
Follow along with the video below to see how to install our site as a web app on your home screen.
Note: This feature may not be available in some browsers.
- Learning English
- Ask a Teacher

[Grammar] After many years of research, they found the solution at last/ at the end.
- Thread starter wotcha
- Start date Aug 27, 2012
- Views : 30,992

Senior Member
- Aug 27, 2012

wotcha said: My grammar book says the answer to the above sentence is ' at last ' and I know both 'at last' and 'at the end' are same in meaning. Click to expand...

charliedeut
Hi Wotcha, Maybe you were thinking of "in the end"? charliedeut
Similar threads
- Jun 12, 2024
- Jun 5, 2024
- Editing & Writing Topics

- Coffee Break
- Sep 19, 2023
- hardyweineberg
- May 28, 2023

- Mar 12, 2023

If you have a question about the English language and would like to ask one of our many English teachers and language experts, please click the button below to let us know:
Share this page
share this!
August 8, 2024
This article has been reviewed according to Science X's editorial process and policies . Editors have highlighted the following attributes while ensuring the content's credibility:
fact-checked
trusted source
written by researcher(s)
Multiple goals, multiple solutions, plenty of second-guessing and revising—here's how science really works
by Soazig Le Bihan, The Conversation

A man in a lab coat bends under a dim light, his strained eyes riveted onto a microscope. He's powered only by caffeine and anticipation.
This solitary scientist will stay on task until he unveils the truth about the cause of the dangerous disease quickly spreading through his vulnerable city. Time is short, the stakes are high, and only he can save everyone.…
That kind of romanticized picture of science was standard for a long time. But it's as far from actual scientific practice as a movie's choreographed martial arts battle is from a real fistfight.
For most of the 20th century, philosophers of science like me maintained somewhat idealistic claims about what good science looks like. Over the past few decades, however, many of us have revised our views to better mirror actual scientific practice .
An update on what to expect from actual science is overdue. I often worry that when the public holds science to unrealistic standards, any scientific claim failing to live up to them arouses suspicion. While public trust is globally strong and has been for decades, it has been eroding. In November 2023, Americans' trust in scientists was 14 points lower than it had been just prior to the COVID-19 pandemic, with its flurry of confusing and sometimes contradictory science-related messages.
When people's expectations are not met about how science works, they may blame scientists. But modifying our expectations might be more useful. Here are three updates I think can help people better understand how science actually works. Hopefully, a better understanding of actual scientific practice will also shore up people's trust in the process.
The many faces of scientific research
First, science is a complex endeavor involving multiple goals and associated activities.
Some scientists search for the causes underlying some observable effect, such as a decimated pine forest or the Earth's global surface temperature increase .
Others may investigate the what rather than the why of things. For example, ecologists build models to estimate gray wolf abundance in Montana . Spotting predators is incredibly challenging. Counting all of them is impractical. Abundance models are neither complete nor 100% accurate—they offer estimates deemed good enough to set harvesting quotas. Perfect scientific models are just not in the cards .
Beyond the what and the why, scientists may focus on the how. For instance, the lives of people living with chronic illnesses can be improved by research on strategies for managing disease —to mitigate symptoms and improve function, even if the true causes of their disorders largely elude current medicine.
It's understandable that some patients may grow frustrated or distrustful of medical providers unable to give clear answers about what causes their ailment. But it's important to grasp that lots of scientific research focuses on how to effectively intervene in the world to reach some specific goals.
Simplistic views represent science as solely focused on providing causal explanations for the various phenomena we observe in this world. The truth is that scientists tackle all kinds of problems, which are best solved using different strategies and approaches and only sometimes involve full-fledged explanations.
Complex problems call for complex solutions
The second aspect of scientific practice worth underscoring is that, because scientists tackle complex problems, they don't typically offer one unique, complete and perfect answer. Instead they consider multiple, partial and possibly conflicting solutions.
Scientific modeling strategies illustrate this point well. Scientific models typically are partial, simplified and sometimes deliberately unrealistic representations of a system of interest. Models can be physical, conceptual or mathematical. The critical point is that they represent target systems in ways that are useful in particular contexts of inquiry. Interestingly, considering multiple possible models is often the best strategy to tackle complex problems.
Scientists consider multiple models of biodiversity , atomic nuclei or climate change . Returning to wolf abundance estimates, multiple models can also fit the bill. Such models rely on various types of data, including acoustic surveys of wolf howls, genetic methods that use fecal samples from wolves, wolf sightings and photographic evidence, aerial surveys, snow track surveys and more.
Weighing the pros and cons of various possible solutions to the problem of interest is part and parcel of the scientific process. Interestingly, in some cases, using multiple conflicting models allows for better predictions than trying to unify all the models into one.
The public may be surprised and possibly suspicious when scientists push forward multiple models that rely on conflicting assumptions and make different predictions. People often think "real science" should provide definite, complete and foolproof answers to their questions. But given various limitations and the world's complexity, keeping multiple perspectives in play is most often the best way for scientists to reach their goals and solve the problems at hand.
Science as a collective, contrarian endeavor
Finally, science is a collective endeavor, where healthy disagreement is a feature, not a bug.
The romanticized version of science pictures scientists working in isolation and establishing absolute truths. Instead, science is a social and contrarian process in which the community's scrutiny ensures we have the best available knowledge. "Best available" does not mean "definitive," but the best we have until we find out how to improve it. Science almost always allows for disagreements among experts.
Controversies are core to how science works at its best and are as old as Western science itself. In the 1600s, Descartes and Leibniz fought over how to best characterize the laws of dynamics and the nature of motion.
The long history of atomism provides a valuable perspective on how science is an intricate and winding process rather than a fast-delivery system of results set in stone. As Jean Baptiste Perrin conducted his 1908 experiments that seemingly settled all discussion regarding the existence of atoms and molecules, the questions of the atom's properties were about to become the topic of decades of controversies with the birth of quantum physics.
The nature and structure of fundamental particles and associated fields have been the subject of scientific research for more than a century. Lively academic discussions abound concerning the difficult interpretation of quantum mechanics , the challenging unification of quantum physics and relativity , and the existence of the Higgs boson , among others.
Distrusting researchers for having healthy scientific disagreements is largely misguided.
A very human practice
To be clear, science is dysfunctional in some respects and contexts. Current institutions have incentives for counterproductive practices, including maximizing publication numbers . Like any human endeavor, science includes people with bad intent, including some trying to discredit legitimate scientific research . Finally, science is sometimes inappropriately influenced by various values in problematic ways.
These are all important considerations when evaluating the trustworthiness of particular scientific claims and recommendations. However, it is unfair, sometimes dangerous, to mistrust science for doing what it does at its best. Science is a multifaceted endeavor focused on solving complex problems that typically just don't have simple solutions. Communities of experts scrutinize those solutions in hopes of providing the best available approach to tackling the problems of interest.
Science is also a fallible and collective process. Ignoring the realities of that process and holding science up to unrealistic standards may result in the public calling science out and losing trust in its reliability for the wrong reasons.
Provided by The Conversation
Explore further
Feedback to editors

Nearly 25% of European landscape could be rewilded, say researchers
4 minutes ago

Wildly divergent skinks provide a window into how evolution works
21 minutes ago

Astronomers identify more than one thousand new star cluster candidates
34 minutes ago

New research expands our understanding of how electrons move in complex fluids

Skin cell discovery could help Atlantic salmon fend off sea lice
35 minutes ago

Method to separate microplastics from water could also speed up blood analyses
50 minutes ago

Enhanced two-photon microscopy method could reveal insights into neural dynamics and neurological diseases

Implementing a new pathway to measure and value biodiversity

Surprise finding in study of environmental bacteria could advance search for better antibiotics

Cactus dreams: Revealing the secrets of mescaline making
2 hours ago
Relevant PhysicsForums posts
Cover songs versus the original track, which ones are better.
9 hours ago
Why are ABBA so popular?
11 hours ago
Biographies, history, personal accounts
Aug 13, 2024
Is "applausive" implied terminology?
"trolling" in new england, for ww2 buffs.
More from Art, Music, History, and Linguistics
Related Stories

Researchers describe how philosophers can bridge the gap between science and policy
May 16, 2024

To accelerate biosphere science, researchers say reconnect three scientific cultures
Apr 23, 2024

Why some people don't trust science—and how to change their minds
Dec 31, 2023

A means for searching for new solutions in mathematics and computer science using an LLM and an evaluator
Dec 15, 2023

How much trust do people have in different types of scientists?
Apr 25, 2024

Study finds American trust in scientific expertise survived polarization and previous administration's attack on science
Mar 8, 2024
Recommended for you

Singing from memory unlocks a surprisingly common musical superpower

Study suggests five-second break can diffuse an argument between coupled partners
23 hours ago

Findings suggest empowering women is key to both sustainable energy and gender justice

Exploring the evolution of social norms with a supercomputer
Aug 9, 2024

Study shows people associate kindness with religious belief

Research demonstrates genetically diverse crowds are wiser
Aug 8, 2024
Let us know if there is a problem with our content
Use this form if you have come across a typo, inaccuracy or would like to send an edit request for the content on this page. For general inquiries, please use our contact form . For general feedback, use the public comments section below (please adhere to guidelines ).
Please select the most appropriate category to facilitate processing of your request
Thank you for taking time to provide your feedback to the editors.
Your feedback is important to us. However, we do not guarantee individual replies due to the high volume of messages.
E-mail the story
Your email address is used only to let the recipient know who sent the email. Neither your address nor the recipient's address will be used for any other purpose. The information you enter will appear in your e-mail message and is not retained by Phys.org in any form.
Newsletter sign up
Get weekly and/or daily updates delivered to your inbox. You can unsubscribe at any time and we'll never share your details to third parties.
More information Privacy policy
Donate and enjoy an ad-free experience
We keep our content available to everyone. Consider supporting Science X's mission by getting a premium account.
E-mail newsletter
Defining the Problem to Find the Solution
“Given one hour to save the world, I would spend 55 minutes defining the problem and 5 minutes finding the solution.” ~ Albert Einstein
When Judith Rodin took over as president of The Rockefeller Foundation in 2005, she had a vision to re-imagine how the Foundation understood and intervened in the pressing challenges that confronted a 21st-century world. This transformation would require the Foundation to re-think its entire strategy model, from the issues it focused on, to the process of finding, testing, and funding solutions.
To do so, under the care of managing director Claudia Juech , who joined in 2007, the Foundation developed a highly strategic, analytical search process appropriately, and fondly, referred to as “Search.” In 2012–13, Juech’s strategic research team led 11 topic searches, which involved coordinating internal staff, consultants, and nearly 200 outside experts.
But the process is not as important as its outputs, and whether or not the Foundation decided to deploy funding into a particular space, the research yielded insights that could benefit the entire social impact field. And that’s exactly what you’ll find in the pages to follow and the additional resources offered online at rockefellerfoundation.org/insights .
In a conversation with Insights , Juech talks of how searches are framed, why the process is unique, and the team’s guiding motto.
Every search is framed around an identified set of “problem spaces.” How does The Rockefeller Foundation define a problem space?
CJ: Albert Einstein, a Rockefeller Foundation grantee, said “given one hour to save the world, I would spend 55 minutes defining the problem and 5 minutes finding the solution.”
This approach is deeply embedded into our process: Before we can solve a problem, we need to know exactly what the problem is, and we should put a good amount of thinking and resources into understanding it. And because today’s problems are so complex, we know they can’t be solved by being broken down into specific components.
“Before we can solve a problem, we need to know exactly what the problem is, and we should put a good amount of thinking and resources into understanding it. “
And so, “problem space” is just a fancy phrase for the framework through which we study a particular challenge, which includes a number of interlinking and underlying issues that must be addressed in order to find a solution. For example, if our central problem is food waste and spoilage, we must also think about farmers’ limited access to finance and reliable buyers for their crops—those are part of the problem space.
We identify problem spaces based on a wide range of inputs, using broad, sweeping horizon-scanning activities, alongside secondary research, expert interviews, and the work Rockefeller has done to date. Through this process, we often find connections and inter-relations among several trends that surface across problem spaces.
How do you assess the potential for innovation in a particular problem space?
CJ: There is very little research that has focused on how to measure innovation potential in a sector with regard to a problem space, so we are really entering new territory here. We have adopted approaches that assess innovation potential at a national level or at an organizational level, but will support further research in the future to refine our frameworks. For now, we are looking at the enabling environment—is there evidence of cross-pollination, e.g., are ideas being shared or replicated across actors, sectors, or geographies? What’s the innovation capacity? Are there active change-makers around or organizations that are capable of testing or scaling innovations? And what’s the institutional environment? Are there legal, policy, or business structures in place that promote or hinder innovation in this area?
How does this process differ from or improve upon what was done before?
CJ: For years, philanthropy has dedicated an enormous amount of resources to evaluating the impact of specific grants after the fact. What we’re trying to do with Search is to make sure the same rigor and critical thought is applied to our strategies from the outset. We also need to be innovative in where we go for information so that we are getting the freshest information—to make sure we are getting the thing that is emerging in the moment and that may lead to significant progress.
What are some other ways in which the Foundation’s process is unique?
CJ: From my experience, one of the most important distinctions is that we don’t start out with a pre-determined point of view or a dominant idea of an answer to a problem. In our process, we investigate an issue and truly test it. Then we come back, and more times than not, we find there’s not the opportunity for us there to achieve impact as major as there may be with another issue that is being reviewed at the same time.
Obviously, the Foundation cannot commit funding to every problem area you research. What kind of criteria does The Rockefeller Foundation consider when you choose where to focus your interventions?
CJ: Ultimately, the Foundation tries to determine whether there is potential for us to have significant impact at scale on a problem that is critical for poor or vulnerable populations or the ecosystems that they depend on. So it is a combination of three different criteria: We want to make sure that we work on problems integral to the lives of poor populations; we want to understand how the specific engagement and investment by The Rockefeller Foundation would make a significant difference; and we are trying to get a sense of whether the context is conducive to transformative change, i.e., is there innovative activity or momentum in regulation or private sector activity that could be catalyzed in order to achieve significant impact.
So while some options don’t move forward, it doesn’t mean they aren’t worthwhile or pressing—it simply means it isn’t the right area in which the Foundation should intervene at this time. But the process is valuable in other ways—in many instances, the findings have contributed to ongoing work or even informed future searches. And we have a robust body of research that we can share widely to help other foundations or other actors who care about social impact to make decisions about their own strategies, or perhaps to illuminate an area they hadn’t considered funding before.
What insight or insights surprised you most from the first rounds of searches?
CJ: The future trajectory of many of the problem spaces that we investigated truly requires a call to action, especially as some of these don’t yet receive the level of attention they deserve. For example, unhealthy food markets and the health implications of different diets will be severe, particularly in Africa.
Many professions have aspirational mottos that are supposed to guide them in their work. The famous one for physicians, for example, is “First, do no harm.” What would be the motto you would hang over your office door?
CJ: Well, the physician motto would be a good one, but I think that for the work we are engaged in it would be “Give a clear-eyed view.” We want to provide decision-making intelligence that is as informed and as objective as possible so that the most good can come from a range of funding sources.
- philanthropy
- strategic philanthropy
Leave a comment
Every print subscription comes with full digital access
Science News
We’ve covered science for 100 years. here’s how it has — and hasn’t — changed.
There’s more detail and sophistication, but some of the questions remain the same

By Tom Siegfried
Contributing Correspondent
April 2, 2021 at 6:00 am

A century ago, articles in the precursor to Science News frequently focused on astronomy and space, exploring such issues as whether other planets existed beyond Neptune.
SCIEPRO/Science Photo Library/Getty Images
Share this:
A century ago, people needed help to understand science. Much as they do today.
Then as now, it wasn’t always easy to sort the accurate from the erroneous. Mainstream media, then as now, regarded science as secondary to other aspects of their mission. And when science made the news, it was often (then as now) garbled, naïve or dangerously misleading.
E.W. Scripps, a prominent newspaper publisher, and William Emerson Ritter, a biologist, perceived a need. They envisioned a service that would provide reliable news about science to the world, dedicated to truth and clarity. For Scripps and Ritter, science journalism had a noble purpose: “To discover the truth about all sorts of things of human concern, and to report it truthfully and in language comprehensible to those whose welfare is involved.”
And so Science Service was born, 100 years ago — soon to give birth to the magazine now known as Science News .
In its first year of existence, Science Service delivered its weekly dispatches to newspapers in the form of mimeographed packets. By 1922 those packets became available to the public by subscription, giving birth to Science News-Letter , the progenitor of Science News . Then as now, the magazine’s readers feasted on a smorgasbord of delicious tidbits from a menu encompassing all flavors of science — from the atom to outer space, from agriculture to oceanography, from transportation to, of course, food and nutrition.
In those early days, much of the new enterprise’s coverage focused on space, such as the possibility of planets beyond Neptune. Experts shared their views on whether spiral-shaped clouds in deep space were far-off entire galaxies of stars , like the Milky Way, or embryonic solar systems just now forming within the Milky Way. Articles explored the latest speculation about life on Venus ( here and here ) or on Mars .
Regular coverage was also devoted to new technologies — particularly radio. One Science Service dispatch informed readers on how to make their own home radio set — for $6 . And in 1922 Science News-Letter reported on an astounding radio breakthrough: a set that could operate without a battery . You could just plug it in to an electrical outlet.

To celebrate our upcoming 100th anniversary, we’ve launched a series that highlights some of the biggest advances in science over the last century. Visit our Century of Science site to see the series as it unfolds.
Much of the century’s scientific future was presaged in those early reports. In May 1921, an article on recent subatomic experiments noted the “dream of scientist and novelist alike that man would one day learn how … to utilize the vast stores of energy inside of atoms .” In 1922 Science Service editor Edwin Slosson speculated that the “smallest unit of positive electricity” (the proton) might “be a complex of many positive and negative particles ,” a dim but prescient preview of the existence of quarks.
True, some prognostications did not age so well. A 1921 prediction that the United States would be forced to adopt the metric system for commercial transactions is still awaiting fulfillment. A simple, common, international auxiliary language — “confidently predicted” in 1921 to become “a part of every educated person’s equipment” — remains unestablished today. And despite serious considerations of calendar reform by astronomers and church dignitaries reported in May 1922, well over 1,000 of the same old months have since passed without the slightest alteration.
On the other hand, “the favorite fruit of Americans of the generations to follow us will be the avocado,” as predicted in 1921, is possibly arguable, though there was no mention of toast — just the suggestion that “a few crackers and an avocado sprinkled with a little salt make a hearty and well-balanced lunch.”
One happily false prognostication was the repeated forecast of the rise of eugenics as a “scientific” endeavor.
“The organization of an artificial selection is only a question of time. It will be possible to renew as a whole, in a few centuries, all humanity, and to replace the mass by another much superior mass,” a “distinguished authority on anthropo-sociology” declared in a Science Service news item from 1921 . Another eugenicist proclaimed that “Eugenic Science” should be applied to “shed the light of reason on the primeval instinct of reproduction,” so that “disgenic marriages” would be banned just as bigamy and incest are.
In the century since, thanks to saner and more sophisticated knowledge of genetics (and more social enlightenment in general), eugenics has been disavowed by science and is now revived in spirit only by the ignorant or malevolent. And during that time, real science has progressed to an elevated degree of sophistication in many other ways, to an extent almost unimaginable to the scientists and journalists of the 1920s.
It turns out that the past century’s groundbreaking experimental discoveries, revolutionary theoretical revelations and prescient speculations have not eliminated science’s familiarity with false starts, unfortunate missteps and shortsighted prejudices.
When Science Service (now Society for Science) launched its mission, astronomers were unaware of the extent of the universe. No biologist knew what DNA did, or how brain chemistry regulated behavior. Geologists saw that Earth’s continents looked like separated puzzle pieces, but declared that to be a coincidence.
Modern scientists know better. Scientists now understand a lot more about the details of the atom’s interior, the molecules of life, the intricacies of the brain , the innards of the Earth and the expanse of the cosmos .
Yet somehow scientists still pursue the same questions, if now on higher levels of theoretical abstraction rooted in deeper layers of empirical evidence. We know how the molecules of life work, but not always how they react to novel diseases. We know how the brain works, except for those afflicted by dementia or depression (or when consciousness is part of the question). We know a lot about how the Earth works, but not enough to always foresee how it will respond to what humans are doing to it. We think we know a lot about the universe, but we’re not sure if ours is the only one, and we can’t explain how gravity, the dominant force across the cosmos, can coexist with the forces governing atoms.
It turns out that the past century’s groundbreaking experimental discoveries, revolutionary theoretical revelations and prescient speculations have not eliminated science’s familiarity with false starts, unfortunate missteps and shortsighted prejudices. Researchers today have expanded the scope of the reality they can explore, yet still stumble through the remaining uncharted jungles of nature’s facts and laws, seeking further clues to how the world works.
To paraphrase an old philosophy joke, science is more like it is today than it ever has been. In other words, science remains as challenging as ever to human inquiry. And the need to communicate its progress, perceived by Scripps and Ritter a century ago, remains as essential now as then.

Trustworthy journalism comes at a price.
Scientists and journalists share a core belief in questioning, observing and verifying to reach the truth. Science News reports on crucial research and discovery across science disciplines. We need your financial support to make it happen – every contribution makes a difference.
More Stories from Science News on Science & Society

Astronauts actually get stuck in space all the time

Scientists are getting serious about UFOs. Here’s why

‘Then I Am Myself the World’ ponders what it means to be conscious

Twisters asks if you can 'tame' a tornado. We have the answer

The world has water problems. This book has solutions

Does social status shape height?

