

Developmental Research Designs
Margaret Clark-Plaskie; Lumen Learning; Angela Lukowski; Helen Milojevich; and Diana Lang
- Compare advantages and disadvantages of developmental research designs (cross-sectional, longitudinal, and sequential)
- Describe challenges associated with conducting research in lifespan development
Now you know about some tools used to conduct research about human development. Remember, research methods are tools that are used to collect information. But it is easy to confuse research methods and research design. Research design is the strategy or blueprint for deciding how to collect and analyze information. Research design dictates which methods are used and how. Developmental research designs are techniques used particularly in lifespan development research. When we are trying to describe development and change, the research designs become especially important because we are interested in what changes and what stays the same with age. These techniques try to examine how age, cohort, gender, and social class impact development. [1]
Cross-sectional designs
The majority of developmental studies use cross-sectional designs because they are less time-consuming and less expensive than other developmental designs. Cross-sectional research designs are used to examine behavior in participants of different ages who are tested at the same point in time (Figure 1). Let’s suppose that researchers are interested in the relationship between intelligence and aging. They might have a hypothesis (an educated guess, based on theory or observations) that intelligence declines as people get older. The researchers might choose to give a certain intelligence test to individuals who are 20 years old, individuals who are 50 years old, and individuals who are 80 years old at the same time and compare the data from each age group. This research is cross-sectional in design because the researchers plan to examine the intelligence scores of individuals of different ages within the same study at the same time; they are taking a “cross-section” of people at one point in time. Let’s say that the comparisons find that the 80-year-old adults score lower on the intelligence test than the 50-year-old adults, and the 50-year-old adults score lower on the intelligence test than the 20-year-old adults. Based on these data, the researchers might conclude that individuals become less intelligent as they get older. Would that be a valid (accurate) interpretation of the results?
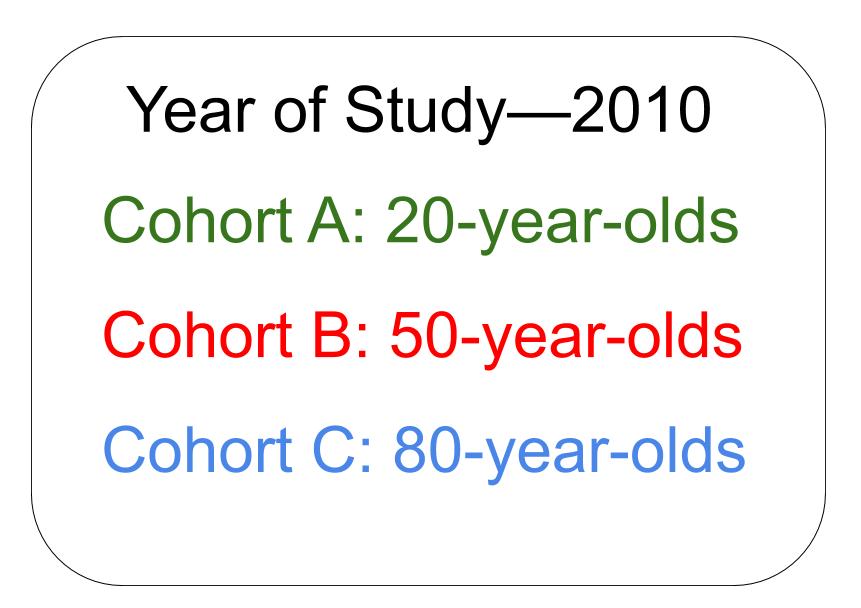
No, that would not be a valid conclusion because the researchers did not follow individuals as they aged from 20 to 50 to 80 years old. One of the primary limitations of cross-sectional research is that the results yield information about age differences not necessarily changes with age or over time. That is, although the study described above can show that in 2010, the 80-year-olds scored lower on the intelligence test than the 50-year-olds, and the 50-year-olds scored lower on the intelligence test than the 20-year-olds, the data used to come up with this conclusion were collected from different individuals (or groups of individuals). It could be, for instance, that when these 20-year-olds get older (50 and eventually 80), they will still score just as high on the intelligence test as they did at age 20. In a similar way, maybe the 80-year-olds would have scored relatively low on the intelligence test even at ages 50 and 20; the researchers don’t know for certain because they did not follow the same individuals as they got older.
It is also possible that the differences found between the age groups are not due to age, per se, but due to cohort effects. The 80-year-olds in this 2010 research grew up during a particular time and experienced certain events as a group. They were born in 1930 and are part of the Traditional or Silent Generation. The 50-year-olds were born in 1960 and are members of the Baby Boomer cohort. The 20-year-olds were born in 1990 and are part of the Millennial or Gen Y Generation. What kinds of things did each of these cohorts experience that the others did not experience or at least not in the same ways?
You may have come up with many differences between these cohorts’ experiences, such as living through certain wars, political and social movements, economic conditions, advances in technology, changes in health and nutrition standards, etc. There may be particular cohort differences that could especially influence their performance on intelligence tests, such as education level and use of computers. That is, many of those born in 1930 probably did not complete high school; those born in 1960 may have high school degrees, on average, but the majority did not attain college degrees; the young adults are probably current college students. And this is not even considering additional factors such as gender, race, or socioeconomic status. The young adults are used to taking tests on computers, but the members of the other two cohorts did not grow up with computers and may not be as comfortable if the intelligence test is administered on computers. These factors could have been a factor in the research results.
Another disadvantage of cross-sectional research is that it is limited to one time of measurement. Data are collected at one point in time and it’s possible that something could have happened in that year in history that affected all of the participants, although possibly each cohort may have been affected differently. Just think about the mindsets of participants in research that was conducted in the United States right after the terrorist attacks on September 11, 2001.
Longitudinal research designs
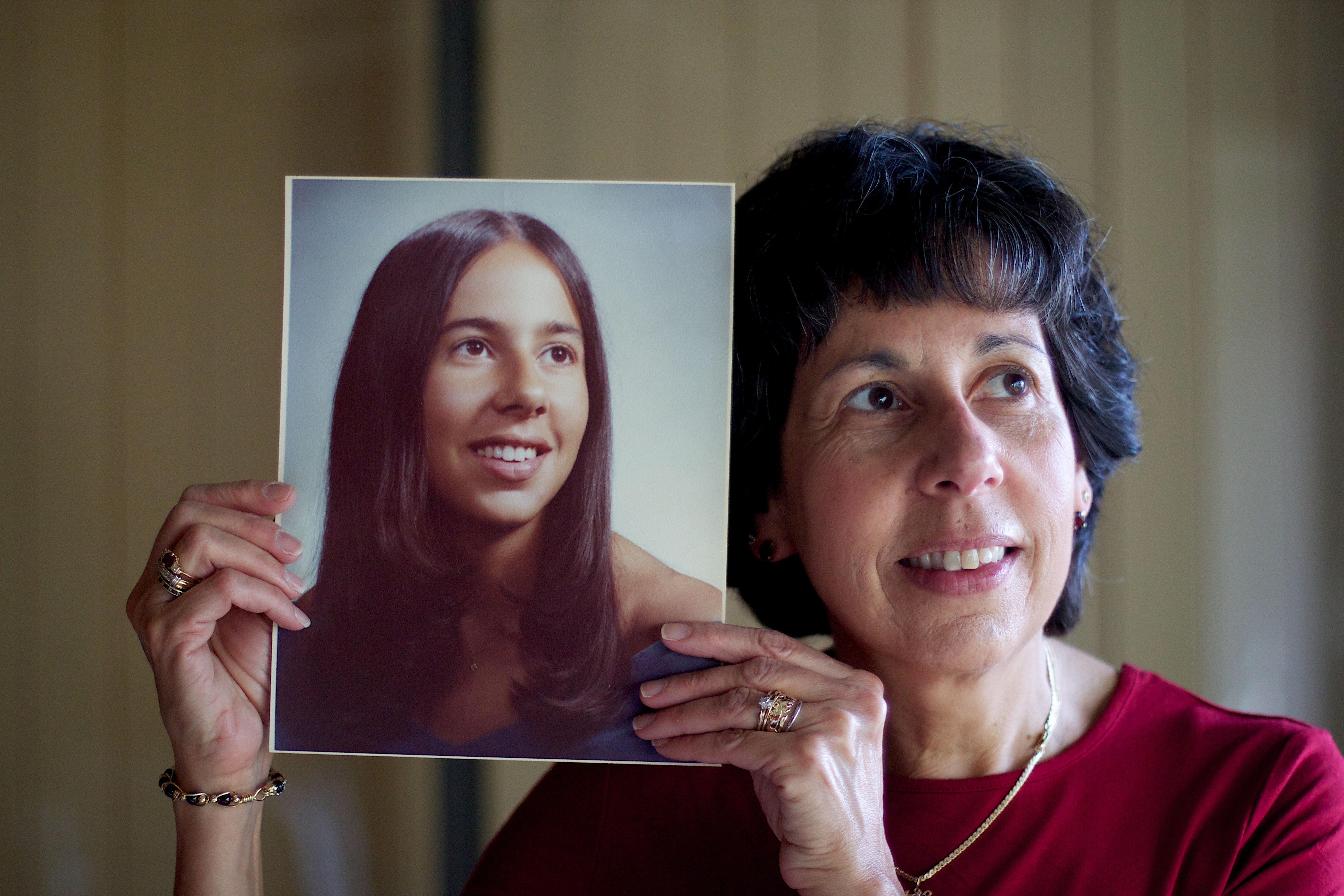
Longitudinal research involves beginning with a group of people who may be of the same age and background (cohort) and measuring them repeatedly over a long period of time (Figure 2 & 3). One of the benefits of this type of research is that people can be followed through time and be compared with themselves when they were younger; therefore changes with age over time are measured. What would be the advantages and disadvantages of longitudinal research? Problems with this type of research include being expensive, taking a long time, and subjects dropping out over time. Think about the film, 63 Up , part of the Up Series mentioned earlier, which is an example of following individuals over time. In the videos, filmed every seven years, you see how people change physically, emotionally, and socially through time; and some remain the same in certain ways, too. But many of the participants really disliked being part of the project and repeatedly threatened to quit; one disappeared for several years; another died before her 63rd year. Would you want to be interviewed every seven years? Would you want to have it made public for all to watch?
Longitudinal research designs are used to examine behavior in the same individuals over time. For instance, with our example of studying intelligence and aging, a researcher might conduct a longitudinal study to examine whether 20-year-olds become less intelligent with age over time. To this end, a researcher might give an intelligence test to individuals when they are 20 years old, again when they are 50 years old, and then again when they are 80 years old. This study is longitudinal in nature because the researcher plans to study the same individuals as they age. Based on these data, the pattern of intelligence and age might look different than from the cross-sectional research; it might be found that participants’ intelligence scores are higher at age 50 than at age 20 and then remain stable or decline a little by age 80. How can that be when cross-sectional research revealed declines in intelligence with age?

Since longitudinal research happens over a period of time (which could be short term, as in months, but is often longer, as in years), there is a risk of attrition. Attrition occurs when participants fail to complete all portions of a study. Participants may move, change their phone numbers, die, or simply become disinterested in participating over time. Researchers should account for the possibility of attrition by enrolling a larger sample into their study initially, as some participants will likely drop out over time. There is also something known as selective attrition— this means that certain groups of individuals may tend to drop out. It is often the least healthy, least educated, and lower socioeconomic participants who tend to drop out over time. That means that the remaining participants may no longer be representative of the whole population, as they are, in general, healthier, better educated, and have more money. This could be a factor in why our hypothetical research found a more optimistic picture of intelligence and aging as the years went by. What can researchers do about selective attrition? At each time of testing, they could randomly recruit more participants from the same cohort as the original members, to replace those who have dropped out.
The results from longitudinal studies may also be impacted by repeated assessments. Consider how well you would do on a math test if you were given the exact same exam every day for a week. Your performance would likely improve over time, not necessarily because you developed better math abilities, but because you were continuously practicing the same math problems. This phenomenon is known as a practice effect. Practice effects occur when participants become better at a task over time because they have done it again and again (not due to natural psychological development). So our participants may have become familiar with the intelligence test each time (and with the computerized testing administration).
