham, Switzerland : Springer , 2014 , 293 – 302 .
Sallama A , Abbod M , Taylor G. Supervisory power system stability control using neuro-fuzzy system and particle swarm optimization algorithm . In: 2014 49th International Universities Power Engineering Conference (UPEC), Cluj-Napoca, Romania, 2–5 September 2014 , 1 – 6 .
Chen B , Li W , Yu P , et al. . Planning approach for cross-regional optical transmission network supporting wide-area stability control services in power system . In: 2018 International Conference on Network Infrastructure and Digital Content (IC-NIDC), Guiyang, China, 22–24 August 2018 , 105 – 109 .
Torkzadeh R , NasrAzadani H , Aliabad AD , et al. . A genetic algorithm optimized fuzzy logic controller for UPFC in order to damp of low frequency oscillations in power systems . In: 2014 22nd Iranian Conference on Electrical Engineering (ICEE), Tehran, Iran, 20–22 May 2014 , 706 – 712 .
Dutta S , Singh SP. Optimal rescheduling of generators for congestion management based on particle swarm optimization . IEEE Trans Power Syst , 2008 , 23 : 1560 – 1569 .
Nam S , Hur J. Probabilistic forecasting model of solar power outputs based on the naive Bayes classifier and kriging models . Energies , 2018 , 11 : 2982 .
Miraftabzadeh SM , Longo M , Foiadelli F , et al. . Advances in the application of machine learning techniques for power system analytics: a survey . Energies , 2021 , 14 : 4776 .
Safari A , Babaei F , Farrokhifar M. A load frequency control using a PSO-based ANN for micro-grids in the presence of electric vehicles . Int J Ambient Energy , 2021 , 42 : 688 – 700 .
Joshi M , Sharma G , Davidson IE. Load frequency control of hydro electric system using application of fuzzy with particle swarm optimization algorithm . In: 2020 International Conference on Artificial Intelligence, Big Data, Computing and Data Communication Systems (icABCD), Durban, South Africa, 6–7 August 2020 , 1 – 6 .
Nguyen GN , Jagatheesan K , Ashour AS , et al. . Ant colony optimization-based load frequency control of multi-area interconnected thermal power system with governor dead-band nonlinearity . In: Yang XS , Nagar AK , Joshi A (eds). Smart Trends in Systems, Security and Sustainability . Singapore : Springer , 2018 , 157 – 167 .
Balamurugan CR. Three area power system load frequency control using fuzzy logic controller . International Journal of Applied Power Engineering (IJAPE) , 2018 , 7 : 18 – 26 .
Otani T , Tanabe R , Koyanagi Y , et al. . Cooperative load frequency control of generator and battery using a recurrent neural network . In: TENCON 2017–2017 IEEE Region 10 Conference, Penang, Malaysia, 5–8 November 2017 , 918 – 923 .
Kumar D , Mathur HD , Bhanot S , et al. . Forecasting of solar and wind power using LSTM RNN for load frequency control in isolated microgrid . Int J Modelling Simul , 2021 , 41 : 311 – 323 .
Arora K , Kumar A , Kamboj VK , et al. . Optimization methodologies and testing on standard benchmark functions of load frequency control for interconnected multi area power system in smart grids . Mathematics , 2020 , 8 : 980 .
Vadivelu KR , Marutheswar GV. Artificial intelligence technique based reactive power planning incorporating FACTS controllers in real time power transmission system . In: 2014 IEEE 2nd International Conference on Electrical Energy Systems (ICEES), Chennai, India, 7–9 January 2014 , 26 – 31 .
Khan MA , Algarni F. A healthcare monitoring system for the diagnosis of heart disease in the IoMT cloud environment using MSSO-ANFIS . IEEE Access , 2020 , 8 : 122259 – 122269 .
Liu M , Dong M , Wu C. A new ANFIS for parameter prediction with numeric and categorical inputs . IEEE Trans Autom Sci Eng , 2010 , 7 : 645 – 653 .
Ghadiri A , Haghifam MR , Larimi SMM. Comprehensive approach for hybrid AC/DC distribution network planning using genetic algorithm . IET Generation, Transmission & Distribution , 2017 , 11 : 3892 – 3902 .
Jain SK , Bhargava A , Pal RK. Three area power system load frequency control using fuzzy logic controller . In: 2015 International Conference on Computer, Communication and Control (IC4), Indore, India, 10–12 September 2015 , 1 – 6 .
Son YS , Kim HJ , Kim JT. A video-quality control scheme using ANFIS architecture in a DASH environment . Journal of Broadcast Engineering , 2018 , 23 : 104 – 114 .
Kannadasan K , Edla DR , Yadav MH , et al. . Intelligent-ANFIS model for predicting measurement of surface roughness and geometric tolerances in three-axis CNC milling . IEEE Trans Instrum Meas , 2020 , 69 : 7683 – 7694 .
Penghui L , Ewees AA , Beyaztas BH , et al. . Metaheuristic optimization algorithms hybridized with artificial intelligence model for soil temperature prediction: novel model . IEEE Access , 2020 , 8 : 51884 – 51904 .
Tawfeek TS , Ahmed AH , Hasan S. Analytical and particle swarm optimization algorithms for optimal allocation of four different distributed generation types in radial distribution networks . Energy Procedia , 2018 , 153 : 86 – 94 .
Yeom CU , Kwak KC. Adaptive neuro-fuzzy inference system predictor with an incremental tree structure based on a context-based fuzzy clustering approach . Applied Sciences , 2020 , 10 : 8495 .
Krasopoulos CT , Beniakar ME , Kladas AG. Multicriteria PM motor design based on ANFIS evaluation of EV driving cycle efficiency . IEEE Trans Transp Electrif , 2018 , 42 : 525 – 535 .
El-Hasnony IM , Barakat SI , Mostafa . Optimized ANFIS model using hybrid metaheuristic algorithms for Parkinson’s disease prediction in IoT environment . IEEE Access , 2020 , 8 : 119252 – 119270 .
Morshedizadeh M , Kordestani M , Carriveau R , et al. . Power production prediction of wind turbines using a fusion of MLP and ANFIS networks . IET Renew Power Gener , 2018 , 12 : 1025 – 1033 .
Khosravi A , Nahavandi S , Creighton D. Prediction interval construction and optimization for adaptive neurofuzzy inference systems . IEEE Trans Fuzzy Syst , 2011 , 19 : 983 – 988 .
Elbaz K , Shen SL , Sun WJ , et al. . Prediction model of shield performance during tunneling via incorporating improved particle swarm optimization into ANFIS . IEEE Access , 2020 , 8 : 39659 – 39671 .
Dovžan D , Škrjanc I. Fuzzy space partitioning based on hyperplanes defined by eigenvectors for Takagi-Sugeno fuzzy model identification . IEEE Trans Ind Electron , 2019 , 67 : 5144 – 5153 .
Castiello C , Fanelli AM , Lucarelli M , et al. . Interpretable fuzzy partitioning of classified data with variable granularity . Appl Soft Comput , 2019 , 74 : 567 – 582 .
Alexandridis A , Chondrodima E , Sarimveis H. Radial basis function network training using a nonsymmetric partition of the input space and particle swarm optimization . IEEE Trans Neural Networks Learn Syst , 2012 , 24 : 219 – 230 .
Verstraete J. The spatial disaggregation problem: simulating reasoning using a fuzzy inference system . IEEE Trans Fuzzy Syst , 2016 , 25 : 627 – 641 .
Olamaei J , Moradi M , Kaboodi T. A new adaptive modified firefly algorithm to solve optimal capacitor placement problem . In: 18th Electric Power Distribution Conference, Kermanshah, Iran, 30 April–1 May 2013 , 1 – 6 .
Lee JS , Teng CL. An enhanced hierarchical clustering approach for mobile sensor networks using fuzzy inference systems . IEEE Internet Things J , 2017 , 4 : 1095 – 1103 .
Su ZG , Denoeux T. BPEC. Belief-peaks evidential clustering . IEEE Trans Fuzzy Syst , 2018 , 27 : 111 – 123 .
Xu P , Deng Z , Cui C , et al. . Concise fuzzy system modeling integrating soft subspace clustering and sparse learning . IEEE Trans Fuzzy Syst , 2019 , 27 : 2176 – 2189 .
Sujil A , Kumar R , Bansal RC. FCM clustering-ANFIS-based PV and wind generation forecasting agent for energy management in a smart microgrid . The Journal of Engineering , 2019 , 2019 : 4852 – 4857 .
Neamatollahi P , Naghibzadeh M , Abrishami S. Fuzzy-based clustering-task scheduling for lifetime enhancement in wireless sensor networks . IEEE Sens J , 2017 , 17 : 6837 – 6844 .
Wang Z , Xu Y. A golden section-based double population genetic algorithm applied to reactive power optimization . IOP Conference Series: Earth and Environmental Science, 2021 , 645 : 012074 .