In ‘Warming Up,’ the sports world’s newest opponent is climate change

‘After 1177 B.C.’ describes how societies fared when the Bronze Age ended
Subscribers, enter your e-mail address for full access to the Science News archives and digital editions.
Not a subscriber? Become one now .
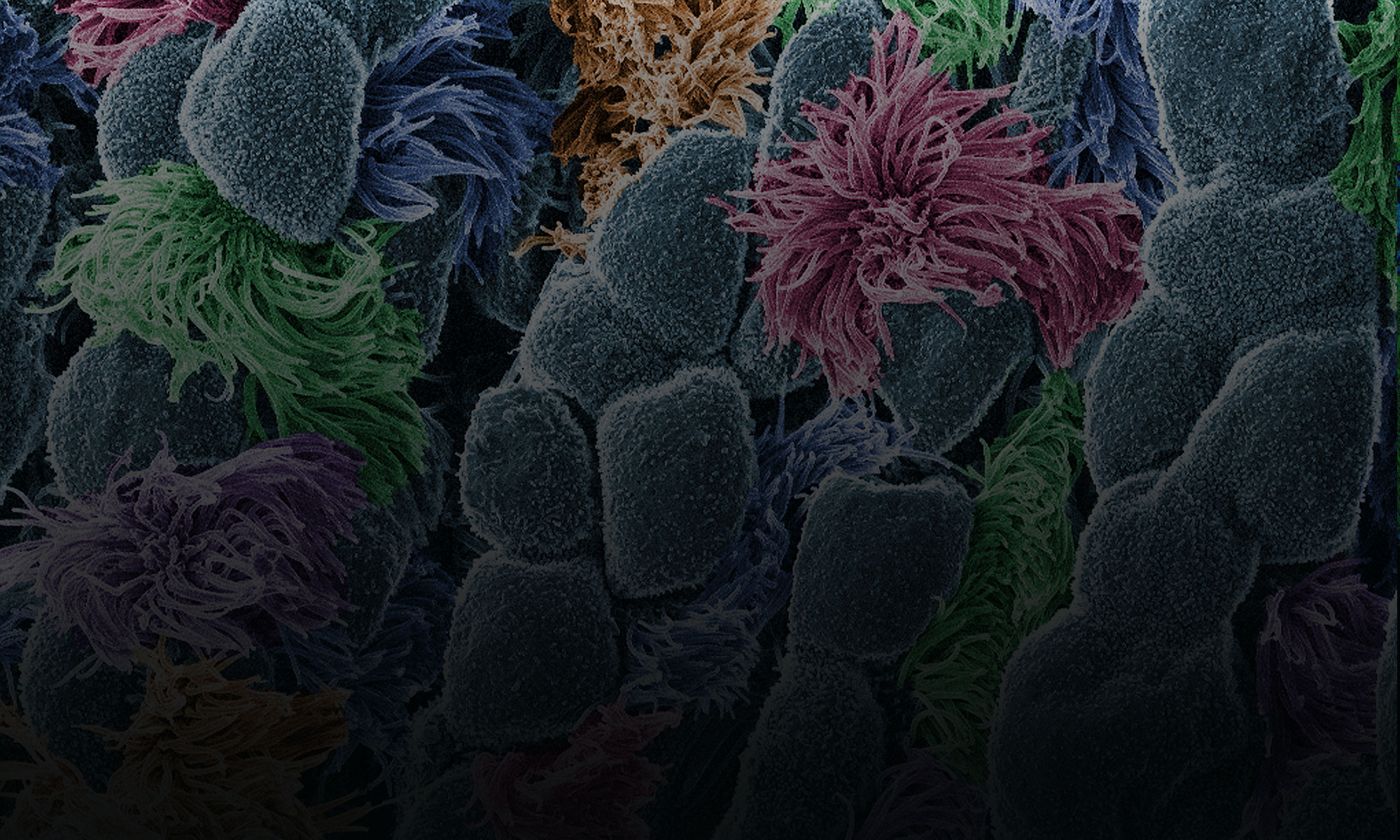
Biomedical Beat Blog – National Institute of General Medical Sciences
Follow the process of discovery
Search this blog
How research works: understanding the process of science.
Have you ever wondered how research works? How scientists make discoveries about our health and the world around us? Whether they’re studying plants, animals, humans, or something else in our world, they follow the scientific method. But this method isn’t always—or even usually—a straight line, and often the answers are unexpected and lead to more questions. Let’s dive in to see how it all works.
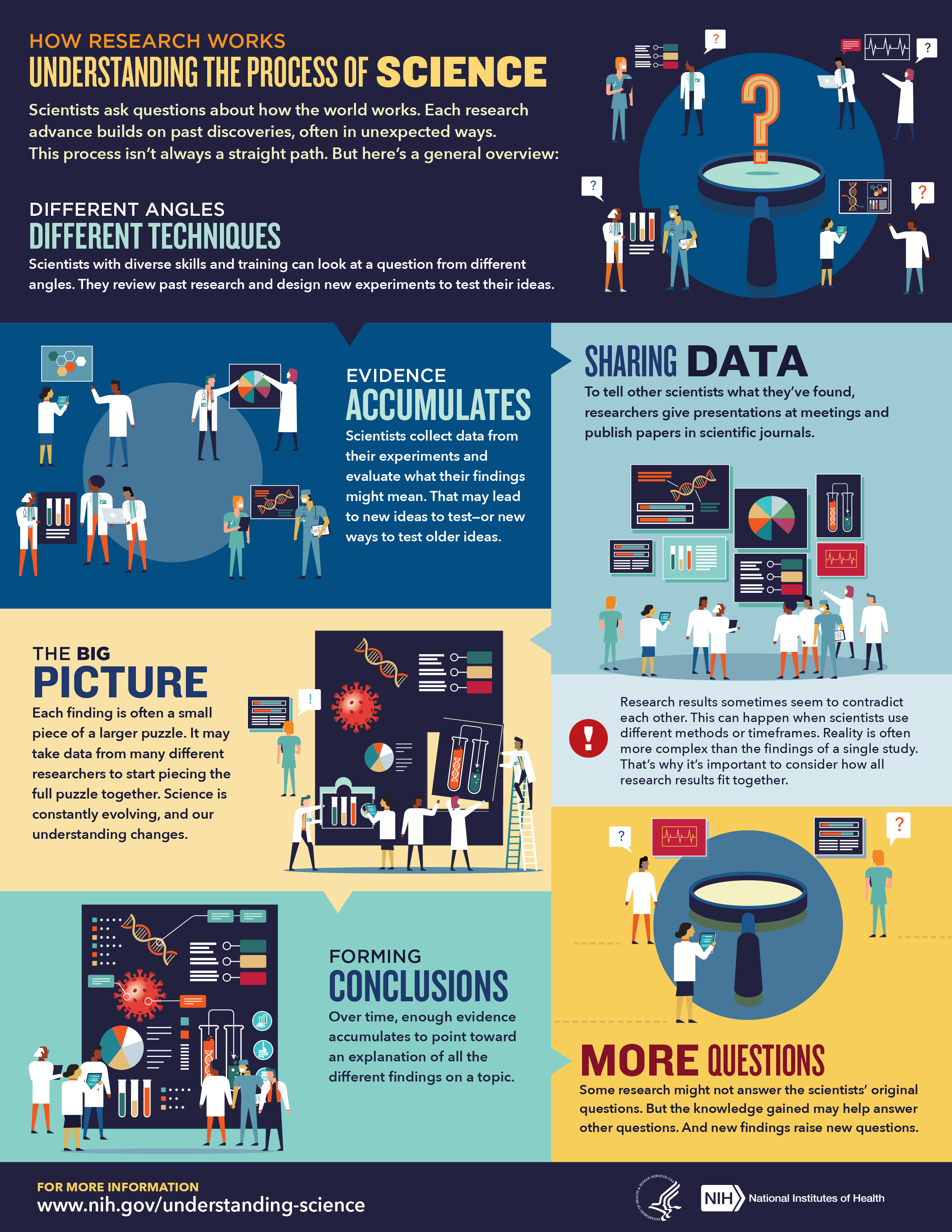
The Question Scientists start with a question about something they observe in the world. They develop a hypothesis, which is a testable prediction of what the answer to their question will be. Often their predictions turn out to be correct, but sometimes searching for the answer leads to unexpected outcomes.
The Techniques To test their hypotheses, scientists conduct experiments. They use many different tools and techniques, and sometimes they need to invent a new tool to fully answer their question. They may also work with one or more scientists with different areas of expertise to approach the question from other angles and get a more complete answer to their question.
The Evidence Throughout their experiments, scientists collect and analyze their data. They reach conclusions based on those analyses and determine whether their results match the predictions from their hypothesis. Often these conclusions trigger new questions and new hypotheses to test.
Researchers share their findings with one another by publishing papers in scientific journals and giving presentations at meetings. Data sharing is very important for the scientific field, and although some results may seem insignificant, each finding is often a small piece of a larger puzzle. That small piece may spark a new question and ultimately lead to new findings.
Sometimes research results seem to contradict each other, but this doesn’t necessarily mean that the results are wrong. Instead, it often means that the researchers used different tools, methods, or timeframes to obtain their results. The results of a single study are usually unable to fully explain the complex systems in the world around us. We must consider how results from many research studies fit together. This perspective gives us a more complete picture of what’s really happening.
Even if the scientific process doesn’t answer the original question, the knowledge gained may help provide other answers that lead to new hypotheses and discoveries.
Learn more about the importance of communicating how this process works in the NIH News in Health article, “ Explaining How Research Works .”
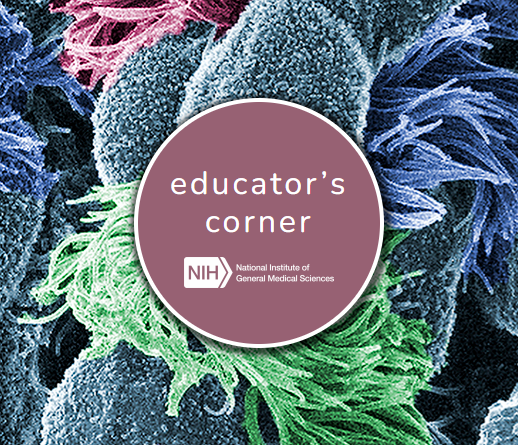
This post is a great supplement to Pathways: The Basic Science Careers Issue.
Pathways introduces the important role that scientists play in understanding the world around us, and all scientists use the scientific method as they make discoveries—which is explained in this post.
Learn more in our Educator’s Corner .
2 Replies to “How Research Works: Understanding the Process of Science”
Nice basic explanation. I believe informing the lay public on how science works, how parts of the body interact, etc. is a worthwhile endeavor. You all Rock! Now, we need to spread the word ‼️❗️‼️ Maybe eith a unique app. And one day, with VR and incentives to read & answer a couple questions.
As you know, the importance of an informed population is what will keep democracy alive. Plus it will improve peoples overall wellness & life outcomes.
Thanks for this clear explanation for the person who does not know science. Without getting too technical or advanced, it might be helpful to follow your explanation of replication with a reference to meta-analysis. You might say something as simple as, “Meta-analysis is a method for doing research on all the best research; meta-analytic research confirms the overall trend in results, even when the best studies show different results.”
Comments are closed.
Subscribe to Biomedical Beat
Get our latest blog posts delivered straight to your inbox! Sign Up Here
Featured Topics
Featured series.
A series of random questions answered by Harvard experts.
Explore the Gazette
Read the latest, should kids play wordle.
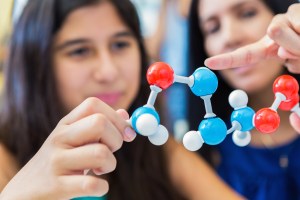
How moms may be affecting STEM gender gap

The answer to your search may depend on where you live
How did life begin on earth a lightning strike of an idea..
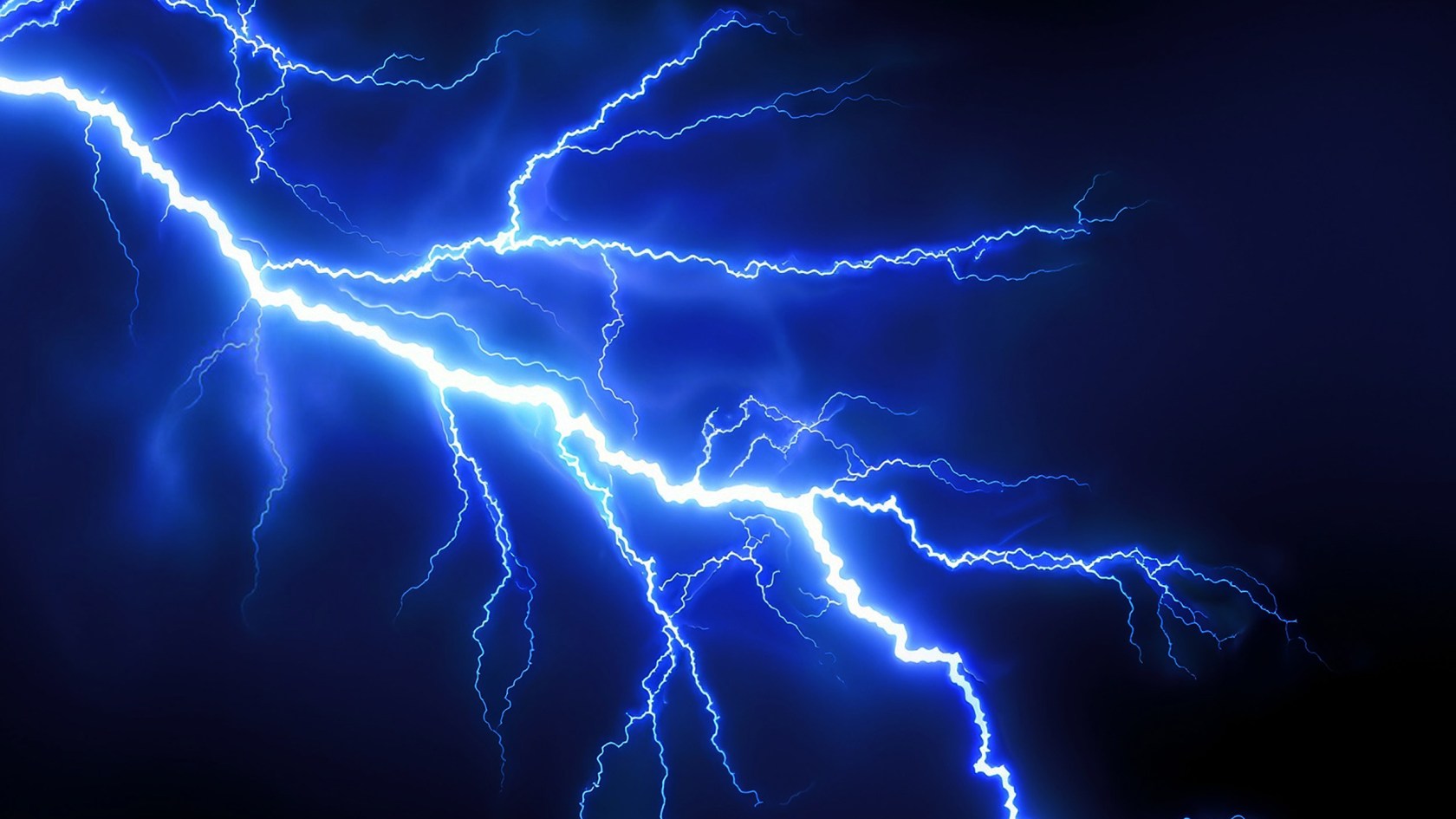
Yahya Chaudhry
Harvard Correspondent
Researchers mimic early conditions on barren planet to test hypothesis of ancient electrochemistry
About four billion years ago, Earth resembled the set of a summer sci-fi blockbuster. The planet’s surface was a harsh and barren landscape, recovering from hellish asteroid strikes, teeming with volcanic eruptions, and lacking enough nutrients to sustain even the simplest forms of life.
The atmosphere was composed predominantly of inert gases like nitrogen and carbon dioxide, meaning they did not easily engage in chemical reactions necessary to form the complex organic molecules that are the building blocks of life. Scientists have long sought to discover the key factors that enabled the planet’s chemistry to change enough to form and sustain life.
Now, new research zeroes in on how lightning strikes may have served as a vital spark, transforming the atmosphere of early Earth into a hotbed of chemical activity. In the study, published in Proceedings of the National Academy of Sciences , a team of Harvard scientists identified lightning-induced plasma electrochemistry as a potential source of reactive carbon and nitrogen compounds necessary for the emergence and survival of early life.
“The origin of life is one of the great unanswered questions facing chemistry,” said George M. Whitesides, senior author and the Woodford L. and Ann A. Flowers University Research Professor in the Department of Chemistry and Chemical Biology. How the fundamental building blocks of “nucleic acids, proteins, and metabolites emerged spontaneously remains unanswered.”
One of the most popular answers to this question is summarized in the so-called RNA World hypothesis, Whitesides said. That is the idea that available forms of the elements, such as water, soluble electrolytes, and common gases, formed the first biomolecules. In their study, the researchers found that lightning could provide accessible forms of nitrogen and carbon that led to the emergence and survival of biomolecules.
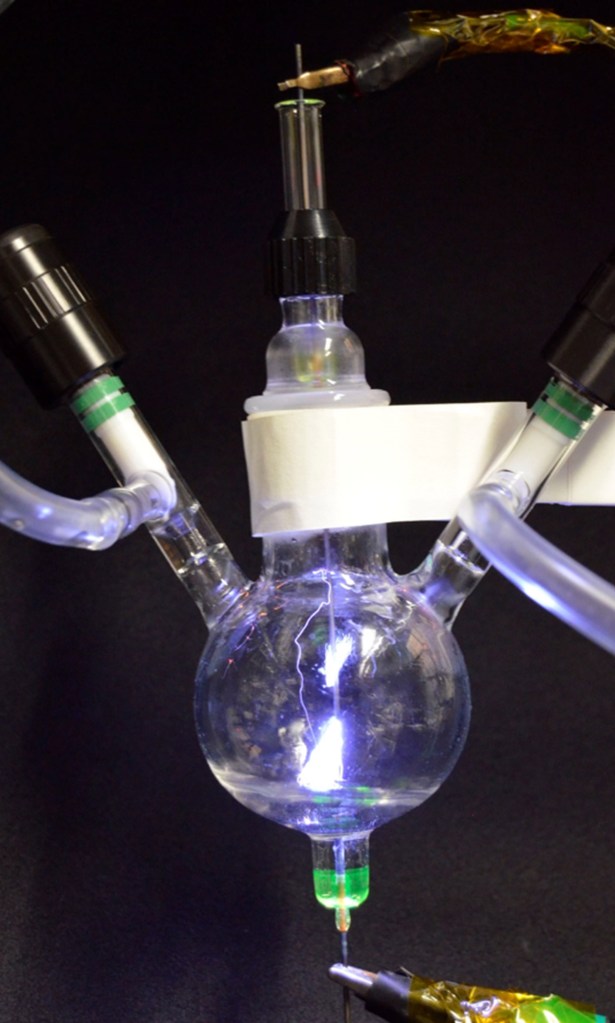
A plasma vessel used to mimic cloud-to-ground lightning and its resulting electrochemical reactions. The setup uses two electrodes, with one in the gas phase and the other submerged in water enriched with inorganic salts.
Credit: Haihui Joy Jiang
Researchers designed a plasma electrochemical setup that allowed them to mimic conditions of the early Earth and study the role lightning strikes might have had on its chemistry. They were able to generate high-energy sparks between gas and liquid phases — akin to the cloud-to-ground lightning strikes that would have been common billions of years ago.
The scientists discovered that their simulated lightning strikes could transform stable gases like carbon dioxide and nitrogen into highly reactive compounds. They found that carbon dioxide could be reduced to carbon monoxide and formic acid, while nitrogen could be converted into nitrate, nitrite, and ammonium ions.
These reactions occurred most efficiently at the interfaces between gas, liquid, and solid phases — regions where lightning strikes would naturally concentrate these products. This suggests that lightning strikes could have locally generated high concentrations of these vital molecules, providing diverse raw materials for the earliest forms of life to develop and thrive.
“Given what we’ve shown about interfacial lightning strikes, we are introducing different subsets of molecules, different concentrations, and different plausible pathways to life in the origin of life community,” said Thomas C. Underwood, co-lead author and Whitesides Lab postdoctoral fellow. “As opposed to saying that there’s one mechanism to create chemically reactive molecules and one key intermediate, we suggest that there is likely more than one reactive molecule that might have contributed to the pathway to life.”
The findings align with previous research suggesting that other energy sources, such as ultraviolet radiation, deep-sea vents, volcanoes, and asteroid impacts, could have also contributed to the formation of biologically relevant molecules. However, the unique advantage of cloud-to-ground lightning is its ability to drive high-voltage electrochemistry across different interfaces, connecting the atmosphere, oceans, and land.
The research adds a significant piece to the puzzle of life’s origins. By demonstrating how lightning could have contributed to the availability of essential nutrients, the study opens new avenues for understanding the chemical pathways that led to the emergence of life on Earth. As the research team continues to explore these reactions, they hope to uncover more about the early conditions that made life possible and to improve modern applications.
“Building on our work, we are now experimentally looking at how plasma electrochemical reactions may influence nitrogen isotopes in products, which has a potential geological relevance,” said co-lead author Haihui Joy Jiang, a former Whitesides lab postdoctoral fellow. “We are also interested in this research from an energy-efficiency and environmentally friendly perspective on chemical production. We are studying plasma as a tool to develop new methods of making chemicals and to drive green chemical processes, such as producing fertilizer used today.”
Harvard co-authors included Professor Dimitar D. Sasselov in the Department of Astronomy and Professor James G. Anderson in the Department of Chemistry and Chemical Biology, Department of Earth and Planetary Sciences, and the Harvard John A. Paulson School of Engineering and Applied Sciences.
The study not only sheds light on the past but also has implications for the search for life on other planets. Processes the researchers described could potentially contribute to the emergence of life beyond Earth.
“Lightning has been observed on Jupiter and Saturn; plasmas and plasma-induced chemistry can exist beyond our solar system,” Jiang said. “Moving forward, our setup is useful for mimicking environmental conditions of different planets, as well as exploring reaction pathways triggered by lightning and its analogs.”
Share this article
You might like.
Early childhood development expert has news for parents who think the popular online game will turn their children into super readers

Research suggests encouragement toward humanities appears to be very influential for daughters

Researchers find ‘language bias’ in various site algorithms, raising concerns about fallout for social divisions among nations
Garber to serve as president through 2026-27 academic year
Search for successor will launch in 2026
Finding right mix on campus speech policies
Legal, political scholars discuss balancing personal safety, constitutional rights, academic freedom amid roiling protests, cultural shifts
Good genes are nice, but joy is better
Harvard study, almost 80 years old, has proved that embracing community helps us live longer, and be happier

An official website of the United States government
The .gov means it’s official. Federal government websites often end in .gov or .mil. Before sharing sensitive information, make sure you’re on a federal government site.
The site is secure. The https:// ensures that you are connecting to the official website and that any information you provide is encrypted and transmitted securely.
- Publications
- Account settings
Preview improvements coming to the PMC website in October 2024. Learn More or Try it out now .
- Advanced Search
- Journal List

Identifying problems and solutions in scientific text
Kevin heffernan.
Department of Computer Science and Technology, University of Cambridge, 15 JJ Thomson Avenue, Cambridge, CB3 0FD UK
Simone Teufel
Research is often described as a problem-solving activity, and as a result, descriptions of problems and solutions are an essential part of the scientific discourse used to describe research activity. We present an automatic classifier that, given a phrase that may or may not be a description of a scientific problem or a solution, makes a binary decision about problemhood and solutionhood of that phrase. We recast the problem as a supervised machine learning problem, define a set of 15 features correlated with the target categories and use several machine learning algorithms on this task. We also create our own corpus of 2000 positive and negative examples of problems and solutions. We find that we can distinguish problems from non-problems with an accuracy of 82.3%, and solutions from non-solutions with an accuracy of 79.7%. Our three most helpful features for the task are syntactic information (POS tags), document and word embeddings.
Introduction
Problem solving is generally regarded as the most important cognitive activity in everyday and professional contexts (Jonassen 2000 ). Many studies on formalising the cognitive process behind problem-solving exist, for instance (Chandrasekaran 1983 ). Jordan ( 1980 ) argues that we all share knowledge of the thought/action problem-solution process involved in real life, and so our writings will often reflect this order. There is general agreement amongst theorists that state that the nature of the research process can be viewed as a problem-solving activity (Strübing 2007 ; Van Dijk 1980 ; Hutchins 1977 ; Grimes 1975 ).
One of the best-documented problem-solving patterns was established by Winter ( 1968 ). Winter analysed thousands of examples of technical texts, and noted that these texts can largely be described in terms of a four-part pattern consisting of Situation, Problem, Solution and Evaluation. This is very similar to the pattern described by Van Dijk ( 1980 ), which consists of Introduction-Theory, Problem-Experiment-Comment and Conclusion. The difference is that in Winter’s view, a solution only becomes a solution after it has been evaluated positively. Hoey changes Winter’s pattern by introducing the concept of Response in place of Solution (Hoey 2001 ). This seems to describe the situation in science better, where evaluation is mandatory for research solutions to be accepted by the community. In Hoey’s pattern, the Situation (which is generally treated as optional) provides background information; the Problem describes an issue which requires attention; the Response provides a way to deal with the issue, and the Evaluation assesses how effective the response is.
An example of this pattern in the context of the Goldilocks story can be seen in Fig. 1 . In this text, there is a preamble providing the setting of the story (i.e. Goldilocks is lost in the woods), which is called the Situation in Hoey’s system. A Problem in encountered when Goldilocks becomes hungry. Her first Response is to try the porridge in big bear’s bowl, but she gives this a negative Evaluation (“too hot!”) and so the pattern returns to the Problem. This continues in a cyclic fashion until the Problem is finally resolved by Goldilocks giving a particular Response a positive Evaluation of baby bear’s porridge (“it’s just right”).

Example of problem-solving pattern when applied to the Goldilocks story.
Reproduced with permission from Hoey ( 2001 )
It would be attractive to detect problem and solution statements automatically in text. This holds true both from a theoretical and a practical viewpoint. Theoretically, we know that sentiment detection is related to problem-solving activity, because of the perception that “bad” situations are transformed into “better” ones via problem-solving. The exact mechanism of how this can be detected would advance the state of the art in text understanding. In terms of linguistic realisation, problem and solution statements come in many variants and reformulations, often in the form of positive or negated statements about the conditions, results and causes of problem–solution pairs. Detecting and interpreting those would give us a reasonably objective manner to test a system’s understanding capacity. Practically, being able to detect any mention of a problem is a first step towards detecting a paper’s specific research goal. Being able to do this has been a goal for scientific information retrieval for some time, and if successful, it would improve the effectiveness of scientific search immensely. Detecting problem and solution statements of papers would also enable us to compare similar papers and eventually even lead to automatic generation of review articles in a field.
There has been some computational effort on the task of identifying problem-solving patterns in text. However, most of the prior work has not gone beyond the usage of keyword analysis and some simple contextual examination of the pattern. Flowerdew ( 2008 ) presents a corpus-based analysis of lexio-grammatical patterns for problem and solution clauses using articles from professional and student reports. Problem and solution keywords were used to search their corpora, and each occurrence was analysed to determine grammatical usage of the keyword. More interestingly, the causal category associated with each keyword in their context was also analysed. For example, Reason–Result or Means-Purpose were common causal categories found to be associated with problem keywords.
The goal of the work by Scott ( 2001 ) was to determine words which are semantically similar to problem and solution, and to determine how these words are used to signal problem-solution patterns. However, their corpus-based analysis used articles from the Guardian newspaper. Since the domain of newspaper text is very different from that of scientific text, we decided not to consider those keywords associated with problem-solving patterns for use in our work.
Instead of a keyword-based approach, Charles ( 2011 ) used discourse markers to examine how the problem-solution pattern was signalled in text. In particular, they examined how adverbials associated with a result such as “thus, therefore, then, hence” are used to signal a problem-solving pattern.
Problem solving also has been studied in the framework of discourse theories such as Rhetorical Structure Theory (Mann and Thompson 1988 ) and Argumentative Zoning (Teufel et al. 2000 ). Problem- and solutionhood constitute two of the original 23 relations in RST (Mann and Thompson 1988 ). While we concentrate solely on this aspect, RST is a general theory of discourse structure which covers many intentional and informational relations. The relationship to Argumentative Zoning is more complicated. The status of certain statements as problem or solutions is one important dimension in the definitions of AZ categories. AZ additionally models dimensions other than problem-solution hood (such as who a scientific idea belongs to, or which intention the authors might have had in stating a particular negative or positive statement). When forming categories, AZ combines aspects of these dimensions, and “flattens” them out into only 7 categories. In AZ it is crucial who it is that experiences the problems or contributes a solution. For instance, the definition of category “CONTRAST” includes statements that some research runs into problems, but only if that research is previous work (i.e., not if it is the work contributed in the paper itself). Similarly, “BASIS” includes statements of successful problem-solving activities, but only if they are achieved by previous work that the current paper bases itself on. Our definition is simpler in that we are interested only in problem solution structure, not in the other dimensions covered in AZ. Our definition is also more far-reaching than AZ, in that we are interested in all problems mentioned in the text, no matter whose problems they are. Problem-solution recognition can therefore be seen as one aspect of AZ which can be independently modelled as a “service task”. This means that good problem solution structure recognition should theoretically improve AZ recognition.
In this work, we approach the task of identifying problem-solving patterns in scientific text. We choose to use the model of problem-solving described by Hoey ( 2001 ). This pattern comprises four parts: Situation, Problem, Response and Evaluation. The Situation element is considered optional to the pattern, and so our focus centres on the core pattern elements.
Goal statement and task
Many surface features in the text offer themselves up as potential signals for detecting problem-solving patterns in text. However, since Situation is an optional element, we decided to focus on either Problem or Response and Evaluation as signals of the pattern. Moreover, we decide to look for each type in isolation. Our reasons for this are as follows: It is quite rare for an author to introduce a problem without resolving it using some sort of response, and so this is a good starting point in identifying the pattern. There are exceptions to this, as authors will sometimes introduce a problem and then leave it to future work, but overall there should be enough signal in the Problem element to make our method of looking for it in isolation worthwhile. The second signal we look for is the use of Response and Evaluation within the same sentence. Similar to Problem elements, we hypothesise that this formulation is well enough signalled externally to help us in detecting the pattern. For example, consider the following Response and Evaluation: “One solution is to use smoothing”. In this statement, the author is explicitly stating that smoothing is a solution to a problem which must have been mentioned in a prior statement. In scientific text, we often observe that solutions implicitly contain both Response and Evaluation (positive) elements. Therefore, due to these reasons there should be sufficient external signals for the two pattern elements we concentrate on here.
When attempting to find Problem elements in text, we run into the issue that the word “problem” actually has at least two word senses that need to be distinguished. There is a word sense of “problem” that means something which must be undertaken (i.e. task), while another sense is the core sense of the word, something that is problematic and negative. Only the latter sense is aligned with our sense of problemhood. This is because the simple description of a task does not predispose problemhood, just a wish to perform some act. Consider the following examples, where the non-desired word sense is being used:
- “Das and Petrov (2011) also consider the problem of unsupervised bilingual POS induction”. (Chen et al. 2011 ).
- “In this paper, we describe advances on the problem of NER in Arabic Wikipedia”. (Mohit et al. 2012 ).
Here, the author explicitly states that the phrases in orange are problems, they align with our definition of research tasks and not with what we call here ‘problematic problems’. We will now give some examples from our corpus for the desired, core word sense:
- “The major limitation of supervised approaches is that they require annotations for example sentences.” (Poon and Domingos 2009 ).
- “To solve the problem of high dimensionality we use clustering to group the words present in the corpus into much smaller number of clusters”. (Saha et al. 2008 ).
When creating our corpus of positive and negative examples, we took care to select only problem strings that satisfy our definition of problemhood; “ Corpus creation ” section will explain how we did that.
Corpus creation
Our new corpus is a subset of the latest version of the ACL anthology released in March, 2016 1 which contains 22,878 articles in the form of PDFs and OCRed text. 2
The 2016 version was also parsed using ParsCit (Councill et al. 2008 ). ParsCit recognises not only document structure, but also bibliography lists as well as references within running text. A random subset of 2500 papers was collected covering the entire ACL timeline. In order to disregard non-article publications such as introductions to conference proceedings or letters to the editor, only documents containing abstracts were considered. The corpus was preprocessed using tokenisation, lemmatisation and dependency parsing with the Rasp Parser (Briscoe et al. 2006 ).
Definition of ground truth
Our goal was to define a ground truth for problem and solution strings, while covering as wide a range as possible of syntactic variations in which such strings naturally occur. We also want this ground truth to cover phenomena of problem and solution status which are applicable whether or not the problem or solution status is explicitly mentioned in the text.
To simplify the task, we only consider here problem and solution descriptions that are at most one sentence long. In reality, of course, many problem descriptions and solution descriptions go beyond single sentence, and require for instance an entire paragraph. However, we also know that short summaries of problems and solutions are very prevalent in science, and also that these tend to occur in the most prominent places in a paper. This is because scientists are trained to express their contribution and the obstacles possibly hindering their success, in an informative, succinct manner. That is the reason why we can afford to only look for shorter problem and solution descriptions, ignoring those that cross sentence boundaries.
To define our ground truth, we examined the parsed dependencies and looked for a target word (“problem/solution”) in subject position, and then chose its syntactic argument as our candidate problem or solution phrase. To increase the variation, i.e., to find as many different-worded problem and solution descriptions as possible, we additionally used semantically similar words (near-synonyms) of the target words “problem” or “solution” for the search. Semantic similarity was defined as cosine in a deep learning distributional vector space, trained using Word2Vec (Mikolov et al. 2013 ) on 18,753,472 sentences from a biomedical corpus based on all full-text Pubmed articles (McKeown et al. 2016 ). From the 200 words which were semantically closest to “problem”, we manually selected 28 clear synonyms. These are listed in Table 1 . From the 200 semantically closest words to “solution” we similarly chose 19 (Table 2 ). Of the sentences matching our dependency search, a subset of problem and solution candidate sentences were randomly selected.
Selected words for use in problem candidate phrase extraction
Bottleneck | Caveat | Challenge | Complication | Conundrum | Difficulty |
Flaw | Impediment | Issue | Limitation | Mistake | Obstacle |
Riddle | Shortcoming | Struggle | Subproblem | Threat | Tragedy |
Dilemma | Disadvantage | Drawback | Fault | Pitfall | Problem |
Uncertainty | Weakness | Trouble | Quandary |
Selected words for use in solution candidate phrase extraction
Solution | Alternative | Suggestion | Idea | Way | Proposal |
Technique | Remedy | Task | Step | Answer | Approach |
Approaches | Strategy | Method | Methodology | Scheme | Answers |
Workaround |
An example of this is shown in Fig. 2 . Here, the target word “drawback” is in subject position (highlighted in red), and its clausal argument (ccomp) is “(that) it achieves low performance” (highlighted in purple). Examples of other arguments we searched for included copula constructions and direct/indirect objects.