Another limitation of longitudinal research is that the data are limited to only one cohort. As an example, think about how comfortable the participants in the 2010 cohort of 20-year-olds are with computers. Since only one cohort is being studied, there is no way to know if findings would be different from other cohorts. In addition, changes that are found as individuals age over time could be due to age or to time of measurement effects. That is, the participants are tested at different periods in history, so the variables of age and time of measurement could be confounded (mixed up). For example, what if there is a major shift in workplace training and education between 2020 and 2040 and many of the participants experience a lot more formal education in adulthood, which positively impacts their intelligence scores in 2040? Researchers wouldn’t know if the intelligence scores increased due to growing older or due to a more educated workforce over time between measurements.
Sequential research designs
Sequential research designs include elements of both longitudinal and cross-sectional research designs. Similar to longitudinal designs, sequential research features participants who are followed over time; similar to cross-sectional designs, sequential research includes participants of different ages. This research design is also distinct from those that have been discussed previously in that individuals of different ages are enrolled into a study at various points in time to examine age-related changes, development within the same individuals as they age, and to account for the possibility of cohort and/or time of measurement effects. In 1965, Schaie [2] (a leading theorist and researcher on intelligence and aging), described particular sequential designs: cross-sequential, cohort sequential, and time-sequential. The differences between them depended on which variables were focused on for analyses of the data (data could be viewed in terms of multiple cross-sectional designs or multiple longitudinal designs or multiple cohort designs). Ideally, by comparing results from the different types of analyses, the effects of age, cohort, and time in history could be separated out.
Consider, once again, our example of intelligence and aging. In a study with a sequential design, a researcher might recruit three separate groups of participants (Groups A, B, and C). Group A would be recruited when they are 20 years old in 2010 and would be tested again when they are 50 and 80 years old in 2040 and 2070, respectively (similar in design to the longitudinal study described previously). Group B would be recruited when they are 20 years old in 2040 and would be tested again when they are 50 years old in 2070. Group C would be recruited when they are 20 years old in 2070 and so on (Figure 4).
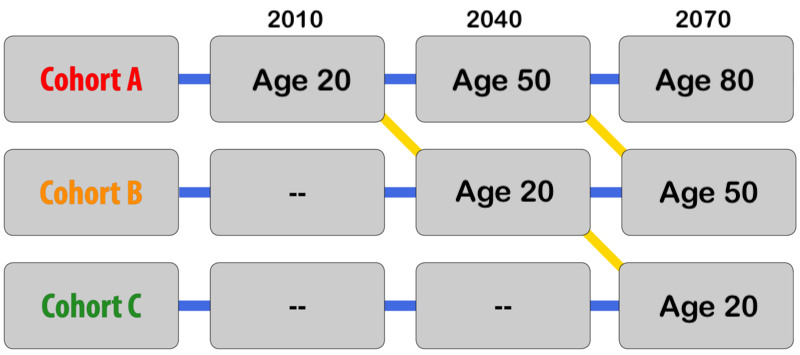
Studies with sequential designs are powerful because they allow for both longitudinal and cross-sectional comparisons—changes and/or stability with age over time can be measured and compared with differences between age and cohort groups. This research design also allows for the examination of cohort and time of measurement effects. For example, the researcher could examine the intelligence scores of 20-year-olds in different times in history and different cohorts (follow the yellow diagonal lines in figure 3). This might be examined by researchers who are interested in sociocultural and historical changes (because we know that lifespan development is multidisciplinary). One way of looking at the usefulness of the various developmental research designs was described by Schaie and Baltes [3] : cross-sectional and longitudinal designs might reveal change patterns while sequential designs might identify developmental origins for the observed change patterns.
Since they include elements of longitudinal and cross-sectional designs, sequential research has many of the same strengths and limitations as these other approaches. For example, sequential work may require less time and effort than longitudinal research (if data are collected more frequently than over the 30-year spans in our example) but more time and effort than cross-sectional research. Although practice effects may be an issue if participants are asked to complete the same tasks or assessments over time, attrition may be less problematic than what is commonly experienced in longitudinal research since participants may not have to remain involved in the study for such a long period of time.
When considering the best research design to use in their research, scientists think about their main research question and the best way to come up with an answer. A table of advantages and disadvantages for each of the described research designs is provided here to help you as you consider what sorts of studies would be best conducted using each of these different approaches.
Research Design | Advantages | Disadvantages |
---|---|---|
Cross-Sectional | ||
Longitudinal | ||
Sequential |
Challenges Associated with Conducting Developmental Research
The previous sections describe research tools to assess development across the lifespan, as well as the ways that research designs can be used to track age-related changes and development over time. Before you begin conducting developmental research, however, you must also be aware that testing individuals of certain ages (such as infants and children) or making comparisons across ages (such as children compared to teens) comes with its own unique set of challenges. In the final section of this module, let’s look at some of the main issues that are encountered when conducting developmental research, namely ethical concerns, recruitment issues, and participant attrition.
Ethical Concerns
As a student of the social sciences, you may already know that Institutional Review Boards (IRBs) must review and approve all research projects that are conducted at universities, hospitals, and other institutions (each broad discipline or field, such as psychology or social work, often has its own code of ethics that must also be followed, regardless of institutional affiliation). An IRB is typically a panel of experts who read and evaluate proposals for research. IRB members want to ensure that the proposed research will be carried out ethically and that the potential benefits of the research outweigh the risks and potential harm (psychological as well as physical harm) for participants.
What you may not know though, is that the IRB considers some groups of participants to be more vulnerable or at-risk than others. Whereas university students are generally not viewed as vulnerable or at-risk, infants and young children commonly fall into this category. What makes infants and young children more vulnerable during research than young adults? One reason infants and young children are perceived as being at increased risk is due to their limited cognitive capabilities, which makes them unable to state their willingness to participate in research or tell researchers when they would like to drop out of a study. For these reasons, infants and young children require special accommodations as they participate in the research process. Similar issues and accommodations would apply to adults who are deemed to be of limited cognitive capabilities.
When thinking about special accommodations in developmental research, consider the informed consent process. If you have ever participated in scientific research, you may know through your own experience that adults commonly sign an informed consent statement (a contract stating that they agree to participate in research) after learning about a study. As part of this process, participants are informed of the procedures to be used in the research, along with any expected risks or benefits. Infants and young children cannot verbally indicate their willingness to participate, much less understand the balance of potential risks and benefits. As such, researchers are oftentimes required to obtain written informed consent from the parent or legal guardian of the child participant, an adult who is almost always present as the study is conducted. In fact, children are not asked to indicate whether they would like to be involved in a study at all (a process known as assent) until they are approximately seven years old. Because infants and young children cannot easily indicate if they would like to discontinue their participation in a study, researchers must be sensitive to changes in the state of the participant (determining whether a child is too tired or upset to continue) as well as to parent desires (in some cases, parents might want to discontinue their involvement in the research). As in adult studies, researchers must always strive to protect the rights and well-being of the minor participants and their parents when conducting developmental research.
This video from the US Department of Health and Human Services provides an overview of the Institutional Review Board process.
You can view the transcript for “How IRBs Protect Human Research Participants” here (opens in new window) .
Recruitment
An additional challenge in developmental science is participant recruitment. Recruiting university students to participate in adult studies is typically easy. Many colleges and universities offer extra credit for participation in research and have locations such as bulletin boards and school newspapers where research can be advertised. Unfortunately, young children cannot be recruited by making announcements in Introduction to Psychology courses, by posting ads on campuses, or through online platforms such as Amazon Mechanical Turk. Given these limitations, how do researchers go about finding infants and young children to be in their studies?
The answer to this question varies along multiple dimensions. Researchers must consider the number of participants they need and the financial resources available to them, among other things. Location may also be an important consideration. Researchers who need large numbers of infants and children may attempt to recruit them by obtaining infant birth records from the state, county, or province in which they reside. Some areas make this information publicly available for free, whereas birth records must be purchased in other areas (and in some locations birth records may be entirely unavailable as a recruitment tool). If birth records are available, researchers can use the obtained information to call families by phone or mail them letters describing possible research opportunities. All is not lost if this recruitment strategy is unavailable, however. Researchers can choose to pay a recruitment agency to contact and recruit families for them. Although these methods tend to be quick and effective, they can also be quite expensive. More economical recruitment options include posting advertisements and fliers in locations frequented by families, such as mommy-and-me classes, local malls, and preschools or daycare centers. Researchers can also utilize online social media outlets like Facebook, which allows users to post recruitment advertisements for a small fee. Of course, each of these different recruitment techniques requires IRB approval. And if children are recruited and/or tested in school settings, permission would need to be obtained ahead of time from teachers, schools, and school districts (as well as informed consent from parents or guardians).
And what about the recruitment of adults? While it is easy to recruit young college students to participate in research, some would argue that it is too easy and that college students are samples of convenience. They are not randomly selected from the wider population, and they may not represent all young adults in our society (this was particularly true in the past with certain cohorts, as college students tended to be mainly white males of high socioeconomic status). In fact, in the early research on aging, this type of convenience sample was compared with another type of convenience sample—young college students tended to be compared with residents of nursing homes! Fortunately, it didn’t take long for researchers to realize that older adults in nursing homes are not representative of the older population; they tend to be the oldest and sickest (physically and/or psychologically). Those initial studies probably painted an overly negative view of aging, as young adults in college were being compared to older adults who were not healthy, had not been in school nor taken tests in many decades, and probably did not graduate high school, let alone college. As we can see, recruitment and random sampling can be significant issues in research with adults, as well as infants and children. For instance, how and where would you recruit middle-aged adults to participate in your research?
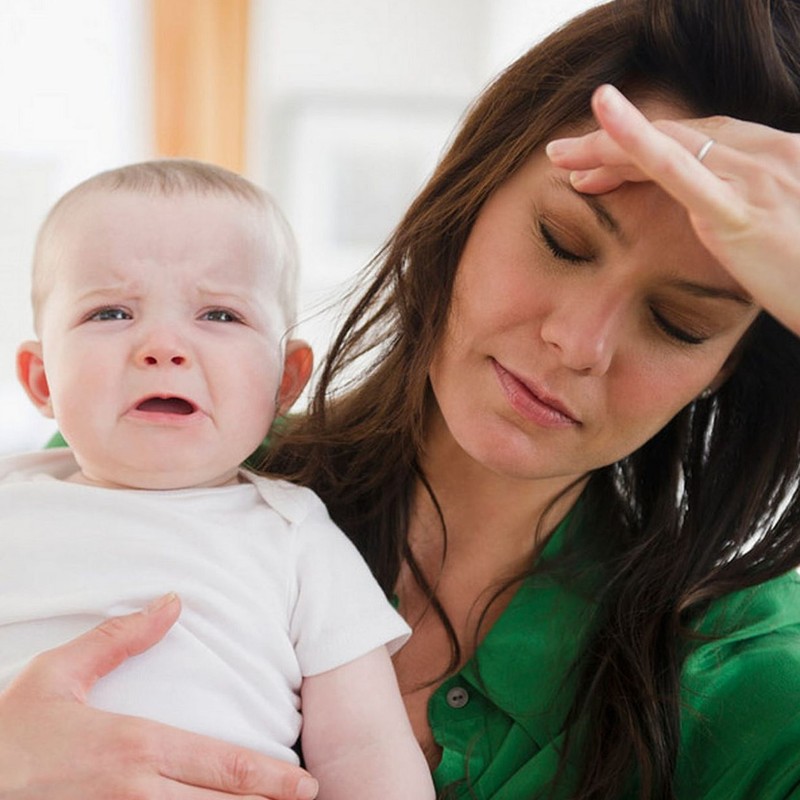
Another important consideration when conducting research with infants and young children is attrition . Although attrition is quite common in longitudinal research in particular (see the previous section on longitudinal designs for an example of high attrition rates and selective attrition in lifespan developmental research), it is also problematic in developmental science more generally, as studies with infants and young children tend to have higher attrition rates than studies with adults. For example, high attrition rates in ERP (event-related potential, which is a technique to understand brain function) studies oftentimes result from the demands of the task: infants are required to sit still and have a tight, wet cap placed on their heads before watching still photographs on a computer screen in a dark, quiet room (Figure 5).