Kahouli O , Alsaif H , Bouteraa Y , et al. . Power system reconfiguration in distribution network for improving reliability using genetic algorithm and particle swarm optimization . Applied Sciences , 2021 , 11 : 3092 .
Žarković SD , Stanković S , Shayesteh E , et al. . Reliability improvement of distribution system through distribution system planning: MILP vs. GA . In: 2019 IEEE Milan PowerTech, Milan, Italy, 23–27 June 2019, 1 – 6 .
Ahmetovic H , Saric M , Hivziefendic J. Reliability based power distribution network planning using fuzzy logic . Advances in Electrical and Electronic Engineering , 2021 , 19 : 123 – 133 .
Suresh M , Sirish TS , Subhashini TV , et al. . Load flow analysis of distribution system using artificial neural networks . In: Satapathy SC , Bhateja V , Udgata SK , Pattnaik PK (eds). Proceedings of the 5th International Conference on Frontiers in Intelligent Computing: Theory and Applications FICTA 2016, Vol. 1 . Springer , Singapore , 2017 , 515 – 524 .
Kumari M , Ranjan R , Singh VR. Optimal power distribution planning using improved particle swarm optimization . International Journal of Intelligent Systems and Applications in Engineering , 2018 , 3 : 170 – 177 .
Saha S , Mukherjee V. A novel multi-objective modified symbiotic organisms search algorithm for optimal allocation of distributed generation in radial distribution system . Neural Comput Appl , 2021 , 33 : 1751 – 1771 .
Hosseini MM , Parvania M. Artificial intelligence for resilience enhancement of power distribution systems . The Electricity Journal , 2021 , 34 : 106880 .
Lytras MD , Chui KT. The recent development of artificial intelligence for smart and sustainable energy systems and applications . Energies , 2019 , 12 : 3108 .
Gandhar S , Ohri J , Singh M. Dynamic reactive power optimization of hybrid micro grid in islanded mode using fuzzy tuned UPFC . J Inf Optim Sci , 2020 , 41 : 305 – 315 .
Tang Y , Hu W , Cao D , et al. . Artificial intelligence-aided minimum reactive power control for the DAB converter based on harmonic analysis method . IEEE Trans Power Electron , 2021 , 36 : 9704 – 9710 .
Harrye YA , Ahmed KH , Aboushady AA. Reactive power minimization of dual active bridge DC/DC converter with triple phase shift control using neural network . In: 2014 International Conference on Renewable Energy Research and Application (ICRERA), Milwaukee, WI, USA, 19–22 October 2014 , 566 – 571 .
Wang Y , Li Y. A hybrid ant colony optimization algorithm for dynamic optimization of vehicle routing problem with time windows . Applied Intelligence , 2021 , 51 : 476 – 491 .
Sharma NK , Babu DS , Choube SC. Application of particle swarm optimization technique for reactive power optimization . In: IEEE International Conference on Advances in Engineering, Science and Management (ICAESM-2012), Nagapattinam, India, 30–31 March 2020 , 88 – 93 .
Bhattacharyya B , Gupta VK. Fuzzy based evolutionary algorithm for reactive power optimization with FACTS devices . International Journal of Electrical Power & Energy Systems , 2014 , 61 : 39 – 47 .
Bharti D. Multi-point optimal placement of shunt capacitor in radial distribution network: a comparison . In: 2020 International Conference on Emerging Frontiers in Electrical and Electronic Technologies (ICEFEET), Patna, India, 10–11 July 2020 , 1 – 6 .
Roy PK , Sultana S. Optimal reconfiguration of capacitor based radial distribution system using chaotic quasi oppositional chemical reaction optimization . Microsyst Technol , 2022 , 28 : 499 – 511 .
Pimentel Filho MC , De Lacerda EGM , Junior MM. Capacitor placement using ant colony optimization and gradient . In: 2009 15th International Conference on Intelligent System Applications to Power Systems, Curitiba, Brazil, 8–12 November 2009 , 1 – 4 .
Isac SJ , Kumar KS , Kumar PV. Optimal capacitor placement in radial distribution system to minimize the loss using fuzzy logic control . In: International Conference on Smart Structures and Systems—ICSSS; 13 , Chennai, India, 28–29 March 2013 , 33 – 40 .
Reddy MD , Reddy VV. Capacitor placement using fuzzy and particle swarm optimization method for maximum annual savings . ARPN Journal of Engineering and Applied Sciences , 2008 , 3 : 25 – 30 .
Shwehdi MH , Mohamed SR , Devaraj D. Optimal capacitor placement on West–East inter-tie in Saudi Arabia using genetic algorithm . Computers & Electrical Engineering , 2018 , 68 : 156 – 169 .
Mahdavian M , Kafi MH , Movahedi A , et al. . Improve performance in electrical power distribution system by optimal capacitor placement using genetic algorithm . In: 2017 14th International Conference on Electrical Engineering/Electronics, Computer, Telecommunications, and Information Technology (ECTI-CON), Phuket, Thailand, 27–30 June 2017 , 749 – 752 .
Month: | Total Views: |
---|---|
November 2023 | 210 |
December 2023 | 416 |
January 2024 | 1,058 |
February 2024 | 1,179 |
March 2024 | 1,923 |
April 2024 | 1,654 |
May 2024 | 1,624 |
June 2024 | 1,376 |
July 2024 | 1,573 |
August 2024 | 589 |
Email alerts
Citing articles via.
- Advertising and Corporate Services
Affiliations
- Online ISSN 2515-396X
- Print ISSN 2515-4230
- Copyright © 2024 National Institute of Clean-and-Low-Carbon Energy
- About Oxford Academic
- Publish journals with us
- University press partners
- What we publish
- New features
- Open access
- Institutional account management
- Rights and permissions
- Get help with access
- Accessibility
- Advertising
- Media enquiries
- Oxford University Press
- Oxford Languages
- University of Oxford
Oxford University Press is a department of the University of Oxford. It furthers the University's objective of excellence in research, scholarship, and education by publishing worldwide
- Copyright © 2024 Oxford University Press
- Cookie settings
- Cookie policy
- Privacy policy
- Legal notice
This Feature Is Available To Subscribers Only
Sign In or Create an Account
This PDF is available to Subscribers Only
For full access to this pdf, sign in to an existing account, or purchase an annual subscription.
IEEE Account
- Change Username/Password
- Update Address
Purchase Details
- Payment Options
- Order History
- View Purchased Documents
Profile Information
- Communications Preferences
- Profession and Education
- Technical Interests
- US & Canada: +1 800 678 4333
- Worldwide: +1 732 981 0060
- Contact & Support
- About IEEE Xplore
- Accessibility
- Terms of Use
- Nondiscrimination Policy
- Privacy & Opting Out of Cookies
A not-for-profit organization, IEEE is the world's largest technical professional organization dedicated to advancing technology for the benefit of humanity. © Copyright 2024 IEEE - All rights reserved. Use of this web site signifies your agreement to the terms and conditions.
Current status and future scenarios of carbon capture from power plants emission: a review
- Review paper
- Published: 01 July 2023
- Volume 22 , pages 799–822, ( 2023 )
Cite this article
- Dalal Alalaiwat 1 , 2 &
- Ezzat Khan ORCID: orcid.org/0000-0001-7849-6083 1 , 3
1433 Accesses
9 Citations
Explore all metrics
The emission from power plants highly contributes to the increase of CO 2 concentration in the atmosphere. Enhancing the utilization of renewable energy and improving energy efficiency are widely considered to be a key to reduce emissions, however, certain solutions need time to be implemented. Carbon Capture and Storage is considered as a crucial solution for the situation that contributes to reduce the ultimate emission. Most studies have dealt with the current available technologies such as pre-combustion capture, post-combustion capture, and oxyfuel combustion. Several challenges of Carbon Capture technologies are being addressed without being given more attention to the optimum solution. Although post-combustion capture is considered one of the best options to be retrofitted to a power plant, the challenges of the processes and the possible solutions have not been dealt with in depth. This review article investigates the three techniques (pre-combustion, post-combustion, and oxyfuel combustion) to identify the best option to be used for CO 2 capture from the power plant. The gap in the field of decarbonization for researchers and policy makers has been identified and a future roadmap has therefore been proposed. Post-combustion capture is the best option for carbon capture from power plants. Solvent selection, process configuration, and process emissions to environment need to be resolved for better capture results. Three future scenarios are hereby proposed, the recirculation of the clean flue gas, synergistic effect of different solvents or replacement of stripper unit with ultrasound technique.