Example of our extraction method for problems using dependencies. (Color figure online)
If more than one candidate was found in a sentence, one was chosen at random. Non-grammatical sentences were excluded; these might appear in the corpus as a result of its source being OCRed text.
800 candidates phrases expressing problems and solutions were automatically extracted (1600 total) and then independently checked for correctness by two annotators (the two authors of this paper). Both authors found the task simple and straightforward. Correctness was defined by two criteria:
- An unexplained phenomenon or a problematic state in science; or
- A research question; or
- An artifact that does not fulfil its stated specification.
- The phrase must not lexically give away its status as problem or solution phrase.
The second criterion saves us from machine learning cues that are too obvious. If for instance, the phrase itself contained the words “lack of” or “problematic” or “drawback”, our manual check rejected it, because it would be too easy for the machine learner to learn such cues, at the expense of many other, more generally occurring cues.
Sampling of negative examples
We next needed to find negative examples for both cases. We wanted them not to stand out on the surface as negative examples, so we chose them so as to mimic the obvious characteristics of the positive examples as closely as possible. We call the negative examples ‘non-problems’ and ‘non-solutions’ respectively. We wanted the only differences between problems and non-problems to be of a semantic nature, nothing that could be read off on the surface. We therefore sampled a population of phrases that obey the same statistical distribution as our problem and solution strings while making sure they really are negative examples. We started from sentences not containing any problem/solution words (i.e. those used as target words). From each such sentence, we at random selected one syntactic subtree contained in it. From these, we randomly selected a subset of negative examples of problems and solutions that satisfy the following conditions:
- The distribution of the head POS tags of the negative strings should perfectly match the head POS tags 3 of the positive strings. This has the purpose of achieving the same proportion of surface syntactic constructions as observed in the positive cases.
- The average lengths of the negative strings must be within a tolerance of the average length of their respective positive candidates e.g., non-solutions must have an average length very similar (i.e. + / - small tolerance) to solutions. We chose a tolerance value of 3 characters.
Again, a human quality check was performed on non-problems and non-solutions. For each candidate non-problem statement, the candidate was accepted if it did not contain a phenomenon, a problematic state, a research question or a non-functioning artefact. If the string expressed a research task, without explicit statement that there was anything problematic about it (i.e., the ‘wrong’ sense of “problem”, as described above), it was allowed as a non-problem. A clause was confirmed as a non-solution if the string did not represent both a response and positive evaluation.
If the annotator found that the sentence had been slightly mis-parsed, but did contain a candidate, they were allowed to move the boundaries for the candidate clause. This resulted in cleaner text, e.g., in the frequent case of coordination, when non-relevant constituents could be removed.
From the set of sentences which passed the quality-test for both independent assessors, 500 instances of positive and negative problems/solutions were randomly chosen (i.e. 2000 instances in total). When checking for correctness we found that most of the automatically extracted phrases which did not pass the quality test for problem-/solution-hood were either due to obvious learning cues or instances where the sense of problem-hood used is relating to tasks (cf. “ Goal statement and task ” section).
Experimental design
In our experiments, we used three classifiers, namely Naïve Bayes, Logistic Regression and a Support Vector Machine. For all classifiers an implementation from the WEKA machine learning library (Hall et al. 2009 ) was chosen. Given that our dataset is small, tenfold cross-validation was used instead of a held out test set. All significance tests were conducted using the (two-tailed) Sign Test (Siegel 1956 ).
Linguistic correlates of problem- and solution-hood
We first define a set of features without taking the phrase’s context into account. This will tell us about the disambiguation ability of the problem/solution description’s semantics alone. In particular, we cut out the rest of the sentence other than the phrase and never use it for classification. This is done for similar reasons to excluding certain ‘give-away’ phrases inside the phrases themselves (as explained above). As the phrases were found using templates, we know that the machine learner would simply pick up on the semantics of the template, which always contains a synonym of “problem” or “solution”, thus drowning out the more hidden features hopefully inherent in the semantics of the phrases themselves. If we allowed the machine learner to use these stronger features, it would suffer in its ability to generalise to the real task.
ngrams Bags of words are traditionally successfully used for classification tasks in NLP, so we included bags of words (lemmas) within the candidate phrases as one of our features (and treat it as a baseline later on). We also include bigrams and trigrams as multi-word combinations can be indicative of problems and solutions e.g., “combinatorial explosion”.
Polarity Our second feature concerns the polarity of each word in the candidate strings. Consider the following example of a problem taken from our dataset: “very conservative approaches to exact and partial string matches overgenerate badly”. In this sentence, words such as “badly” will be associated with negative polarity, therefore being useful in determining problem-hood. Similarly, solutions will often be associated with a positive sentiment e.g. “smoothing is a good way to overcome data sparsity” . To do this, we perform word sense disambiguation of each word using the Lesk algorithm (Lesk 1986 ). The polarity of the resulting synset in SentiWordNet (Baccianella et al. 2010 ) was then looked up and used as a feature.
Syntax Next, a set of syntactic features were defined by using the presence of POS tags in each candidate. This feature could be helpful in finding syntactic patterns in problems and solutions. We were careful not to base the model directly on the head POS tag and the length of each candidate phrase, as these are defining characteristics used for determining the non-problem and non-solution candidate set.
Negation Negation is an important property that can often greatly affect the polarity of a phrase. For example, a phrase containing a keyword pertinent to solution-hood may be a good indicator but with the presence of negation may flip the polarity to problem-hood e.g., “this can’t work as a solution”. Therefore, presence of negation is determined.
Exemplification and contrast Problems and solutions are often found to be coupled with examples as they allow the author to elucidate their point. For instance, consider the following solution: “Once the translations are generated, an obvious solution is to pick the most fluent alternative, e.g., using an n-gram language model”. (Madnani et al. 2012 ). To acknowledge this, we check for presence of exemplification. In addition to examples, problems in particular are often found when contrast is signalled by the author (e.g. “however, “but”), therefore we also check for presence of contrast in the problem and non-problem candidates only.
Discourse Problems and solutions have also been found to have a correlation with discourse properties. For example, problem-solving patterns often occur in the background sections of a paper. The rationale behind this is that the author is conventionally asked to objectively criticise other work in the background (e.g. describing research gaps which motivate the current paper). To take this in account, we examine the context of each string and capture the section header under which it is contained (e.g. Introduction, Future work). In addition, problems and solutions are often found following the Situation element in the problem-solving pattern (cf. “ Introduction ” section). This preamble setting up the problem or solution means that these elements are likely not to be found occurring at the beginning of a section (i.e. it will usually take some sort of introduction to detail how something is problematic and why a solution is needed). Therefore we record the distance from the candidate string to the nearest section header.
Subcategorisation and adverbials Solutions often involve an activity (e.g. a task). We also model the subcategorisation properties of the verbs involved. Our intuition was that since problematic situations are often described as non-actions, then these are more likely to be intransitive. Conversely solutions are often actions and are likely to have at least one argument. This feature was calculated by running the C&C parser (Curran et al. 2007 ) on each sentence. C&C is a supertagger and parser that has access to subcategorisation information. Solutions are also associated with resultative adverbial modification (e.g. “thus, therefore, consequently”) as it expresses the solutionhood relation between the problem and the solution. It has been seen to occur frequently in problem-solving patterns, as studied by Charles ( 2011 ). Therefore, we check for presence of resultative adverbial modification in the solution and non-solution candidate only.
Embeddings We also wanted to add more information using word embeddings. This was done in two different ways. Firstly, we created a Doc2Vec model (Le and Mikolov 2014 ), which was trained on ∼ 19 million sentences from scientific text (no overlap with our data set). An embedding was created for each candidate sentence. Secondly, word embeddings were calculated using the Word2Vec model (cf. “ Corpus creation ” section). For each candidate head, the full word embedding was included as a feature. Lastly, when creating our polarity feature we query SentiWordNet using synsets assigned by the Lesk algorithm. However, not all words are assigned a sense by Lesk, so we need to take care when that happens. In those cases, the distributional semantic similarity of the word is compared to two words with a known polarity, namely “poor” and “excellent”. These particular words have traditionally been consistently good indicators of polarity status in many studies (Turney 2002 ; Mullen and Collier 2004 ). Semantic similarity was defined as cosine similarity on the embeddings of the Word2Vec model (cf. “ Corpus creation ” section).
Modality Responses to problems in scientific writing often express possibility and necessity, and so have a close connection with modality. Modality can be broken into three main categories, as described by Kratzer ( 1991 ), namely epistemic (possibility), deontic (permission / request / wish) and dynamic (expressing ability).
Problems have a strong relationship to modality within scientific writing. Often, this is due to a tactic called “hedging” (Medlock and Briscoe 2007 ) where the author uses speculative language, often using Epistemic modality, in an attempt to make either noncommital or vague statements. This has the effect of allowing the author to distance themselves from the statement, and is often employed when discussing negative or problematic topics. Consider the following example of Epistemic modality from Nakov and Hearst ( 2008 ): “A potential drawback is that it might not work well for low-frequency words”.
To take this linguistic correlate into account as a feature, we replicated a modality classifier as described by (Ruppenhofer and Rehbein 2012 ). More sophisticated modality classifiers have been recently introduced, for instance using a wide range of features and convolutional neural networks, e.g, (Zhou et al. 2015 ; Marasović and Frank 2016 ). However, we wanted to check the effect of a simpler method of modality classification on the final outcome first before investing heavily into their implementation. We trained three classifiers using the subset of features which Ruppenhofer et al. reported as performing best, and evaluated them on the gold standard dataset provided by the authors 4 . The results of the are shown in Table 3 . The dataset contains annotations of English modal verbs on the 535 documents of the first MPQA corpus release (Wiebe et al. 2005 ).
Modality classifier results (precision/recall/f-measure) using Naïve Bayes (NB), logistic regression, and a support vector machine (SVM)
Modality | Classification accuracy | ||
---|---|---|---|
NB | LR | SVM | |
Epistemic | .74/.74/.74 | .75/.85/.80 | |
Deontic | .94/.72/.81 | .86/.81/.83 | |
Dynamic | .69/.70/.70 |
Italicized results reflect highest f-measure reported per modal category
Logistic Regression performed best overall and so this model was chosen for our upcoming experiments. With regards to the optative and concessive modal categories, they can be seen to perform extremely poorly, with the optative category receiving a null score across all three classifiers. This is due to a limitation in the dataset, which is unbalanced and contains very few instances of these two categories. This unbalanced data also is the reason behind our decision of reporting results in terms of recall, precision and f-measure in Table 3 .
The modality classifier was then retrained on the entirety of the dataset used by Ruppenhofer and Rehbein ( 2012 ) using the best performing model from training (Logistic Regression). This new model was then used in the upcoming experiment to predict modality labels for each instance in our dataset.
As can be seen from Table 4 , we are able to achieve good results for distinguishing a problematic statement from non-problematic one. The bag-of-words baseline achieves a very good performance of 71.0% for the Logistic Regression classifier, showing that there is enough signal in the candidate phrases alone to distinguish them much better than random chance.
Results distinguishing problems from non-problems using Naïve Bayes (NB), logistic regression (LR) and a support vector machine (SVM)
Feature sets | Classification accuracy | |||
---|---|---|---|---|
NB | SVM | LR | ||
1 | Baseline | 65.6 | 67.8 | 71.0 |
2 | Bigrams | 61.3 | 60.5 | 59.0 |
3 | Contrast | 50.6 | 50.8 | 50.5 |
4 | Discourse | 60.3 | 60.2 | 60.0 |
5 | Doc2vec | 72.9* | 72.7 | 72.3 |
6 | Exemplification | 50.3 | 50.2 | 50.0 |
7 | Modality | 52.3 | 52.3 | 50.3 |
8 | Negation | 59.9 | 59.9 | 59.9 |
9 | Polarity | 60.2 | 66.3 | 65.5 |
10 | Syntax | 73.6* | 76.2** | 74.4 |
11 | Subcategorisation | 46.9 | 47.3 | 49.1 |
12 | Trigrams | 57.7 | 51.2 | 54.0 |
13 | Word2vec | 57.9 | 64.1 | 64.7 |
14 | Word2vec | 76.2*** | 77.2** | 76.6 |
15 | All features | 79.3*** | 81.8*** | ** |
16 | All features-{2,3,7,12} | 79.0** |
Each feature set’s performance is shown in isolation followed by combinations with other features. Tenfold stratified cross-validation was used across all experiments. Statistical significance with respect to the baseline at the p < 0.05 , 0.01, 0.001 levels is denoted by *, ** and *** respectively
Taking a look at Table 5 , which shows the information gain for the top lemmas,
Information gain (IG) in bits of top lemmas from the bag-of-words baseline in Table 4
IG | Features |
---|---|
0.048 | Not |
0.019 | Do |
0.018 | Single |
0.013 | Limited, experiment |
0.010 | Data, information |
0.009 | Error, many |
0.008 | Take, explosion |
we can see that the top lemmas are indeed indicative of problemhood (e.g. “limit”,“explosion”). Bigrams achieved good performance on their own (as did negation and discourse) but unfortunately performance deteriorated when using trigrams, particularly with the SVM and LR. The subcategorisation feature was the worst performing feature in isolation. Upon taking a closer look at our data, we saw that our hypothesis that intransitive verbs are commonly used in problematic statements was true, with over 30% of our problems (153) using them. However, due to our sampling method for the negative cases we also picked up many intransitive verbs (163). This explains the almost random chance performance (i.e. 50%) given that the distribution of intransitive verbs amongst the positive and negative candidates was almost even.
The modality feature was the most expensive to produce, but also didn’t perform very well is isolation. This surprising result may be partly due to a data sparsity issue
where only a small portion (169) of our instances contained modal verbs. The breakdown of how many types of modal senses which occurred is displayed in Table 6 . The most dominant modal sense was epistemic. This is a good indicator of problemhood (e.g. hedging, cf. “ Linguistic correlates of problem- and solution-hood ” section) but if the accumulation of additional data was possible, we think that this feature may have the potential to be much more valuable in determining problemhood. Another reason for the performance may be domain dependence of the classifier since it was trained on text from different domains (e.g. news). Additionally, modality has also shown to be helpful in determining contextual polarity (Wilson et al. 2005 ) and argumentation (Becker et al. 2016 ), so using the output from this modality classifier may also prove useful for further feature engineering taking this into account in future work.
Number of instances of modal senses
No. of instances | |
---|---|
Epistemic | 97 |
Deontic | 22 |
Dynamic | 50 |
Polarity managed to perform well but not as good as we hoped. However, this feature also suffers from a sparsity issue resulting from cases where the Lesk algorithm (Lesk 1986 ) is not able to resolve the synset of the syntactic head.
Knowledge of syntax provides a big improvement with a significant increase over the baseline results from two of the classifiers.
Examining this in greater detail, POS tags with high information gain mostly included tags from open classes (i.e. VB-, JJ-, NN- and RB-). These tags are often more associated with determining polarity status than tags such as prepositions and conjunctions (i.e. adverbs and adjectives are more likely to be describing something with a non-neutral viewpoint).
The embeddings from Doc2Vec allowed us to obtain another significant increase in performance (72.9% with Naïve Bayes) over the baseline and polarity using Word2Vec provided the best individual feature result (77.2% with SVM).
Combining all features together, each classifier managed to achieve a significant result over the baseline with the best result coming from the SVM (81.8%). Problems were also better classified than non-problems as shown in the confusion matrix in Table 7 . The addition of the Word2Vec vectors may be seen as a form of smoothing in cases where previous linguistic features had a sparsity issue i.e., instead of a NULL entry, the embeddings provide some sort of value for each candidate. Particularly wrt. the polarity feature, cases where Lesk was unable to resolve a synset meant that a ZERO entry was added to the vector supplied to the machine learner. Amongst the possible combinations, the best subset of features was found by combining all features with the exception of bigrams, trigrams, subcategorisation and modality. This subset of features managed to improve results in both the Naïve Bayes and SVM classifiers with the highest overall result coming from the SVM (82.3%).
Confusion matrix for problems
Predicted | ||
---|---|---|
Problem | Non-problem | |
Actual | ||
Problem | 414 | 86 |
Non-problem | 91 | 409 |
The results for disambiguation of solutions from non-solutions can be seen in Table 8 . The bag-of-words baseline performs much better than random, with the performance being quite high with regard to the SVM (this result was also higher than any of the baseline performances from the problem classifiers). As shown in Table 9 , the top ranked lemmas from the best performing model (using information gain) included “use” and “method”. These lemmas are very indicative of solutionhood and so give some insight into the high baseline returned from the machine learners. Subcategorisation and the result adverbials were the two worst performing features. However, the low performance for subcategorisation is due to the sampling of the non-solutions (the same reason for the low performance of the problem transitivity feature). When fitting the POS-tag distribution for the negative samples, we noticed that over 80% of the head POS-tags were verbs (much higher than the problem heads). The most frequent verb type being the infinite form.
Results distinguishing solutions from non-solutions using Naïve Bayes (NB), logistic regression (LR) and a support vector machine (SVM)
Feature sets | Classification accuracy | |||
---|---|---|---|---|
NB | SVM | LR | ||
1 | Baseline | 72.5 | 73.6 | 70.7 |
2 | Adverbial of result | 48.3 | 50.5 | 50.3 |
3 | Bigrams | 63.1 | 65.1 | 59.8 |
4 | Discourse | 56.9 | 56.4 | 58.2 |
5 | Doc2vec | 65.9 | 68.7 | 67.7 |
6 | Exemplification | 63.1 | 65.1 | 59.8 |
7 | Negation | 63.1 | 65.1 | 59.8 |
8 | Polarity | 63.1 | 65.1 | 59.8 |
9 | Subcategorisation | 55.4 | 53.3 | 55.3 |
10 | Syntax | 61.9 | 62.2 | 64.4 |
11 | Trigrams | 63.1 | 65.1 | 59.8 |
12 | Word2vec | 68.2 | 70.7 | 68.9 |
13 | Word2vec | 72.1 | 73.4 | 69.4 |
14 | All features | 79.5 | 73.1 | |
15 | All features-{2,3,6,7,8,13} | 73.8 |
Each feature set’s performance is shown in isolation followed by combinations with other features. Tenfold stratified cross-validation was used across all experiments
Information gain (IG) in bits of top lemmas from the bag-of-words baseline in Table 8
IG | Features |
---|---|
0.076 | Be |
0.047 | Use |
0.014 | Method |
0.013 | Argument |
0.012 | Dependency |
0.011 | Configuration, sequence, subject |
0.009 | Label, weakest |
0.008 | Following, edge, employ |
This is not surprising given that a very common formulation to describe a solution is to use the infinitive “TO” since it often describes a task e.g., “One solution is to find the singletons and remove them”. Therefore, since the head POS tags of the non-solutions had to match this high distribution of infinitive verbs present in the solution, the subcategorisation feature is not particularly discriminatory. Polarity, negation, exemplification and syntactic features were slightly more discriminate and provided comparable results. However, similar to the problem experiment, the embeddings from Word2Vec and Doc2Vec proved to be the best features, with polarity using Word2Vec providing the best individual result (73.4% with SVM).
Combining all features together managed to improve over each feature in isolation and beat the baseline using all three classifiers. Furthermore, when looking at the confusion matrix in Table 10 the solutions were classified more accurately than the non-solutions. The best subset of features was found by combining all features without adverbial of result, bigrams, exemplification, negation, polarity and subcategorisation. The best result using this subset of features was achieved by the SVM with 79.7%. It managed to greatly improve upon the baseline but was just shy of achieving statistical significance ( p = 0.057 ).
Confusion matrix for solutions
Predicted | ||
---|---|---|
Solution | Non-solution | |
Actual | ||
Solution | 411 | 89 |
Non-solution | 114 | 386 |
In this work, we have presented new supervised classifiers for the task of identifying problem and solution statements in scientific text. We have also introduced a new corpus for this task and used it for evaluating our classifiers. Great care was taken in constructing the corpus by ensuring that the negative and positive samples were closely matched in terms of syntactic shape. If we had simply selected random subtrees for negative samples without regard for any syntactic similarity with our positive samples, the machine learner may have found easy signals such as sentence length. Additionally, since we did not allow the machine learner to see the surroundings of the candidate string within the sentence, this made our task even harder. Our performance on the corpus shows promise for this task, and proves that there are strong signals for determining both the problem and solution parts of the problem-solving pattern independently.
With regard to classifying problems from non-problems, features such as the POS tag, document and word embeddings provide the best features, with polarity using the Word2Vec embeddings achieving the highest feature performance. The best overall result was achieved using an SVM with a subset of features (82.3%). Classifying solutions from non-solutions also performs well using the embedding features, with the best feature also being polarity using the Word2Vec embeddings, and the highest result also coming from the SVM with a feature subset (79.7%).
In future work, we plan to link problem and solution statements which were found independently during our corpus creation. Given that our classifiers were trained on data solely from the ACL anthology, we also hope to investigate the domain specificity of our classifiers and see how well they can generalise to domains other than ACL (e.g. bioinformatics). Since we took great care at removing the knowledge our classifiers have of the explicit statements of problem and solution (i.e. the classifiers were trained only on the syntactic argument of the explicit statement of problem-/solution-hood), our classifiers should in principle be in a good position to generalise, i.e., find implicit statements too. In future work, we will measure to which degree this is the case.
To facilitate further research on this topic, all code and data used in our experiments can be found here: www.cl.cam.ac.uk/~kh562/identifying-problems-and-solutions.html
Acknowledgements
The first author has been supported by an EPSRC studentship (Award Ref: 1641528). We thank the reviewers for their helpful comments.
1 http://acl-arc.comp.nus.edu.sg/ .
2 The corpus comprises 3,391,198 sentences, 71,149,169 words and 451,996,332 characters.
3 The head POS tags were found using a modification of the Collins’ Head Finder. This modified algorithm addresses some of the limitations of the head finding heuristics described by Collins ( 2003 ) and can be found here: http://nlp.stanford.edu/nlp/javadoc/javanlp/edu/stanford/nlp/trees/ModCollinsHeadFinder.html .
4 https://www.uni-hildesheim.de/ruppenhofer/data/modalia_release1.0.tgz.
Contributor Information
Kevin Heffernan, Email: [email protected] .
Simone Teufel, Email: [email protected] .
- Baccianella S, Esuli A, Sebastiani F. Sentiwordnet 3.0: An enhanced lexical resource for sentiment analysis and opinion mining. LREC. 2010; 10 :2200–2204. [ Google Scholar ]
- Becker, M., Palmer, A., & Frank, A. (2016). Clause types and modality in argumentative microtexts. In Workshop on foundations of the language of argumentation (in conjunction with COMMA) .
- Briscoe, T., Carroll, J., & Watson, R. (2006). The second release of the rasp system. In Proceedings of the COLING/ACL on interactive presentation sessions, association for computational linguistics pp. 77–80.
- Chandrasekaran B. Towards a taxonomy of problem solving types. AI Magazine. 1983; 4 (1):9. [ Google Scholar ]
- Charles M. Adverbials of result: Phraseology and functions in the problem-solution pattern. Journal of English for Academic Purposes. 2011; 10 (1):47–60. doi: 10.1016/j.jeap.2011.01.002. [ CrossRef ] [ Google Scholar ]
- Chen, D., Dyer, C., Cohen, S. B., & Smith, N. A. (2011). Unsupervised bilingual pos tagging with markov random fields. In Proceedings of the first workshop on unsupervised learning in NLP, association for computational linguistics pp. 64–71.
- Collins M. Head-driven statistical models for natural language parsing. Computational Linguistics. 2003; 29 (4):589–637. doi: 10.1162/089120103322753356. [ CrossRef ] [ Google Scholar ]
- Councill, I. G., Giles, C. L., & Kan, M. Y. (2008). Parscit: An open-source CRF reference string parsing package. In LREC .
- Curran, J. R., Clark, S., & Bos, J. (2007). Linguistically motivated large-scale NLP with C&C and boxer. In Proceedings of the 45th annual meeting of the ACL on interactive poster and demonstration sessions, association for computational linguistics pp. 33–36.
- Flowerdew L. Corpus-based analyses of the problem-solution pattern: A phraseological approach. Amsterdam: John Benjamins Publishing; 2008. [ Google Scholar ]
- Grimes JE. The thread of discourse. Berlin: Walter de Gruyter; 1975. [ Google Scholar ]
- Hall M, Frank E, Holmes G, Pfahringer B, Reutemann P, Witten IH. The weka data mining software: An update. ACM SIGKDD Explorations Newsletter. 2009; 11 (1):10–18. doi: 10.1145/1656274.1656278. [ CrossRef ] [ Google Scholar ]
- Hoey M. Textual interaction: An introduction to written discourse analysis. Portland: Psychology Press; 2001. [ Google Scholar ]
- Hutchins J. On the structure of scientific texts. UEA Papers in Linguistics. 1977; 5 (3):18–39. [ Google Scholar ]
- Jonassen DH. Toward a design theory of problem solving. Educational Technology Research and Development. 2000; 48 (4):63–85. doi: 10.1007/BF02300500. [ CrossRef ] [ Google Scholar ]
- Jordan MP. Short texts to explain problem-solution structures-and vice versa. Instructional Science. 1980; 9 (3):221–252. doi: 10.1007/BF00177328. [ CrossRef ] [ Google Scholar ]
- Kratzer, A. (1991). Modality. In von Stechow & Wunderlich (Eds.), Semantics: An international handbook of contemporary research .
- Le QV, Mikolov T. Distributed representations of sentences and documents. ICML. 2014; 14 :1188–1196. [ Google Scholar ]
- Lesk, M. (1986). Automatic sense disambiguation using machine readable dictionaries: How to tell a pine cone from an ice cream cone. In Proceedings of the 5th annual international conference on Systems documentation, ACM (pp. 24–26).
- Madnani, N., Tetreault, J., & Chodorow, M. (2012). Exploring grammatical error correction with not-so-crummy machine translation. In Proceedings of the seventh workshop on building educational applications using NLP, association for computational linguistics pp. 44–53.
- Mann WC, Thompson SA. Rhetorical structure theory: Toward a functional theory of text organization. Text-Interdisciplinary Journal for the Study of Discourse. 1988; 8 (3):243–281. doi: 10.1515/text.1.1988.8.3.243. [ CrossRef ] [ Google Scholar ]
- Marasović, A., & Frank, A. (2016). Multilingual modal sense classification using a convolutional neural network. In Proceedings of the 1st Workshop on Representation Learning for NLP .
- McKeown K, Daume H, Chaturvedi S, Paparrizos J, Thadani K, Barrio P, Biran O, Bothe S, Collins M, Fleischmann KR, et al. Predicting the impact of scientific concepts using full-text features. Journal of the Association for Information Science and Technology. 2016; 67 :2684–2696. doi: 10.1002/asi.23612. [ CrossRef ] [ Google Scholar ]
- Medlock B, Briscoe T. Weakly supervised learning for hedge classification in scientific literature. ACL, Citeseer. 2007; 2007 :992–999. [ Google Scholar ]
- Mikolov, T., Sutskever, I., Chen, K., Corrado, G. S., & Dean, J. (2013). Distributed representations of words and phrases and their compositionality. In Advances in neural information processing systems (pp. 3111–3119).
- Mohit, B., Schneider, N., Bhowmick, R., Oflazer, K., & Smith, N. A. (2012). Recall-oriented learning of named entities in arabic wikipedia. In Proceedings of the 13th conference of the European chapter of the association for computational linguistics, association for computational linguistics (pp. 162–173).
- Mullen T, Collier N. Sentiment analysis using support vector machines with diverse information sources. EMNLP. 2004; 4 :412–418. [ Google Scholar ]
- Nakov, P., Hearst, M. A. (2008). Solving relational similarity problems using the web as a corpus. In: ACL (pp. 452–460).
- Poon, H., & Domingos, P. (2009). Unsupervised semantic parsing. In Proceedings of the 2009 conference on empirical methods in natural language processing: Volume 1-association for computational linguistics (pp. 1–10).
- Ruppenhofer, J., & Rehbein, I. (2012). Yes we can!? Annotating the senses of English modal verbs. In Proceedings of the 8th international conference on language resources and evaluation (LREC), Citeseer (pp. 24–26).
- Saha, S. K., Mitra, P., & Sarkar, S. (2008). Word clustering and word selection based feature reduction for maxent based hindi ner. In ACL (pp. 488–495).
- Scott, M. (2001). Mapping key words to problem and solution. In Patterns of text: In honour of Michael Hoey Benjamins, Amsterdam (pp. 109–127).
- Siegel S. Nonparametric statistics for the behavioral sciences. New York: McGraw-hill; 1956. [ Google Scholar ]
- Strübing, J. (2007). Research as pragmatic problem-solving: The pragmatist roots of empirically-grounded theorizing. In The Sage handbook of grounded theory (pp. 580–602).
- Teufel, S., et al. (2000). Argumentative zoning: Information extraction from scientific text. PhD Thesis, Citeseer .
- Turney, P. D. (2002). Thumbs up or thumbs down?: Semantic orientation applied to unsupervised classification of reviews. In Proceedings of the 40th annual meeting on association for computational linguistics, association for computational linguistics (pp. 417–424).
- Van Dijk TA. Text and context explorations in the semantics and pragmatics of discourse. London: Longman; 1980. [ Google Scholar ]
- Wiebe J, Wilson T, Cardie C. Annotating expressions of opinions and emotions in language. Language Resources and Evaluation. 2005; 39 (2):165–210. doi: 10.1007/s10579-005-7880-9. [ CrossRef ] [ Google Scholar ]
- Wilson, T., Wiebe, J., & Hoffmann, P. (2005). Recognizing contextual polarity in phrase-level sentiment analysis. In Proceedings of the conference on human language technology and empirical methods in natural language processing, association for computational linguistics (pp. 347–354).
- Winter, E. O. (1968). Some aspects of cohesion. In Sentence and clause in scientific English . University College London.
- Zhou, M., Frank, A., Friedrich, A., & Palmer, A. (2015). Semantically enriched models for modal sense classification. In Workshop on linking models of lexical, sentential and discourse-level semantics (LSDSem) (p. 44).