In other cases, attrition may be due to motivation (or a lack thereof). Whereas adults may be motivated to participate in research in order to receive money or extra course credit, infants and young children are not as easily enticed. In addition, infants and young children are more likely to tire easily, become fussy, and lose interest in the study procedures than are adults. For these reasons, research studies should be designed to be as short as possible – it is likely better to break up a large study into multiple short sessions rather than cram all of the tasks into one long visit to the lab. Researchers should also allow time for breaks in their study protocols so that infants can rest or have snacks as needed. Happy, comfortable participants provide the best data.
Conclusions
Lifespan development is a fascinating field of study – but care must be taken to ensure that researchers use appropriate methods to examine human behavior, use the correct experimental design to answer their questions, and be aware of the special challenges that are part-and-parcel of developmental research. After reading this module, you should have a solid understanding of these various issues and be ready to think more critically about research questions that interest you. For example, what types of questions do you have about lifespan development? What types of research would you like to conduct? Many interesting questions remain to be examined by future generations of developmental scientists – maybe you will make one of the next big discoveries!
- attrition : occurs when participants fail to complete all portions of a study
- cross-sectional research : used to examine behavior in participants of different ages who are tested at the same point in time; may confound age and cohort differences
- i nformed consent : a process of informing a research participant what to expect during a study, any risks involved, and the implications of the research, and then obtaining the person’s agreement to participate
- Institutional Review Boards (IRBs) : a panel of experts who review research proposals for any research to be conducted in association with the institution (for example, a university)
- longitudinal research : studying a group of people who may be of the same age and background (cohort), and measuring them repeatedly over a long period of time; may confound age and time of measurement effects
- research design : the strategy or blueprint for deciding how to collect and analyze information; dictates which methods are used and how
- selective attrition : certain groups of individuals may tend to drop out more frequently resulting in the remaining participants no longer being representative of the whole population
- sequential research design : combines aspects of cross-sectional and longitudinal designs, but also adding new cohorts at different times of measurement; allows for analyses to consider effects of age, cohort, time of measurement, and socio-historical change
- This chapter was adapted from Lumen Learning's Lifespan Development , created by Margaret Clark-Plaskie for Lumen Learning and adapted from Research Methods in Developmental Psychology by Angela Lukowski and Helen Milojevich for Noba Psychology, available under a Creative Commons NonCommercial Sharealike Attribution license . ↵
- Schaie, K. W. (1965). A general model for the study of developmental problems. Psychological Bulletin, 64 (2), 92-107. ↵
- Schaie, K.W. & Baltes, B.P. (1975). On sequential strategies in developmental research: Description or Explanation. Human Development, 18, 384-390. ↵
Developmental Research Designs Copyright © 2022 by Margaret Clark-Plaskie; Lumen Learning; Angela Lukowski; Helen Milojevich; and Diana Lang is licensed under a Creative Commons Attribution-NonCommercial-ShareAlike 4.0 International License , except where otherwise noted.
Want to create or adapt books like this? Learn more about how Pressbooks supports open publishing practices.
3.4 Developmental Research Designs
Learning objectives.
- Compare advantages and disadvantages of developmental research designs (cross-sectional, longitudinal, and sequential)
Now you know about some tools used to conduct research about human development. Remember, research methods are tools that are used to collect information. But it is easy to confuse research methods and research design. Research design is the strategy or blueprint for deciding how to collect and analyze information. Research design dictates which methods are used and how. Developmental research designs are techniques used particularly in lifespan development research. When we are trying to describe development and change, the research designs become especially important because we are interested in what changes and what stays the same with age. These techniques try to examine how age, cohort, gender, and social class impact development.
Cross-sectional designs
The majority of developmental studies use cross-sectional designs because they are less time-consuming and less expensive than other developmental designs. Cross-sectional research designs are used to examine behavior in participants of different ages who are tested at the same point in time. Let’s suppose that researchers are interested in the relationship between intelligence and aging. They might have a hypothesis (an educated guess, based on theory or observations) that intelligence declines as people get older. The researchers might choose to give a certain intelligence test to individuals who are 20 years old, individuals who are 50 years old, and individuals who are 80 years old at the same time and compare the data from each age group. This research is cross-sectional in design because the researchers plan to examine the intelligence scores of individuals of different ages within the same study at the same time; they are taking a “cross-section” of people at one point in time. Let’s say that the comparisons find that the 80-year-old adults score lower on the intelligence test than the 50-year-old adults, and the 50-year-old adults score lower on the intelligence test than the 20-year-old adults. Based on these data, the researchers might conclude that individuals become less intelligent as they get older. Would that be a valid (accurate) interpretation of the results?
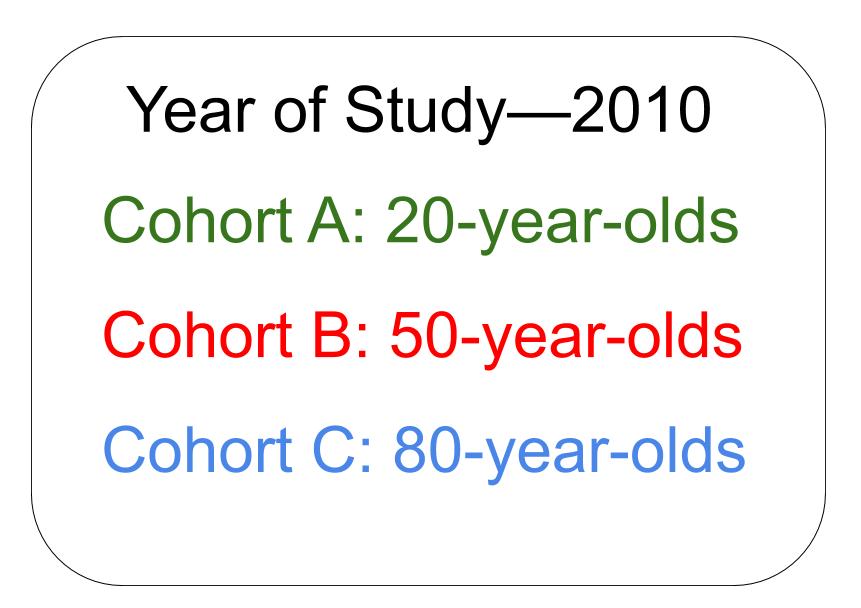
No, that would not be a valid conclusion because the researchers did not follow individuals as they aged from 20 to 50 to 80 years old. One of the primary limitations of cross-sectional research is that the results yield information about age differences not necessarily changes with age or over time. That is, although the study described above can show that in 2010, the 80-year-olds scored lower on the intelligence test than the 50-year-olds, and the 50-year-olds scored lower on the intelligence test than the 20-year-olds, the data used to come up with this conclusion were collected from different individuals (or groups of individuals). It could be, for instance, that when these 20-year-olds get older (50 and eventually 80), they will still score just as high on the intelligence test as they did at age 20. In a similar way, maybe the 80-year-olds would have scored relatively low on the intelligence test even at ages 50 and 20; the researchers don’t know for certain because they did not follow the same individuals as they got older.
It is also possible that the differences found between the age groups are not due to age, per se, but due to cohort effects. The 80-year-olds in this 2010 research grew up during a particular time and experienced certain events as a group. They were born in 1930 and are part of the Traditional or Silent Generation. The 50-year-olds were born in 1960 and are members of the Baby Boomer cohort. The 20-year-olds were born in 1990 and are part of the Millennial or Gen Y Generation. What kinds of things did each of these cohorts experience that the others did not experience or at least not in the same ways?
You may have come up with many differences between these cohorts’ experiences, such as living through certain wars, political and social movements, economic conditions, advances in technology, changes in health and nutrition standards, etc. There may be particular cohort differences that could especially influence their performance on intelligence tests, such as education level and use of computers. That is, many of those born in 1930 probably did not complete high school; those born in 1960 may have high school degrees, on average, but the majority did not attain college degrees; the young adults are probably current college students. And this is not even considering additional factors such as gender, race, or socioeconomic status. The young adults are used to taking tests on computers, but the members of the other two cohorts did not grow up with computers and may not be as comfortable if the intelligence test is administered on computers. These factors could have been a factor in the research results.
Another disadvantage of cross-sectional research is that it is limited to one time of measurement. Data are collected at one point in time and it’s possible that something could have happened in that year in history that affected all of the participants, although possibly each cohort may have been affected differently. Just think about the mindsets of participants in research that was conducted in the United States right after the terrorist attacks on September 11, 2001.
Longitudinal research designs
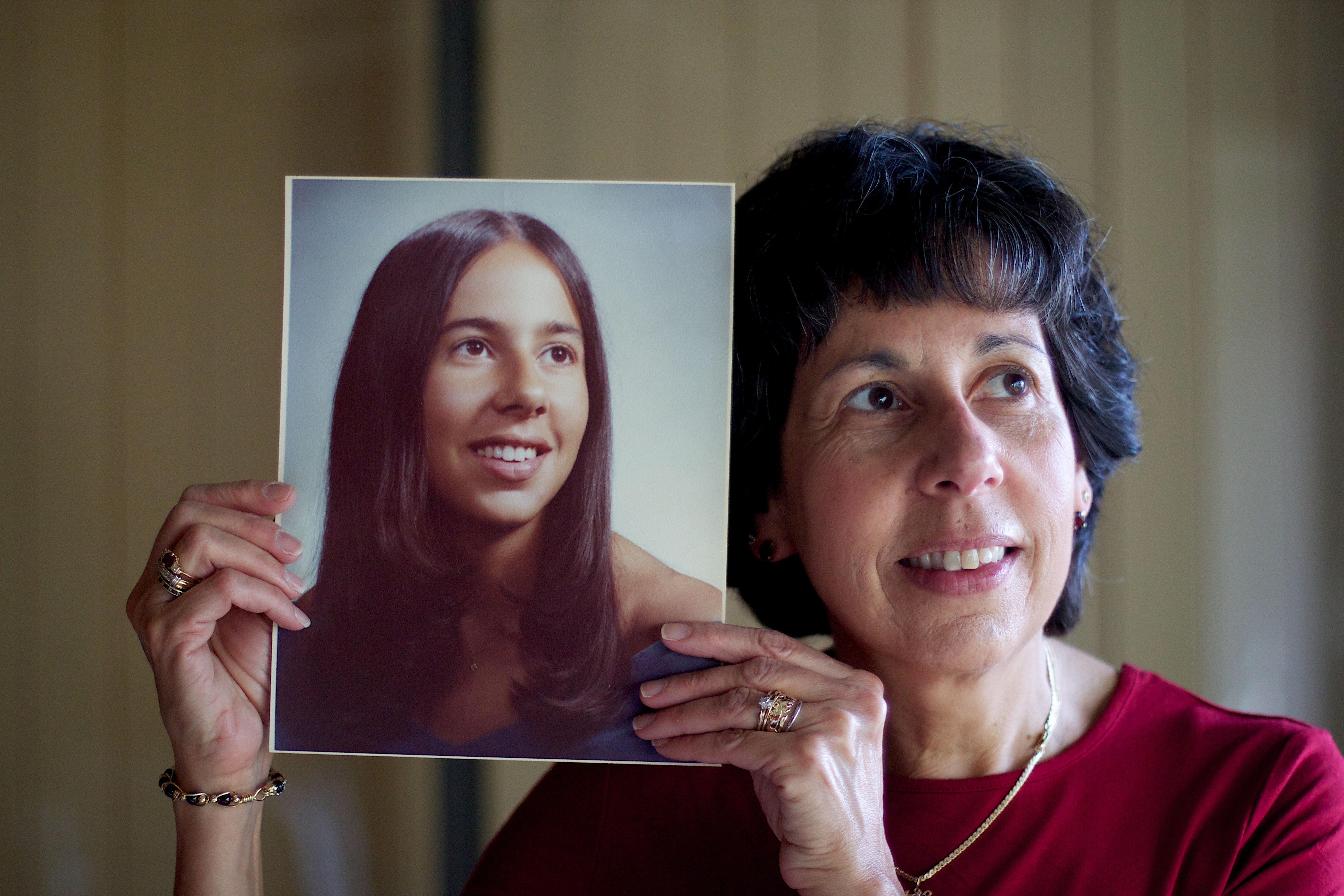
Longitudinal research involves beginning with a group of people who may be of the same age and background (cohort) and measuring them repeatedly over a long period of time. One of the benefits of this type of research is that people can be followed through time and be compared with themselves when they were younger; therefore changes with age over time are measured. What would be the advantages and disadvantages of longitudinal research? Problems with this type of research include being expensive, taking a long time, and participants dropping out over time. Think about the film, 63 Up , part of the Up Series mentioned earlier, which is an example of following individuals over time. In the videos, filmed every seven years, you see how people change physically, emotionally, and socially through time; and some remain the same in certain ways, too. But many of the participants really disliked being part of the project and repeatedly threatened to quit; one disappeared for several years; another died before her 63rd year. Would you want to be interviewed every seven years? Would you want to have it made public for all to watch?