Graphical abstract
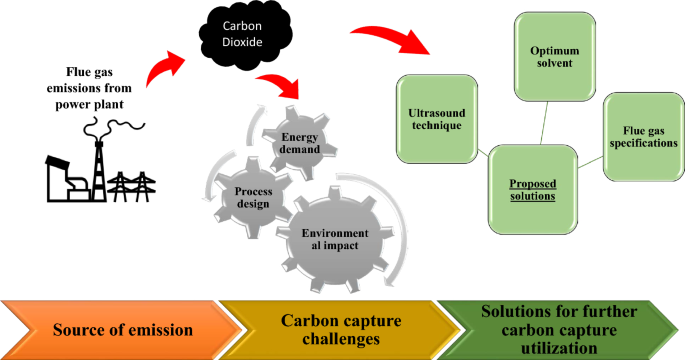
This is a preview of subscription content, log in via an institution to check access.
Access this article
Subscribe and save.
- Get 10 units per month
- Download Article/Chapter or eBook
- 1 Unit = 1 Article or 1 Chapter
- Cancel anytime
Price includes VAT (Russian Federation)
Instant access to the full article PDF.
Rent this article via DeepDyve
Institutional subscriptions
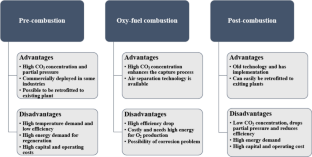
Similar content being viewed by others
Carbon Capture Technologies
A Review on the Advanced Techniques Used for the Capturing and Storage of CO2 from Fossil Fuel Power Plants
Co2 capture rd&d proceedings in china huaneng group, explore related subjects.
- Environmental Chemistry
Al-Mamoori A, Krishnamurthy A, Rownaghi AA, Rezaei F (2017) Carbon capture and utilization update. Energy Technol 5(6):834–849. https://doi.org/10.1002/ente.201600747
Article Google Scholar
Amrollahi Z, Ertesvåg IS, Bolland O (2011) Optimized process configurations of post-combustion CO 2 capture for natural-gas-fired power plant—exergy analysis. Int J Greenh Gas Control 5(6):1393–1405. https://doi.org/10.1016/j.ijggc.2011.09.004
Article CAS Google Scholar
Apaiyakul R, Nimmanterdwong P, Kanchanakungvalkul T, Puapan P, Gao H, Liang Z, Tontiwachwuthikul P, Sema T (2022) Precipitation behavior, density, viscosity, and CO 2 absorption capacity of highly concentrated ternary AMP-PZ-MEA solvents. Int J Greenh Gas Control 120:103775. https://doi.org/10.1016/j.ijggc.2022.103775
Arnette AN (2017) Renewable energy and carbon capture and sequestration for a reduced carbon energy plan: an optimization model. Renew Sustain Energy Rev 70:254–265. https://doi.org/10.1016/j.rser.2016.11.218
Bahman N, Alalaiwat D, Abdulmohsen Z, Khalifa MA, Baharna SA, Al-Mannai MA, Younis A (2022) A critical review on global CO 2 emission: Where do industries stand? Rev Environ Health. https://doi.org/10.1515/reveh-2022-0105
Balraj A, Sekaran APC, Ramamurthy N, Babarao R, Nagarajan KK, Mayilvahanan SA (2022) Systematic review on sono-assisted CO2 stripping, solvent recovery and energy demand aspects in solvent-based post-combustion carbon dioxide capture process. Chem Eng Process Process Intensif 170:108723. https://doi.org/10.1016/j.cep.2021.108723
Barchas R, Davis R (1992) The Kerr-McGee/ABB Lummus Crest technology for the recovery of CO 2 from stack gases. Energy Convers Manag 33(5–8):333–340. https://doi.org/10.1016/0196-8904(92)90028-U
Bishnoi S, Rochelle GT (2000) Physical and chemical solubility of carbon dioxide in aqueous methyldiethanolamine. Fluid Phase Equilib 168(2):241–258. https://doi.org/10.1016/0196-8904(92)90028-U
Bravo J, Drapanauskaite D, Sarunac N, Romero C, Jesikiewicz T, Baltrusaitis J (2021) Optimization of energy requirements for CO 2 post-combustion capture process through advanced thermal integration. Fuel 283:118940. https://doi.org/10.1016/j.fuel.2020.118940
Brúder P, Grimstvedt A, Mejdell T, Svendsen HF (2011) CO 2 capture into aqueous solutions of piperazine activated 2-amino-2-methyl-1-propanol. Chem Eng Sci 66(23):6193–6198. https://doi.org/10.1016/j.ces.2011.08.051
Budzianowski WM (2015) Single solvents, solvent blends, and advanced solvent systems in CO 2 capture by absorption: a review. Int J Global Warm 7(2):184–225. https://doi.org/10.1504/IJGW.2015.067749
Bui M, Adjiman CS, Bardow A, Anthony EJ, Boston A, Brown S, Fennell PS, Fuss S, Galindo A, Hackett LA (2018) Carbon capture and storage (CCS): the way forward. Energy Environ Sci 11(5):1062–1176. https://doi.org/10.1039/C7EE02342A
Cao X, Zhang M, Mujumdar AS, Zhong Q, Wang Z (2018) Effects of ultrasonic pretreatments on quality, energy consumption and sterilization of barley grass in freeze drying. Ultrason Sonochem 40:333–340. https://doi.org/10.1016/j.ultsonch.2017.06.014
Carreira-Casais A, Otero P, Garcia-Perez P, Garcia-Oliveira P, Pereira AG, Carpena M, Soria-Lopez A, Simal-Gandara J, Prieto MA (2021) Benefits and drawbacks of ultrasound-assisted extraction for the recovery of bioactive compounds from marine algae. Int J Environ Res Public Health 18(17):9153
Chao C, Deng Y, Dewil R, Baeyens J, Fan X (2021) Post-combustion carbon capture. Renew Sustain Energy Rev 138:110490. https://doi.org/10.1016/j.rser.2020.110490
Chen Z, Deng S, Wei H, Wang B, Huang J, Yu G (2013) Activated carbons and amine-modified materials for carbon dioxide capture—a review. Front Environ Sci Eng 7(3):326–340. https://doi.org/10.1007/s11783-013-0510-7
Chen H, Mu Y, Hardacre C, Fan X (2020) Integration of membrane separation with nonthermal plasma catalysis: a proof-of-concept for CO 2 capture and utilization. Ind Eng Chem Res 59(17):8202–8211. https://doi.org/10.1021/acs.iecr.0c01067
Cheng C-Y, Kuo C-C, Yang M-W, Zhuang Z-Y, Lin P-W, Chen Y-F, Yang H-S, Chou C-T (2021) CO 2 Capture from flue gas of a coal-fired power plant using three-bed PSA process. Energies 14(12):3582
Clausse M, Merel J, Meunier F (2011) Numerical parametric study on CO2 capture by indirect thermal swing adsorption. Int J Greenh Gas Control 5(5):1206–1213. https://doi.org/10.1016/j.ijggc.2011.05.036
Cormos C-C (2012) Hydrogen and power co-generation based on coal and biomass/solid wastes co-gasification with carbon capture and storage. Int J Hydrog Energy 37(7):5637–5648. https://doi.org/10.1016/j.ijhydene.2011.12.132
Cormos A-M, Dinca C, Cormos C-C (2015) Multi-fuel multi-product operation of IGCC power plants with carbon capture and storage (CCS). Appl Thermal Eng 74:20–27. https://doi.org/10.1016/j.applthermaleng.2013.12.080
Croiset E, Thambimuthu K (2001) NOx and SO 2 emissions from O 2 /CO 2 recycle coal combustion. Fuel 80(14):2117–2121. https://doi.org/10.1016/S0016-2361(00)00197-6
Dai N, Shah AD, Hu L, Plewa MJ, McKague B, Mitch WA (2012) Measurement of nitrosamine and nitramine formation from NOx reactions with amines during amine-based carbon dioxide capture for postcombustion carbon sequestration. Environ Sci Technol 46(17):9793–9801. https://doi.org/10.1021/es301867b
Davis J, Rochelle G (2009) Thermal degradation of monoethanolamine at stripper conditions. Energy Procedia 1(1):327–333. https://doi.org/10.1016/j.egypro.2009.01.045
Davison J (2007) Performance and costs of power plants with capture and storage of CO 2 . Energy 32(7):1163–1176. https://doi.org/10.1016/j.energy.2006.07.039
Davoudi M, Safadoust AR, Mansoori SAA, Mottaghi HR (2014) The impurities effect on thermal degradation and corrosivity of amine solution in South Pars gas sweetening plants. J Nat Gas Sci Eng 19:116–124. https://doi.org/10.1016/j.jngse.2014.05.001
De Visser E, Hendriks C, Barrio M, Mølnvik MJ, de Koeijer G, Liljemark S, Le Gallo Y (2008) Dynamis CO 2 quality recommendations. Int J Greenh Gas Control 2(4):478–484. https://doi.org/10.1016/j.ijggc.2008.04.006
Deiana P, Bassano C, Calì G, Miraglia P, Maggio E (2017) CO 2 capture and amine solvent regeneration in Sotacarbo pilot plant. Fuel 207:663–670. https://doi.org/10.1016/j.fuel.2017.05.066
Derks PWJ, Kleingeld T, van Aken C, Hogendoorn JA, Versteeg GF (2006) Kinetics of absorption of carbon dioxide in aqueous piperazine solutions. Chem Eng Sci 61(20):6837–6854. https://doi.org/10.1016/j.ces.2006.07.009
Dinca C, Slavu N, Cormoş C-C, Badea A (2018) CO 2 capture from syngas generated by a biomass gasification power plant with chemical absorption process. Energy 149:925–936. https://doi.org/10.1016/j.energy.2018.02.109
Eide L, Bailey D (2005) Precombustion decarbonisation processes. Oil Gas Sci Technol 60(3):475–484. https://doi.org/10.2516/ogst:2005029