The science behind creativity
Psychologists and neuroscientists are exploring where creativity comes from and how to increase your own
Vol. 53 No. 3 Print version: page 40
- Neuropsychology
- Creativity and Innovation
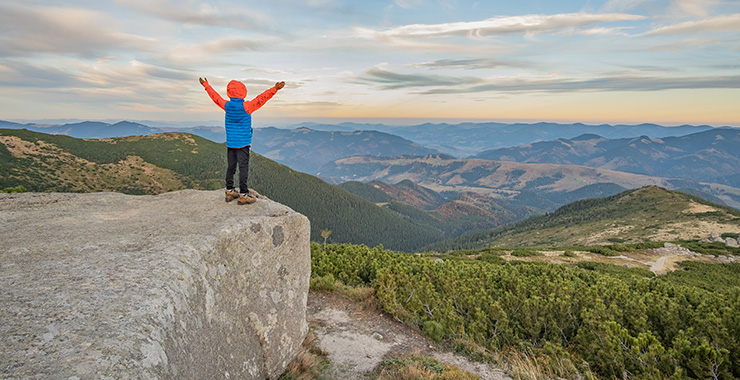
Paul Seli, PhD, is falling asleep. As he nods off, a sleep-tracking glove called Dormio, developed by scientists at the Massachusetts Institute of Technology, detects his nascent sleep state and jars him awake. Pulled back from the brink, he jots down the artistic ideas that came to him during those semilucid moments.
Seli is an assistant professor of psychology and neuroscience at the Duke Institute for Brain Sciences and also an artist. He uses Dormio to tap into the world of hypnagogia, the transitional state that exists at the boundary between wakefulness and sleep. In a mini-experiment, he created a series of paintings inspired by ideas plucked from his hypnagogic state and another series from ideas that came to him during waking hours. Then he asked friends to rate how creative the paintings were, without telling them which were which. They judged the hypnagogic paintings as significantly more creative. “In dream states, we seem to be able to link things together that we normally wouldn’t connect,” Seli said. “It’s like there’s an artist in my brain that I get to know through hypnagogia.”
The experiment is one of many novel—and, yes, creative—ways that psychologists are studying the science of creativity. At an individual level, creativity can lead to personal fulfillment and positive academic and professional outcomes, and even be therapeutic. People take pleasure in creative thoughts, research suggests—even if they don’t think of themselves as especially creative. Beyond those individual benefits, creativity is an endeavor with implications for society, said Jonathan Schooler, PhD, a professor of psychological and brain sciences at the University of California, Santa Barbara. “Creativity is at the core of innovation. We rely on innovation for advancing humanity, as well as for pleasure and entertainment,” he said. “Creativity underlies so much of what humans value.”
In 1950, J. P. Guilford, PhD, then president of APA, laid out his vision for the psychological study of creativity ( American Psychologist , Vol. 5, No. 9, 1950). For half a century, researchers added to the scientific understanding of creativity incrementally, said John Kounios, PhD, an experimental psychologist who studies creativity and insight at Drexel University in Philadelphia. Much of that research focused on the personality traits linked to creativity and the cognitive aspects of the creative process.
But in the 21st century, the field has blossomed thanks to new advances in neuroimaging. “It’s become a tsunami of people studying creativity,” Kounios said. Psychologists and neuroscientists are uncovering new details about what it means to be creative and how to nurture that skill. “Creativity is of incredible real-world value,” Kounios said. “The ultimate goal is to figure out how to enhance it in a systematic way.”
Creativity in the brain
What, exactly, is creativity? The standard definition used by researchers characterizes creative ideas as those that are original and effective, as described by psychologist Mark A. Runco, PhD, director of creativity research and programming at Southern Oregon University ( Creativity Research Journal , Vol. 24, No. 1, 2012). But effectiveness, also called utility, is a slippery concept. Is a poem useful? What makes a sculpture effective? “Most researchers use some form of this definition, but most of us are also dissatisfied with it,” Kounios said.
Runco is working on an updated definition and has considered at least a dozen suggestions from colleagues for new components to consider. One frequently suggested feature is authenticity. “Creativity involves an honest expression,” he said.
Meanwhile, scientists are also struggling with the best way to measure the concept. As a marker of creativity, researchers often measure divergent thinking—the ability to generate a lot of possible solutions to a problem or question. The standard test of divergent thinking came from Guilford himself. Known as the alternate-uses test, the task asks participants to come up with novel uses for a common object such as a brick. But measures of divergent thinking haven’t been found to correlate well with real-world creativity. Does coming up with new uses for a brick imply a person will be good at abstract art or composing music or devising new methods for studying the brain? “It strikes me as using way too broad a brush,” Seli said. “I don’t think we measure creativity in the standard way that people think about creativity. As researchers, we need to be very clear about what we mean.”
One way to do that may be to move away from defining creativity based on a person’s creative output and focus instead on what’s going on in the brain, said Adam Green, PhD, a cognitive neuroscientist at Georgetown University and founder of the Society for the Neuroscience of Creativity . “The standard definition, that creativity is novel and useful, is a description of a product,” he noted. “By looking inward, we can see the process in action and start to identify the characteristics of creative thought. Neuroimaging is helping to shift the focus from creative product to creative process.”
That process seems to involve the coupling of disparate brain regions. Specifically, creativity often involves coordination between the cognitive control network, which is involved in executive functions such as planning and problem-solving, and the default mode network, which is most active during mind-wandering or daydreaming (Beaty, R. E., et al., Cerebral Cortex , Vol. 31, No. 10, 2021). The cooperation of those networks may be a unique feature of creativity, Green said. “These two systems are usually antagonistic. They rarely work together, but creativity seems to be one instance where they do.”
Green has also found evidence that an area called the frontopolar cortex, in the brain’s frontal lobes, is associated with creative thinking. And stimulating the area seems to boost creative abilities. He and his colleagues used transcranial direct current stimulation (tDCS) to stimulate the frontopolar cortex of participants as they tried to come up with novel analogies. Stimulating the area led participants to make analogies that were more semantically distant from one another—in other words, more creative ( Cerebral Cortex , Vol. 27, No. 4, 2017).
Green’s work suggests that targeting specific areas in the brain, either with neuromodulation or cognitive interventions, could enhance creativity. Yet no one is suggesting that a single brain region, or even a single neural network, is responsible for creative thought. “Creativity is not one system but many different mechanisms that, under ideal circumstances, work together in a seamless way,” Kounios said.
In search of the eureka moment
Creativity looks different from person to person. And even within one brain, there are different routes to a creative spark, Kounios explained. One involves what cognitive scientists call “System 1” (also called “Type 1”) processes: quick, unconscious thoughts—aha moments—that burst into consciousness. A second route involves “System 2” processes: thinking that is slow, deliberate, and conscious. “Creativity can use one or the other or a combination of the two,” he said. “You might use Type 1 thinking to generate ideas and Type 2 to critique and refine them.”
Which pathway a person uses might depend, in part, on their expertise. Kounios and his colleagues used electroencephalography (EEG) to examine what was happening in jazz musicians’ brains as they improvised on the piano. Then skilled jazz instructors rated those improvisations for creativity, and the researchers compared each musician’s most creative compositions. They found that for highly experienced musicians, the mechanisms used to generate creative ideas were largely automatic and unconscious, and they came from the left posterior part of the brain. Less-experienced pianists drew on more analytical, deliberative brain processes in the right frontal region to devise creative melodies, as Kounios and colleagues described in a special issue of NeuroImage on the neuroscience of creativity (Vol. 213, 2020). “It seems there are at least two pathways to get from where you are to a creative idea,” he said.
Coming up with an idea is only one part of the creative process. A painter needs to translate their vision to canvas. An inventor has to tinker with their concept to make a prototype that actually works. Still, the aha moment is an undeniably important component of the creative process. And science is beginning to illuminate those “lightbulb moments.”
Kounios examined the relationship between creative insight and the brain’s reward system by asking participants to solve anagrams in the lab. In people who were highly sensitive to rewards, a creative insight led to a burst of brain activity in the orbitofrontal cortex, the area of the brain that responds to basic pleasures like delicious food or addictive drugs ( NeuroImage , Vol. 214, 2020). That neural reward may explain, from an evolutionary standpoint, why humans seem driven to create, he said. “We seem wired to take pleasure in creative thoughts. There are neural rewards for thinking in a creative fashion, and that may be adaptive for our species.”
The rush you get from an aha moment might also signal that you’re onto something good, Schooler said. He and his colleagues studied these flashes of insight among creative writers and physicists. They surveyed the participants daily for two weeks, asking them to note their creative ideas and when they occurred. Participants reported that about a fifth of the most important ideas of the day happened when they were mind-wandering and not working on a task at hand ( Psychological Science , Vol. 30, No. 3, 2019). “These solutions were more likely to be associated with an aha moment and often overcoming an impasse of some sort,” Schooler said.
Six months later, the participants revisited those ideas and rated them for creative importance. This time, they rated their previous ideas as creative, but less important than they’d initially thought. That suggests that the spark of a eureka moment may not be a reliable clue that an idea has legs. “It seems like the aha experience may be a visceral marker of an important idea. But the aha experience can also inflate the meaningfulness of an idea that doesn’t have merit,” Schooler said. “We have to be careful of false ahas.”
Boosting your creativity
Much of the research in this realm has focused on creativity as a trait. Indeed, some people are naturally more creative than others. Creative individuals are more likely than others to possess the personality trait of openness. “Across different age groups, the best predictor of creativity is openness to new experiences,” said Anna Abraham, PhD, the E. Paul Torrance Professor and director of the Torrance Center for Creativity and Talent Development at the University of Georgia. “Creative people have the kind of curiosity that draws them toward learning new things and experiencing the world in new ways,” she said.
We can’t all be Thomas Edison or Maya Angelou. But creativity is also a state, and anyone can push themselves to be more creative. “Creativity is human capacity, and there’s always room for growth,” Runco said. A tolerant environment is often a necessary ingredient, he added. “Tolerant societies allow individuals to express themselves and explore new things. And as a parent or a teacher, you can model that creativity is valued and be open-minded when your child gives an answer you didn’t expect.”
One way to let your own creativity flow may be by tapping into your untethered mind. Seli is attempting to do so through his studies on hypnagogia. After pilot testing the idea on himself, he’s now working on a study that uses the sleep-tracking glove to explore creativity in a group of Duke undergrads. “In dream states, there seems to be connectivity between disparate ideas. You tend to link things together you normally wouldn’t, and this should lead to novel outcomes,” he said. “Neurally speaking, the idea is to increase connectivity between different areas of the brain.”
You don’t have to be asleep to forge those creative connections. Mind-wandering can also let the ideas flow. “Letting yourself daydream with a purpose, on a regular basis, might allow brain networks that don’t usually cooperate to literally form stronger connections,” Green said.
However, not all types of daydreams will get you there. Schooler found that people who engage in more personally meaningful daydreams (such as fantasizing about a future vacation or career change) report greater artistic achievement and more daily inspiration. People who are prone to fantastical daydreaming (such as inventing alternate realities or imaginary worlds) produced higher-quality creative writing in the lab and reported more daily creative behavior. But daydreams devoted to planning or problem-solving were not associated with creative behaviors ( Psychology of Aesthetics, Creativity, and the Arts , Vol. 15, No. 4, 2021).
It’s not just what you think about when you daydream, but where you are when you do it. Some research suggests spending time in nature can enhance creativity. That may be because of the natural world’s ability to restore attention, or perhaps it’s due to the tendency to let your mind wander when you’re in the great outdoors (Williams, K. J. H., et al., Journal of Environmental Psychology , Vol. 59, 2018). “A lot of creative figures go on walks in big, expansive environments. In a large space, your perceptual attention expands and your scope of thought also expands,” Kounios said. “That’s why working in a cubicle is bad for creativity. But working near a window can help.”
Wherever you choose to do it, fostering creativity requires time and effort. “People want the booster shot for creativity. But creativity isn’t something that comes magically. It’s a skill, and as with any new skill, the more you practice, the better you get,” Abraham said. In a not-yet-published study, she found three factors predicted peak originality in teenagers: openness to experience, intelligence, and, importantly, time spent engaged in creative hobbies. That is, taking the time to work on creative pursuits makes a difference. And the same is true for adults, she said. “Carve out time for yourself, figure out the conditions that are conducive to your creativity, and recognize that you need to keep pushing yourself. You won’t get to where you want to go if you don’t try.”
Those efforts can benefit your own sense of creative fulfillment and perhaps lead to rewards on an even grander scale. “I think everyday creativity is the most important kind,” Runco said. “If we can support the creativity of each and every individual, we’ll change the world.”
How to become more creative
1. Put in the work: People often think of creativity as a bolt of inspiration, like a lightbulb clicking on. But being creative in a particular domain—whether in the arts, in your work, or in your day-to-day life—is a skill. Carve out time to learn and practice.
2. Let your mind wander: Experts recommend “daydreaming with purpose.” Make opportunities to let your daydreams flow, while gently nudging them toward the creative challenge at hand. Some research suggests meditation may help people develop the habit of purposeful daydreaming.
3. Practice remote associations: Brainstorm ideas, jotting down whatever thoughts or notions come to you, no matter how wild. You can always edit later.
4. Go outside: Spending time in nature and wide-open spaces can expand your attention, enhance beneficial mind-wandering, and boost creativity.
5. Revisit your creative ideas: Aha moments can give you a high—but that rush might make you overestimate the merit of a creative idea. Don’t be afraid to revisit ideas to critique and tweak them later.
Further reading
Creativity: An introduction Kaufman, J. C., and Sternberg, R. J. (Eds.), Cambridge University Press, 2021
The eureka factor: Aha moments, creative insight, and the brain Kounios, J., & Beeman, M., Random House, 2015
Creativity anxiety: Evidence for anxiety that is specific to creative thinking, from STEM to the arts Daker, R. J., et al., Journal of Experimental Psychology: General , 2020
Predictors of creativity in young people: Using frequentist and Bayesian approaches in estimating the importance of individual and contextual factors Asquith, S. L., et al., Psychology of Aesthetics, Creativity, and the Arts , 2020
Recommended Reading
Contact apa, you may also like.
Research does solve real-world problems: experts must work together to make it happen
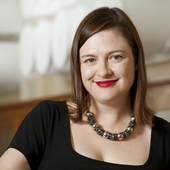
Deputy Vice Chancellor Research & Innovation, University of South Australia
Disclosure statement
Tanya Monro receives funding from the Australian Research Council. She is Deputy Vice Chancellor of the University of South Australia, a member of the Commonwealth Science Council, the CSIRO board, the SA Economic Development Board and Defence SA.
University of South Australia provides funding as a member of The Conversation AU.
View all partners
Generating knowledge is one of the most exciting aspects of being human. The inventiveness required to apply this knowledge to solve practical problems is perhaps our most distinctive attribute.
But right now we have before us some hairy challenges – whether that be figuring our how to save our coral reefs from warmer water , landing a human on Mars , eliminating the gap in life expectancy between the “haves” and “have-nots” or delivering reliable carbon-free energy .
It’s commonly said that an interdisciplinary approach is vital if we are to tackle such real world challenges. But what does this really mean?
Read more: It takes a community to raise a startup
Listen and read with care and you’ll start to notice that the words crossdisciplinary, multidisciplinary, interdisciplinary and transdisciplinary are used interchangeably. These words describe distinctly different ways of harnessing the power of disciplinary expertise to chart a course into the unknown.
In navigation, the tools and methods matter – choose differently and you’ll end up in a different spot. How we go about creating knowledge and solving problems really matters – it changes not only what questions can be asked and answered but fundamentally shapes what’s possible.
What is a discipline?
For centuries we have organised research within disciplines, and this has delivered extraordinary depths of knowledge.
But what is a discipline? It’s a shared language, an environment in which there’s no need to explain the motivation for one’s work, and in which people have a shared sense of what’s valuable.
For example, my background discipline is optical physics. I know what it’s like to be able to skip down the corridor and say,
“I’ve figured out how we can get broadband flat dispersion - we just need to tailor the radial profile!”
…and have people instantly not just know what I mean, but be able to add their own ideas and drive the work forward.
In long-established disciplines it’s often necessary to focus in a narrow area to be able to extend the limits of knowledge within the time-frame of a PhD. And while it’s rarely obvious at the time what benefits will flow from digging a little deeper, our way of life has been transformed over and over as result.
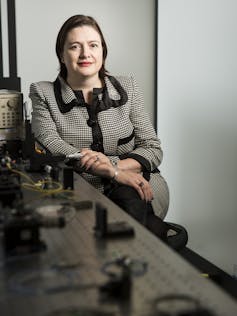
Disciplines focus talent and so can be amazingly efficient ways of generating knowledge. But they can also be extraordinarily difficult to penetrate from the outside without understanding that discipline’s particular language and shared values.
The current emphasis on real-world impact has sharpened awareness on the need to translate knowledge into outcomes. It has also brought attention to the critical role partnerships with industry and other end-users of research play in this process.
Creating impact across disciplines
Try to solve a problem with the tools of a single discipline alone, and it’s as if you have a hammer - everything starts to look like a nail. It’s usually obvious when expertise from more than one discipline is needed.
Consider a panel of experts drawn from different fields to each apply the tools of their field to a problem that’s been externally framed. This has traditionally been how expertise from the social sciences is brought to bear on challenges in public health or the environment.
This is a crossdisciplinary approach , which can produce powerful outcomes provided that those who posed the question are positioned to make decisions based on the knowledge generated. But the research fields themselves are rarely influenced by this glancing encounter with different approaches to knowledge generation.
Multidisciplinary research involves the application of tools from one discipline to questions from other fields. An example is the application of crystallography, discovered by the Braggs, to unravel the structure of proteins . This requires concepts to transfer across domains, sometimes in real time but usually with a lag of years or decades.
Read more: If we really want an ideas boom, we need more women at the top tiers of science
Interdisciplinary research happens when researchers from different fields come together to pose a challenge that wouldn’t be possible in isolation. One example is the highly transparent optical fibres that underpin intercontinental telecommunication networks.
The knowledge creation that made this possible involved glass chemists, optical physicists and communication engineers coming together to articulate the possible, and develop the shared language required to make it a reality. When fields go on this journey together over decades, new fields are born.
In this example the question itself was clear – how can we harness the transparency of silica glass to create optical transmission systems that can transport large volumes of data over long distances?
But what about the questions we don’t know how to pose because without knowledge of another field we don’t know what’s possible? This line of reasoning leads us into the domain of transdisciplinary research .
Transdisciplinary research requires a willingness to craft new questions – whether because they were considered intractable or because without the inspiration from left field they simply didn’t arise. An example of this is applying photonics to IVF incubators - the idea that it could be possible to “listen” to how embryos experience their environment is unlikely to have arisen without bringing these fields together.
Read more: National Science Statement a positive gesture but lacks policy solutions: experts
In my own field, physics, I discovered that when talking to people from other areas the simple question “what would you like to measure?” quickly led to uncharted territory.
Before long we were usually, together, posing fundamentally new questions and establishing teams to tackle them. This can be scary territory but it’s tremendously rewarding and creates space for creativity and the emergence of disruptive technologies.

Excellence, communication, co-location, funding
One of the best ways of getting out of a disciplinary silo is to take every opportunity to talk to others outside your field. Disciplinary excellence is the starting point to get to the table.
And while disciplinary collaborations can flourish over large distances because they share a language and values, it’s usually true that once you mix disciplines co-location becomes a real asset. Then of course there are the questions of how we fund and organise research concentrations to allow inter- and transdisciplinary research to flourish.
With the increased emphasis on impact, these questions are becoming ever more pressing. Organisations that get this right will thrive.
- Research impact
- cross-disciplinary

Senior Laboratory Technician

Manager, Centre Policy and Translation

Newsletter and Deputy Social Media Producer

College Director and Principal | Curtin College

Head of School: Engineering, Computer and Mathematical Sciences

- SUGGESTED TOPICS
- The Magazine
- Newsletters
- Managing Yourself
- Managing Teams
- Work-life Balance
- The Big Idea
- Data & Visuals
- Reading Lists
- Case Selections
- HBR Learning
- Topic Feeds
- Account Settings
- Email Preferences
Are You Solving the Right Problem?
- Dwayne Spradlin
Most firms aren’t, and that undermines their innovation efforts.
Reprint: R1209F
The rigor with which a problem is defined is the most important factor in finding a good solution. Many organizations, however, are not proficient at articulating their problems and identifying which ones are crucial to their strategies.
They may even be trying to solve the wrong problems—missing opportunities and wasting resources in the process. The key is to ask the right questions.
The author describes a process that his firm, InnoCentive, has used to help clients define and articulate business, technical, social, and policy challenges and then present them to an online community of more than 250,000 solvers. The four-step process consists of asking a series of questions and using the answers to create a problem statement that will elicit novel ideas from an array of experts.
- Establish the need for a solution. What is the basic need? Who will benefit from a solution?
- Justify the need. Why should your organization attempt to solve this problem? Is it aligned with your strategy? If a solution is found, who will implement it?
- Contextualize the problem. What have you and others already tried? Are there internal and external constraints to implementing a solution?
- Write the problem statement. What requirements must a solution meet? What language should you use to describe the problem? How will you evaluate solutions and measure success?
EnterpriseWorks/VITA, a nonprofit organization, used this process to find a low-cost, lightweight, and convenient product that expands access to clean drinking water in the developing world.
“If I were given one hour to save the planet, I would spend 59 minutes defining the problem and one minute resolving it,” Albert Einstein said.