Longitudinal research designs are used to examine behavior in the same individuals over time. For instance, with our example of studying intelligence and aging, a researcher might conduct a longitudinal study to examine whether 20-year-olds become less intelligent with age over time. To this end, a researcher might give an intelligence test to individuals when they are 20 years old, again when they are 50 years old, and then again when they are 80 years old. This study is longitudinal in nature because the researcher plans to study the same individuals as they age. Based on these data, the pattern of intelligence and age might look different than from the cross-sectional research; it might be found that participants’ intelligence scores are higher at age 50 than at age 20 and then remain stable or decline a little by age 80. How can that be when cross-sectional research revealed declines in intelligence with age?

Since longitudinal research happens over a period of time (which could be short term, as in months, but is often longer, as in years), there is a risk of attrition. Attrition occurs when participants fail to complete all portions of a study. Participants may move, change their phone numbers, die, or simply become disinterested in participating over time. Researchers should account for the possibility of attrition by enrolling a larger sample into their study initially, as some participants will likely drop out over time. There is also something known as selective attrition— this means that certain groups of individuals may tend to drop out. It is often the least healthy, least educated, and lower socioeconomic participants who tend to drop out over time. That means that the remaining participants may no longer be representative of the whole population, as they are, in general, healthier, better educated, and have more money. This could be a factor in why our hypothetical research found a more optimistic picture of intelligence and aging as the years went by. What can researchers do about selective attrition? At each time of testing, they could randomly recruit more participants from the same cohort as the original members, to replace those who have dropped out.
The results from longitudinal studies may also be impacted by repeated assessments. Consider how well you would do on a math test if you were given the exact same exam every day for a week. Your performance would likely improve over time, not necessarily because you developed better math abilities, but because you were continuously practicing the same math problems. This phenomenon is known as a practice effect. Practice effects occur when participants become better at a task over time because they have done it again and again (not due to natural psychological development). So our participants may have become familiar with the intelligence test each time (and with the computerized testing administration).
Another limitation of longitudinal research is that the data are limited to only one cohort. As an example, think about how comfortable the participants in the 2010 cohort of 20-year-olds are with computers. Since only one cohort is being studied, there is no way to know if findings would be different from other cohorts. In addition, changes that are found as individuals age over time could be due to age or to time of measurement effects. That is, the participants are tested at different periods in history, so the variables of age and time of measurement could be confounded (mixed up). For example, what if there is a major shift in workplace training and education between 2020 and 2040 and many of the participants experience a lot more formal education in adulthood, which positively impacts their intelligence scores in 2040? Researchers wouldn’t know if the intelligence scores increased due to growing older or due to a more educated workforce over time between measurements.
Sequential research designs
Sequential research designs include elements of both longitudinal and cross-sectional research designs. Similar to longitudinal designs, sequential research features participants who are followed over time; similar to cross-sectional designs, sequential research includes participants of different ages. This research design is also distinct from those that have been discussed previously in that individuals of different ages are enrolled into a study at various points in time to examine age-related changes, development within the same individuals as they age, and to account for the possibility of cohort and/or time of measurement effects. In 1965, K. Warner Schaie [1] (a leading theorist and researcher on intelligence and aging), described particular sequential designs: cross-sequential, cohort sequential, and time-sequential. The differences between them depended on which variables were focused on for analyses of the data (data could be viewed in terms of multiple cross-sectional designs or multiple longitudinal designs or multiple cohort designs). Ideally, by comparing results from the different types of analyses, the effects of age, cohort, and time in history could be separated out.
Consider, once again, our example of intelligence and aging. In a study with a sequential design, a researcher might recruit three separate groups of participants (Groups A, B, and C). Group A would be recruited when they are 20 years old in 2010 and would be tested again when they are 50 and 80 years old in 2040 and 2070, respectively (similar in design to the longitudinal study described previously). Group B would be recruited when they are 20 years old in 2040 and would be tested again when they are 50 years old in 2070. Group C would be recruited when they are 20 years old in 2070 and so on.
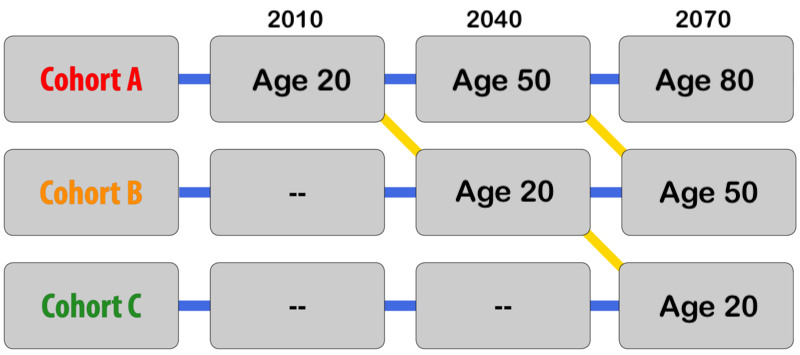
Studies with sequential designs are powerful because they allow for both longitudinal and cross-sectional comparisons—changes and/or stability with age over time can be measured and compared with differences between age and cohort groups. This research design also allows for the examination of cohort and time of measurement effects. For example, the researcher could examine the intelligence scores of 20-year-olds in different times in history and different cohorts (follow the yellow diagonal lines in figure 3). This might be examined by researchers who are interested in sociocultural and historical changes (because we know that lifespan development is multidisciplinary). One way of looking at the usefulness of the various developmental research designs was described by Schaie and Baltes (1975) [2] : cross-sectional and longitudinal designs might reveal change patterns while sequential designs might identify developmental origins for the observed change patterns.
Since they include elements of longitudinal and cross-sectional designs, sequential research has many of the same strengths and limitations as these other approaches. For example, sequential work may require less time and effort than longitudinal research (if data are collected more frequently than over the 30-year spans in our example) but more time and effort than cross-sectional research. Although practice effects may be an issue if participants are asked to complete the same tasks or assessments over time, attrition may be less problematic than what is commonly experienced in longitudinal research since participants may not have to remain involved in the study for such a long period of time.
When considering the best research design to use in their research, scientists think about their main research question and the best way to come up with an answer. A table of advantages and disadvantages for each of the described research designs is provided here to help you as you consider what sorts of studies would be best conducted using each of these different approaches.
Research Design | Advantages | Disadvantages |
---|---|---|
Cross-Sectional | ||
Longitudinal | ||
Sequential |
- “ Developmental Research Designs.” Provided by : Lumen Learning. License : CC BY-NC-SA: Attribution-NonCommercial-ShareAlike
- Psyc 200 Lifespan Psychology. Authored by : Laura Overstreet. Located at : http://opencourselibrary.org/econ-201/ . License : CC BY: Attribution
- Research Designs. Authored by : Christie Napa Scollon. Provided by : Singapore Management University. Project : The Noba Project. License : CC BY-NC-SA: Attribution-NonCommercial-ShareAlike
- Vocabulary and review about correlational research. Provided by : Lumen Learning. Located at : https://courses.lumenlearning.com/waymaker-psychology/wp-admin/post.php?post=1848&action=edit . License : CC BY: Attribution
- Grit: The power of passion and perseverance. Authored by : Angela Lee Duckworth. Provided by : TED. Located at : https://www.ted.com/talks/angela_lee_duckworth_grit_the_power_of_passion_and_perseverance . License : CC BY-NC-ND: Attribution-NonCommercial-NoDerivatives
- Schaie, K.W. (1965). A general model for the study of developmental problems. Psychological Bulletin, 64(2), 92-107. ↵
- Schaie, K.W. & Baltes, B.P. (1975). On sequential strategies in developmental research: Description or Explanation. Human Development, 18: 384-390. ↵
3.4 Developmental Research Designs Copyright © by Meredith Palm is licensed under a Creative Commons Attribution 4.0 International License , except where otherwise noted.
Share This Book

Experimental Research Design — 6 mistakes you should never make!
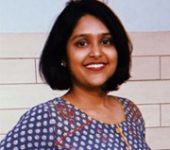
Since school days’ students perform scientific experiments that provide results that define and prove the laws and theorems in science. These experiments are laid on a strong foundation of experimental research designs.
An experimental research design helps researchers execute their research objectives with more clarity and transparency.
In this article, we will not only discuss the key aspects of experimental research designs but also the issues to avoid and problems to resolve while designing your research study.
Table of Contents
What Is Experimental Research Design?
Experimental research design is a framework of protocols and procedures created to conduct experimental research with a scientific approach using two sets of variables. Herein, the first set of variables acts as a constant, used to measure the differences of the second set. The best example of experimental research methods is quantitative research .
Experimental research helps a researcher gather the necessary data for making better research decisions and determining the facts of a research study.
When Can a Researcher Conduct Experimental Research?
A researcher can conduct experimental research in the following situations —
- When time is an important factor in establishing a relationship between the cause and effect.
- When there is an invariable or never-changing behavior between the cause and effect.
- Finally, when the researcher wishes to understand the importance of the cause and effect.
Importance of Experimental Research Design
To publish significant results, choosing a quality research design forms the foundation to build the research study. Moreover, effective research design helps establish quality decision-making procedures, structures the research to lead to easier data analysis, and addresses the main research question. Therefore, it is essential to cater undivided attention and time to create an experimental research design before beginning the practical experiment.
By creating a research design, a researcher is also giving oneself time to organize the research, set up relevant boundaries for the study, and increase the reliability of the results. Through all these efforts, one could also avoid inconclusive results. If any part of the research design is flawed, it will reflect on the quality of the results derived.
Types of Experimental Research Designs
Based on the methods used to collect data in experimental studies, the experimental research designs are of three primary types:
1. Pre-experimental Research Design
A research study could conduct pre-experimental research design when a group or many groups are under observation after implementing factors of cause and effect of the research. The pre-experimental design will help researchers understand whether further investigation is necessary for the groups under observation.
Pre-experimental research is of three types —
- One-shot Case Study Research Design
- One-group Pretest-posttest Research Design
- Static-group Comparison
2. True Experimental Research Design
A true experimental research design relies on statistical analysis to prove or disprove a researcher’s hypothesis. It is one of the most accurate forms of research because it provides specific scientific evidence. Furthermore, out of all the types of experimental designs, only a true experimental design can establish a cause-effect relationship within a group. However, in a true experiment, a researcher must satisfy these three factors —
- There is a control group that is not subjected to changes and an experimental group that will experience the changed variables
- A variable that can be manipulated by the researcher
- Random distribution of the variables
This type of experimental research is commonly observed in the physical sciences.
3. Quasi-experimental Research Design
The word “Quasi” means similarity. A quasi-experimental design is similar to a true experimental design. However, the difference between the two is the assignment of the control group. In this research design, an independent variable is manipulated, but the participants of a group are not randomly assigned. This type of research design is used in field settings where random assignment is either irrelevant or not required.
The classification of the research subjects, conditions, or groups determines the type of research design to be used.