Falk-Pedersen O, Grønvold MS, Nøkleby P, Bjerve F, Svendsen HF (2005) CO 2 Capture with membrane contactors. Int J Green Energy 2(2):157–165. https://doi.org/10.1081/GE-200058965
Feron PH (2010) Exploring the potential for improvement of the energy performance of coal fired power plants with post-combustion capture of carbon dioxide. Int J Greenh Gas Control 4(2):152–160. https://doi.org/10.1016/j.ijggc.2009.10.018
Ferrara G, Lanzini A, Leone P, Ho M, Wiley D (2017) Exergetic and exergoeconomic analysis of post-combustion CO 2 capture using MEA-solvent chemical absorption. Energy 130:113–128. https://doi.org/10.1016/j.energy.2017.04.096
Figueroa JD, Fout T, Plasynski S, McIlvried H, Srivastava RD (2008) Advances in CO 2 capture technology—the US department of energy’s carbon sequestration program. Int J Greenh Gas Control 2(1):9–20. https://doi.org/10.1016/S1750-5836(07)00094-1
Freeman SA, Dugas R, Van Wagener DH, Nguyen T, Rochelle GT (2010) Carbon dioxide capture with concentrated, aqueous piperazine. Int J Greenh Gas Control 4(2):119–124. https://doi.org/10.1016/j.ijggc.2009.10.008
Frimpong RA, Nikolic H, Pelgen J, Ghorbanian M, Figueroa JD, Liu K (2019) Evaluation of different solvent performance in a 0.7 MWe pilot scale CO 2 capture unit. Chem Eng Res Des 148:11–20. https://doi.org/10.1016/j.cherd.2019.05.053
Fu C, Gundersen T (2012) Using exergy analysis to reduce power consumption in air separation units for oxy-combustion processes. Energy 44(1):60–68. https://doi.org/10.1016/j.energy.2012.01.065
Fu W, Wang L, Yang Y (2021) Optimal design for double reheat coal-fired power plants with post-combustion CO 2 capture: a novel thermal system integration with a carbon capture turbine. Energy 221:119838. https://doi.org/10.1016/j.energy.2021.119838
Gantert S, Möller D (2012) Ultrasonic desorption of CO 2 –a new technology to save energy and prevent solvent degradation. Chem Eng Technol 35(3):576–578. https://doi.org/10.1002/ceat.201100395
Goto K, Yogo K, Higashii T (2013) A review of efficiency penalty in a coal-fired power plant with post-combustion CO 2 capture. Appl Energy 111:710–720. https://doi.org/10.1016/j.apenergy.2013.05.020
Gür TM (2022) Carbon dioxide emissions, capture, storage and utilization: review of materials, processes and technologies. Progr Energy Combust Sci 89:100965. https://doi.org/10.1016/j.pecs.2021.100965
Haase S, Tolvanen P, Russo V (2022) Process intensification in chemical reaction engineering. Processes 10(1):99. https://doi.org/10.3390/pr10010099
Hanak DP, Biliyok C, Yeung H, Białecki R (2014) Heat integration and exergy analysis for a supercritical high-ash coal-fired power plant integrated with a post-combustion carbon capture process. Fuel 134:126–139. https://doi.org/10.1016/j.fuel.2014.05.036
Herzog H, Golomb D (2004) Carbon capture and storage from fossil fuel use. Encycl Energy 1(6562):277–287. https://doi.org/10.1016/B0-12-176480-X/00422-8
Hong WY (2022) A techno-economic review on carbon capture, utilisation and storage systems for achieving a net-zero CO 2 emissions future. Carbon Capture Sci Technol. https://doi.org/10.1016/j.ccst.2022.100044
Hosseini-Ardali SM, Hazrati-Kalbibaki M, Fattahi M, Lezsovits F (2020) Multi-objective optimization of post combustion CO2 capture using methyldiethanolamine (MDEA) and piperazine (PZ) bi-solvent. Energy 211:119035. https://doi.org/10.1016/j.energy.2020.119035
House KZ, Harvey CF, Aziz MJ, Schrag DP (2009) The energy penalty of post-combustion CO 2 capture and storage and its implications for retrofitting the US installed base. Energy Environ Sci 2(2):193–205. https://doi.org/10.1039/B811608C
Hwang J, Kim J, Lee HW, Na J, Ahn BS, Lee SD, Kim HS, Lee H, Lee U (2019) An experimental based optimization of a novel water lean amine solvent for post combustion CO 2 capture process. Appl Energy 248:174–184. https://doi.org/10.1016/j.apenergy.2019.04.135
IEA (2018) International Energy Agency: World energy outlook. http://www.iea.org/t&c/
IEA (2020) International Energy Agency (IEA), Data and statistics. https://www.iea.org/data-and-statisticscountry=WORLD&fuel=CO220emissions&indicator=CO2BySector
IPCC (2005) IPCC special report on carbon dioxide capture and storage (0521685516). https://www.ipcc.ch/report/carbon-dioxide-capture-and-storage/
IPCC (2018) The Context of Strengthening the Global Response to the Threat Climate Change, Sustainable Development, and Efforts to Eradicate Poverty (Global Warming of 1.5C. An IPCC Special Report on the Ompact of Global Warming of 1.5C above Pre-industrial Level and Related Global Greenhouse Gas Emission Pathways, Issue. https://www.ipcc.ch/sr15/
Ishibashi M, Ota H, Akutsu N, Umeda S, Tajika M, Izumi J, Yasutake A, Kabata T, Kageyama Y (1996) Technology for removing carbon dioxide from power plant flue gas by the physical adsorption method. Energy Convers Manag 37(6–8):929–933. https://doi.org/10.1016/0196-8904(95)00279-0
Ismail BB, Yusuf HL, Pu Y, Zhao H, Guo M, Liu D (2020) Ultrasound-assisted adsorption/desorption for the enrichment and purification of flavonoids from baobab ( Adansonia digitata ) fruit pulp. Ultrason Sonochem 65:104980. https://doi.org/10.1016/j.ultsonch.2020.104980
Jansen D, Gazzani M, Manzolini G, van Dijk E, Carbo M (2015) Pre-combustion CO 2 capture. Int J Greenh Gas Control 40:167–187. https://doi.org/10.1016/j.ijggc.2015.05.028
Jiang N, Shen Y, Liu B, Zhang D, Tang Z, Li G, Fu B (2020) CO 2 capture from dry flue gas by means of VPSA, TSA and TVSA. J CO2 Util 35:153–168. https://doi.org/10.1016/j.jcou.2019.09.012
Jiang Y, Mathias PM, Freeman CJ, Swisher JA, Zheng RF, Whyatt GA, Heldebrant DJ (2021) Techno-economic comparison of various process configurations for post-combustion carbon capture using a single-component water-lean solvent. Int J Greenh Gas Control 106:103279. https://doi.org/10.1016/j.ijggc.2021.103279
Kadam SU, Tiwari BK, O’Donnell CP (2013) Application of novel extraction technologies for bioactives from marine algae. J Agric Food Chem 61(20):4667–4675. https://doi.org/10.1021/jf400819p
Kearns D, Liu H, Consoli C (2021) Technology readiness and costs of CCS (Global CCS institute, Issue. https://www.globalccsinstitute.com/resources/publications-reports-research/technology-readiness-and-costs-of-ccs/
Kheirinik M, Ahmed S, Rahmanian N (2021) Comparative techno-economic analysis of carbon capture processes: pre-combustion, post-combustion, and oxy-fuel combustion operations. Sustainability 13(24):13567
Kikkinides ES, Yang R, Cho S (1993) Concentration and recovery of carbon dioxide from flue gas by pressure swing adsorption. Ind Eng Chem Res 32(11):2714–2720. https://doi.org/10.1021/ie00023a038
Kishimoto S, Hirata T, Iijima M, Ohishi T, Higaki K, Mitchell R (2009) Current status of MHI’s CO 2 recovery technology and optimization of CO 2 recovery plant with a PC fired power plant. Energy Procedia 1(1):1091–1098. https://doi.org/10.1016/j.egypro.2009.01.144
Kunze C, Spliethoff H (2012) Assessment of oxy-fuel, pre-and post-combustion-based carbon capture for future IGCC plants. Appl Energy 94:109–116. https://doi.org/10.1016/j.apenergy.2012.01.013
Kuramochi T, Ramírez A, Turkenburg W, Faaij A (2012) Comparative assessment of CO 2 capture technologies for carbon-intensive industrial processes. Progr Energy Combust Sci 38(1):87–112. https://doi.org/10.1016/j.pecs.2011.05.001
Leeson D, Mac Dowell N, Shah N, Petit C, Fennell PS (2017) A Techno-economic analysis and systematic review of carbon capture and storage (CCS) applied to the iron and steel, cement, oil refining and pulp and paper industries, as well as other high purity sources. Int J Greenh Gas Control 61:71–84. https://doi.org/10.1016/j.ijggc.2017.03.020