- DS Dwayne Spradlin is the president and CEO of InnoCentive , an online marketplace that connects organizations with freelance problem solvers in a multitude of fields. He is a coauthor, with Alpheus Bingham, of The Open Innovation Marketplace: Creating Value in the Challenge Driven Enterprise (FT Press, 2011).
Partner Center
March 1, 2014
Why Your First Idea Can Blind You to a Better One
While we are working through a problem, the brain’s tendency to stick with familiar ideas can literally blind us to superior solutions
By Merim Bilalić & Peter McLeod
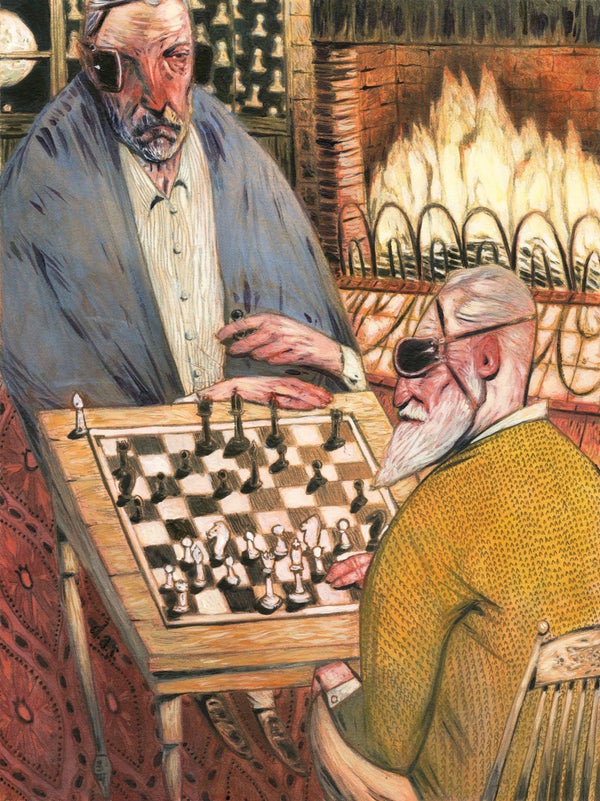
Danny Schwartz
I n a classic 1942 experiment, American psychologist Abraham Luchins asked volunteers to do some basic math by picturing water jugs in their mind. Given three empty containers, for example, each with a different capacity—21, 127 and three units of water—the participants had to figure out how to transfer liquid between the containers to measure out precisely 100 units. They could fill and empty each jug as many times as they wanted, but they had to fill the vessels to their limits. The solution was to first fill the second jug to its capacity of 127 units, then empty it into the first to remove 21 units, leaving 106, and finally to fill the third jug twice to subtract six units for a remainder of 100. Luchins presented his volunteers with several more problems that could be solved with essentially the same three steps; they made quick work of them. Yet when he gave them a problem with a simpler and faster solution than the previous tasks, they failed to see it.
This time Luchins asked the participants to measure out 20 units of water using containers that could hold 23, 49 and three liquid units. The solution is obvious, right? Simply fill the first jug and empty it into the third one: 23 – 3 = 20. Yet many people in Luchins’s experiment persistently tried to solve the easier problem the old way, emptying the second container into the first and then into the third twice: 49 – 23 – 3 – 3 = 20. And when Luchins gave them a problem that had a two-step solution but could not be solved using the three-step method to which the volunteers had become accustomed, they gave up, saying it was impossible.
The water jug experiment is one of the most famous examples of the Einstellung effect: the human brain’s dogged tendency to stick with a familiar solution to a problem—the one that first comes to mind—and to ignore alternatives. Often this type of thinking is a useful heuristic. Once you have hit on a successful method to, say, peel garlic, there is no point in trying an array of different techniques every time you need a new clove. The trouble with this cognitive shortcut, however, is that it sometimes prevents people from seeing more efficient or appropriate solutions than the ones they already know.
On supporting science journalism
If you're enjoying this article, consider supporting our award-winning journalism by subscribing . By purchasing a subscription you are helping to ensure the future of impactful stories about the discoveries and ideas shaping our world today.
Building on Luchins’s early work, psychologists replicated the Einstellung effect in many different laboratory studies with both novices and experts exercising a range of mental abilities, but exactly how and why it happened was never clear. About 15 years ago, by recording the eye movements of highly skilled chess players, we solved the mystery. It turns out that people under the influence of this cognitive shortcut literally do not see certain details in their environment that could provide them with a more effective solution. Research also suggests that many different cognitive biases discovered by psychologists over the years—those in the courtroom and the hospital, for instance—are in fact variations of the Einstellung effect.
Back to Square One
Since at least the early 1990s, psychologists have studied the Einstellung effect by recruiting chess players of varying skill levels, from amateur to grand master. In such experiments, researchers have presented players with specific arrangements of chess pieces on virtual chessboards and asked them to achieve a checkmate in as few moves as possible. Our own studies, for instance, provided expert chess players with scenarios in which they could accomplish a checkmate using a well-known sequence called smothered mate. In this five-step maneuver, the queen is sacrificed to draw one of the opponent’s pieces onto a square to block off the king’s escape route. The players also had the option to checkmate the king in just three moves with a much less familiar sequence. As in Luchins’s water jug studies, most of the players failed to find the more efficient solution.
During some of these studies, we asked the players what was going through their mind. They said they had found the smothered mate solution and insisted they were searching for a shorter one, to no avail. But the verbal reports offered no insight into why they could not find the swifter solution. In 2007 we decided to try something a little more objective: tracking eye movements with an infrared camera. Which part of the board people looked at and how long they looked at different areas would unequivocally tell us which aspects of the problem they were noticing and ignoring.
In this experiment, we followed the gaze of five expert chess players as they examined a board that could be solved either with the longer smothered mate maneuver or with the shorter three-move sequence. After an average of 37 seconds, all the players insisted that the smothered mate was the speediest possible way to corner the king. When we presented them with a board that could be solved only with the three-sequence move, however, they found it with no problem. And when we told the players that this same swift checkmate had been possible in the previous chessboard, they were shocked. “No, it is impossible,” one player exclaimed. “It is a different problem; it must be. I would have noticed such a simple solution.” Clearly, the mere possibility of the smothered mate move was stubbornly masking alternative solutions. In fact, the Einstellung effect was powerful enough to temporarily lower expert chess masters to the level of much weaker players.
The infrared camera revealed that even when the players said they were looking for a faster solution—and indeed believed they were doing so—they did not actually shift their gaze away from the squares they had already identified as part of the smothered mate move. In contrast, when presented with the one-solution chessboard, players initially looked at the squares and pieces important for the smothered mate and, once they realized it would not work, directed their attention toward other squares and soon hit on the shorter solution.
Basis for Bias
In 2013 Heather Sheridan, now at the University of Albany, and Eyal M. Reingold of the University of Toronto published studies that corroborate and complement our eye-tracking experiments. They presented 17 novice and 17 expert chess players with two different situations. In one scenario, a familiar checkmate maneuver such as the smothered mate was advantageous but second best to a distinct and less obvious solution. In the second situation, the more familiar sequence would be a clear blunder. As in our experiments, once amateurs and master chess players locked onto the helpful familiar maneuver, their eyes rarely drifted to squares that would clue them in to the better solution. When the well-known sequence was obviously a mistake, however, all the experts (and most of the novices) detected the alternative.
Credit: George Retseck
The Einstellung effect is by no means limited to controlled experiments in the lab or even to mentally challenging games such as chess. Rather it is the basis for many cognitive biases. English philosopher, scientist and essayist Francis Bacon was especially eloquent about one of the most common forms of cognitive bias in his 1620 book Novum Organum: “The human understanding when it has once adopted an opinion … draws all things else to support and agree with it. And though there be a greater number and weight of instances to be found on the other side, yet these it either neglects or despises, or else by some distinction sets aside and rejects…. Men … mark the events where they are fulfilled, but where they fail, though this happen much oftener, neglect and pass them by. But with far more subtlety does this mischief insinuate itself into philosophy and the sciences, in which the first conclusion colours and brings into conformity with itself all that comes after.”
In the 1960s English psychologist Peter Wason gave this particular bias a name: “confirmation bias.” In controlled experiments, he demonstrated that even when people attempt to test theories in an objective way, they tend to seek evidence that confirms their ideas and to ignore anything that contradicts them.
In The Mismeasure of Man , for example, Stephen Jay Gould of Harvard University reanalyzed data cited by researchers trying to estimate the relative intelligence of different racial groups, social classes and sexes by measuring the volumes of their skulls or weighing their brains, on the assumption that intelligence was correlated with brain size. Gould uncovered massive data distortion. On discovering that French brains were on average smaller than their German counterparts, French neurologist Paul Broca explained away the discrepancy as a result of the difference in average body size between citizens of the two nations. After all, he could not accept that the French were less intelligent than the Germans. Yet when he found that women’s brains were smaller than those in men’s noggins, he did not apply the same correction for body size, because he did not have any problem with the idea that women were less intelligent than men.
Somewhat surprisingly, Gould concluded that Broca and others like him were not as reprehensible as we might think. “In most cases discussed in this book we can be fairly certain that biases … were unknowingly influential and that scientists believed they were pursuing unsullied truth,” Gould wrote. In other words, just as we observed in our chess experiments, comfortably familiar ideas blinded Broca and his contemporaries to the errors in their reasoning. Here is the real danger of the Einstellung effect. We may believe that we are thinking in an open-minded way, completely unaware that our brain is selectively directing attention away from aspects of our environment that could inspire new thoughts. Any data that do not fit the solution or theory we are already clinging to are ignored or discarded.
The surreptitious nature of confirmation bias has unfortunate consequences in everyday life, as documented in studies on decision-making among doctors and juries. In a review of errors in medical thought, physician Jerome Groopman noted that in most cases of misdiagnosis, “the doctors didn’t stumble because of their ignorance of clinical facts; rather, they missed diagnoses because they fell into cognitive traps.” When doctors inherit a patient from another doctor, for example, the first clinician’s diagnosis can block the second from seeing important and contradictory details of the patient’s health that might change the diagnosis. It is easier to just accept the diagnosis—the “solution”—that is already in front of them than to rethink the entire situation. Similarly, radiologists examining chest x-rays often fixate on the first abnormality they find and fail to notice further signs of illness that should be obvious, such as a swelling that could indicate cancer. If those secondary details are presented alone, however, radiologists see them right away.
Related studies have revealed that jurors begin to decide whether someone is innocent or guilty long before all the evidence has been presented. In addition, their initial impressions of the defendant change how they weigh subsequent evidence and even their memory of evidence they saw before. Likewise, if an interviewer finds a candidate to be physically attractive, he or she will automatically perceive that person’s intelligence and personality in a more positive light, and vice versa. These biases, too, are driven by the Einstellung effect. It is easier to make a decision about someone if one maintains a consistent view of that person rather than sorting through contradictory evidence.
Can we learn to resist the Einstellung effect? Perhaps. In our chess experiments and the follow-up experiments by Sheridan and Reingold, some exceptionally skilled experts, such as grand masters, did in fact spot the less obvious optimal solution even when a slower but more familiar sequence of moves was possible. This suggests that the more expertise someone has in their field—whether chess, science or medicine—the more immune they are to cognitive bias.
But no one is completely impervious; even the grand masters failed when we made the situation tricky enough. Actively remembering that you are susceptible to the Einstellung effect is another way to counteract it. When considering the evidence on, say, the relative contribution of human-made and naturally occurring greenhouse gases to global temperature, remember that if you already think you know the answer, you will not judge the evidence objectively. Instead you will notice evidence that supports the opinion you already hold, evaluate it as stronger than it really is and find it more memorable than evidence that does not support your view.
We must try to learn to accept our errors if we sincerely want to improve our ideas. English naturalist Charles Darwin came up with a remarkably simple and effective technique to do just this. “I had … during many years, followed a golden rule, namely, that whenever a published fact, a new observation or thought came across me, which was opposed by my general results, to make a memorandum of it without fail and at once,” he wrote. “For I had found by experience that such facts and thoughts were far more apt to escape from memory than favourable ones.”
After 10 Years, ‘Many Labs’ Comes to an End – But Its Success Is Replicable
May 23, 2022 • By Eric Williamson, [email protected] Eric Williamson, [email protected]
- Eric Williamson, [email protected]

Photo illustration by Sako Yamaguchi, University Communications
Brian Nosek, a professor of psychology at the University of Virginia who studies human biases, was a graduate student in the 1990s when he first started thinking closely about one of science’s open secrets: failure to replicate research findings is a common occurrence.
“At that time, the textbooks had been talking for 30 years about the problem,” Nosek said. “I thought, ‘What’s going on here? Is there actually a problem?’”
He couldn’t have known then that this fascination would someday make him one of the most hated figures in science, at least for a while, and also one of the most respected.
Replication is integral to the scientific method. It’s the lather-rinse-repeat approach that either increases the likelihood that a theory holds legitimacy, or forces reconsideration. If results fail to replicate, was something off in the experimental process? If not, are the conclusions wrong?
But with careers and grant monies so often in the balance, the incentives align with generating new discoveries over running old experiments repeatedly.
“Replication is a core concept of science, and yet it’s not very often practiced,” said Nosek, who is also co-founder and executive director of the independent nonprofit Center for Open Science, which advocates for transparency in research methods and data. “Innovation without verification gets us nowhere. But any individual researcher has choices to make about how they spend their time. The answer is pretty easy when thinking about career advancement: Try something new.”
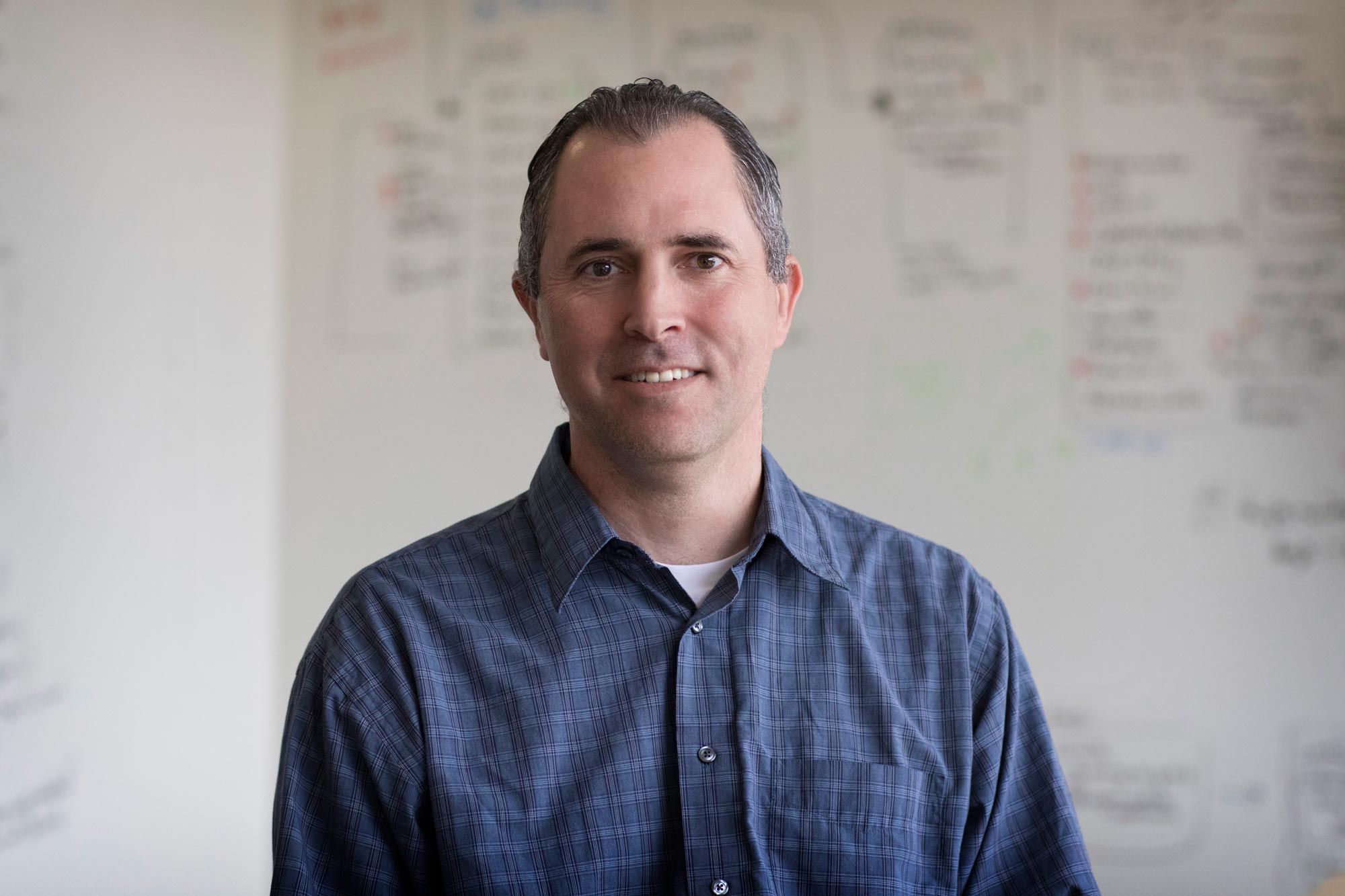
UVA psychology professor Brian Nosek is an expert in human biases, and co-founder and executive director of the independent Center for Open Science. (Photo by Dan Addison, University Communications)
Ten years ago, mindful of the trend of research being increasingly generated, but not increasingly revisited, Nosek and colleagues decided to re-run a series of published scientific experiments, creating the “Many Labs” project. The global effort, which at times has been both headline-grabbing and apple cart-turning, wrapped up at the end of April.
“It’s hard to overstate how central Brian Nosek’s role in the reform movement, sometimes called the ‘replication crisis’ or ‘credibility revolution,’ has been,” commented Simine Vazire, a professor at the University of Melbourne who studies psychology ethics and science’s ability to self-correct. “Brian has been a leader in the movement, a diplomat reaching out across sharp dividing lines in our field, and someone who gets things done.
“Among the many projects that Nosek and the Center for Open Science made possible, the Many Labs projects, which Brian collaborated on, but which were individually led by Richard Klein and Charlie Ebersole, are among the most impressive and important for science. Each of these five projects was a gargantuan effort, and tackled a question that had been the topic of much debate, but virtually no empirical tests.”
‘#Repligate’ Lights Up the Scientific Community
The Many Labs revolution has a complex history, with Nosek running similar projects simultaneously. He began his initial Many Labs replication studies in 2011. It wasn’t long before the scientific community was feeling “twitchy,” The Guardian reported.
Nosek reflected, “Because performing replication was so unusual, when someone said, ‘I want to replicate your study,’ instead of the person taking it as a compliment, it was often considered a threat. Like, ‘What, you don’t trust me? What’s up?’”
He thinks of 2012 as when Many Labs officially started, however. That’s when the journal Social Psychology accepted his and Dutch researcher Daniël Lakens’ pitch to serve as guest editors for a special issue with a unique approach. They would invite researchers to submit proposed replications and put the designs through peer review before knowing the results, so that no one would be biased for or against the studies based on the outcomes.
The first Many Labs project was one of the papers in this special issue, and its approach was slightly different: It tested the replicability of 13 classic and contemporary studies.
Many Labs 1 (Klein et al., 2014) was part of a special issue of Social Psychology that introduced Registered Reports edited by @lakens and I. The issue spawned the infamous #repligate , summarized here: https://t.co/xNSPRaZii4 — Brian Nosek (@BrianNosek) April 29, 2022
A sampling of the inquiries for that component included: Can people’s behavior be “primed” by visual cues? Would a quote be perceived differently if someone thought it came from Thomas Jefferson versus Soviet founder Vladimir Lenin? Can a chance observation – in this case a dice roll – influence thoughts about what might have happened prior?
Overall, the crowd-sourced Many Labs experiments spanned 36 independent research sites, utilizing a total of 6,344 participants.
Recognizing the depth of the challenge, Nosek embarked with a simple design.
“With Lab 1, we wanted to look at a number of different findings in an easy-to-transfer protocol,” Nosek said. Using a combination of online and in-person methods, “We chose research findings in which the study and procedure could be done in 1 to 3 minutes.”
At first blush, the results might not have seemed revolutionary. There was arguably good news: 10 of the 13 studies replicated their original findings, a much higher percentage than Nosek and colleagues might have expected.
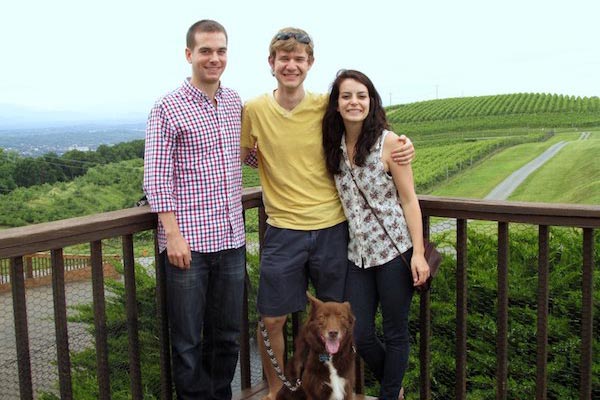
Rick Klein (leader of Many Labs 1, 2, and 4), Charlie Ebersole (leader of Many Labs 3 and 5), and Olivia Atherton (leader of Many Labs 3) enjoy some down time from the research. (Contributed photo)
But that was bad news for the remaining three studies. There was weak support for one theory, that imagining contact with someone can reduce prejudice, as well as in general for the theory of “priming,” which was integral to the other two studies.
In one of the two, participants were shown a subtle image of an American flag to see if it would influence their sense of conservatism. No strong evidence could be found for the visual cue having an effect on their subsequent behavior. With priming having previously been a well-accepted theory, social media users wagged their tongues at this and related findings from the special issue, often flagging the conversation as “#repligate.”
Nosek, with 269 collaborators, followed up Many Labs the next year with the concurrent research titled “The Reproducibility Project: Psychology.”
While not formally part of Many Labs, the work continued its themes. The researchers conducted replication attempts on research published in three journals in 2008. They were only able to reproduce results in fewer than 50 of the 100 cases – worse odds than flipping a coin.
The marquee observation was that, ironically, 97 of the original studies claimed significant effects. Even the studies that did successfully replicate didn’t usually do so with as much oomph as originally touted. In a few worst-case scenarios that were realized, the new researchers even found effects that were opposite of the initial findings.

Does loneliness amplify a belief in the supernatural? Maybe, but researchers couldn’t confirm a connection. The same goes for finding causation between racial prejudice and how people responded to pictures of ethnically diverse people with guns.
As the new research dropped, The Atlantic described Nosek as a “respected” and “level-headed figure” who expressed no glee in the outcomes. In fact, when he found, oddly, that the cognitive psychology experiments (about how we think) were about twice as likely to replicate as the social psychology experiments (involving how people influence each other), Nosek cringed for fear of scientific in-fighting.
If people were talking, though, that was what mattered most, he told himself.
“Little of the criticism I received suggested that I was doing something misaligned with my principles or values,” he said. ‘Most of the criticism was that I might embarrass the field or that I am incompetent. The possibility that the work might illuminate something embarrassing only reinforced my view of the importance of doing the research. If it revealed something embarrassing, all the more important that we know about it to fix it.”
“I never felt any conflict about which path to take.”
Prior to replication studies like his, potentially flawed research was only whispered about at conferences, he said. Now, disparate findings were being discussed openly. He and his many collaborators carefully prepared every word of their reports with an understanding of the many sensitivities. Yet even so, Nosek was both the hero and the goat for leading the revolution.
“Getting criticized and losing friends was not fun, at all,” he said. “For me, this was a situation in which doing the right thing and doing the popular thing were sometimes in conflict. I never felt any conflict about which path to take.”
But what about any conflicts in how he orchestrated the work?
Recall Nosek’s expertise: biases. While the importance of the initial findings focused on where scientists “could” be wrong, Nosek was also obsessed with where his efforts could stray – including his own potentially unrealized, or “implicit,” biases in setting up Many Labs. This awareness set the stage for the labs to come.
Many Labs 2-5: ‘Many’ More Takeaways
Failures to replicate could represent flaws in either the original experiments or on the part of the Many Labs, so Nosek wanted to take any criticism he received seriously. There were notions that some research didn’t replicate because of geographical differences on the part of participants. Others pointed to the time of year. College students are often targeted to take part in research for the obvious reason of their accessibility to university researchers. One assumption on the part of critics was that maybe an exams period, for example, could throw the results.
“It seemed wise to lean into the areas of debate.”
“The basic idea was each time we started a new Many Lab, the most important thing we couldn’t resolve in the last one would become an important feature in the next one,” Nosek said. “It seemed wise to lean into the areas of debate.”
So Lab 2 added more types of testing sites in different cultural contexts around the world. Lab 3 adjusted the times during the semester that the researchers conducted the experiments.
While Nosek acknowledged that culture can have an impact on how a research participant responds, culture couldn’t account for whether a finding replicated successfully or not. Similarly, the time of the semester didn’t seem to matter.
The key outcomes of ML3 were (a) oh boy, more findings seem harder to replicate than we thought, and (b) with good coordination and materials sharing, it is possible to do a follow-up replication with 1200+ participants across 10 labs in a few months. — Brian Nosek (@BrianNosek) April 29, 2022
Next, Labs 4 and 5 placed an emphasis on shoring up any potential variability from the original experimental design, including by allowing original authors to have greater participation in the replication efforts. Failure to truly understand the original experimental process, because those duplicating it weren’t “the” experts, was a legitimate possibility for failure to replicate.
But every scientist also knew that “p-hacking” was another possibility.
“The ‘p’ refers to a statistical outcome used in most science to decide whether an observed finding is unlikely to have occurred by chance,” Nosek explained. “Researchers are motivated to find low p-values so that they can claim to have discovered something. But, in ordinary practice, researchers have flexibility in the decisions they make when they analyze their data, which might erroneously increase the occurrence of low p-values.”
Nosek’s academic career started by investigating the concept of implicit bias: how people might inadvertently behave in ways that are misaligned with their intentions and ethics.
And he’s studied a number of these biases – including the public belief that if science has a gender, it is probably male .
The bias is often characterized by a lack of self-awareness. So, in theory, a self-respecting scientist could both be appalled at the thought that she would cherry-pick evidence, and yet still be guilty of it.
“The other major criticism is: ‘You guys are just idiots.’”
There are other possibilities in a hypothetical laundry list of scientific errors, but Nosek said introspection is required as the process of elimination continues to scratch them off. He himself never rules out the possibility of his own incompetence being the reason that he failed to replicate others’ findings. After all, how would an incompetent person know?
“The other major criticism is: ‘You guys are just idiots,’” he said. “We might be idiots, but we constructed our replication studies to minimize the likelihood of that by getting materials and advice from original authors in advance, by preregistering our design and analysis plan, and by transparently reporting all of our findings and data.
“Also, in a couple investigations, we directly tested whether including more of the original authors’ expertise in the design of the research would improve replicability, and it did not. We may well be idiots, but – outside of assertions by my teenage daughters – we haven’t yet found any evidence to support that.”
The Long Tail of the Many Labs Approach
Over its decade, the sprawling Many Labs endeavor generated five major papers, extending the evidence provided by The Reproducibility Project: Psychology, and put numerous theories to the test. One, called terror management theory, which asserts that human beings embrace certain mindsets in order to tamp down the fear of death, didn’t fare well in new trials. Another, a Nobel Prize-winning theory positing that losses loom larger in people’s minds than gains, seemed to hold up.
But, more importantly, Many Labs put to bed assumptions.
“There had been a lot of talk about how psychological findings are very sensitive to these kinds of variations in contexts, and leaders in the field argued, sometimes in the pages of The New York Times, that this explains why replications often fail,” said Vazire, of the University of Melbourne. “This was meant as a defense of the field of psychology – the idea was that failed replications do not represent a symptom of a serious problem, but are to be expected even when research is conducted to the highest standards. But all of these claims were based on speculation – no one bothered to test whether these contextual factors actually matter, and no one had bothered to check whether experts, who supposedly know just the right conditions that should produce the effect, can actually get the effects to replicate.”
“There is a lot still to do, but there is a strong community spirit to improve.”
Lab 5’s results on terror management, published April 29, marked the end of the Many Labs component of Nosek’s replication work. “That program of research accomplished what I wanted to accomplish,” he said. “Time to move on to bigger and better things that build on it.”
But instead of being punctuated by a period, the experiments ended with an ellipsis.
“It has been incredibly gratifying to see a dozen other groups form and adapt the Many Labs concept in their own area of research,” Nosek said. “There are many ‘Manys’ now – Many Babies , Many Smiles , Many Dogs , Many Birds , Many EEGs , etc. Each of those projects are examining replicability of findings in their fields with big collaborative teams. It is very gratifying to see communities form and advance this approach across the scientific community.”
Congratulations & thank you to everyone that contributed to the Many Labs projects over the last 10 years, especially @raklein3 & @CharlieEbersole for their leadership. The "Many Labs" series may be ending, but there is so much interesting work now that builds on this foundation. — Brian Nosek (@BrianNosek) April 29, 2022
The conversation about how much trust the public has, or should have, in published research also continues – as does the hard work of a community focusing inward.
“There is a lot still to do,” he said, “but there is a strong community spirit to improve, and tangible results indicating that some of the reforms are working.”
Journals have gotten more discerning in what they accept, as have some grant providers in what they look for methodologically, Nosek noted. Many Labs helped lead that discussion with its “predictions markets,” in which researchers attempted to prognosticate the ability to replicate, based on their relative confidence in the stated approaches.
“For me, the most important thing is for the research to be transparent and open.”
They were right 71% of the time. Red flags might be if the methods are vague, for example, or if it appears as if only the original researchers would have the know-how to reproduce the results.
Unfortunately, “There is no one thing that clarifies whether a finding is trustworthy or not,” he said. “Research is hard; lots of things can go wrong. For me, the most important thing is for the research to be transparent and open. We can’t expect to get everything right, but we can expect that researchers will show each other how they got to their claims, so that productive critique will occur to reveal error and accelerate progress.”
Nosek earned his master’s and doctoral degrees in psychology from Yale University, after obtaining his bachelor’s degrees from California Polytechnic State University in psychology, women’s studies and computer science. Over his illustrious career, he co-developed the Implicit Association Test, a method that advanced research and public interest in implicit bias. In addition to co-founding the Center for Open Science, he also co-founded two other nonprofit organizations: Project Implicit, and the Society for the Improvement of Psychological Science.
I, like many I’m sure, read at least one of these papers and had my research focus flipped on it’s head. How many people can say they’ve had that kind of impact on the field? Kudos to all involved. https://t.co/0w5eHIOAdE — John Mills (@drjpmills) April 30, 2022
In 2018, the American Association for the Advancement of Science named Nosek a fellow. The group cited him for inclusion with this language: “In the field of psychology, for unsurpassed leadership in the quest to improve openness, integrity, and reproducibility in research, not only for psychological science, but for all of science.”
He’s currently working on a new replication-related project that would use algorithms to provide an early indicator of whether a finding is credible. “Initial evidence is promising,” he said. “And, if it works, it could help to direct attention toward findings that are interesting, but need more work to determine if they are credible.”
So, in the end, how excited should any of us get about the latest trumpeted discovery?
Nosek said new possibilities are always worthy of cautious enthusiasm, but “we should simultaneously recognize that it is uncertain, and be eager to support the follow-on research to assess its reliability and validity.”
The Complete List of Many Labs Replication Studies, Psychology
A Critique of the Many Labs Projects
Related Research
The Reproducibility Project: Psychology
The Reproducibility Project: Cancer Biology
Media Contact
Eric Williamson
Communications Manager School of Engineering and Applied Science
[email protected] (434) 924-1321
Article Information
August 15, 2024
You May Also Like