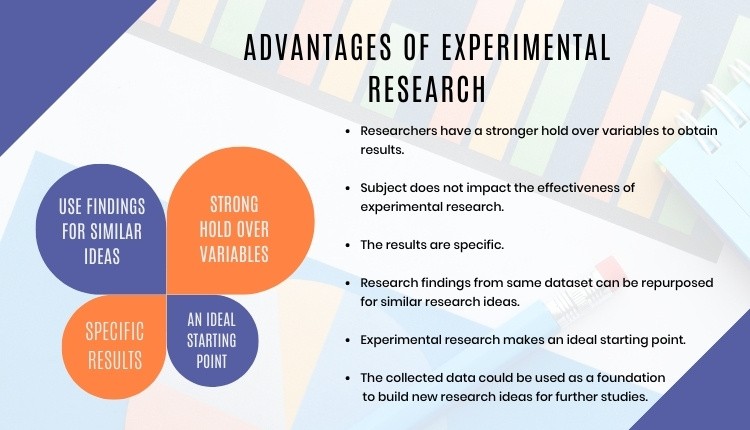
Advantages of Experimental Research
Experimental research allows you to test your idea in a controlled environment before taking the research to clinical trials. Moreover, it provides the best method to test your theory because of the following advantages:
- Researchers have firm control over variables to obtain results.
- The subject does not impact the effectiveness of experimental research. Anyone can implement it for research purposes.
- The results are specific.
- Post results analysis, research findings from the same dataset can be repurposed for similar research ideas.
- Researchers can identify the cause and effect of the hypothesis and further analyze this relationship to determine in-depth ideas.
- Experimental research makes an ideal starting point. The collected data could be used as a foundation to build new research ideas for further studies.
6 Mistakes to Avoid While Designing Your Research
There is no order to this list, and any one of these issues can seriously compromise the quality of your research. You could refer to the list as a checklist of what to avoid while designing your research.
1. Invalid Theoretical Framework
Usually, researchers miss out on checking if their hypothesis is logical to be tested. If your research design does not have basic assumptions or postulates, then it is fundamentally flawed and you need to rework on your research framework.
2. Inadequate Literature Study
Without a comprehensive research literature review , it is difficult to identify and fill the knowledge and information gaps. Furthermore, you need to clearly state how your research will contribute to the research field, either by adding value to the pertinent literature or challenging previous findings and assumptions.
3. Insufficient or Incorrect Statistical Analysis
Statistical results are one of the most trusted scientific evidence. The ultimate goal of a research experiment is to gain valid and sustainable evidence. Therefore, incorrect statistical analysis could affect the quality of any quantitative research.
4. Undefined Research Problem
This is one of the most basic aspects of research design. The research problem statement must be clear and to do that, you must set the framework for the development of research questions that address the core problems.
5. Research Limitations
Every study has some type of limitations . You should anticipate and incorporate those limitations into your conclusion, as well as the basic research design. Include a statement in your manuscript about any perceived limitations, and how you considered them while designing your experiment and drawing the conclusion.
6. Ethical Implications
The most important yet less talked about topic is the ethical issue. Your research design must include ways to minimize any risk for your participants and also address the research problem or question at hand. If you cannot manage the ethical norms along with your research study, your research objectives and validity could be questioned.
Experimental Research Design Example
In an experimental design, a researcher gathers plant samples and then randomly assigns half the samples to photosynthesize in sunlight and the other half to be kept in a dark box without sunlight, while controlling all the other variables (nutrients, water, soil, etc.)
By comparing their outcomes in biochemical tests, the researcher can confirm that the changes in the plants were due to the sunlight and not the other variables.
Experimental research is often the final form of a study conducted in the research process which is considered to provide conclusive and specific results. But it is not meant for every research. It involves a lot of resources, time, and money and is not easy to conduct, unless a foundation of research is built. Yet it is widely used in research institutes and commercial industries, for its most conclusive results in the scientific approach.
Have you worked on research designs? How was your experience creating an experimental design? What difficulties did you face? Do write to us or comment below and share your insights on experimental research designs!
Frequently Asked Questions
Randomization is important in an experimental research because it ensures unbiased results of the experiment. It also measures the cause-effect relationship on a particular group of interest.
Experimental research design lay the foundation of a research and structures the research to establish quality decision making process.
There are 3 types of experimental research designs. These are pre-experimental research design, true experimental research design, and quasi experimental research design.
The difference between an experimental and a quasi-experimental design are: 1. The assignment of the control group in quasi experimental research is non-random, unlike true experimental design, which is randomly assigned. 2. Experimental research group always has a control group; on the other hand, it may not be always present in quasi experimental research.
Experimental research establishes a cause-effect relationship by testing a theory or hypothesis using experimental groups or control variables. In contrast, descriptive research describes a study or a topic by defining the variables under it and answering the questions related to the same.

good and valuable
Very very good
Good presentation.
Rate this article Cancel Reply
Your email address will not be published.
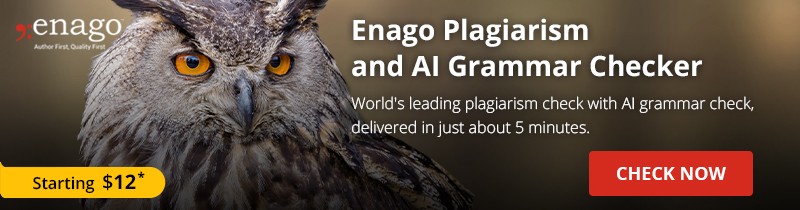
Enago Academy's Most Popular Articles
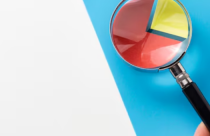
- Promoting Research
Graphical Abstracts Vs. Infographics: Best practices for using visual illustrations for increased research impact
Dr. Sarah Chen stared at her computer screen, her eyes staring at her recently published…
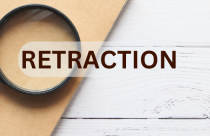
- Publishing Research
10 Tips to Prevent Research Papers From Being Retracted
Research paper retractions represent a critical event in the scientific community. When a published article…
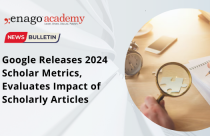
- Industry News
Google Releases 2024 Scholar Metrics, Evaluates Impact of Scholarly Articles
Google has released its 2024 Scholar Metrics, assessing scholarly articles from 2019 to 2023. This…
![experimental developmental research design What is Academic Integrity and How to Uphold it [FREE CHECKLIST]](https://www.enago.com/academy/wp-content/uploads/2024/05/FeatureImages-59-210x136.png)
Ensuring Academic Integrity and Transparency in Academic Research: A comprehensive checklist for researchers
Academic integrity is the foundation upon which the credibility and value of scientific findings are…
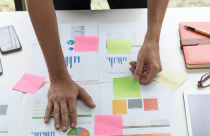
- Reporting Research
How to Optimize Your Research Process: A step-by-step guide
For researchers across disciplines, the path to uncovering novel findings and insights is often filled…
Choosing the Right Analytical Approach: Thematic analysis vs. content analysis for…
Comparing Cross Sectional and Longitudinal Studies: 5 steps for choosing the right…

Sign-up to read more
Subscribe for free to get unrestricted access to all our resources on research writing and academic publishing including:
- 2000+ blog articles
- 50+ Webinars
- 10+ Expert podcasts
- 50+ Infographics
- 10+ Checklists
- Research Guides
We hate spam too. We promise to protect your privacy and never spam you.
- AI in Academia
- Career Corner
- Diversity and Inclusion
- Infographics
- Expert Video Library
- Other Resources
- Enago Learn
- Upcoming & On-Demand Webinars
- Peer Review Week 2024
- Open Access Week 2023
- Conference Videos
- Enago Report
- Journal Finder
- Enago Plagiarism & AI Grammar Check
- Editing Services
- Publication Support Services
- Research Impact
- Translation Services
- Publication solutions
- AI-Based Solutions
- Thought Leadership
- Call for Articles
- Call for Speakers
- Author Training
- Edit Profile
I am looking for Editing/ Proofreading services for my manuscript Tentative date of next journal submission:
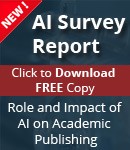
Which among these features would you prefer the most in a peer review assistant?
- Bipolar Disorder
- Therapy Center
- When To See a Therapist
- Types of Therapy
- Best Online Therapy
- Best Couples Therapy
- Managing Stress
- Sleep and Dreaming
- Understanding Emotions
- Self-Improvement
- Healthy Relationships
- Student Resources
- Personality Types
- Guided Meditations
- Verywell Mind Insights
- 2024 Verywell Mind 25
- Mental Health in the Classroom
- Editorial Process
- Meet Our Review Board
- Crisis Support
Developmental Psychology Research Methods
Kendra Cherry, MS, is a psychosocial rehabilitation specialist, psychology educator, and author of the "Everything Psychology Book."
:max_bytes(150000):strip_icc():format(webp)/IMG_9791-89504ab694d54b66bbd72cb84ffb860e.jpg)
Emily is a board-certified science editor who has worked with top digital publishing brands like Voices for Biodiversity, Study.com, GoodTherapy, Vox, and Verywell.
:max_bytes(150000):strip_icc():format(webp)/Emily-Swaim-1000-0f3197de18f74329aeffb690a177160c.jpg)
Jose Luis Pelaez Inc/Getty Images
Cross-Sectional Research Methods
Longitudinal research methods, correlational research methods, experimental research methods.
There are many different developmental psychology research methods, including cross-sectional, longitudinal, correlational, and experimental. Each has its own specific advantages and disadvantages. The one that a scientist chooses depends largely on the aim of the study and the nature of the phenomenon being studied.
Research design provides a standardized framework to test a hypothesis and evaluate whether the hypothesis is correct, incorrect, or inconclusive. Even if the hypothesis is untrue, the research can often provide insights that may prove valuable or move research in an entirely new direction.
At a Glance
In order to study developmental psychology, researchers utilize a number of different research methods. Some involve looking at different cross-sections of a population, while others look at how participants change over time. In other cases, researchers look at how whether certain variables appear to have a relationship with one another. In order to determine if there is a cause-and-effect relationship, however, psychologists much conduct experimental research.
Learn more about each of these different types of developmental psychology research methods, including when they are used and what they can reveal about human development.
Cross-sectional research involves looking at different groups of people with specific characteristics.
For example, a researcher might evaluate a group of young adults and compare the corresponding data from a group of older adults.
The benefit of this type of research is that it can be done relatively quickly; the research data is gathered at the same point in time. The disadvantage is that the research aims to make a direct association between a cause and an effect. This is not always so easy. In some cases, there may be confounding factors that contribute to the effect.
To this end, a cross-sectional study can suggest the odds of an effect occurring both in terms of the absolute risk (the odds of something happening over a period of time) and the relative risk (the odds of something happening in one group compared to another).
Longitudinal research involves studying the same group of individuals over an extended period of time.
Data is collected at the outset of the study and gathered repeatedly through the course of study. In some cases, longitudinal studies can last for several decades or be open-ended. One such example is the Terman Study of the Gifted , which began in the 1920s and followed 1528 children for over 80 years.
The benefit of this longitudinal research is that it allows researchers to look at changes over time. By contrast, one of the obvious disadvantages is cost. Because of the expense of a long-term study, they tend to be confined to a smaller group of subjects or a narrower field of observation.
Challenges of Longitudinal Research
While revealing, longitudinal studies present a few challenges that make them more difficult to use when studying developmental psychology and other topics.
- Longitudinal studies are difficult to apply to a larger population.
- Another problem is that the participants can often drop out mid-study, shrinking the sample size and relative conclusions.
- Moreover, if certain outside forces change during the course of the study (including economics, politics, and science), they can influence the outcomes in a way that significantly skews the results.
For example, in Lewis Terman's longitudinal study, the correlation between IQ and achievement was blunted by such confounding forces as the Great Depression and World War II (which limited educational attainment) and gender politics of the 1940s and 1950s (which limited a woman's professional prospects).
Correlational research aims to determine if one variable has a measurable association with another.
In this type of non-experimental study, researchers look at relationships between the two variables but do not introduce the variables themselves. Instead, they gather and evaluate the available data and offer a statistical conclusion.