Leung DY, Caramanna G, Maroto-Valer MM (2014) An overview of current status of carbon dioxide capture and storage technologies. Renew Sustain Energy Rev 39:426–443. https://doi.org/10.1016/j.rser.2014.07.093
Li K, Cousins A, Yu H, Feron P, Tade M, Luo W, Chen J (2016) Systematic study of aqueous monoethanolamine-based CO 2 capture process: model development and process improvement. Energy Sci Eng 4(1):23–39. https://doi.org/10.1002/ese3.101
Li Y, You Y, Dai M, Chen X, Yang J (2020) Physical properties and CO2 absorption capacity of propylene carbonate+ poly (propylene glycol) monobutyl ether systems. J Chem Eng Data 65(2):896–905. https://doi.org/10.1021/acs.jced.9b01081
Liang ZH, Rongwong W, Liu H, Fu K, Gao H, Cao F, Zhang R, Sema T, Henni A, Sumon K (2015) Recent progress and new developments in post-combustion carbon-capture technology with amine based solvents. Int J Greenh Gas Control 40:26–54
Liang Z, Fu K, Idem R, Tontiwachwuthikul P (2016) Review on current advances, future challenges and consideration issues for post-combustion CO 2 capture using amine-based absorbents. Chinese J Chem Eng 24(2):278–288. https://doi.org/10.1016/j.cjche.2015.06.013
Liu J, Baeyens J, Deng Y, Tan T, Zhang H (2020) The chemical CO 2 capture by carbonation-decarbonation cycles. J Environ Manag 260:110054. https://doi.org/10.1016/j.jenvman.2019.110054
Luis P (2016) Use of monoethanolamine (MEA) for CO 2 capture in a global scenario: consequences and alternatives. Desalination 380:93–99. https://doi.org/10.1016/j.desal.2015.08.004
Ma Y, Liao Y, Su Y, Wang B, Yang Y, Ji D, Li H, Zhou H, Wang D (2021) Comparative investigation of different CO 2 capture technologies for coal to ethylene glycol process. Processes 9(2):207. https://doi.org/10.3390/pr9020207
Ma Q, Wang S, Fu Y, Zhou W, Shi M, Peng X, Lv H, Zhao W, Zhang X (2023) China’s policy framework for carbon capture, utilization and storage: review, analysis, and outlook. Front Energy. https://doi.org/10.1007/s11708-023-0862-z
Magee P (1971) Toxicity of nitrosamines: their possible human health hazards. Food Cosmet Toxicol 9(2):207–218. https://doi.org/10.1016/0015-6264(71)90306-3
Maniarasu R, Rathore SK, Murugan S (2021) A review on materials and processes for carbon dioxide separation and capture. Energy Environ. https://doi.org/10.1177/0958305X211050984
Merkel TC, Lin H, Wei X, Baker R (2010) Power plant post-combustion carbon dioxide capture: an opportunity for membranes. J Membr Sci 359(1–2):126–139. https://doi.org/10.1016/j.memsci.2009.10.041
Metz B, Davidson O, De Coninck H, Loos M, Meyer L (2005) IPCC special report on carbon dioxide capture and storage. Cambridge University Press, Cambridge
Google Scholar
Miller MB, Chen D-L, Luebke DR, Johnson JK, Enick RM (2011) Critical assessment of CO2 solubility in volatile solvents at 298.15 K. J Chem Eng Data 56(4):1565–1572. https://doi.org/10.1021/je101161d
Mofidipour E, Babaelahi M (2020) New procedure in solar system dynamic simulation, thermodynamic analysis, and multi-objective optimization of a post-combustion carbon dioxide capture coal-fired power plant. Energy Convers Manag 224:113321. https://doi.org/10.1016/j.enconman.2020.113321
Mondal MK, Balsora HK, Varshney P (2012) Progress and trends in CO 2 capture/separation technologies: a review. Energy 46(1):431–441. https://doi.org/10.1016/j.energy.2012.08.006
Mostafavi E, Ashrafi O, Navarri P (2021) Assessment of process modifications for amine-based post-combustion carbon capture processes. Clean Eng Technol 4:100249. https://doi.org/10.1016/j.clet.2021.100249
Mukherjee A, Okolie JA, Abdelrasoul A, Niu C, Dalai AK (2019) Review of post-combustion carbon dioxide capture technologies using activated carbon. J Environ Sci 83:46–63. https://doi.org/10.1016/j.jes.2019.03.014
Mumford KA, Wu Y, Smith KH, Stevens GW (2015) Review of solvent based carbon-dioxide capture technologies. Front Chem Sci Eng 9(2):125–141. https://doi.org/10.1007/s11705-015-1514-6
Norahim N, Yaisanga P, Faungnawakij K, Charinpanitkul T, Klaysom C (2018) Recent membrane developments for CO 2 separation and capture. Chem Eng Technol 41(2):211–223. https://doi.org/10.1002/ceat.201700406
Nwaoha C, Idem R, Supap T, Saiwan C, Tontiwachwuthikul P, Rongwong W, Al-Marri MJ, Benamor A (2017) Heat duty, heat of absorption, sensible heat and heat of vaporization of 2–Amino–2–Methyl–1–propanol (AMP), piperazine (PZ) and monoethanolamine (MEA) tri–solvent blend for carbon dioxide (CO 2 ) capture. Chem Eng Sci 170:26–35. https://doi.org/10.1016/j.ces.2017.03.025
Ochedi FO, Yu J, Yu H, Liu Y, Hussain A (2021) Carbon dioxide capture using liquid absorption methods: a review. Environ Chem Lett 19(1):77–109. https://doi.org/10.1007/s10311-020-01093-8
Oexmann J, Kather A (2009) Post-combustion CO 2 capture in coal-fired power plants: comparison of integrated chemical absorption processes with piperazine promoted potassium carbonate and MEA. Energy Procedia 1(1):799–806. https://doi.org/10.1016/j.egypro.2009.01.106
Oh S-Y, Yun S, Kim J-K (2018) Process integration and design for maximizing energy efficiency of a coal-fired power plant integrated with amine-based CO 2 capture process. Appl Energy 216:311–322. https://doi.org/10.1016/j.apenergy.2018.02.100
Oh H-T, Ju Y, Chung K, Lee C-H (2020) Techno-economic analysis of advanced stripper configurations for post-combustion CO 2 capture amine processes. Energy 206:118164. https://doi.org/10.1016/j.energy.2020.118164
Olajire AA (2010) CO 2 capture and separation technologies for end-of-pipe applications–a review. Energy 35(6):2610–2628. https://doi.org/10.1016/j.energy.2010.02.030
Osman AI, Hefny M, Abdel Maksoud MIA, Elgarahy AM, Rooney DW (2021) Recent advances in carbon capture storage and utilisation technologies: a review. Environ Chem Lett 19(2):797–849. https://doi.org/10.1007/s10311-020-01133-3
Otitoju O, Oko E, Wang M (2021) Technical and economic performance assessment of post-combustion carbon capture using piperazine for large scale natural gas combined cycle power plants through process simulation. Appl Energy 292:116893. https://doi.org/10.1016/j.apenergy.2021.116893
Pal P, AbuKashabeh A, Al-Asheh S, Banat F (2014) Accumulation of heat stable salts and degraded products during thermal degradation of aqueous methyldiethanolamine (MDEA) using microwave digester and high pressure reactor. J Nat Gas Sci Eng 21:1043–1047. https://doi.org/10.1016/j.jngse.2014.11.007
Pan M, Aziz F, Li B, Perry S, Zhang N, Bulatov I, Smith R (2016) Application of optimal design methodologies in retrofitting natural gas combined cycle power plants with CO 2 capture. Appl Energy 161:695–706. https://doi.org/10.1016/j.apenergy.2015.03.035
Paraschiv S, Paraschiv LS (2020) Trends of carbon dioxide (CO 2 ) emissions from fossil fuels combustion (coal, gas and oil) in the EU member states from 1960 to 2018. Energy Rep 6:237–242. https://doi.org/10.1016/j.egyr.2020.11.116
Pera-Titus M (2014) Porous inorganic membranes for CO 2 capture: present and prospects. Chem Rev 114(2):1413–1492. https://doi.org/10.1021/cr400237k
Pérez-Calvo J-F, Mazzotti M (2022) Techno-economic assessment of post-combustion CO 2 capture using aqueous piperazine at different flue gas compositions and flowrates via a general optimization methodology. Int J Greenh Gas Control 114:103587. https://doi.org/10.1016/j.ijggc.2022.103587
Pires J, Martins F, Alvim-Ferraz M, Simões M (2011) Recent developments on carbon capture and storage: an overview. Chem Eng Res Des 89(9):1446–1460. https://doi.org/10.1016/j.cherd.2011.01.028