Want To Better Connect With Your iPhone? Use a Skin Softener

Should You Be ‘Hope Scrolling?’ This Researcher Says It’s Not a Bad Idea
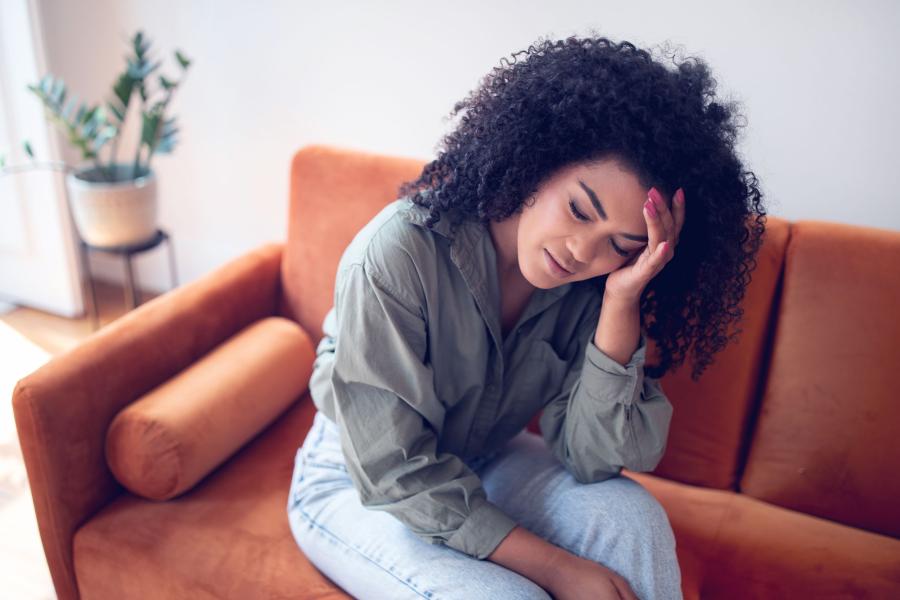
Hormone Receptors in the Brain Could Be a Target To Ease Migraines
Module 1: Research and the Writing Process
The seven steps of the research process.
The following seven steps outline a simple and effective strategy for finding information for a research paper and documenting the sources you find. Depending on your topic and your familiarity with the library, you may need to rearrange or recycle these steps. Adapt this outline to your needs.
Step 1: Identify and Develop Your Topic
State your topic idea as a question. For example, if you are interested in finding out about use of alcoholic beverages by college students, you might pose the question, “What effect does use of alcoholic beverages have on the health of college students?” Identify the main concepts or keywords in your question. In this case they are alcoholic beverages, health, and college students.
Step 2: Find Background Information
After you identify your research topic and some keywords that describe it, find and read articles in subject encyclopedias, dictionaries, and handbooks. These articles will help you understand the context (historical, cultural, disciplinary) of your topic. They are the foundation supporting further research. The most common background sources are subject encyclopedias and dictionariesfrom our print and online reference collection. Class textbooks also provide definitions of terms and background information.
Look up your keywords in the indexes to subject encyclopedias. Read articles in these encyclopedias to set the context for your research. Note any relevant items in the bibliographies at the end of the encyclopedia articles. Additional background information may be found in your lecture notes, textbooks, and reserve readings.
Tip: Exploit Bibliographies
- Read the background information and note any useful sources (books, journals, magazines, etc.) listed in the bibliography at the end of the encyclopedia article or dictionary entry. The sources cited in the bibliography are good starting points for further research.
- Look up these sources in our catalogs and periodical databases. Check the subject headings listed in the subject field of the online record for these books and articles. Then do subject searches using those subject headings to locate additional titles.
- Remember that many of the books and articles you find will themselves have bibliographies. Check these bibliographies for additional useful resources for your research.
By using this technique of routinely following up on sources cited in bibliographies, you can generate a surprisingly large number of books and articles on your topic in a relatively short time.
Step 3: Use Catalogs to Find Books and Media
Use guided keyword searching to find materials by topic or subject. Print or write down the citation (author, title,etc.) and the location information (call number and library). Note the circulation status. When you pull the book from the shelf, scan the bibliography for additional sources. Watch for book-length bibliographies and annual reviews on your subject; they list citations to hundreds of books and articles in one subject area.
Step 4: Use Indexes to Find Periodical Articles
Use periodical indexes and abstracts to find citations to articles. The indexes and abstracts may be in print or computer-based formats or both. Choose the indexes and format best suited to your particular topic; ask at the reference desk of your library if you need help figuring out which index and format will be best.
You can find periodical articles by the article author, title, or keyword by using periodical indexes. If the full text is not linked in the index you are using, write down the citation from the index and search for the title of the periodical in your library’s catalog.
Step 5: Find Internet Resources
Use search engines. Check to see if your class has a bibliography or research guide created by librarians. Some search tools include:
- Search Engines – Comparison table of recommended search engines; how search engines work
- Subject Directories – Table comparing some of the best human-selected collections of web pages
- Meta-Search Engines – Use at your own risk: not recommended as a substitute for directly using search engines
- Invisible Web – What it is, how to find it, and its inherent ambiguity (searchable databases on the Web)
Step 6: Evaluate What You Find
Critically analyzing information sources, initial appraisal.
- What are the author’s credentials–institutional affiliation (where he or she works), educational background, past writings, or experience? Is the book or article written on a topic in the author’s area of expertise? You can use the various Who’s Who publications for the U.S. and other countries and for specific subjects and the biographical information located in the publication itself to help determine the author’s affiliation and credentials.
- Has your instructor mentioned this author? Have you seen the author’s name cited in other sources or bibliographies? Respected authors are cited frequently by other scholars. For this reason, always note those names that appear in many different sources.
- Is the author associated with a reputable institution or organization? What are the basic values or goals of the organization or institution?
Date of Publication
- When was the source published? This date is often located on the face of the title page below the name of the publisher. If it is not there, look for the copyright date on the reverse of the title page. On Web pages, the date of the last revision is usually at the bottom of the home page, sometimes every page.
- Is the source current or out-of-date for your topic? Topic areas of continuing and rapid development, such as the sciences, demand more current information. On the other hand, topics in the humanities often require material that was written many years ago. At the other extreme, some news sources on the Web now note the hour and minute that articles are posted on their site.
Edition or Revision
Is this a first edition of this publication or not? Further editions indicate a source has been revised and updated to reflect changes in knowledge, include omissions, and harmonize with its intended reader’s needs. Also, many printings or editions may indicate that the work has become a standard source in the area and is reliable. If you are using a Web source, do the pages indicate revision dates?
Note the publisher. If the source is published by a university press, it is likely to be scholarly. Although the fact that the publisher is reputable does not necessarily guarantee quality, it does show that the publisher may have high regard for the source being published.
Title of Journal
Is this a scholarly or a popular journal? This distinction is important because it indicates different levels of complexity in conveying ideas. If you need help in determining the type of journal, see Distinguishing Scholarly from Non-Scholarly Periodicals . Or you may wish to check your journal title in the latest edition of Katz’s Magazines for Libraries (Olin Ref Z 6941 .K21, shelved at the reference desk) for a brief evaluative description.
Critical Analysis of the Content
Content analysis.
Having made an initial appraisal, you should now examine the body of the source. Read the preface to determine the author’s intentions for the book. Scan the table of contents and the index to get a broad overview of the material it covers. Note whether bibliographies are included. Read the chapters that specifically address your topic. Reading the article abstract and scanning the table of contents of a journal or magazine issue is also useful. As with books, the presence and quality of a bibliography at the end of the article may reflect the care with which the authors have prepared their work.
Intended Audience
What type of audience is the author addressing? Is the publication aimed at a specialized or a general audience? Is this source too elementary, too technical, too advanced, or just right for your needs?
Objective Reasoning
- Is the information covered fact, opinion, or propaganda? It is not always easy to separate fact from opinion. Facts can usually be verified; opinions, though they may be based on factual information, evolve from the interpretation of facts. Skilled writers can make you think their interpretations are facts.
- Does the information appear to be valid and well-researched, or is it questionable and unsupported by evidence? Assumptions should be reasonable. Note errors or omissions.
- Are the ideas and arguments advanced more or less in line with other works you have read on the same topic? The more radically an author departs from the views of others in the same field, the more carefully and critically you should scrutinize his or her ideas.
- Is the author’s point of view objective and impartial? Is the language free of emotion-arousing words and bias?
- Does the work update other sources, substantiate other materials you have read, or add new information? Does it extensively or marginally cover your topic? You should explore enough sources to obtain a variety of viewpoints.
- Is the material primary or secondary in nature? Primary sources are the raw material of the research process. Secondary sources are based on primary sources. For example, if you were researching Konrad Adenauer’s role in rebuilding West Germany after World War II, Adenauer’s own writings would be one of many primary sources available on this topic. Others might include relevant government documents and contemporary German newspaper articles. Scholars use this primary material to help generate historical interpretations–a secondary source. Books, encyclopedia articles, and scholarly journal articles about Adenauer’s role are considered secondary sources. In the sciences, journal articles and conference proceedings written by experimenters reporting the results of their research are primary documents. Choose both primary and secondary sources when you have the opportunity.
Writing Style
Is the publication organized logically? Are the main points clearly presented? Do you find the text easy to read, or is it stilted or choppy? Is the author’s argument repetitive?
Evaluative Reviews
- Locate critical reviews of books in a reviewing source , such as Summon’s Advanced Search , Book Review Index , Book Review Digest, and ProQuest Research Library . Is the review positive? Is the book under review considered a valuable contribution to the field? Does the reviewer mention other books that might be better? If so, locate these sources for more information on your topic.
- Do the various reviewers agree on the value or attributes of the book or has it aroused controversy among the critics?
- For Web sites, consider consulting one of the evaluation and reviewing sources on the Internet .
Video: How to Identify Scholarly Articles
Video: How to Identify Substantive News Articles
Step 7: Cite What You Find Using a Standard Format
Give credit where credit is due; cite your sources.
Citing or documenting the sources used in your research serves two purposes, it gives proper credit to the authors of the materials used, and it allows those who are reading your work to duplicate your research and locate the sources that you have listed as references. Knowingly representing the work of others as your own is plagiarism. Use one of the styles listed below or another style approved by your instructor.
Modern Language Association (MLA)
MLA Handbook for Writers of Research Papers. 7th ed. New York: MLA, 2009.
This handbook is intended as an aid for college students writing research papers. Included here is information on selecting a topic, researching the topic, note taking, the writing of footnotes and bibliographies, as well as sample pages of a research paper. Useful for the beginning researcher.
American Psychological Association (APA)
Publication Manual of the American Psychological Association. 6th ed. Washington: APA, 2010
The authoritative style manual for anyone writing in the field of psychology. Useful for the social sciences generally. Chapters discuss the content and organization of a manuscript, writing style, the American Psychological Association citation style, and typing, mailing and proofreading.
Research Tips
- Work from the general to the specific. Find background information first, then use more specific and recent sources.
- Record what you find and where you found it. Record the complete citation for each source you find; you may need it again later.
- Translate your topic into the subject language of the indexes and catalogs you use. Check your topic words against a thesaurus or subject heading list.
- The Seven Steps of the Research Process. Provided by : Cornell University Library. Located at : http://guides.library.cornell.edu/sevensteps . License : CC BY-NC-SA: Attribution-NonCommercial-ShareAlike
- Finding Information on the Internet: A Tutorial. Provided by : University of California at Berkley. Located at : http://www.lib.berkeley.edu/TeachingLib/Guides/Internet/FindInfo.html . License : CC BY-NC: Attribution-NonCommercial
- Research Minutes: How to Read Citations. Authored by : Olin & Uris Libraries, Cornell University. Provided by : Cornell University. Located at : http://youtu.be/R1yNDvmjqaE . License : All Rights Reserved . License Terms : Standard YouTube license
- Research Minutes: How to Identify Scholarly Journal Articles. Provided by : Olin & Uris Libraries, Cornell University. Located at : http://youtu.be/uDGJ2CYfY9A . License : All Rights Reserved . License Terms : Standard YouTube license
- Research Minutes: How to Identify Substantive News Articles. Provided by : Olin & Uris Libraries, Cornell University. Located at : http://youtu.be/QAiJL5B5esM . License : All Rights Reserved . License Terms : Standard YouTube license
6.2 Creative Problem-Solving Process
Portions of the material in this section are based on original work by Geoffrey Graybeal and produced with support from the Rebus Community. The original is freely available under the terms of the CC BY 4.0 license at https://press.rebus.community/media-innovation-and-entrepreneurship/.
Learning Objectives
By the end of this section, you will be able to:
- Describe the five steps in the creative problem-solving process
- Identify and describe common creative problem-solving tools
Creativity can be an important trait of an entrepreneur, as the chapter on Creativity, Innovation, and Invention discussed. In that discussion, we learned about creativity’s role in innovation . Here, we will look in more depth at creativity’s role in problem solving . Let’s first formally define creativity as the development of original ideas to solve an issue. The intent of being an entrepreneur is to break away from practical norms and use imagination to embrace quick and effective solutions to an existing problem, usually outside the corporate environment.
The Steps of the Creative Problem-Solving Process
Training oneself to think like an entrepreneur means learning the steps to evaluating a challenge: clarify, ideate, develop, implement, and evaluate ( Figure 6.9 ).
Step 1: Clarify
To clarify is the critical step of recognizing the existence of a gap between the current state and a desired state. This can also be thought of as having need awareness , which occurs when the entrepreneur notes a gap between societal or customer needs and actual circumstances. Clarifying the problem by speaking with clients and developing a detailed description of the problem brings the specifics of a problem to light. Failure to identify the specifics of a problem leaves the entrepreneur with the impossible task of solving a ghost problem, a problem that is fully unknown or unseen. To establish and maintain credibility, an entrepreneur must clarify the problem by focusing on solving the problem itself, rather than solving a symptom of the problem.
For example, a farm could have polluted water, but it would not be enough to solve the problem only on that farm. Clarifying would involve identifying the source of the pollution to adequately tackle the problem. After gaining an understanding of a problem, the entrepreneur should begin to formulate plans for eliminating the gap. A fishbone diagram , as shown in Figure 6.10 , is a tool that can be used to identify the causes of such a problem.
In the case of our water pollution example, a fishbone diagram exploring the issue might reveal the items shown in Figure 6.11 .
Step 2: Ideate
To ideate is the step of the creative problem-solving process that involves generating and detailing ideas by the entrepreneur. After collecting all information relevant to the problem, the entrepreneur lists as many causes of the problem as possible. This is the step in which the largest variety of ideas are put forth. Each idea must be evaluated for feasibility and cost as a solution to the problem. If a farm does not have clean water, for example, the entrepreneur must list causes of toxic water and eliminate as many of those causes as possible. The entrepreneur must then move forward investigating solutions to bring the water back to a safe state. If, say, nearby livestock are polluting the water, the livestock should be isolated from the water source.
Step 3: Develop
To develop is the step in which the entrepreneur takes the list of ideas generated and tests each solution for feasibility. The entrepreneur must consider the cost of each idea and the obstacles to implementation. In the preceding example, adding a chemical to the water may not be a feasible solution to the farmer. Not every farmer wants additional chloride or fluoride added to the water due to the effect on both humans and livestock. These tradeoffs should be addressed in the feasibility assessment. The farmer might prefer a filtration system, but the cost of that solution might not be practicable. The entrepreneur should identify and assess alternative solutions to find one that is most cost-effective and feasible to the customer.
Step 4: Implement
To implement is the step in which the solution to the problem is tested and evaluated. The entrepreneur walks through the planned implementation with the client and tests each part of the solution, if a service, or thoroughly tests a developed good. The entrepreneur implements the solution and goes through a structured system of follow-up to ensure the solution remains effective and viable. In the water example, the solution would be reducing runoff from toxic insecticides by adding prairie strips, buffers of grass, and vegetation along banks of streams.
Step 5: Evaluate
To evaluate is the step in which the final solution is assessed. This is a very important step that entrepreneurs often overlook. Any fallacy in the implementation of the product or service is reassessed, and new solutions are implemented. A continual testing process may be needed to find the final solution. The prairie strips, buffers of grass, and vegetation along banks of streams chosen in the farming water example should then be analyzed and tested to ensure the chosen solution changed the content of the water.
Are You Ready?
Implementing creative problem solving.
Removing waste is a problem, and it can also present an entrepreneurial opportunity. Try to examine ways in which waste products that you usually pay to have hauled away can now generate revenue. Whether it’s recycling aluminum cans or cardboard, or garbage that could be used to feed animals, your task is to come up with solutions to this entrepreneurial-oriented problem.
- Try following the first step of the creative problem-solving process and clearly identify the problem.
- Next, gather data and formulate the challenge.
- Then, explore ideas and come up with solutions.
- Develop a plan of action.
- Finally, note how you would evaluate the effectiveness of your solution.
Using Creativity to Solve Problems
Entrepreneurs are faced with solving many problems as they develop their ideas for filling gaps, whether those opportunities involve establishing a new company or starting a new enterprise within an existing company. Some of these problems include staffing, hiring and managing employees, handling legal compliance, funding, marketing, and paying taxes. Beyond the mundane activities listed, the entrepreneur, or the team that the entrepreneur puts in place, is indispensable in maintaining the ongoing creativity behind the product line or service offered. Innovation and creativity in the business are necessary to expand the product line or develop a groundbreaking service.
It is not necessary for the entrepreneur to feel isolated when it comes to finding creative solutions to a problem. There are societies, tools, and new methods available to spur the creativity of the entrepreneur that will further support the success and expansion of a new enterprise. 14 Learning and using entrepreneurial methods to solve problems alleviates the stress many startup owners feel. The entrepreneur’s creativity will increase using collaborative methodologies . Some entrepreneurial collaborative methodologies include crowdsourcing, brainstorming, storyboarding, conducting quick online surveys to test ideas and concepts, and team creativity activities.
Crowdsourcing
Professor Daren Brabham at the University of Southern California has written books on crowdsourcing and touts its potential in for-profit and not-for-profit business sectors. He defines it simply as “an online, distributed problem-solving and production model.” 15 Crowdsourcing involves teams of amateurs and nonexperts working together to form a solution to a problem. 16 The idea, as cbsnews.com’s Jennifer Alsever has put it, is to “tap into the collective intelligence of the public at large to complete business-related tasks that a company would normally either perform itself or outsource to a third-party provider. Yet free labor is only a narrow part of crowdsourcing's appeal. More importantly, it enables managers to expand the size of their talent pool while also gaining deeper insight into what customers really want. The challenge is to take a cautionary approach to the ‘wisdom of the crowd,’ which can lead to a ‘herd’ mentality.” 17
Link to Learning
Read this article that discusses what crowdsourcing is, how to use it, and its benefits for more information.
This new business prototype, similar to outsourcing, features an enterprise posting a problem online and asking for volunteers to consider the problem and propose solutions. Volunteers earn a reward, such as prize money, promotional materials like a T-shirt, royalties on creative outlets like photos or designs, and in some cases, compensation for their labor. Before proposing the solution, volunteers learn that the solutions become the intellectual property of the startup posting the problem. The solution is then mass produced for profit by the startup that posted the problem. 18 The process evolves into the crowdsourcing process after the enterprise mass produces and profits from the labor of the volunteers and the team. Entrepreneurs should consider that untapped masses have solutions for many issues for which agendas do not yet exist. Crowdsourcing can exploit those agendas and add to the tools used to stimulate personal creativity. This type of innovation is planned and strategically implemented for profit.
For example, Bombardier held a crowdsourced innovation contest to solicit input on the future of train interiors, including seat design and coach class interior. A corporate jury judged the submissions, with the top ten receiving computers or cash prizes. Companies are often constrained, however, by internal rules limiting open source or external idea sourcing, as they could be accused of “stealing” an idea. While crowdsourcing outside of software can be problematic, some products such as MakerBot ’s 3D printers, 3DR’ s drones, and Jibo ’s Social Robot have used developer kits and “makers” to help build a community and stimulate innovation from the outside.
Work It Out
A crowdsourced potato chip.
In an effort to increase sales among millennials, PepsiCo turned to crowdsourcing to get new flavor ideas for their Lay’s potato chips (called Walker’s in the UK). Their 2012 campaign, “Do Us a Flavor,” was so successful that they received over 14 million submissions. The winner was Cheesy Garlic Bread, which increased their potato chip sales by 8 percent during the first three months after the launch.
- What are some other products that would work well for a crowdsourced campaign contest?
- What items wouldn’t work well?
Amazon ’s Mechanical Turk is an online crowdsourcing platform that allows individuals to post tasks for workers to complete. In many instances, these tasks are compensated, but the payment can be less than one dollar per item completed. Mechanical Turk is one of the largest and most well-known crowdsourcing platforms, but there are a number of other more niche ones as well that would apply to smaller markets. In the case of innovation contests and outsourced tasks from corporations, those tasks may be hosted internally by the corporation.
Brainstorming
Brainstorming is the generation of ideas in an environment free of judgment or dissension with the goal of creating solutions. See Creativity, Innovation, and Invention to refresh yourself on this technique. Brainstorming is meant to stimulate participants into thinking about problem solving in a new way. Using a multifunctional group, meaning participants come from different departments and with different skill sets, gives entrepreneurs and support teams a genuine chance to suggest and actualize ideas. The group works together to refine and prototype potential solutions to a problem.
Brainstorming is a highly researched and often practiced technique for the development of innovative solutions. One of the more successful proponents of brainstorming is the United Nations Children’s Fund (UNICEF) . UNICEF faces unique problems of solving resource problems for mothers and children in underdeveloped nations. See how UNICEF practices brainstorming to solve problems including child survival, gender inclusion, refugee crises, education, and others.
The setting for a brainstorming session should remain as informal and relaxed as possible. The group needs to avoid standard solutions. All ideas are welcome and listed and considered with no censorship and with no regard to administrative restrictions. All team members have an equal voice. The focus of brainstorming is on quantity of ideas rather than on the ideal solution provided in every suggestion. A classic entrepreneurial brainstorming activity, as popularized by business software developer Strategyzer , is known as the “silly cow” exercise. Teams come up with ideas for new business models pertaining to a cow, with the results often outrageous, ranging from sponsored cows to stroking cows for therapeutic release. Participants are asked to identify some aspect of a cow and develop three business models around that concept in a short time period, typically two minutes or fewer. The activity is designed to get creative juices flowing.
Watch this video from ABC’s Nightline that shows how IDEO designed a new shopping cart for an example of a design process that involves brainstorming.
Storyboarding
Storyboarding is the process of presenting an idea in a step-by-step graphic format, as Figure 6.12 shows. This tool is useful when the entrepreneur is attempting to visualize a solution to a problem. The steps to the solution of a problem are sketched and hung in graphic format. Once the original graphic is placed, images of steps working toward a solution are added, subtracted, and rearranged on a continual basis, until the ultimate solution emerges in the ultimate graphic format. For many years, entrepreneurs have used this process to create a pre-visual for various media sequences.
Team Creativity
Team creativity is the process whereby an entrepreneur works with a team to create an unexpected solution for an issue or challenge. Teams progress through the same creative problem-solving process described already: clarify, ideate, develop, implement, and evaluate. The main advantage of team creativity is the collaboration and support members receive from one another. Great teams trust in other team members, have diverse members with diverse points of view, are cohesive, and have chemistry.
Team members should work in a stress-free and relaxing environment. Reinforcement and expansion of ideas in the team environment motivates the team to continually expand horizons toward problem solution. A small idea in a team may spark the imagination of a team member to an original idea. Mark Zuckerberg , cofounder of Facebook , once said, “The most important thing for you as an entrepreneur trying to build something is, you need to build a really good team. And that’s what I spend all my time on.” 19
Entrepreneur In Action
Taaluma totes 20.
Young entrepreneurs Jack DuFour and Alley Heffern began to notice the beautiful fabrics that came from the different countries they visited. The entrepreneurs thought about what could be done with the fabrics to create employment opportunities both in the country from which the fabric originated and in their home base of Virginia. They decided to test producing totes from the fabrics they found and formed Taaluma Totes ( Figure 6.13 ). DuFour and Heffern also wanted to promote the production of these fabrics and help underserved populations in countries where the fabric originated maintain a living or follow a dream.
The team continued to test the process and gathered original fabrics, which they sent to Virginia to create totes. They trained individuals with disabilities in Virginia to manufacture the totes, thus serving populations in the United States. The entrepreneurs then decided to take 20 percent of their profits and make microloans to farmers and small business owners in the countries where the fabric originated to create jobs there. Microloans are small loans, below $50,000, which certain lenders offer to enterprising startups. These startups, for various reasons (they are in poor nations, at poverty level), can’t afford a traditional loan from a major bank. The lenders offer business support to the borrower, which in turn helps the borrower repay the microloan. The microloans from Taaluma are repaid when the borrower is able. Repayments are used to buy more fabric, completing Taaluma’s desire to serve dual populations. If the process proved unsuccessful, the co-owners would revise the process to meet the plan’s requirements.
DuFour and Heffern now have fabrics from dozens of countries from Thailand to Ecuador. The totes are specialized with features to meet individual needs. The product line is innovated regularly and Taaluma Totes serves a dual purpose of employing persons with disabilities in Virginia and creating employment for underserved populations in other countries.
- 14 “Creating a World of Opportunities.” The Collegiate Entrepreneurs’ Organization . n.d. https://www.c-e-o.org/
- 15 Daren C. Brabham. “Crowdsourcing as a Model for Problem Solving: An Introduction and Cases.” Convergence: The International Journal of Research into New Media Technologies 14, no. 1 (2008): 75–90.
- 16 Michael Houlihan and Bonnie Harvey. “How Crowdsourcing Is Shaping the Future of Everything.” Entrepreneur. January 13, 2018. https://www.entrepreneur.com/article/307438
- 17 Jennifer Alsever. “What Is Crowdsourcing?” CBS News . May 1, 2008. https://www.cbsnews.com/news/what-is-crowdsourcing
- 18 Daren C. Brabham. “Crowdsourcing as a Model for Problem Solving: An Introduction and Cases.” Convergence: The International Journal of Research into New Media Technologies 14, no. 1 (2008): 75–90.
- 19 “Three Tips for Entrepreneurs Creating the Perfect Team.” Virgin . n.d. https://www.virgin.com/entrepreneur/three-tips-entrepreneurs-creating-perfect-team
- 20 “Backpacks That Carry a Country.” Taaluma Totes. n.d. https://www.carryacountry.com/pages/about
This book may not be used in the training of large language models or otherwise be ingested into large language models or generative AI offerings without OpenStax's permission.
Want to cite, share, or modify this book? This book uses the Creative Commons Attribution License and you must attribute OpenStax.
Access for free at https://openstax.org/books/entrepreneurship/pages/1-introduction
- Authors: Michael Laverty, Chris Littel
- Publisher/website: OpenStax
- Book title: Entrepreneurship
- Publication date: Jan 16, 2020
- Location: Houston, Texas
- Book URL: https://openstax.org/books/entrepreneurship/pages/1-introduction
- Section URL: https://openstax.org/books/entrepreneurship/pages/6-2-creative-problem-solving-process
© Jun 26, 2024 OpenStax. Textbook content produced by OpenStax is licensed under a Creative Commons Attribution License . The OpenStax name, OpenStax logo, OpenStax book covers, OpenStax CNX name, and OpenStax CNX logo are not subject to the Creative Commons license and may not be reproduced without the prior and express written consent of Rice University.
What Is Research, and Why Do People Do It?
- Open Access
- First Online: 03 December 2022
Cite this chapter
You have full access to this open access chapter
- James Hiebert 6 ,
- Jinfa Cai 7 ,
- Stephen Hwang 7 ,
- Anne K Morris 6 &
- Charles Hohensee 6
Part of the book series: Research in Mathematics Education ((RME))
21k Accesses
Abstractspiepr Abs1
Every day people do research as they gather information to learn about something of interest. In the scientific world, however, research means something different than simply gathering information. Scientific research is characterized by its careful planning and observing, by its relentless efforts to understand and explain, and by its commitment to learn from everyone else seriously engaged in research. We call this kind of research scientific inquiry and define it as “formulating, testing, and revising hypotheses.” By “hypotheses” we do not mean the hypotheses you encounter in statistics courses. We mean predictions about what you expect to find and rationales for why you made these predictions. Throughout this and the remaining chapters we make clear that the process of scientific inquiry applies to all kinds of research studies and data, both qualitative and quantitative.
You have full access to this open access chapter, Download chapter PDF
Part I. What Is Research?
Have you ever studied something carefully because you wanted to know more about it? Maybe you wanted to know more about your grandmother’s life when she was younger so you asked her to tell you stories from her childhood, or maybe you wanted to know more about a fertilizer you were about to use in your garden so you read the ingredients on the package and looked them up online. According to the dictionary definition, you were doing research.
Recall your high school assignments asking you to “research” a topic. The assignment likely included consulting a variety of sources that discussed the topic, perhaps including some “original” sources. Often, the teacher referred to your product as a “research paper.”
Were you conducting research when you interviewed your grandmother or wrote high school papers reviewing a particular topic? Our view is that you were engaged in part of the research process, but only a small part. In this book, we reserve the word “research” for what it means in the scientific world, that is, for scientific research or, more pointedly, for scientific inquiry .
Exercise 1.1
Before you read any further, write a definition of what you think scientific inquiry is. Keep it short—Two to three sentences. You will periodically update this definition as you read this chapter and the remainder of the book.
This book is about scientific inquiry—what it is and how to do it. For starters, scientific inquiry is a process, a particular way of finding out about something that involves a number of phases. Each phase of the process constitutes one aspect of scientific inquiry. You are doing scientific inquiry as you engage in each phase, but you have not done scientific inquiry until you complete the full process. Each phase is necessary but not sufficient.
In this chapter, we set the stage by defining scientific inquiry—describing what it is and what it is not—and by discussing what it is good for and why people do it. The remaining chapters build directly on the ideas presented in this chapter.
A first thing to know is that scientific inquiry is not all or nothing. “Scientificness” is a continuum. Inquiries can be more scientific or less scientific. What makes an inquiry more scientific? You might be surprised there is no universally agreed upon answer to this question. None of the descriptors we know of are sufficient by themselves to define scientific inquiry. But all of them give you a way of thinking about some aspects of the process of scientific inquiry. Each one gives you different insights.
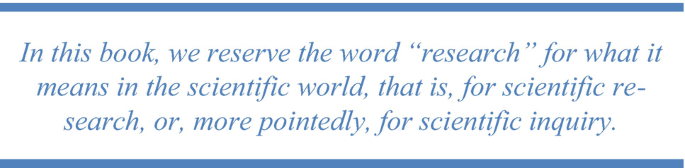
Exercise 1.2
As you read about each descriptor below, think about what would make an inquiry more or less scientific. If you think a descriptor is important, use it to revise your definition of scientific inquiry.
Creating an Image of Scientific Inquiry
We will present three descriptors of scientific inquiry. Each provides a different perspective and emphasizes a different aspect of scientific inquiry. We will draw on all three descriptors to compose our definition of scientific inquiry.
Descriptor 1. Experience Carefully Planned in Advance
Sir Ronald Fisher, often called the father of modern statistical design, once referred to research as “experience carefully planned in advance” (1935, p. 8). He said that humans are always learning from experience, from interacting with the world around them. Usually, this learning is haphazard rather than the result of a deliberate process carried out over an extended period of time. Research, Fisher said, was learning from experience, but experience carefully planned in advance.
This phrase can be fully appreciated by looking at each word. The fact that scientific inquiry is based on experience means that it is based on interacting with the world. These interactions could be thought of as the stuff of scientific inquiry. In addition, it is not just any experience that counts. The experience must be carefully planned . The interactions with the world must be conducted with an explicit, describable purpose, and steps must be taken to make the intended learning as likely as possible. This planning is an integral part of scientific inquiry; it is not just a preparation phase. It is one of the things that distinguishes scientific inquiry from many everyday learning experiences. Finally, these steps must be taken beforehand and the purpose of the inquiry must be articulated in advance of the experience. Clearly, scientific inquiry does not happen by accident, by just stumbling into something. Stumbling into something unexpected and interesting can happen while engaged in scientific inquiry, but learning does not depend on it and serendipity does not make the inquiry scientific.
Descriptor 2. Observing Something and Trying to Explain Why It Is the Way It Is
When we were writing this chapter and googled “scientific inquiry,” the first entry was: “Scientific inquiry refers to the diverse ways in which scientists study the natural world and propose explanations based on the evidence derived from their work.” The emphasis is on studying, or observing, and then explaining . This descriptor takes the image of scientific inquiry beyond carefully planned experience and includes explaining what was experienced.
According to the Merriam-Webster dictionary, “explain” means “(a) to make known, (b) to make plain or understandable, (c) to give the reason or cause of, and (d) to show the logical development or relations of” (Merriam-Webster, n.d. ). We will use all these definitions. Taken together, they suggest that to explain an observation means to understand it by finding reasons (or causes) for why it is as it is. In this sense of scientific inquiry, the following are synonyms: explaining why, understanding why, and reasoning about causes and effects. Our image of scientific inquiry now includes planning, observing, and explaining why.