For example, the researchers may look at whether academic success in elementary school leads to better-paying jobs in the future. While the researchers can collect and evaluate the data, they do not manipulate any of the variables in question.
A correlational study can be appropriate and helpful if you cannot manipulate a variable because it is impossible, impractical, or unethical.
For example, imagine that a researcher wants to determine if living in a noisy environment makes people less efficient in the workplace. It would be impractical and unreasonable to artificially inflate the noise level in a working environment. Instead, researchers might collect data and then look for correlations between the variables of interest.
Limitations of Correlational Research
Correlational research has its limitations. While it can identify an association, it does not necessarily suggest a cause for the effect. Just because two variables have a relationship does not mean that changes in one will affect a change in the other.
Unlike correlational research, experimentation involves both the manipulation and measurement of variables . This model of research is the most scientifically conclusive and commonly used in medicine, chemistry, psychology, biology, and sociology.
Experimental research uses manipulation to understand cause and effect in a sampling of subjects. The sample is comprised of two groups: an experimental group in whom the variable (such as a drug or treatment) is introduced and a control group in whom the variable is not introduced.
Deciding the sample groups can be done in a number of ways:
- Population sampling, in which the subjects represent a specific population
- Random selection , in which subjects are chosen randomly to see if the effects of the variable are consistently achieved
Challenges in Experimental Resarch
While the statistical value of an experimental study is robust, it may be affected by confirmation bias . This is when the investigator's desire to publish or achieve an unambiguous result can skew the interpretations, leading to a false-positive conclusion.
One way to avoid this is to conduct a double-blind study in which neither the participants nor researchers are aware of which group is the control. A double-blind randomized controlled trial (RCT) is considered the gold standard of research.
What This Means For You
There are many different types of research methods that scientists use to study developmental psychology and other areas. Knowing more about how each of these methods works can give you a better understanding of what the findings of psychological research might mean for you.
Capili B. Cross-sectional studies . Am J Nurs . 2021;121(10):59-62. doi:10.1097/01.NAJ.0000794280.73744.fe
Kesmodel US. Cross-sectional studies - what are they good for? . Acta Obstet Gynecol Scand . 2018;97(4):388–393. doi:10.1111/aogs.13331
Noordzij M, van Diepen M, Caskey FC, Jager KJ. Relative risk versus absolute risk: One cannot be interpreted without the other . Nephrology Dialysis Transplantation. 2017;32(S2):ii13-ii18. doi:10.1093/ndt/gfw465
Kell HJ, Wai J. Terman Study of the Gifted . In: Frey B, ed. The SAGE Encyclopedia of Educational Research, Measurement, and Evaluation . Vol. 4. Thousand Oaks, CA: SAGE Publications, Inc.; 2018. doi:10.4135/9781506326139.n691
Curtis EA, Comiskey C, Dempsey O. Importance and use of correlational research . Nurse Res . 2016;23(6):20–25. doi:10.7748/nr.2016.e1382
Misra S. Randomized double blind placebo control studies, the "Gold Standard" in intervention based studies . Indian J Sex Transm Dis AIDS . 2012;33(2):131-4. doi:10.4103/2589-0557.102130
By Kendra Cherry, MSEd Kendra Cherry, MS, is a psychosocial rehabilitation specialist, psychology educator, and author of the "Everything Psychology Book."

Want to create or adapt books like this? Learn more about how Pressbooks supports open publishing practices.
Chapter 2: Psychological Research
Developmental Research Designs
Sometimes, especially in developmental research, the researcher is interested in examining changes over time and will need to consider a research design that will capture these changes. Remember, research methods are tools that are used to collect information, while research design is the strategy or blueprint for deciding how to collect and analyze information. Research design dictates which methods are used and how. There are three types of developmental research designs: cross-sectional, longitudinal, and sequential.
Video 2.12 Developmental Research Design summarizes the benefits of challenges of the three developmental design models.
Cross-Sectional Designs
The majority of developmental studies use cross-sectional designs because they are less time-consuming and less expensive than other developmental designs. Cross-sectional research designs are used to examine behavior in participants of different ages who are tested at the same point in time. Let’s suppose that researchers are interested in the relationship between intelligence and aging. They might have a hypothesis that intelligence declines as people get older. The researchers might choose to give a particular intelligence test to individuals who are 20 years old, individuals who are 50 years old, and individuals who are 80 years old at the same time and compare the data from each age group. This research is cross-sectional in design because the researchers plan to examine the intelligence scores of individuals of different ages within the same study at the same time; they are taking a “cross-section” of people at one point in time. Let’s say that the comparisons find that the 80-year-old adults score lower on the intelligence test than the 50-year-old adults, and the 50-year-old adults score lower on the intelligence test than the 20-year-old adults. Based on these data, the researchers might conclude that individuals become less intelligent as they get older. Would that be a valid (accurate) interpretation of the results?
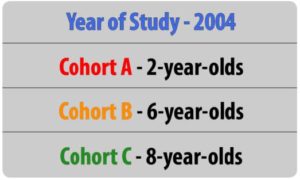
Figure 2.13 Example of cross-sectional research design
No, that would not be a valid conclusion because the researchers did not follow individuals as they aged from 20 to 50 to 80 years old. One of the primary limitations of cross-sectional research is that the results yield information about age differences not necessarily changes over time. That is, although the study described above can show that the 80-year-olds scored lower on the intelligence test than the 50-year-olds, and the 50-year-olds scored lower than the 20-year-olds, the data used for this conclusion were collected from different individuals (or groups). It could be, for instance, that when these 20-year-olds get older, they will still score just as high on the intelligence test as they did at age 20. Similarly, maybe the 80-year-olds would have scored relatively low on the intelligence test when they were young; the researchers don’t know for certain because they did not follow the same individuals as they got older.
With each cohort being members of a different generation, it is also possible that the differences found between the groups are not due to age, per se, but due to cohort effects . Differences between these cohorts’ IQ results could be due to differences in life experiences specific to their generation, such as differences in education, economic conditions, advances in technology, or changes in health and nutrition standards, and not due to age-related changes.
Another disadvantage of cross-sectional research is that it is limited to one time of measurement. Data are collected at one point in time, and it’s possible that something could have happened in that year in history that affected all of the participants, although possibly each cohort may have been affected differently.
Longitudinal Research Designs
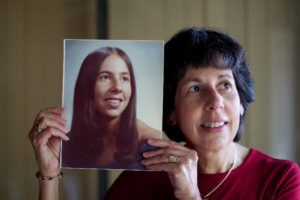
Longitudinal research designs are used to examine behavior in the same individuals over time. For instance, with our example of studying intelligence and aging, a researcher might conduct a longitudinal study to examine whether 20-year-olds become less intelligent with age over time. To this end, a researcher might give an intelligence test to individuals when they are 20 years old, again when they are 50 years old, and then again when they are 80 years old. This study is longitudinal in nature because the researcher plans to study the same individuals as they age. Based on these data, the pattern of intelligence and age might look different than from the cross-sectional research; it might be found that participants’ intelligence scores are higher at age 50 than at age 20 and then remain stable or decline a little by age 80. How can that be when cross-sectional research revealed declines in intelligence with age?

Figure 2.14 Example of a longitudinal research design
Because longitudinal research happens over a period of time (which could be short-term, as in months, but also longer, as in years), there is a risk of attrition. Attrition occurs when participants fail to complete all portions of a study. Participants may move, change their phone numbers, die, or simply become disinterested in participating over time. Researchers should account for the possibility of attrition by enrolling a larger sample into their study initially, as some participants will likely drop out over time. There is also something known as selective attrition — this means that certain groups of individuals may tend to drop out. It is often the least healthy, least educated, and lower socioeconomic participants who tend to drop out over time. That means that the remaining participants may no longer be representative of the whole population, as they are, in general, healthier, better educated, and have more money. This could be a factor in why our hypothetical research found a more optimistic picture of intelligence and aging as the years went by. What can researchers do about selective attrition? At each time of testing, they could randomly recruit more participants from the same cohort as the original members to replace those who have dropped out.
The results from longitudinal studies may also be impacted by repeated assessments. Consider how well you would do on a math test if you were given the exact same exam every day for a week. Your performance would likely improve over time, not necessarily because you developed better math abilities, but because you were continuously practicing the same math problems. This phenomenon is known as a practice effect . Practice effects occur when participants become better at a task over time because they have done it again and again (not due to natural psychological development). So, our participants may have become familiar with the intelligence test each time (and with the computerized testing administration).
Another limitation of longitudinal research is that the data are limited to only one cohort. As an example, think about how comfortable the participants in the 2010 cohort of 20-year-olds are with computers. Because only one cohort is being studied, there is no way to know if findings would be different from other cohorts. In addition, changes that are found as individuals age over time could be due to age or to time of measurement effects. That is, the participants are tested at different periods in history, so the variables of age and time of measurement could be confounded (mixed up). For example, what if there is a major shift in workplace training and education between 2020 and 2040, and many of the participants experience a lot more formal education in adulthood, which positively impacts their intelligence scores in 2040? Researchers wouldn’t know if the intelligence scores increased due to growing older or due to a more educated workforce over time between measurements.
Sequential Research Designs
Sequential research designs include elements of both longitudinal and cross-sectional research designs. Similar to longitudinal designs, sequential research features participants who are followed over time; similar to cross-sectional designs, sequential research includes participants of different ages. This research design is also distinct from those that have been discussed previously in that individuals of different ages are enrolled into a study at various points in time to examine age-related changes, development within the same individuals as they age, and to account for the possibility of cohort and/or time of measurement effects
Consider, once again, our example of intelligence and aging. In a study with a sequential design, a researcher might recruit three separate groups of participants (Groups A, B, and C). Group A would be recruited when they are 20 years old in 2010 and would be tested again when they are 50 and 80 years old in 2040 and 2070, respectively (similar in design to the longitudinal study described previously). Group B would be recruited when they are 20 years old in 2040 and would be tested again when they are 50 years old in 2070. Group C would be recruited when they are 20 years old in 2070, and so on.
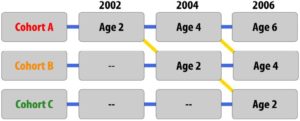
Figure 2.15 Example of sequential research design
Studies with sequential designs are powerful because they allow for both longitudinal and cross-sectional comparisons—changes and/or stability with age over time can be measured and compared with differences between age and cohort groups. This research design also allows for the examination of cohort and time of measurement effects. For example, the researcher could examine the intelligence scores of 20-year-olds at different times in history and different cohorts (follow the yellow diagonal lines in figure 2.15). This might be examined by researchers who are interested in sociocultural and historical changes (because we know that lifespan development is multidisciplinary). One way of looking at the usefulness of the various developmental research designs was described by Schaie and Baltes (1975): cross-sectional and longitudinal designs might reveal change patterns while sequential designs might identify developmental origins for the observed change patterns.
Because they include elements of longitudinal and cross-sectional designs, sequential research has many of the same strengths and limitations as these other approaches. For example, sequential work may require less time and effort than longitudinal research (if data are collected more frequently than over the 30-year spans in our example) but more time and effort than cross-sectional research. Although practice effects may be an issue if participants are asked to complete the same tasks or assessments over time, attrition may be less problematic than what is commonly experienced in longitudinal research because participants may not have to remain involved in the study for such a long period of time.
Comparing Developmental Research Designs
When considering the best research design to use in their research, scientists think about their main research question and the best way to come up with an answer. A table of advantages and disadvantages for each of the described research designs is provided here to help you as you consider what sorts of studies would be best conducted using each of these different approaches.
Table 2.2 Advantages and disadvantages of different research designs
Cross-Sectional | ||
Longitudinal | ||
Sequential |
used to examine behavior in participants of different ages who are tested at the same point in time; may confound age and cohort differences
experiences specific to their generation, such as differences in education, economic conditions, advances in technology, or changes in health and nutrition standards, and not due to age-related changes
studying a group of people who may be of the same age and background (cohort), and measuring them repeatedly over a long period of time; may confound age and time of measurement effects
loss of participants over time
loss of certain groups of individuals over time
participants becoming better at a task over time because they have done it again and again
combines aspects of cross-sectional and longitudinal designs, but also adding new cohorts at different times of measurement; allows for analyses to consider effects of age, cohort, time of measurement, and socio-historical change
Child and Adolescent Development Copyright © 2023 by Krisztina Jakobsen and Paige Fischer is licensed under a Creative Commons Attribution-NonCommercial-ShareAlike 4.0 International License , except where otherwise noted.