Qian F, Chyang C-S, Chiou J-B, Tso J (2011) Effect of flue gas recirculation (FGR) on NOx emission in a pilot-scale vortexing fluidized-bed combustor. Energy Fuels 25(12):5639–5646. https://doi.org/10.1021/ef201394e
Rabensteiner M, Kinger G, Koller M, Gronald G, Hochenauer C (2015) Investigation of carbon dioxide capture with aqueous piperazine on a post combustion pilot plant—part II: parameter study and emission measurement. Int J Greenh Gas Control 37:471–480. https://doi.org/10.1016/j.ijggc.2015.04.011
Rao AD, Francuz DJ (2013) An evaluation of advanced combined cycles. Appl Energy 102:1178–1186. https://doi.org/10.1016/j.apenergy.2012.06.035
Rey A, Gouedard C, Ledirac N, Cohen M, Dugay J, Vial J, Pichon V, Bertomeu L, Picq D, Bontemps D (2013) Amine degradation in CO2 capture. 2. New degradation products of MEA. Pyrazine and alkylpyrazines: analysis, mechanism of formation and toxicity. Int J Greenh Gas Control 19:576–583. https://doi.org/10.1016/j.ijggc.2013.10.018
Rochelle GT (2012) Thermal degradation of amines for CO 2 capture. Curr Opin Chem Eng 1(2):183–190. https://doi.org/10.1016/j.coche.2012.02.004
Rochelle G, Chen E, Freeman S, Van Wagener D, Xu Q, Voice A (2011) Aqueous piperazine as the new standard for CO 2 capture technology. Chem Eng J 171(3):725–733. https://doi.org/10.1016/j.cej.2011.02.011
Romero-García AG, Mora-Morales C, Chargoy-Amador JP, Ramírez-Corona N, Sánchez-Ramírez E, Segovia-Hernández JG (2022) Implementing CO 2 capture process in power plants: optimization procedure and environmental impact. Chem Eng Res Des 180:232–242. https://doi.org/10.1016/j.cherd.2022.02.023
Rubin ES, Mantripragada H, Marks A, Versteeg P, Kitchin J (2012) The outlook for improved carbon capture technology. Progr Energy Combust Sci 38(5):630–671. https://doi.org/10.1016/j.pecs.2012.03.003
Rubin ES, Davison JE, Herzog HJ (2015) The cost of CO 2 capture and storage. Int J Greenh Gas Control 40:378–400. https://doi.org/10.1016/j.ijggc.2015.05.018
Sachde D, Rochelle GT (2014) Absorber intercooling configurations using aqueous piperazine for capture from sources with 4 to 27% CO 2 . Energy Procedia 63:1637–1656. https://doi.org/10.1016/j.egypro.2014.11.174
Scherffius JR, Reddy S, Klumpyan JP, Armpriester A (2013) Large-scale CO 2 capture demonstration plant using fluor’s econamine FG PlusSM technology at NRG’s WA parish electric generating station. Energy Procedia 37:6553–6561. https://doi.org/10.1016/j.egypro.2013.06.587
Shaw D (2009) Cansolv CO 2 capture: the value of integration. Energy Procedia 1(1):237–246. https://doi.org/10.1016/j.egypro.2009.01.034
Shaw R, Mukherjee S (2022) The development of carbon capture and storage (CCS) in India: a critical review. Carbon Capture Sci Technol. https://doi.org/10.1016/j.ccst.2022.100036
Siefert N, Agarwal S, Shi F, Shi W, Roth E, Hopkinson D, Kusuma V, Thompson R, Luebke D, Nulwala H (2016) Hydrophobic physical solvents for pre-combustion CO 2 capture: experiments, computational simulations, and techno-economic analysis. Int J Greenh Gas Control 49:364–371. https://doi.org/10.1016/j.ijggc.2016.03.014
Smith KH, Ashkanani HE, Morsi BI, Siefert NS (2022) Physical solvents and techno-economic analysis for pre-combustion CO 2 capture: a review. Int J Greenh Gas Control 118:103694. https://doi.org/10.1016/j.ijggc.2022.103694
Spigarelli BP, Kawatra SK (2013) Opportunities and challenges in carbon dioxide capture. J CO2 Util 1:69–87. https://doi.org/10.1016/j.jcou.2013.03.002
Stéphenne K (2014) Start-up of world’s first commercial post-combustion coal fired CCS project: contribution of Shell Cansolv to SaskPower Boundary Dam ICCS project. Energy Procedia 63:6106–6110. https://doi.org/10.1016/j.egypro.2014.11.642
Stöver B, Bergins C, Klebes J (2011) Optimized post combustion carbon capturing on coal fired power plants. Energy Procedia 4:1637–1643. https://doi.org/10.1016/j.egypro.2011.02.035
Su F, Lu C (2012) CO 2 capture from gas stream by zeolite 13X using a dual-column temperature/vacuum swing adsorption. Energy Environ Sci 5(10):9021–9027. https://doi.org/10.1039/C2EE22647B
Sultan H, Muhammad HA, Bhatti UH, Min GH, Baek IH, Baik Y-J, Nam SC (2021) Reducing the efficiency penalty of carbon dioxide capture and compression process in a natural gas combined cycle power plant by process modification and liquefied natural gas cold energy integration. Energy Convers Manage 244:114495
Sun W, Shao Y, Zhao L, Wang Q (2020) Co-removal of CO 2 and particulate matter from industrial flue gas by connecting an ammonia scrubber and a granular bed filter. J Clean Prod 257:120511. https://doi.org/10.1016/j.jclepro.2020.120511
Supap T, Saiwan C, Idem R, Tontiwachwuthikul PP (2011) Part 2: solvent management: solvent stability and amine degradation in CO 2 capture processes. Carbon Manag 2(5):551–566. https://doi.org/10.4155/cmt.11.55
Tan Y, Croiset E, Douglas MA, Thambimuthu KV (2006) Combustion characteristics of coal in a mixture of oxygen and recycled flue gas. Fuel 85(4):507–512. https://doi.org/10.1016/j.fuel.2005.08.010
Tiwari V, Garg A, Kapshe M, Deshpande A, Vishwanathan S (2023) Assessing possibilities for coal continuance in India under climate constraints. Int J Greenh Gas Control 122:103811. https://doi.org/10.1016/j.ijggc.2022.103811
Tlili N, Grévillot G, Vallières C (2009) Carbon dioxide capture and recovery by means of TSA and/or VSA. Int J Greenh Gas Control 3(5):519–527. https://doi.org/10.1016/j.ijggc.2009.04.005
Toftegaard MB, Brix J, Jensen PA, Glarborg P, Jensen AD (2010) Oxy-fuel combustion of solid fuels. Progr Energy Combust Sci 36(5):581–625. https://doi.org/10.1016/j.pecs.2010.02.001
Vega F, Camino S, Camino JA, Garrido J, Navarrete B (2019) Partial oxy-combustion technology for energy efficient CO 2 capture process. Appl Energy 253:113519. https://doi.org/10.1016/j.apenergy.2019.113519
Wall T, Stanger R, Santos S (2011) Demonstrations of coal-fired oxy-fuel technology for carbon capture and storage and issues with commercial deployment. Int J Greenh Gas Control 5:S5–S15. https://doi.org/10.1016/j.ijggc.2011.03.014
Walters MS, Edgar TF, Rochelle GT (2016) Dynamic modeling and control of an intercooled absorber for post-combustion CO 2 capture. Chem Eng Process Process Intensif 107:1–10. https://doi.org/10.1016/j.cep.2016.05.012
Wang X, Song C (2020) Carbon capture from flue gas and the atmosphere: a perspective. Front Energy Res 8:560849. https://doi.org/10.3389/fenrg.2020.560849
Wang M, Lawal A, Stephenson P, Sidders J, Ramshaw C (2011) Post-combustion CO 2 capture with chemical absorption: a state-of-the-art review. Chem Eng Res Des 89(9):1609–1624. https://doi.org/10.1016/j.cherd.2010.11.005
Wang L, Yang Y, Shen W, Kong X, Li P, Yu J, Rodrigues AE (2013) CO 2 capture from flue gas in an existing coal-fired power plant by two successive pilot-scale VPSA units. Ind Eng Chem Res 52(23):7947–7955. https://doi.org/10.1021/ie4009716
Wang M, Zhao J, Wang X, Liu A, Gleason KK (2017) Recent progress on submicron gas-selective polymeric membranes. J Mater Chem A 5(19):8860–8886. https://doi.org/10.1039/C7TA01862B
Wu F, Argyle MD, Dellenback PA, Fan M (2018) Progress in O2 separation for oxy-fuel combustion–a promising way for cost-effective CO2 capture: a review. Progr Energy Combust Sci 67:188–205. https://doi.org/10.1016/j.pecs.2018.01.004
Xu Y, Wang H, Liu X, Zhu J, Xu J, Xu M (2022) Mitigating CO 2 emission in pulverized coal-fired power plant via co-firing ammonia: a simulation study of flue gas streams and exergy efficiency. Energy Convers Manag 256:115328. https://doi.org/10.1016/j.enconman.2022.115328