We need to add a final note about this descriptor. We have phrased it in a way that suggests “observing something” means you are observing something in real time—observing the way things are or the way things are changing. This is often true. But, observing could mean observing data that already have been collected, maybe by someone else making the original observations (e.g., secondary analysis of NAEP data or analysis of existing video recordings of classroom instruction). We will address secondary analyses more fully in Chap. 4 . For now, what is important is that the process requires explaining why the data look like they do.
We must note that for us, the term “data” is not limited to numerical or quantitative data such as test scores. Data can also take many nonquantitative forms, including written survey responses, interview transcripts, journal entries, video recordings of students, teachers, and classrooms, text messages, and so forth.
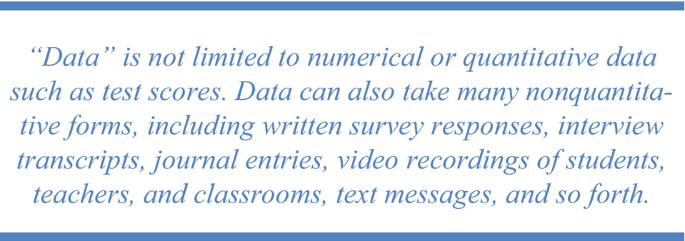
Exercise 1.3
What are the implications of the statement that just “observing” is not enough to count as scientific inquiry? Does this mean that a detailed description of a phenomenon is not scientific inquiry?
Find sources that define research in education that differ with our position, that say description alone, without explanation, counts as scientific research. Identify the precise points where the opinions differ. What are the best arguments for each of the positions? Which do you prefer? Why?
Descriptor 3. Updating Everyone’s Thinking in Response to More and Better Information
This descriptor focuses on a third aspect of scientific inquiry: updating and advancing the field’s understanding of phenomena that are investigated. This descriptor foregrounds a powerful characteristic of scientific inquiry: the reliability (or trustworthiness) of what is learned and the ultimate inevitability of this learning to advance human understanding of phenomena. Humans might choose not to learn from scientific inquiry, but history suggests that scientific inquiry always has the potential to advance understanding and that, eventually, humans take advantage of these new understandings.
Before exploring these bold claims a bit further, note that this descriptor uses “information” in the same way the previous two descriptors used “experience” and “observations.” These are the stuff of scientific inquiry and we will use them often, sometimes interchangeably. Frequently, we will use the term “data” to stand for all these terms.
An overriding goal of scientific inquiry is for everyone to learn from what one scientist does. Much of this book is about the methods you need to use so others have faith in what you report and can learn the same things you learned. This aspect of scientific inquiry has many implications.
One implication is that scientific inquiry is not a private practice. It is a public practice available for others to see and learn from. Notice how different this is from everyday learning. When you happen to learn something from your everyday experience, often only you gain from the experience. The fact that research is a public practice means it is also a social one. It is best conducted by interacting with others along the way: soliciting feedback at each phase, taking opportunities to present work-in-progress, and benefitting from the advice of others.
A second implication is that you, as the researcher, must be committed to sharing what you are doing and what you are learning in an open and transparent way. This allows all phases of your work to be scrutinized and critiqued. This is what gives your work credibility. The reliability or trustworthiness of your findings depends on your colleagues recognizing that you have used all appropriate methods to maximize the chances that your claims are justified by the data.
A third implication of viewing scientific inquiry as a collective enterprise is the reverse of the second—you must be committed to receiving comments from others. You must treat your colleagues as fair and honest critics even though it might sometimes feel otherwise. You must appreciate their job, which is to remain skeptical while scrutinizing what you have done in considerable detail. To provide the best help to you, they must remain skeptical about your conclusions (when, for example, the data are difficult for them to interpret) until you offer a convincing logical argument based on the information you share. A rather harsh but good-to-remember statement of the role of your friendly critics was voiced by Karl Popper, a well-known twentieth century philosopher of science: “. . . if you are interested in the problem which I tried to solve by my tentative assertion, you may help me by criticizing it as severely as you can” (Popper, 1968, p. 27).
A final implication of this third descriptor is that, as someone engaged in scientific inquiry, you have no choice but to update your thinking when the data support a different conclusion. This applies to your own data as well as to those of others. When data clearly point to a specific claim, even one that is quite different than you expected, you must reconsider your position. If the outcome is replicated multiple times, you need to adjust your thinking accordingly. Scientific inquiry does not let you pick and choose which data to believe; it mandates that everyone update their thinking when the data warrant an update.
Doing Scientific Inquiry
We define scientific inquiry in an operational sense—what does it mean to do scientific inquiry? What kind of process would satisfy all three descriptors: carefully planning an experience in advance; observing and trying to explain what you see; and, contributing to updating everyone’s thinking about an important phenomenon?
We define scientific inquiry as formulating , testing , and revising hypotheses about phenomena of interest.
Of course, we are not the only ones who define it in this way. The definition for the scientific method posted by the editors of Britannica is: “a researcher develops a hypothesis, tests it through various means, and then modifies the hypothesis on the basis of the outcome of the tests and experiments” (Britannica, n.d. ).

Notice how defining scientific inquiry this way satisfies each of the descriptors. “Carefully planning an experience in advance” is exactly what happens when formulating a hypothesis about a phenomenon of interest and thinking about how to test it. “ Observing a phenomenon” occurs when testing a hypothesis, and “ explaining ” what is found is required when revising a hypothesis based on the data. Finally, “updating everyone’s thinking” comes from comparing publicly the original with the revised hypothesis.
Doing scientific inquiry, as we have defined it, underscores the value of accumulating knowledge rather than generating random bits of knowledge. Formulating, testing, and revising hypotheses is an ongoing process, with each revised hypothesis begging for another test, whether by the same researcher or by new researchers. The editors of Britannica signaled this cyclic process by adding the following phrase to their definition of the scientific method: “The modified hypothesis is then retested, further modified, and tested again.” Scientific inquiry creates a process that encourages each study to build on the studies that have gone before. Through collective engagement in this process of building study on top of study, the scientific community works together to update its thinking.
Before exploring more fully the meaning of “formulating, testing, and revising hypotheses,” we need to acknowledge that this is not the only way researchers define research. Some researchers prefer a less formal definition, one that includes more serendipity, less planning, less explanation. You might have come across more open definitions such as “research is finding out about something.” We prefer the tighter hypothesis formulation, testing, and revision definition because we believe it provides a single, coherent map for conducting research that addresses many of the thorny problems educational researchers encounter. We believe it is the most useful orientation toward research and the most helpful to learn as a beginning researcher.
A final clarification of our definition is that it applies equally to qualitative and quantitative research. This is a familiar distinction in education that has generated much discussion. You might think our definition favors quantitative methods over qualitative methods because the language of hypothesis formulation and testing is often associated with quantitative methods. In fact, we do not favor one method over another. In Chap. 4 , we will illustrate how our definition fits research using a range of quantitative and qualitative methods.
Exercise 1.4
Look for ways to extend what the field knows in an area that has already received attention by other researchers. Specifically, you can search for a program of research carried out by more experienced researchers that has some revised hypotheses that remain untested. Identify a revised hypothesis that you might like to test.
Unpacking the Terms Formulating, Testing, and Revising Hypotheses
To get a full sense of the definition of scientific inquiry we will use throughout this book, it is helpful to spend a little time with each of the key terms.
We first want to make clear that we use the term “hypothesis” as it is defined in most dictionaries and as it used in many scientific fields rather than as it is usually defined in educational statistics courses. By “hypothesis,” we do not mean a null hypothesis that is accepted or rejected by statistical analysis. Rather, we use “hypothesis” in the sense conveyed by the following definitions: “An idea or explanation for something that is based on known facts but has not yet been proved” (Cambridge University Press, n.d. ), and “An unproved theory, proposition, or supposition, tentatively accepted to explain certain facts and to provide a basis for further investigation or argument” (Agnes & Guralnik, 2008 ).
We distinguish two parts to “hypotheses.” Hypotheses consist of predictions and rationales . Predictions are statements about what you expect to find when you inquire about something. Rationales are explanations for why you made the predictions you did, why you believe your predictions are correct. So, for us “formulating hypotheses” means making explicit predictions and developing rationales for the predictions.
“Testing hypotheses” means making observations that allow you to assess in what ways your predictions were correct and in what ways they were incorrect. In education research, it is rarely useful to think of your predictions as either right or wrong. Because of the complexity of most issues you will investigate, most predictions will be right in some ways and wrong in others.
By studying the observations you make (data you collect) to test your hypotheses, you can revise your hypotheses to better align with the observations. This means revising your predictions plus revising your rationales to justify your adjusted predictions. Even though you might not run another test, formulating revised hypotheses is an essential part of conducting a research study. Comparing your original and revised hypotheses informs everyone of what you learned by conducting your study. In addition, a revised hypothesis sets the stage for you or someone else to extend your study and accumulate more knowledge of the phenomenon.
We should note that not everyone makes a clear distinction between predictions and rationales as two aspects of hypotheses. In fact, common, non-scientific uses of the word “hypothesis” may limit it to only a prediction or only an explanation (or rationale). We choose to explicitly include both prediction and rationale in our definition of hypothesis, not because we assert this should be the universal definition, but because we want to foreground the importance of both parts acting in concert. Using “hypothesis” to represent both prediction and rationale could hide the two aspects, but we make them explicit because they provide different kinds of information. It is usually easier to make predictions than develop rationales because predictions can be guesses, hunches, or gut feelings about which you have little confidence. Developing a compelling rationale requires careful thought plus reading what other researchers have found plus talking with your colleagues. Often, while you are developing your rationale you will find good reasons to change your predictions. Developing good rationales is the engine that drives scientific inquiry. Rationales are essentially descriptions of how much you know about the phenomenon you are studying. Throughout this guide, we will elaborate on how developing good rationales drives scientific inquiry. For now, we simply note that it can sharpen your predictions and help you to interpret your data as you test your hypotheses.
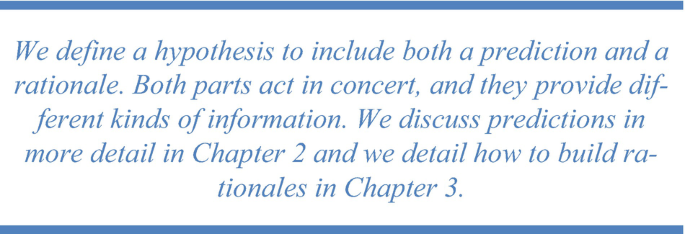
Hypotheses in education research take a variety of forms or types. This is because there are a variety of phenomena that can be investigated. Investigating educational phenomena is sometimes best done using qualitative methods, sometimes using quantitative methods, and most often using mixed methods (e.g., Hay, 2016 ; Weis et al. 2019a ; Weisner, 2005 ). This means that, given our definition, hypotheses are equally applicable to qualitative and quantitative investigations.
Hypotheses take different forms when they are used to investigate different kinds of phenomena. Two very different activities in education could be labeled conducting experiments and descriptions. In an experiment, a hypothesis makes a prediction about anticipated changes, say the changes that occur when a treatment or intervention is applied. You might investigate how students’ thinking changes during a particular kind of instruction.
A second type of hypothesis, relevant for descriptive research, makes a prediction about what you will find when you investigate and describe the nature of a situation. The goal is to understand a situation as it exists rather than to understand a change from one situation to another. In this case, your prediction is what you expect to observe. Your rationale is the set of reasons for making this prediction; it is your current explanation for why the situation will look like it does.
You will probably read, if you have not already, that some researchers say you do not need a prediction to conduct a descriptive study. We will discuss this point of view in Chap. 2 . For now, we simply claim that scientific inquiry, as we have defined it, applies to all kinds of research studies. Descriptive studies, like others, not only benefit from formulating, testing, and revising hypotheses, but also need hypothesis formulating, testing, and revising.
One reason we define research as formulating, testing, and revising hypotheses is that if you think of research in this way you are less likely to go wrong. It is a useful guide for the entire process, as we will describe in detail in the chapters ahead. For example, as you build the rationale for your predictions, you are constructing the theoretical framework for your study (Chap. 3 ). As you work out the methods you will use to test your hypothesis, every decision you make will be based on asking, “Will this help me formulate or test or revise my hypothesis?” (Chap. 4 ). As you interpret the results of testing your predictions, you will compare them to what you predicted and examine the differences, focusing on how you must revise your hypotheses (Chap. 5 ). By anchoring the process to formulating, testing, and revising hypotheses, you will make smart decisions that yield a coherent and well-designed study.
Exercise 1.5
Compare the concept of formulating, testing, and revising hypotheses with the descriptions of scientific inquiry contained in Scientific Research in Education (NRC, 2002 ). How are they similar or different?
Exercise 1.6
Provide an example to illustrate and emphasize the differences between everyday learning/thinking and scientific inquiry.
Learning from Doing Scientific Inquiry
We noted earlier that a measure of what you have learned by conducting a research study is found in the differences between your original hypothesis and your revised hypothesis based on the data you collected to test your hypothesis. We will elaborate this statement in later chapters, but we preview our argument here.
Even before collecting data, scientific inquiry requires cycles of making a prediction, developing a rationale, refining your predictions, reading and studying more to strengthen your rationale, refining your predictions again, and so forth. And, even if you have run through several such cycles, you still will likely find that when you test your prediction you will be partly right and partly wrong. The results will support some parts of your predictions but not others, or the results will “kind of” support your predictions. A critical part of scientific inquiry is making sense of your results by interpreting them against your predictions. Carefully describing what aspects of your data supported your predictions, what aspects did not, and what data fell outside of any predictions is not an easy task, but you cannot learn from your study without doing this analysis.
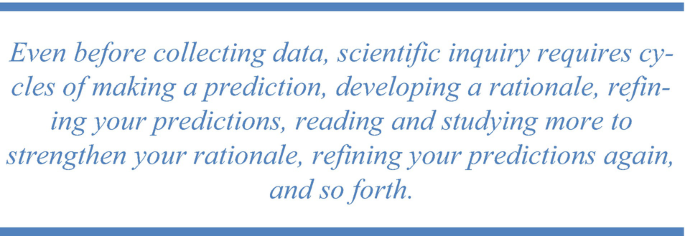
Analyzing the matches and mismatches between your predictions and your data allows you to formulate different rationales that would have accounted for more of the data. The best revised rationale is the one that accounts for the most data. Once you have revised your rationales, you can think about the predictions they best justify or explain. It is by comparing your original rationales to your new rationales that you can sort out what you learned from your study.
Suppose your study was an experiment. Maybe you were investigating the effects of a new instructional intervention on students’ learning. Your original rationale was your explanation for why the intervention would change the learning outcomes in a particular way. Your revised rationale explained why the changes that you observed occurred like they did and why your revised predictions are better. Maybe your original rationale focused on the potential of the activities if they were implemented in ideal ways and your revised rationale included the factors that are likely to affect how teachers implement them. By comparing the before and after rationales, you are describing what you learned—what you can explain now that you could not before. Another way of saying this is that you are describing how much more you understand now than before you conducted your study.
Revised predictions based on carefully planned and collected data usually exhibit some of the following features compared with the originals: more precision, more completeness, and broader scope. Revised rationales have more explanatory power and become more complete, more aligned with the new predictions, sharper, and overall more convincing.
Part II. Why Do Educators Do Research?
Doing scientific inquiry is a lot of work. Each phase of the process takes time, and you will often cycle back to improve earlier phases as you engage in later phases. Because of the significant effort required, you should make sure your study is worth it. So, from the beginning, you should think about the purpose of your study. Why do you want to do it? And, because research is a social practice, you should also think about whether the results of your study are likely to be important and significant to the education community.
If you are doing research in the way we have described—as scientific inquiry—then one purpose of your study is to understand , not just to describe or evaluate or report. As we noted earlier, when you formulate hypotheses, you are developing rationales that explain why things might be like they are. In our view, trying to understand and explain is what separates research from other kinds of activities, like evaluating or describing.
One reason understanding is so important is that it allows researchers to see how or why something works like it does. When you see how something works, you are better able to predict how it might work in other contexts, under other conditions. And, because conditions, or contextual factors, matter a lot in education, gaining insights into applying your findings to other contexts increases the contributions of your work and its importance to the broader education community.
Consequently, the purposes of research studies in education often include the more specific aim of identifying and understanding the conditions under which the phenomena being studied work like the observations suggest. A classic example of this kind of study in mathematics education was reported by William Brownell and Harold Moser in 1949 . They were trying to establish which method of subtracting whole numbers could be taught most effectively—the regrouping method or the equal additions method. However, they realized that effectiveness might depend on the conditions under which the methods were taught—“meaningfully” versus “mechanically.” So, they designed a study that crossed the two instructional approaches with the two different methods (regrouping and equal additions). Among other results, they found that these conditions did matter. The regrouping method was more effective under the meaningful condition than the mechanical condition, but the same was not true for the equal additions algorithm.
What do education researchers want to understand? In our view, the ultimate goal of education is to offer all students the best possible learning opportunities. So, we believe the ultimate purpose of scientific inquiry in education is to develop understanding that supports the improvement of learning opportunities for all students. We say “ultimate” because there are lots of issues that must be understood to improve learning opportunities for all students. Hypotheses about many aspects of education are connected, ultimately, to students’ learning. For example, formulating and testing a hypothesis that preservice teachers need to engage in particular kinds of activities in their coursework in order to teach particular topics well is, ultimately, connected to improving students’ learning opportunities. So is hypothesizing that school districts often devote relatively few resources to instructional leadership training or hypothesizing that positioning mathematics as a tool students can use to combat social injustice can help students see the relevance of mathematics to their lives.
We do not exclude the importance of research on educational issues more removed from improving students’ learning opportunities, but we do think the argument for their importance will be more difficult to make. If there is no way to imagine a connection between your hypothesis and improving learning opportunities for students, even a distant connection, we recommend you reconsider whether it is an important hypothesis within the education community.
Notice that we said the ultimate goal of education is to offer all students the best possible learning opportunities. For too long, educators have been satisfied with a goal of offering rich learning opportunities for lots of students, sometimes even for just the majority of students, but not necessarily for all students. Evaluations of success often are based on outcomes that show high averages. In other words, if many students have learned something, or even a smaller number have learned a lot, educators may have been satisfied. The problem is that there is usually a pattern in the groups of students who receive lower quality opportunities—students of color and students who live in poor areas, urban and rural. This is not acceptable. Consequently, we emphasize the premise that the purpose of education research is to offer rich learning opportunities to all students.
One way to make sure you will be able to convince others of the importance of your study is to consider investigating some aspect of teachers’ shared instructional problems. Historically, researchers in education have set their own research agendas, regardless of the problems teachers are facing in schools. It is increasingly recognized that teachers have had trouble applying to their own classrooms what researchers find. To address this problem, a researcher could partner with a teacher—better yet, a small group of teachers—and talk with them about instructional problems they all share. These discussions can create a rich pool of problems researchers can consider. If researchers pursued one of these problems (preferably alongside teachers), the connection to improving learning opportunities for all students could be direct and immediate. “Grounding a research question in instructional problems that are experienced across multiple teachers’ classrooms helps to ensure that the answer to the question will be of sufficient scope to be relevant and significant beyond the local context” (Cai et al., 2019b , p. 115).
As a beginning researcher, determining the relevance and importance of a research problem is especially challenging. We recommend talking with advisors, other experienced researchers, and peers to test the educational importance of possible research problems and topics of study. You will also learn much more about the issue of research importance when you read Chap. 5 .
Exercise 1.7
Identify a problem in education that is closely connected to improving learning opportunities and a problem that has a less close connection. For each problem, write a brief argument (like a logical sequence of if-then statements) that connects the problem to all students’ learning opportunities.
Part III. Conducting Research as a Practice of Failing Productively
Scientific inquiry involves formulating hypotheses about phenomena that are not fully understood—by you or anyone else. Even if you are able to inform your hypotheses with lots of knowledge that has already been accumulated, you are likely to find that your prediction is not entirely accurate. This is normal. Remember, scientific inquiry is a process of constantly updating your thinking. More and better information means revising your thinking, again, and again, and again. Because you never fully understand a complicated phenomenon and your hypotheses never produce completely accurate predictions, it is easy to believe you are somehow failing.
The trick is to fail upward, to fail to predict accurately in ways that inform your next hypothesis so you can make a better prediction. Some of the best-known researchers in education have been open and honest about the many times their predictions were wrong and, based on the results of their studies and those of others, they continuously updated their thinking and changed their hypotheses.
A striking example of publicly revising (actually reversing) hypotheses due to incorrect predictions is found in the work of Lee J. Cronbach, one of the most distinguished educational psychologists of the twentieth century. In 1955, Cronbach delivered his presidential address to the American Psychological Association. Titling it “Two Disciplines of Scientific Psychology,” Cronbach proposed a rapprochement between two research approaches—correlational studies that focused on individual differences and experimental studies that focused on instructional treatments controlling for individual differences. (We will examine different research approaches in Chap. 4 ). If these approaches could be brought together, reasoned Cronbach ( 1957 ), researchers could find interactions between individual characteristics and treatments (aptitude-treatment interactions or ATIs), fitting the best treatments to different individuals.
In 1975, after years of research by many researchers looking for ATIs, Cronbach acknowledged the evidence for simple, useful ATIs had not been found. Even when trying to find interactions between a few variables that could provide instructional guidance, the analysis, said Cronbach, creates “a hall of mirrors that extends to infinity, tormenting even the boldest investigators and defeating even ambitious designs” (Cronbach, 1975 , p. 119).
As he was reflecting back on his work, Cronbach ( 1986 ) recommended moving away from documenting instructional effects through statistical inference (an approach he had championed for much of his career) and toward approaches that probe the reasons for these effects, approaches that provide a “full account of events in a time, place, and context” (Cronbach, 1986 , p. 104). This is a remarkable change in hypotheses, a change based on data and made fully transparent. Cronbach understood the value of failing productively.
Closer to home, in a less dramatic example, one of us began a line of scientific inquiry into how to prepare elementary preservice teachers to teach early algebra. Teaching early algebra meant engaging elementary students in early forms of algebraic reasoning. Such reasoning should help them transition from arithmetic to algebra. To begin this line of inquiry, a set of activities for preservice teachers were developed. Even though the activities were based on well-supported hypotheses, they largely failed to engage preservice teachers as predicted because of unanticipated challenges the preservice teachers faced. To capitalize on this failure, follow-up studies were conducted, first to better understand elementary preservice teachers’ challenges with preparing to teach early algebra, and then to better support preservice teachers in navigating these challenges. In this example, the initial failure was a necessary step in the researchers’ scientific inquiry and furthered the researchers’ understanding of this issue.
We present another example of failing productively in Chap. 2 . That example emerges from recounting the history of a well-known research program in mathematics education.
Making mistakes is an inherent part of doing scientific research. Conducting a study is rarely a smooth path from beginning to end. We recommend that you keep the following things in mind as you begin a career of conducting research in education.
First, do not get discouraged when you make mistakes; do not fall into the trap of feeling like you are not capable of doing research because you make too many errors.
Second, learn from your mistakes. Do not ignore your mistakes or treat them as errors that you simply need to forget and move past. Mistakes are rich sites for learning—in research just as in other fields of study.
Third, by reflecting on your mistakes, you can learn to make better mistakes, mistakes that inform you about a productive next step. You will not be able to eliminate your mistakes, but you can set a goal of making better and better mistakes.
Exercise 1.8
How does scientific inquiry differ from everyday learning in giving you the tools to fail upward? You may find helpful perspectives on this question in other resources on science and scientific inquiry (e.g., Failure: Why Science is So Successful by Firestein, 2015).
Exercise 1.9
Use what you have learned in this chapter to write a new definition of scientific inquiry. Compare this definition with the one you wrote before reading this chapter. If you are reading this book as part of a course, compare your definition with your colleagues’ definitions. Develop a consensus definition with everyone in the course.
Part IV. Preview of Chap. 2
Now that you have a good idea of what research is, at least of what we believe research is, the next step is to think about how to actually begin doing research. This means how to begin formulating, testing, and revising hypotheses. As for all phases of scientific inquiry, there are lots of things to think about. Because it is critical to start well, we devote Chap. 2 to getting started with formulating hypotheses.
Agnes, M., & Guralnik, D. B. (Eds.). (2008). Hypothesis. In Webster’s new world college dictionary (4th ed.). Wiley.
Google Scholar
Britannica. (n.d.). Scientific method. In Encyclopaedia Britannica . Retrieved July 15, 2022 from https://www.britannica.com/science/scientific-method
Brownell, W. A., & Moser, H. E. (1949). Meaningful vs. mechanical learning: A study in grade III subtraction . Duke University Press..
Cai, J., Morris, A., Hohensee, C., Hwang, S., Robison, V., Cirillo, M., Kramer, S. L., & Hiebert, J. (2019b). Posing significant research questions. Journal for Research in Mathematics Education, 50 (2), 114–120. https://doi.org/10.5951/jresematheduc.50.2.0114
Article Google Scholar
Cambridge University Press. (n.d.). Hypothesis. In Cambridge dictionary . Retrieved July 15, 2022 from https://dictionary.cambridge.org/us/dictionary/english/hypothesis
Cronbach, J. L. (1957). The two disciplines of scientific psychology. American Psychologist, 12 , 671–684.
Cronbach, L. J. (1975). Beyond the two disciplines of scientific psychology. American Psychologist, 30 , 116–127.
Cronbach, L. J. (1986). Social inquiry by and for earthlings. In D. W. Fiske & R. A. Shweder (Eds.), Metatheory in social science: Pluralisms and subjectivities (pp. 83–107). University of Chicago Press.
Hay, C. M. (Ed.). (2016). Methods that matter: Integrating mixed methods for more effective social science research . University of Chicago Press.
Merriam-Webster. (n.d.). Explain. In Merriam-Webster.com dictionary . Retrieved July 15, 2022, from https://www.merriam-webster.com/dictionary/explain
National Research Council. (2002). Scientific research in education . National Academy Press.
Weis, L., Eisenhart, M., Duncan, G. J., Albro, E., Bueschel, A. C., Cobb, P., Eccles, J., Mendenhall, R., Moss, P., Penuel, W., Ream, R. K., Rumbaut, R. G., Sloane, F., Weisner, T. S., & Wilson, J. (2019a). Mixed methods for studies that address broad and enduring issues in education research. Teachers College Record, 121 , 100307.
Weisner, T. S. (Ed.). (2005). Discovering successful pathways in children’s development: Mixed methods in the study of childhood and family life . University of Chicago Press.
Download references
Author information
Authors and affiliations.
School of Education, University of Delaware, Newark, DE, USA
James Hiebert, Anne K Morris & Charles Hohensee
Department of Mathematical Sciences, University of Delaware, Newark, DE, USA
Jinfa Cai & Stephen Hwang
You can also search for this author in PubMed Google Scholar
Rights and permissions
Open Access This chapter is licensed under the terms of the Creative Commons Attribution 4.0 International License ( http://creativecommons.org/licenses/by/4.0/ ), which permits use, sharing, adaptation, distribution and reproduction in any medium or format, as long as you give appropriate credit to the original author(s) and the source, provide a link to the Creative Commons license and indicate if changes were made.
The images or other third party material in this chapter are included in the chapter's Creative Commons license, unless indicated otherwise in a credit line to the material. If material is not included in the chapter's Creative Commons license and your intended use is not permitted by statutory regulation or exceeds the permitted use, you will need to obtain permission directly from the copyright holder.
Reprints and permissions
Copyright information
© 2023 The Author(s)
About this chapter
Hiebert, J., Cai, J., Hwang, S., Morris, A.K., Hohensee, C. (2023). What Is Research, and Why Do People Do It?. In: Doing Research: A New Researcher’s Guide. Research in Mathematics Education. Springer, Cham. https://doi.org/10.1007/978-3-031-19078-0_1
Download citation
DOI : https://doi.org/10.1007/978-3-031-19078-0_1
Published : 03 December 2022
Publisher Name : Springer, Cham
Print ISBN : 978-3-031-19077-3
Online ISBN : 978-3-031-19078-0
eBook Packages : Education Education (R0)
Share this chapter
Anyone you share the following link with will be able to read this content:
Sorry, a shareable link is not currently available for this article.
Provided by the Springer Nature SharedIt content-sharing initiative
- Publish with us
Policies and ethics
- Find a journal
- Track your research