Share This Book
Have a language expert improve your writing
Run a free plagiarism check in 10 minutes, generate accurate citations for free.
- Knowledge Base
Methodology
- What Is a Research Design | Types, Guide & Examples
What Is a Research Design | Types, Guide & Examples
Published on June 7, 2021 by Shona McCombes . Revised on September 5, 2024 by Pritha Bhandari.
A research design is a strategy for answering your research question using empirical data. Creating a research design means making decisions about:
- Your overall research objectives and approach
- Whether you’ll rely on primary research or secondary research
- Your sampling methods or criteria for selecting subjects
- Your data collection methods
- The procedures you’ll follow to collect data
- Your data analysis methods
A well-planned research design helps ensure that your methods match your research objectives and that you use the right kind of analysis for your data.
You might have to write up a research design as a standalone assignment, or it might be part of a larger research proposal or other project. In either case, you should carefully consider which methods are most appropriate and feasible for answering your question.
Table of contents
Step 1: consider your aims and approach, step 2: choose a type of research design, step 3: identify your population and sampling method, step 4: choose your data collection methods, step 5: plan your data collection procedures, step 6: decide on your data analysis strategies, other interesting articles, frequently asked questions about research design.
- Introduction
Before you can start designing your research, you should already have a clear idea of the research question you want to investigate.
There are many different ways you could go about answering this question. Your research design choices should be driven by your aims and priorities—start by thinking carefully about what you want to achieve.
The first choice you need to make is whether you’ll take a qualitative or quantitative approach.
Qualitative approach | Quantitative approach |
---|---|
and describe frequencies, averages, and correlations about relationships between variables |
Qualitative research designs tend to be more flexible and inductive , allowing you to adjust your approach based on what you find throughout the research process.
Quantitative research designs tend to be more fixed and deductive , with variables and hypotheses clearly defined in advance of data collection.
It’s also possible to use a mixed-methods design that integrates aspects of both approaches. By combining qualitative and quantitative insights, you can gain a more complete picture of the problem you’re studying and strengthen the credibility of your conclusions.
Practical and ethical considerations when designing research
As well as scientific considerations, you need to think practically when designing your research. If your research involves people or animals, you also need to consider research ethics .
- How much time do you have to collect data and write up the research?
- Will you be able to gain access to the data you need (e.g., by travelling to a specific location or contacting specific people)?
- Do you have the necessary research skills (e.g., statistical analysis or interview techniques)?
- Will you need ethical approval ?
At each stage of the research design process, make sure that your choices are practically feasible.
Prevent plagiarism. Run a free check.
Within both qualitative and quantitative approaches, there are several types of research design to choose from. Each type provides a framework for the overall shape of your research.
Types of quantitative research designs
Quantitative designs can be split into four main types.
- Experimental and quasi-experimental designs allow you to test cause-and-effect relationships
- Descriptive and correlational designs allow you to measure variables and describe relationships between them.
Type of design | Purpose and characteristics |
---|---|
Experimental | relationships effect on a |
Quasi-experimental | ) |
Correlational | |
Descriptive |
With descriptive and correlational designs, you can get a clear picture of characteristics, trends and relationships as they exist in the real world. However, you can’t draw conclusions about cause and effect (because correlation doesn’t imply causation ).
Experiments are the strongest way to test cause-and-effect relationships without the risk of other variables influencing the results. However, their controlled conditions may not always reflect how things work in the real world. They’re often also more difficult and expensive to implement.
Types of qualitative research designs
Qualitative designs are less strictly defined. This approach is about gaining a rich, detailed understanding of a specific context or phenomenon, and you can often be more creative and flexible in designing your research.
The table below shows some common types of qualitative design. They often have similar approaches in terms of data collection, but focus on different aspects when analyzing the data.
Type of design | Purpose and characteristics |
---|---|
Grounded theory | |
Phenomenology |
Your research design should clearly define who or what your research will focus on, and how you’ll go about choosing your participants or subjects.
In research, a population is the entire group that you want to draw conclusions about, while a sample is the smaller group of individuals you’ll actually collect data from.
Defining the population
A population can be made up of anything you want to study—plants, animals, organizations, texts, countries, etc. In the social sciences, it most often refers to a group of people.
For example, will you focus on people from a specific demographic, region or background? Are you interested in people with a certain job or medical condition, or users of a particular product?
The more precisely you define your population, the easier it will be to gather a representative sample.
- Sampling methods
Even with a narrowly defined population, it’s rarely possible to collect data from every individual. Instead, you’ll collect data from a sample.
To select a sample, there are two main approaches: probability sampling and non-probability sampling . The sampling method you use affects how confidently you can generalize your results to the population as a whole.
Probability sampling | Non-probability sampling |
---|---|
Probability sampling is the most statistically valid option, but it’s often difficult to achieve unless you’re dealing with a very small and accessible population.
For practical reasons, many studies use non-probability sampling, but it’s important to be aware of the limitations and carefully consider potential biases. You should always make an effort to gather a sample that’s as representative as possible of the population.
Case selection in qualitative research
In some types of qualitative designs, sampling may not be relevant.
For example, in an ethnography or a case study , your aim is to deeply understand a specific context, not to generalize to a population. Instead of sampling, you may simply aim to collect as much data as possible about the context you are studying.
In these types of design, you still have to carefully consider your choice of case or community. You should have a clear rationale for why this particular case is suitable for answering your research question .
For example, you might choose a case study that reveals an unusual or neglected aspect of your research problem, or you might choose several very similar or very different cases in order to compare them.
Data collection methods are ways of directly measuring variables and gathering information. They allow you to gain first-hand knowledge and original insights into your research problem.
You can choose just one data collection method, or use several methods in the same study.
Survey methods
Surveys allow you to collect data about opinions, behaviors, experiences, and characteristics by asking people directly. There are two main survey methods to choose from: questionnaires and interviews .
Questionnaires | Interviews |
---|---|
) |
Observation methods
Observational studies allow you to collect data unobtrusively, observing characteristics, behaviors or social interactions without relying on self-reporting.
Observations may be conducted in real time, taking notes as you observe, or you might make audiovisual recordings for later analysis. They can be qualitative or quantitative.
Quantitative observation | |
---|---|
Other methods of data collection
There are many other ways you might collect data depending on your field and topic.
Field | Examples of data collection methods |
---|---|
Media & communication | Collecting a sample of texts (e.g., speeches, articles, or social media posts) for data on cultural norms and narratives |
Psychology | Using technologies like neuroimaging, eye-tracking, or computer-based tasks to collect data on things like attention, emotional response, or reaction time |
Education | Using tests or assignments to collect data on knowledge and skills |
Physical sciences | Using scientific instruments to collect data on things like weight, blood pressure, or chemical composition |
If you’re not sure which methods will work best for your research design, try reading some papers in your field to see what kinds of data collection methods they used.
Secondary data
If you don’t have the time or resources to collect data from the population you’re interested in, you can also choose to use secondary data that other researchers already collected—for example, datasets from government surveys or previous studies on your topic.
With this raw data, you can do your own analysis to answer new research questions that weren’t addressed by the original study.
Using secondary data can expand the scope of your research, as you may be able to access much larger and more varied samples than you could collect yourself.
However, it also means you don’t have any control over which variables to measure or how to measure them, so the conclusions you can draw may be limited.
As well as deciding on your methods, you need to plan exactly how you’ll use these methods to collect data that’s consistent, accurate, and unbiased.
Planning systematic procedures is especially important in quantitative research, where you need to precisely define your variables and ensure your measurements are high in reliability and validity.
Operationalization
Some variables, like height or age, are easily measured. But often you’ll be dealing with more abstract concepts, like satisfaction, anxiety, or competence. Operationalization means turning these fuzzy ideas into measurable indicators.
If you’re using observations , which events or actions will you count?
If you’re using surveys , which questions will you ask and what range of responses will be offered?
You may also choose to use or adapt existing materials designed to measure the concept you’re interested in—for example, questionnaires or inventories whose reliability and validity has already been established.
Reliability and validity
Reliability means your results can be consistently reproduced, while validity means that you’re actually measuring the concept you’re interested in.
Reliability | Validity |
---|---|
) ) |
For valid and reliable results, your measurement materials should be thoroughly researched and carefully designed. Plan your procedures to make sure you carry out the same steps in the same way for each participant.
If you’re developing a new questionnaire or other instrument to measure a specific concept, running a pilot study allows you to check its validity and reliability in advance.
Sampling procedures
As well as choosing an appropriate sampling method , you need a concrete plan for how you’ll actually contact and recruit your selected sample.
That means making decisions about things like:
- How many participants do you need for an adequate sample size?
- What inclusion and exclusion criteria will you use to identify eligible participants?
- How will you contact your sample—by mail, online, by phone, or in person?
If you’re using a probability sampling method , it’s important that everyone who is randomly selected actually participates in the study. How will you ensure a high response rate?
If you’re using a non-probability method , how will you avoid research bias and ensure a representative sample?
Data management
It’s also important to create a data management plan for organizing and storing your data.
Will you need to transcribe interviews or perform data entry for observations? You should anonymize and safeguard any sensitive data, and make sure it’s backed up regularly.
Keeping your data well-organized will save time when it comes to analyzing it. It can also help other researchers validate and add to your findings (high replicability ).
On its own, raw data can’t answer your research question. The last step of designing your research is planning how you’ll analyze the data.
Quantitative data analysis
In quantitative research, you’ll most likely use some form of statistical analysis . With statistics, you can summarize your sample data, make estimates, and test hypotheses.
Using descriptive statistics , you can summarize your sample data in terms of:
- The distribution of the data (e.g., the frequency of each score on a test)
- The central tendency of the data (e.g., the mean to describe the average score)
- The variability of the data (e.g., the standard deviation to describe how spread out the scores are)
The specific calculations you can do depend on the level of measurement of your variables.
Using inferential statistics , you can:
- Make estimates about the population based on your sample data.
- Test hypotheses about a relationship between variables.
Regression and correlation tests look for associations between two or more variables, while comparison tests (such as t tests and ANOVAs ) look for differences in the outcomes of different groups.
Your choice of statistical test depends on various aspects of your research design, including the types of variables you’re dealing with and the distribution of your data.
Qualitative data analysis
In qualitative research, your data will usually be very dense with information and ideas. Instead of summing it up in numbers, you’ll need to comb through the data in detail, interpret its meanings, identify patterns, and extract the parts that are most relevant to your research question.
Two of the most common approaches to doing this are thematic analysis and discourse analysis .
Approach | Characteristics |
---|---|
Thematic analysis | |
Discourse analysis |
There are many other ways of analyzing qualitative data depending on the aims of your research. To get a sense of potential approaches, try reading some qualitative research papers in your field.
If you want to know more about the research process , methodology , research bias , or statistics , make sure to check out some of our other articles with explanations and examples.
- Simple random sampling
- Stratified sampling
- Cluster sampling
- Likert scales
- Reproducibility
Statistics
- Null hypothesis
- Statistical power
- Probability distribution
- Effect size
- Poisson distribution
Research bias
- Optimism bias
- Cognitive bias
- Implicit bias
- Hawthorne effect
- Anchoring bias
- Explicit bias
A research design is a strategy for answering your research question . It defines your overall approach and determines how you will collect and analyze data.
A well-planned research design helps ensure that your methods match your research aims, that you collect high-quality data, and that you use the right kind of analysis to answer your questions, utilizing credible sources . This allows you to draw valid , trustworthy conclusions.
Quantitative research designs can be divided into two main categories:
- Correlational and descriptive designs are used to investigate characteristics, averages, trends, and associations between variables.
- Experimental and quasi-experimental designs are used to test causal relationships .
Qualitative research designs tend to be more flexible. Common types of qualitative design include case study , ethnography , and grounded theory designs.