Yadav S, Mondal S (2022) A review on the progress and prospects of oxy-fuel carbon capture and sequestration (CCS) technology. Fuel 308:122057. https://doi.org/10.1016/j.fuel.2021.122057
Yang H, Xu Z, Fan M, Gupta R, Slimane RB, Bland AE, Wright I (2008) Progress in carbon dioxide separation and capture: a review. J Environ Sci 20(1):14–27. https://doi.org/10.1016/S1001-0742(08)60002-9
Yao Y, Pan Y, Liu S (2020) Power ultrasound and its applications: a state-of-the-art review. Ultrason Sonochem 62:104722. https://doi.org/10.1016/j.ultsonch.2019.104722
Ying J, Eimer DA, Mathisen A, Brakstad F, Haugen HA (2019) Ultrasound intensify CO2 desorption from pressurized loaded monoethanolamine solutions. II. Optimization and cost estimation. Energy 173:218–228. https://doi.org/10.1016/j.energy.2019.02.070
Younas M, Sohail M, Leong L, Bashir M, Sumathi S (2016) Feasibility of CO 2 adsorption by solid adsorbents: a review on low-temperature systems. Int J Environ Sci Technol 13(7):1839–1860. https://doi.org/10.1007/s13762-016-1008-1
Yu C-H, Huang C-H, Tan C-S (2012) A review of CO 2 capture by absorption and adsorption. Aerosol Air Qual Res 12(5):745–769. https://doi.org/10.4209/aaqr.2012.05.0132
Yuan Z, Eden MR, Gani R (2016) Toward the development and deployment of large-scale carbon dioxide capture and conversion processes. Ind Eng Chem Res 55(12):3383–3419. https://doi.org/10.1021/acs.iecr.5b03277
Yun S, Oh S-Y, Kim J-K (2020) Techno-economic assessment of absorption-based CO2 capture process based on novel solvent for coal-fired power plant. Appl Energy 268:114933. https://doi.org/10.1016/j.apenergy.2020.114933
Zanco SE, Pérez-Calvo J-F, Gasós A, Cordiano B, Becattini V, Mazzotti M (2021) Postcombustion CO 2 capture: a comparative techno-economic assessment of three technologies using a solvent, an adsorbent, and a membrane. ACS Eng Au 1(1):50–72. https://doi.org/10.1021/acsengineeringau.1c00002
Zhang Z (2015) Techno-economic assessment of carbon capture and storage facilities coupled to coal-fired power plants. Energy Environ 26(6–7):1069–1080. https://doi.org/10.1260/0958-305X.26.6-7.1069
Zhang X, Zhang C-F, Qin S-J, Zheng Z-S (2001) A kinetics study on the absorption of carbon dioxide into a mixed aqueous solution of methyldiethanolamine and piperazine. Ind Eng Chem Res 40(17):3785–3791. https://doi.org/10.1021/ie000956i
Zhang J, Webley PA, Xiao P (2008) Effect of process parameters on power requirements of vacuum swing adsorption technology for CO2 capture from flue gas. Energy Convers Manag 49(2):346–356. https://doi.org/10.1016/j.enconman.2007.06.007
Zhang Z, Borhani TN, El-Naas MH (2018) Carbon capture Exergetic, energetic and environmental dimensions. In: Exergetic, energetic and environmental dimensions (pp 997–1016). Elsevier
Zhao L, Riensche E, Blum L, Stolten D (2010) Multi-stage gas separation membrane processes used in post-combustion capture: energetic and economic analyses. J Membr Sci 359(1–2):160–172. https://doi.org/10.1016/j.memsci.2010.02.003
Zhao S, Feron PH, Deng L, Favre E, Chabanon E, Yan S, Hou J, Chen V, Qi H (2016) Status and progress of membrane contactors in post-combustion carbon capture: a state-of-the-art review of new developments. J Membr Sci 511:180–206. https://doi.org/10.1016/j.memsci.2016.03.051
Zhao B, Liu F, Cui Z, Liu C, Yue H, Tang S, Liu Y, Lu H, Liang B (2017) Enhancing the energetic efficiency of MDEA/PZ-based CO 2 capture technology for a 650 MW power plant: process improvement. Appl Energy 185:362–375. https://doi.org/10.1016/j.apenergy.2016.11.009
Zheng L, Landon J, Zou W, Liu K (2014) Corrosion benefits of piperazine as an alternative CO 2 capture solvent. Ind Eng Chem Res 53(29):11740–11746. https://doi.org/10.1021/ie501346z
Zhuang Q, Clements B, Li B (2017) Emerging new types of absorbents for Postcombustion carbon capture. Recent Adv Carbon Capture Storage. https://doi.org/10.5772/65739
Download references
Acknowledgements
The authors are thankful to Dr. Soumyajit Mukherjee, IIT, Bombay and an anonymous reviewer for their constructive criticism and fruitful suggestions which helped us to improve the manuscript. This project receives no funding from any source to be acknowledged.
Author information
Authors and affiliations.
Environment and Sustainable Development Program, College of Science, University of Bahrain, Main Campus Sakhir, P.O. Box 32038, Zallaq, Kingdom of Bahrain
Dalal Alalaiwat & Ezzat Khan
Department of Mathematics and Science, College of Engineering, University of Technology Bahrain, P.O. Box 18041, Salmabad, Kingdom of Bahrain
Dalal Alalaiwat
Department of Chemistry, University of Malakand, Chakdara, Lower Dir, 18800, Khyber Pakhtunkhwa, Pakistan
You can also search for this author in PubMed Google Scholar
Contributions
DA: Conceptualization, Writing—original draft, Methodology, Writing—review and editing. EK: Conceptualization, Supervision, Writing—review and editing.
Corresponding author
Correspondence to Ezzat Khan .
Ethics declarations
Conflict of interest.
The authors declare that they have no known competing financial interests or personal relationships that could have appeared to influence the work reported in this paper.
Additional information
Publisher's note.
Springer Nature remains neutral with regard to jurisdictional claims in published maps and institutional affiliations.
Rights and permissions
Springer Nature or its licensor (e.g. a society or other partner) holds exclusive rights to this article under a publishing agreement with the author(s) or other rightsholder(s); author self-archiving of the accepted manuscript version of this article is solely governed by the terms of such publishing agreement and applicable law.
Reprints and permissions
About this article
Alalaiwat, D., Khan, E. Current status and future scenarios of carbon capture from power plants emission: a review. Rev Environ Sci Biotechnol 22 , 799–822 (2023). https://doi.org/10.1007/s11157-023-09663-2
Download citation
Received : 25 March 2023
Accepted : 19 June 2023
Published : 01 July 2023
Issue Date : September 2023
DOI : https://doi.org/10.1007/s11157-023-09663-2
Share this article
Anyone you share the following link with will be able to read this content:
Sorry, a shareable link is not currently available for this article.
Provided by the Springer Nature SharedIt content-sharing initiative
- Pre-combustion capture
- Post-combustion capture
- Oxyfuel-combustion capture
- Regeneration energy
- Synergistic effect
- Find a journal
- Publish with us
- Track your research
Accessibility Links
- Skip to content
- Skip to search IOPscience
- Skip to Journals list
- Accessibility help
- Accessibility Help
Click here to close this panel.
Purpose-led Publishing is a coalition of three not-for-profit publishers in the field of physical sciences: AIP Publishing, the American Physical Society and IOP Publishing.
Together, as publishers that will always put purpose above profit, we have defined a set of industry standards that underpin high-quality, ethical scholarly communications.
We are proudly declaring that science is our only shareholder.
Sustainability of hydropower as source of renewable and clean energy
J Luis 1 , L M Sidek 2 , M N M Desa 3 and P Y Julien 4
Published under licence by IOP Publishing Ltd IOP Conference Series: Earth and Environmental Science , Volume 16 , 4th International Conference on Energy and Environment 2013 (ICEE 2013) 5–6 March 2013, Putrajaya, Malaysia Citation J Luis et al 2013 IOP Conf. Ser.: Earth Environ. Sci. 16 012050 DOI 10.1088/1755-1315/16/1/012050
Article metrics
8968 Total downloads
Share this article
Author affiliations.