Making educational experiences better for everyone.
Immersive learning for 25 languages
Marketplace for millions of educator-created resources
Fast, easy, reliable language certification
Fun educational games for kids
Comprehensive K-12 personalized learning
Trusted tutors for 300+ subjects
35,000+ worksheets, games, and lesson plans
Adaptive learning for English vocabulary
- Election 2024
- Entertainment
- Newsletters
- Photography
- AP Buyline Personal Finance
- AP Buyline Shopping
- Press Releases
- Israel-Hamas War
- Russia-Ukraine War
- Global elections
- Asia Pacific
- Latin America
- Middle East
- Delegate Tracker
- AP & Elections
- 2024 Paris Olympic Games
- Auto Racing
- Movie reviews
- Book reviews
- Financial Markets
- Business Highlights
- Financial wellness
- Artificial Intelligence
- Social Media
Here’s a look at questions about Tim Walz’s military record
Walz’s military record under scrutiny as Vance, GOP question his service
FILE - Minnesota Gov. Tim Walz, the running mate of Democratic presidential nominee Vice President Kamala Harris, is pictured at a campaign rally in Philadelphia, Aug. 6, 2024. (AP Photo/Matt Rourke)
- Copy Link copied

CINCINNATI (AP) — Republicans are questioning Minnesota Gov. Tim Walz’s military record after Vice President Kamala Harris named him as her running mate this week.
Here’s a look at the issue:
He retired before his unit’s deployment to Iraq
Walz served a total of 24 years in various units and jobs in the Army National Guard. But it’s his retirement in 2005 that’s prompting criticism from some Republicans who are suggesting he abandoned his team to pursue a campaign for Congress.
As he ramped up for a congressional bid in 2005, Walz’s campaign in March issued a statement saying he still planned to run despite a possible mobilization of Minnesota National Guard soldiers to Iraq. According to the Guard, Walz retired from service in May of that year.
In August 2005, the Department of the Army issued a mobilization order for Walz’s unit. The unit mobilized in October of that year before it deployed to Iraq in March 2006 .
There is no evidence that Walz timed his departure with the intent of avoiding deployment. But the fact remains that he left ahead of his unit’s departure. In a statement, the Harris campaign pushed back on GOP characterizations of Walz’s service, and also noted that he advocated for veterans once he was elected to the U.S. House.
“After 24 years of military service, Governor Walz retired in 2005 and ran for Congress, where he chaired Veterans Affairs and was a tireless advocate for our men and women in uniform — and as Vice President of the United States he will continue to be a relentless champion for our veterans and military families,” the campaign said.
Before leaving Detroit, where she and Walz played up their support for organized labor , Harris on Thursday responded to a question about the criticism of her running mate’s record.
“Listen, I praise anyone who has presented themselves to serve our country,” she said. “And I think that we all should.”
Walz didn’t serve in a combat zone
Earlier this week Harris’ campaign circulated on X a 2018 clip of Walz speaking out against gun violence, and saying, “We can make sure that those weapons of war, that I carried in war, is the only place where those weapons are at.” That comment suggests that Walz portrayed himself as someone who spent time in a combat zone.
According to the Nebraska Army National Guard, Walz enlisted in April 1981 — just two days after his 17th birthday — and entered service as an infantryman, completing a 12-week Army infantry basic training course before graduating from high school.
While attending the University of Houston in 1985, he was reclassified as a field artillery cannoneer as a member of the Texas Army National Guard, later serving as an instructor with the Arkansas Army National Guard.
In 1987, Walz returned to Nebraska’s Guard detachment, continuing field artillery assignments while he completed a college degree. By 1996, he transferred to the Minnesota Army National Guard. In 2003, he deployed to Italy in a support position of active military forces in Iraq and Afghanistan. But he was not in a combat zone himself.
“Do not pretend to be something that you’re not,” Republican vice presidential nominee JD Vance said Wednesday as he campaigned in Michigan. “I’d be ashamed if I was saying that I lied about my military service like you did.”
Vance enlisted in the Marine Corps after graduating high school, serving four years as a combat correspondent, a type of military journalist, and deploying to Iraq in that capacity in 2005.
What to know about the 2024 Election
- Today’s news: Follow live updates from the campaign trail from the AP.
- Ground Game: Sign up for AP’s weekly politics newsletter to get it in your inbox every Monday.
- AP’s Role: The Associated Press is the most trusted source of information on election night, with a history of accuracy dating to 1848. Learn more.
Neither Trump nor Harris has served in the U.S. military. Trump received a series of deferments during Vietnam, including one attained with a physician’s letter stating that he suffered from bone spurs in his feet.
The Harris campaign statement said Walz “would never insult or undermine any American’s service to this country” and “thanks Senator Vance for putting his life on the line for our country. It’s the American way.”
What about his rank?
Harris’ campaign has referred to Walz as a “retired Command Sergeant Major,” one of the top ranks for an enlisted soldier. He did in fact achieve that rank, but personnel files show he was reduced in rank months after retiring. That left him as a master sergeant for benefits purposes.
Minnesota National Guard officials have said that Walz retired before completing coursework at the U.S. Army Sergeants Major Academy, along with other requirements associated with his promotion.
Associated Press writers Darlene Superville, Trenton Daniel and Richard Lardner contributed to this report.
Meg Kinnard can be reached at http://x.com/MegKinnardAP

Stack Exchange Network
Stack Exchange network consists of 183 Q&A communities including Stack Overflow , the largest, most trusted online community for developers to learn, share their knowledge, and build their careers.
Q&A for work
Connect and share knowledge within a single location that is structured and easy to search.
What are some examples of research that does not solve a existing problem (maybe the problem is not obvious yet) but present a unique discovery? [closed]
Do you need a problem to find a new discovery? Are there any publications that solely exist to classify parts of existing theories without targeting a real-world problem?
- research-process
- What about things discovered that solve a problem that was not the target of the original work? – Solar Mike Commented Sep 15, 2019 at 20:28
- Viagra leaps to attention as it was originally being tested for cardiovascular diseases... – Solar Mike Commented Sep 15, 2019 at 21:18
- You seem to think of only strictly scientific/mathematical research here. – Dilworth Commented Sep 15, 2019 at 21:48
- 4 Welcome to Academia SE. As it stands, your question suffers from two problems: 1) It is not clear what exactly you are looking for. Your second question seems to be different from your first one. 2) Your question is very broad having a huge amount of possible answers (as I understand it, I could present almost all of my own papers here). — If you can, please edit it to amend this. – Wrzlprmft ♦ Commented Sep 16, 2019 at 5:12
4 Answers 4
It is a bit unclear what you mean or whether you are only interested in applications of research when you say "real-world".
Almost all research starts out without a well defined problem that is known in the literature. There are classic unsolved problems, of course, and people work on those, but graduate students are advised to avoid them. If a problem has been known for a while it has probably been worked on and the solution is (a) likely pretty hard and (b) the field hasn't yet developed the base to make a solution accessible. If hundreds of people have looked at a problem without a solution it is probably pretty hard.
The Four Color Theorem is instructive. Can you color every planar map with only four colors so that adjacent regions have different colors. The theorem has now been proven, but until computers came along the proof was pretty inaccessible. The proof is also unsatisfying to many mathematicians, since the analysis of a large set of cases that the proof requires looks pretty boring. Perhaps someone someday will have the insight for an elegant proof. But for a doctoral student to stake his/her future on finding that proof would be a tactical mistake.
Most research starts out with someone simply wondering about a question they have based on their readings. They don't find the question stated somewhere most likely, but they are puzzled by something and wonder if they can resolve their puzzlement somehow. "How do people balance themselves?" "How do bats find insects in the dark?" "Why do large dinosaurs have holes in their skulls?" "How does the local behavior of a real valued function affect the global behavior?"
A lot of research (doctoral level) starts out with an advisor giving a recent paper to a student with instructions to read it and then answer the question "What do you think about that?". Not very well formed. A research question might arise from their discussion. Is it correct? Can it be extended? Can it be combined with this other thing? Suppose look at what happens if we change this assumption?
The research grows out of those questions by finding some methodology that can get close to an answer.
But if you are only interested in applications, I think that the original researchers in most fields don't think much about that. Some do, of course, but more are interested in the pure knowledge that can arise.
The original researchers about bat echolocation weren't thinking about radar and how to catch speeding automobiles. That came later.
Most pure mathematicians study math for the ideas. My own dissertation was so esoteric that I assumed (not quite fifty years ago) that it would never find application in the real world. My results were unique , but not useful, most likely.. After thirty years or so, I was proven wrong when someone else found a "real world" use for what I did purely for the intellectual challenge (and the degree, of course). And I was surprised to see the application appear.
Applied mathematicians, on the other hand, do start with a given problem, but, again, it is probably a problem that they formulate themselves, rather than one that they found already fully formed in the literature.
Likewise, people in product development start with an idea for a useful product and work to create it. But that is a bit different from research as an intellectual activity. But no one looked in the literature for the problem "How do you create an iPod?" back in the day. The creation of the problem itself was an important part of the process. _

- 1 There was the guy who was late to his lecture and wrote down the problem on the board... Spent 3 weeks doing the “homework” ... When he submitted the solution the prof was rather surprised - that “homework” was him explaining an unsolved problem :) iirc it was CalTech or MIT... but the moral don’t be late... – Solar Mike Commented Sep 16, 2019 at 4:23
Feynman noticing the periodicity of wobble and rotation of a dinner plate. The fractional quantum Hall effect. HTSC cuprates. Initial discovery of superconductivity. Michaelson Morely. Many discoveries of new plants and animals. Penicillin. Teflon. The discovery of the Americas.
- 11 I would add lasers. In the 1960's nobody knew how many cats would enjoy chasing a spot of light. – Patricia Shanahan Commented Sep 15, 2019 at 20:39
- 1 Check out the book Laser by Nick Taylor. It was a big innovation but I'm not so clear that it was really something people were not seeing as a path to pursue. The discovery was contested. And it was building on designs and ideas in interferometry. And people did see the value in a coherent light source. – guest Commented Sep 15, 2019 at 20:43
- @PatriciaShanahan you don’t need a laser - cats will chase torch beams no problem, cheaper and less dangerous... – Solar Mike Commented Sep 15, 2019 at 21:39
The example that popped into my head was the discovery of the muon , about which I. I. Rabi famously quipped, "Who ordered that?" No one had predicted that any such particle would exist, and we still have no idea why it exists (more precisely, why there are three copies of all of the fundamental fermions). They were looking for pions , which happen to have a similar mass but are otherwise unrelated.
Since their discovery muons have seen a few practical applications, such as muon tomography .
Probably very common in mathematics, e.g., the Radon transform which was first described in 1917 and found its major applications in the 1960s with the invention of computer tomography.
Not the answer you're looking for? Browse other questions tagged research-process .
- Featured on Meta
- Bringing clarity to status tag usage on meta sites
- We've made changes to our Terms of Service & Privacy Policy - July 2024
- Announcing a change to the data-dump process
Hot Network Questions
- Why is Excel not counting time with COUNTIF?
- When would it be legal to ask back (parts of) the salary?
- Would several years of appointment as a lecturer hurt you when you decide to go for a tenure-track position later on?
- Short story about a committee planning to eliminate 1 in 10 people
- How to interpret coefficients of logistic regression using natural cubic splines?
- Why does editing '/etc/shells' file using 'sudo open' show an error saying I don't own the file?
- Why did Resolve[] fail to verify a statement whose counter-example was proven to be nonexistant by FindInstance[]?
- Should I pay off my mortgage if the cash is available?
- How to Show a Functional Has Infinitely Many Minimizers?
- The minimal Anti-Sudoku
- What mode of transport is ideal for the cold post-apocalypse?
- Does the First Amendment protect deliberately publicizing the incorrect date for an election?
- How are USB-C cables so thin?
- Generating a normally distributed variable using a known range, but an unknown mean, in R
- I need to better understand this clause in an independent contract agreement for Waiverability:
- How can I cross an overpass in "Street View" without being dropped to the roadway below?
- How much air escapes into space every day, and how long before it makes Earth air pressure too low for humans to breathe?
- I submitted a paper and later realised one reference was missing, although I had written the authors in the body text. What could happen?
- Cutting a 27×27 square into incomparable rectangles
- A study on the speed of gravity
- A post-apocalyptic short story where very sick people from our future save people in our timeline that would be killed in plane crashes
- Why does Air Force Two lack a tail number?
- If there is no free will, doesn't that provide a framework for an ethical model?
- How do I loosen this nut of my toilet lid?

IMAGES
COMMENTS
After many years of research, they found the solution at last/ at the end. My grammar book says answer to the above sentence is at last and I know both 'at last' and 'at the end' are same in meaning. Can you please tell me subtle difference between the two clauses? :oops:
When it comes to research, you never really know in which fantastic directions it might take you. A discovery in one research area can, many years later, change the world in another.
The many faces of scientific research. First, science is a complex endeavor involving multiple goals and associated activities. Some scientists search for the causes underlying some observable ...
Unrealistic, outdated ideas that idealize science can set the public up to distrust scientists and the research process. A philosopher of science describes 3 aspects of how science really gets done.
Before you can solve a problem, you need to know what exactly you're trying to solve. Unfortunately, too many of us want to rush to conclusions before clearly understanding the problem. The ...
Before we can solve a problem, we need to know exactly what the problem is, and we should put a good amount of thinking and resources into understanding it.
We've covered science for 100 years. Here's how it has — and hasn't — changed There's more detail and sophistication, but some of the questions remain the same
Have you ever wondered how research works? How scientists make discoveries about our health and the world around us? Whether they're studying plants, animals, humans, or something else in our world, they follow the scientific method. But this method isn't always—or even usually—a straight line, and often the answers are unexpected and lead to more questions. Let's dive in to see how ...
Learn how to use research effectively to find solutions and make decisions for any problem. Follow these six steps and tips to improve your problem solving skills.
About four billion years ago, Earth resembled the set of a summer sci-fi blockbuster. ... Now, new research zeroes in on how lightning strikes may have served as a vital spark, transforming the atmosphere of early Earth into a hotbed of chemical activity. ... They found that carbon dioxide could be reduced to carbon monoxide and formic acid ...
Research is often described as a problem-solving activity, and as a result, descriptions of problems and solutions are an essential part of the scientific discourse used to describe research activity. We present an automatic classifier that, given a phrase ...
The experiment is one of many novel—and, yes, creative—ways that psychologists are studying the science of creativity. At an individual level, creativity can lead to personal fulfillment and positive academic and professional outcomes, and even be therapeutic. People take pleasure in creative thoughts, research suggests—even if they don't think of themselves as especially creative ...
Interdisciplinary research happens when researchers from different fields come together to pose a challenge that wouldn't be possible in isolation.
Research is often described as a problem-solving activity, and as a result, descriptions of problems and solutions are an essential part of the scientific discourse used to describe research activity. We present an automatic classifier that, given a phrase that may or may not be a description of a scientific problem or a solution, makes a binary decision about problemhood and solutionhood of ...
Learn about important problem-solving steps, including defining a problem, understanding its cause, developing alternative solutions and choosing a solution.
Reprint: R1209F The rigor with which a problem is defined is the most important factor in finding a good solution. Many organizations, however, are not proficient at articulating their problems ...
Insight is the sudden a ha! solution to a problem, the birth of a new idea to simplify a complex situation. Solutions found through insight are often more incisive than those from step-by-step analysis. A quick solution process requires insight to select productive moves at different stages of the problem-solving cycle.
They said they had found the smothered mate solution and insisted they were searching for a shorter one, to no avail.
The notions of science and scientific research are discussed and the motivations for doing research are analyzed. Research can span a broad range of approaches, from purely theoretical to practice-oriented; different approaches often coexist and fertilize each other....
After 10 Years, 'Many Labs' Comes to an End - But Its Success Is Replicable. Brian Nosek, a professor of psychology at the University of Virginia who studies human biases, was a graduate student in the 1990s when he first started thinking closely about one of science's open secrets: failure to replicate research findings is a common ...
Step 2: Find Background Information After you identify your research topic and some keywords that describe it, find and read articles in subject encyclopedias, dictionaries, and handbooks. These articles will help you understand the context (historical, cultural, disciplinary) of your topic. They are the foundation supporting further research. The most common background sources are subject ...
Training oneself to think like an entrepreneur means learning the steps to evaluating a challenge: clarify, ideate, develop, implement, and evaluate (Fi...
In a study published in Nature in January 2024, researchers reported that they actually found examples of photosynthesis in microfossils in an ancient rock formation from about 1.75 billion years ago.
Every day people do research as they gather information to learn about something of interest. In the scientific world, however, research means something different than simply gathering information. Scientific research is characterized by its careful planning and...
The phrase "by the end" is used to indicate that something was discovered or achieved after a certain period of time or at the conclusion of a process. In this context, it conveys that the solution was found after many years of research, marking the culmination of the research efforts.
Translate After many years of research, they found the solution. See Spanish-English translations with audio pronunciations, examples, and word-by-word explanations.
Republicans are questioning Minnesota Gov. Tim Walz's characterization of his time in uniform and his departure from military service.
Almost all research starts out without a well defined problem that is known in the literature. There are classic unsolved problems, of course, and people work on those, but graduate students are advised to avoid them. If a problem has been known for a while it has probably been worked on and the solution is (a) likely pretty hard and (b) the field hasn't yet developed the base to make a ...