1 Post Graduate Student, UNITEN, Jalan IKRAM-UNITEN, 43000 Kajang, Selangor, Malaysia
2 Centre for Sustainable Technology and Environment, UNITEN, Jalan IKRAM-UNITEN, 43000 Kajang, Selangor, Malaysia
3 Centre for Stormwater and Geohazard Management, UNITEN, Jalan IKRAM-UNITEN, 43000 Kajang, Selangor, Malaysia
4 Department of Civil Engineering, Colorado State University, Fort Collins, USA
Buy this article in print
Hydroelectric energy has been in recent times placed as an important future source of renewable and clean energy. The advantage of hydropower as a renewable energy is that it produces negligible amounts of greenhouse gases, it stores large amounts of electricity at low cost and it can be adjusted to meet consumer demand. This noble vision however is becoming more challenging due to rapid urbanization development and increasing human activities surrounding the catchment area. Numerous studies have shown that there are several contributing factors that lead towards the loss of live storage in reservoir, namely geology, ground slopes, climate, drainage density and human activities. Sediment deposition in the reservoir particularly for hydroelectric purposes has several major concerns due to the reduced water storage volume which includes increase in the risk of flooding downstream which directly effects the safety of human population and properties, contributes to economic losses not only in revenue for power generation but also large capital and maintenance cost for reservoir restorations works. In the event of functional loss of capabilities of a hydropower reservoir as a result of sedimentation or siltation could lead to both economical and environmental impact. The objective of this paper is aimed present the importance of hydropower as a source of renewable and clean energy in the national energy mix and the increasing challenges of sustainability.
Export citation and abstract BibTeX RIS
Content from this work may be used under the terms of the Creative Commons Attribution 3.0 licence . Any further distribution of this work must maintain attribution to the author(s) and the title of the work, journal citation and DOI.

IMAGES
COMMENTS
This study investigates the role and impact of artificial intelligence (AI) in the electric power industry through a thematic analysis of corporate communications. As AI technologies proliferate, industries—such as the electric power industry—are undergoing significant transformations. The research problem addressed in this study involves understanding how electric power companies perceive ...
This unique power plant was part of a national research and development project to remotely connect energy assets in real time using the Department of Energy's (DOE's) Energy Sciences Network (ESnet). By linking capabilities at the National Renewable Energy Laboratory (NREL) and the Idaho National Laboratory (INL), the researchers created a ...
The stability of smart grids is crucial for ensuring reliable and efficient power distribution in modern energy systems. This paper presents an optimized Long Short-Term Memory model for predicting smart grid stability, leveraging the Novel Guide-Waterwheel Plant Algorithm (Guide-WWPA) for enhanced performance.
Diablo Canyon, the only operational nuclear power plant left in California, is seeking to extend operations past its scheduled decommissioning in 2025. (George Rose/Getty Images) A majority of U.S. adults remain supportive of expanding nuclear power in the country, according to a Pew Research Center survey from May. Overall, 56% say they favor ...
Virtual power plants represent the most immediate future of electricity generation, as they allow for intelligent consumption of energy in a distributed environment through the optimal management of demand and power generation. ... As a result, research papers incorporate this capacity to participate in the day-ahead electricity market in their ...
Fig. 3 showed an increase of 2.9% in global energy demand in 2018. This is the strongest growth since the year 2010, almost doubling the 10-year average. There was a significant increase in the demand for all sources of energy; however, gas witnesses the strongest increase in demand, presenting an estimated demand of 168 million tons (about 43 % of the total increase in global demand).
A Virtual Power Plant (VPP) is a practical concept that aggregates various Renewable Energy Sources (RESs) to improve energy management efficiency and facilitate energy trading. ... have been overlooked in both research papers. In [69], [70], a day-ahead scheduling program has been investigated to maximize the profit considering reserve power.
Plant efficiency is calculated when these sophisticated controllers are used. NLC Tamilnadu Power Ltd (NTPL), a 500 MW capacity plant has operating efficiency of 37.2% is given in Table 2.From Table 2, Load variation is achieved in NTPL plant in the order of 5 MW.As per the plant design, time to achieve 5 MW change was found to be 60sec when the conventional controller was used.
This review concentrated on a simple cycle GT power plant (GTPP) with a 2-shaft, regenerative, reheat, intercooler, complex cycle GT with effect intercooler, regenerative and reheat.
The power generation during summer monsoon is higher than usual; the western coast of India has higher capacity than eastern coast (15.5 to 19.3 kW/m). In the study it has been found that on the contrary, the power generation in the studied locations is lower than the hot zones (1.8 to 7.6 kW/m). The wave power potential in India as shown in ...
Energy Science & Engineering is a sustainable energy journal publishing high-impact fundamental and applied research that will help secure an ... thermal energy is harnessed employing concentrated solar power (CSP) plants such as Linear Fresnel collectors and parabolic trough collectors. In this paper, solar thermal technologies including soar ...
Finally, research trends in the development of solar power plants are presented. The credibility of the Photovoltaic system, types and limitations is the discussion under study system makes use of sun's energy to generate electricity with the help of varied procedural systems; stand-alone, hybrid or grid charged.
The data of the power plant were obtained for a period of 8 years (2010-2017) from CEGCO annual reports 20,21.Throughout the studied period, several major malfunctions occurred to the power plant.
The world's electricity generation has increased with renewable energy technologies such as solar (solar power plant), wind energy (wind turbines), heat energy, and even ocean waves. Iran is in the best condition to receive solar radiation due to its proximity to the equator (25.2969° N). In 2020, Iran was able to supply only 900 MW (about 480 solar power plants and 420 MW home solar power ...
than 95% of global module production (Fraunhofer ISE 2019 ). Monocrystalline solar cells have the highest ef ciency rates, typically 15-20%. but the highest quality panels can reach up to 23% ef ...
The focus of this research is on energy output, with 1-year 100MW-QASP power plant data being examined for the EE. In this paper, it has been studied the different curves of power generation, irradiation curve and PR based on 4 quarterly datasheets of FY (2020-2021).
Abstract. More than hundred million tons of coal fly ash is produced annually in India from combustion of coal in power plants. It is expected that about 150 million tons of coal ash will be produced due to burning of coal in power plants by the year of 2015. This will require about 30,000 hectare of land for the disposal of ash.
The object of study in this paper is a combined power plant. The initial design was that of a traditional CCP-220T combined-cycle plant with a dual-circuit steam turbine cycle. As a promising design, we have considered a combined plant based on GTE-160 using carbon dioxide recovery Brayton cycles with recompression and base version .
The use of AI algorithms in power plants has been under continual investigation since the advent of the expert system technique . However, in most situations, issues such as long cycle times, complex computation and difficulty in learning arise with classic AI methods. ... Selection criteria for shortlisted research papers.
A thermal power plant is a facility where heat energy is converted into electrical energy. This is achieved by transforming heat or steam into mechanical energy, which is then utilized to generate electricity. b. Energy Conversion Process: Most thermal power plants rely on steam or heat as the initial source of energy. This heat energy
This research paper investigated machine learning algorithms utilised for energy efficiency improvement at coal-fired thermal power plants by conducting a systematic literature review. Subsequently, this paper provided an overview of the identified relevant studies, focusing on the type of machine learning algorithms and the application thereof.
Explore the latest full-text research PDFs, articles, conference papers, preprints and more on POWER PLANTS. Find methods information, sources, references or conduct a literature review on POWER ...
The power grid is undergoing a transformation from synchronous generators (SGs) toward inverter-based resources (IBRs). The stochasticity, asynchronicity, and limited-inertia characteristics of IBRs bring about challenges to grid resilience. Virtual power plants (VPPs) are emerging technologies to improve the grid resilience and advance the transformation. By judiciously aggregating ...
These interactions between grid operator, Virtual Power Plants operator and distributed energy resources are complex both in terms of contractual agreements and provision of services. On all layers, accurate tracking of distributed energy resource usage and execution of contracts is required for establishing trust between transacting participants.
The emission from power plants highly contributes to the increase of CO2 concentration in the atmosphere. Enhancing the utilization of renewable energy and improving energy efficiency are widely considered to be a key to reduce emissions, however, certain solutions need time to be implemented. Carbon Capture and Storage is considered as a crucial solution for the situation that contributes to ...
technology, hydropower provides 16,4% of global electricity production. [7] As to the energy. storage the storage hydropower (including pumpe d storage, PS) represents between 96 - 99%. of the ...
Abstract. Hydroelectric energy has been in recent times placed as an important future source of renewable and clean energy. The advantage of hydropower as a renewable energy is that it produces negligible amounts of greenhouse gases, it stores large amounts of electricity at low cost and it can be adjusted to meet consumer demand.