The priorities of a research design can vary depending on the field, but you usually have to specify:
- Your research questions and/or hypotheses
- Your overall approach (e.g., qualitative or quantitative )
- The type of design you’re using (e.g., a survey , experiment , or case study )
- Your data collection methods (e.g., questionnaires , observations)
- Your data collection procedures (e.g., operationalization , timing and data management)
- Your data analysis methods (e.g., statistical tests or thematic analysis )
A sample is a subset of individuals from a larger population . Sampling means selecting the group that you will actually collect data from in your research. For example, if you are researching the opinions of students in your university, you could survey a sample of 100 students.
In statistics, sampling allows you to test a hypothesis about the characteristics of a population.
Operationalization means turning abstract conceptual ideas into measurable observations.
For example, the concept of social anxiety isn’t directly observable, but it can be operationally defined in terms of self-rating scores, behavioral avoidance of crowded places, or physical anxiety symptoms in social situations.
Before collecting data , it’s important to consider how you will operationalize the variables that you want to measure.
A research project is an academic, scientific, or professional undertaking to answer a research question . Research projects can take many forms, such as qualitative or quantitative , descriptive , longitudinal , experimental , or correlational . What kind of research approach you choose will depend on your topic.
Cite this Scribbr article
If you want to cite this source, you can copy and paste the citation or click the “Cite this Scribbr article” button to automatically add the citation to our free Citation Generator.
McCombes, S. (2024, September 05). What Is a Research Design | Types, Guide & Examples. Scribbr. Retrieved September 30, 2024, from https://www.scribbr.com/methodology/research-design/
Is this article helpful?
Shona McCombes
Other students also liked, guide to experimental design | overview, steps, & examples, how to write a research proposal | examples & templates, ethical considerations in research | types & examples, what is your plagiarism score.
- Privacy Policy

Home » Experimental Design – Types, Methods, Guide
Experimental Design – Types, Methods, Guide
Table of Contents

Experimental Design
Experimental design is a process of planning and conducting scientific experiments to investigate a hypothesis or research question. It involves carefully designing an experiment that can test the hypothesis, and controlling for other variables that may influence the results.
Experimental design typically includes identifying the variables that will be manipulated or measured, defining the sample or population to be studied, selecting an appropriate method of sampling, choosing a method for data collection and analysis, and determining the appropriate statistical tests to use.
Types of Experimental Design
Here are the different types of experimental design:
Completely Randomized Design
In this design, participants are randomly assigned to one of two or more groups, and each group is exposed to a different treatment or condition.
Randomized Block Design
This design involves dividing participants into blocks based on a specific characteristic, such as age or gender, and then randomly assigning participants within each block to one of two or more treatment groups.
Factorial Design
In a factorial design, participants are randomly assigned to one of several groups, each of which receives a different combination of two or more independent variables.
Repeated Measures Design
In this design, each participant is exposed to all of the different treatments or conditions, either in a random order or in a predetermined order.
Crossover Design
This design involves randomly assigning participants to one of two or more treatment groups, with each group receiving one treatment during the first phase of the study and then switching to a different treatment during the second phase.
Split-plot Design
In this design, the researcher manipulates one or more variables at different levels and uses a randomized block design to control for other variables.
Nested Design
This design involves grouping participants within larger units, such as schools or households, and then randomly assigning these units to different treatment groups.
Laboratory Experiment
Laboratory experiments are conducted under controlled conditions, which allows for greater precision and accuracy. However, because laboratory conditions are not always representative of real-world conditions, the results of these experiments may not be generalizable to the population at large.
Field Experiment
Field experiments are conducted in naturalistic settings and allow for more realistic observations. However, because field experiments are not as controlled as laboratory experiments, they may be subject to more sources of error.
Experimental Design Methods
Experimental design methods refer to the techniques and procedures used to design and conduct experiments in scientific research. Here are some common experimental design methods:
Randomization
This involves randomly assigning participants to different groups or treatments to ensure that any observed differences between groups are due to the treatment and not to other factors.
Control Group
The use of a control group is an important experimental design method that involves having a group of participants that do not receive the treatment or intervention being studied. The control group is used as a baseline to compare the effects of the treatment group.
Blinding involves keeping participants, researchers, or both unaware of which treatment group participants are in, in order to reduce the risk of bias in the results.
Counterbalancing
This involves systematically varying the order in which participants receive treatments or interventions in order to control for order effects.
Replication
Replication involves conducting the same experiment with different samples or under different conditions to increase the reliability and validity of the results.
This experimental design method involves manipulating multiple independent variables simultaneously to investigate their combined effects on the dependent variable.
This involves dividing participants into subgroups or blocks based on specific characteristics, such as age or gender, in order to reduce the risk of confounding variables.
Data Collection Method
Experimental design data collection methods are techniques and procedures used to collect data in experimental research. Here are some common experimental design data collection methods:
Direct Observation
This method involves observing and recording the behavior or phenomenon of interest in real time. It may involve the use of structured or unstructured observation, and may be conducted in a laboratory or naturalistic setting.
Self-report Measures
Self-report measures involve asking participants to report their thoughts, feelings, or behaviors using questionnaires, surveys, or interviews. These measures may be administered in person or online.
Behavioral Measures
Behavioral measures involve measuring participants’ behavior directly, such as through reaction time tasks or performance tests. These measures may be administered using specialized equipment or software.
Physiological Measures
Physiological measures involve measuring participants’ physiological responses, such as heart rate, blood pressure, or brain activity, using specialized equipment. These measures may be invasive or non-invasive, and may be administered in a laboratory or clinical setting.
Archival Data
Archival data involves using existing records or data, such as medical records, administrative records, or historical documents, as a source of information. These data may be collected from public or private sources.
Computerized Measures
Computerized measures involve using software or computer programs to collect data on participants’ behavior or responses. These measures may include reaction time tasks, cognitive tests, or other types of computer-based assessments.
Video Recording
Video recording involves recording participants’ behavior or interactions using cameras or other recording equipment. This method can be used to capture detailed information about participants’ behavior or to analyze social interactions.
Data Analysis Method
Experimental design data analysis methods refer to the statistical techniques and procedures used to analyze data collected in experimental research. Here are some common experimental design data analysis methods:
Descriptive Statistics
Descriptive statistics are used to summarize and describe the data collected in the study. This includes measures such as mean, median, mode, range, and standard deviation.
Inferential Statistics
Inferential statistics are used to make inferences or generalizations about a larger population based on the data collected in the study. This includes hypothesis testing and estimation.
Analysis of Variance (ANOVA)
ANOVA is a statistical technique used to compare means across two or more groups in order to determine whether there are significant differences between the groups. There are several types of ANOVA, including one-way ANOVA, two-way ANOVA, and repeated measures ANOVA.
Regression Analysis
Regression analysis is used to model the relationship between two or more variables in order to determine the strength and direction of the relationship. There are several types of regression analysis, including linear regression, logistic regression, and multiple regression.
Factor Analysis
Factor analysis is used to identify underlying factors or dimensions in a set of variables. This can be used to reduce the complexity of the data and identify patterns in the data.
Structural Equation Modeling (SEM)
SEM is a statistical technique used to model complex relationships between variables. It can be used to test complex theories and models of causality.
Cluster Analysis
Cluster analysis is used to group similar cases or observations together based on similarities or differences in their characteristics.
Time Series Analysis
Time series analysis is used to analyze data collected over time in order to identify trends, patterns, or changes in the data.
Multilevel Modeling
Multilevel modeling is used to analyze data that is nested within multiple levels, such as students nested within schools or employees nested within companies.

Applications of Experimental Design
Experimental design is a versatile research methodology that can be applied in many fields. Here are some applications of experimental design:
- Medical Research: Experimental design is commonly used to test new treatments or medications for various medical conditions. This includes clinical trials to evaluate the safety and effectiveness of new drugs or medical devices.
- Agriculture : Experimental design is used to test new crop varieties, fertilizers, and other agricultural practices. This includes randomized field trials to evaluate the effects of different treatments on crop yield, quality, and pest resistance.
- Environmental science: Experimental design is used to study the effects of environmental factors, such as pollution or climate change, on ecosystems and wildlife. This includes controlled experiments to study the effects of pollutants on plant growth or animal behavior.
- Psychology : Experimental design is used to study human behavior and cognitive processes. This includes experiments to test the effects of different interventions, such as therapy or medication, on mental health outcomes.
- Engineering : Experimental design is used to test new materials, designs, and manufacturing processes in engineering applications. This includes laboratory experiments to test the strength and durability of new materials, or field experiments to test the performance of new technologies.
- Education : Experimental design is used to evaluate the effectiveness of teaching methods, educational interventions, and programs. This includes randomized controlled trials to compare different teaching methods or evaluate the impact of educational programs on student outcomes.
- Marketing : Experimental design is used to test the effectiveness of marketing campaigns, pricing strategies, and product designs. This includes experiments to test the impact of different marketing messages or pricing schemes on consumer behavior.
Examples of Experimental Design
Here are some examples of experimental design in different fields:
- Example in Medical research : A study that investigates the effectiveness of a new drug treatment for a particular condition. Patients are randomly assigned to either a treatment group or a control group, with the treatment group receiving the new drug and the control group receiving a placebo. The outcomes, such as improvement in symptoms or side effects, are measured and compared between the two groups.
- Example in Education research: A study that examines the impact of a new teaching method on student learning outcomes. Students are randomly assigned to either a group that receives the new teaching method or a group that receives the traditional teaching method. Student achievement is measured before and after the intervention, and the results are compared between the two groups.
- Example in Environmental science: A study that tests the effectiveness of a new method for reducing pollution in a river. Two sections of the river are selected, with one section treated with the new method and the other section left untreated. The water quality is measured before and after the intervention, and the results are compared between the two sections.
- Example in Marketing research: A study that investigates the impact of a new advertising campaign on consumer behavior. Participants are randomly assigned to either a group that is exposed to the new campaign or a group that is not. Their behavior, such as purchasing or product awareness, is measured and compared between the two groups.
- Example in Social psychology: A study that examines the effect of a new social intervention on reducing prejudice towards a marginalized group. Participants are randomly assigned to either a group that receives the intervention or a control group that does not. Their attitudes and behavior towards the marginalized group are measured before and after the intervention, and the results are compared between the two groups.
When to use Experimental Research Design
Experimental research design should be used when a researcher wants to establish a cause-and-effect relationship between variables. It is particularly useful when studying the impact of an intervention or treatment on a particular outcome.
Here are some situations where experimental research design may be appropriate:
- When studying the effects of a new drug or medical treatment: Experimental research design is commonly used in medical research to test the effectiveness and safety of new drugs or medical treatments. By randomly assigning patients to treatment and control groups, researchers can determine whether the treatment is effective in improving health outcomes.
- When evaluating the effectiveness of an educational intervention: An experimental research design can be used to evaluate the impact of a new teaching method or educational program on student learning outcomes. By randomly assigning students to treatment and control groups, researchers can determine whether the intervention is effective in improving academic performance.
- When testing the effectiveness of a marketing campaign: An experimental research design can be used to test the effectiveness of different marketing messages or strategies. By randomly assigning participants to treatment and control groups, researchers can determine whether the marketing campaign is effective in changing consumer behavior.
- When studying the effects of an environmental intervention: Experimental research design can be used to study the impact of environmental interventions, such as pollution reduction programs or conservation efforts. By randomly assigning locations or areas to treatment and control groups, researchers can determine whether the intervention is effective in improving environmental outcomes.
- When testing the effects of a new technology: An experimental research design can be used to test the effectiveness and safety of new technologies or engineering designs. By randomly assigning participants or locations to treatment and control groups, researchers can determine whether the new technology is effective in achieving its intended purpose.
How to Conduct Experimental Research
Here are the steps to conduct Experimental Research:
- Identify a Research Question : Start by identifying a research question that you want to answer through the experiment. The question should be clear, specific, and testable.
- Develop a Hypothesis: Based on your research question, develop a hypothesis that predicts the relationship between the independent and dependent variables. The hypothesis should be clear and testable.
- Design the Experiment : Determine the type of experimental design you will use, such as a between-subjects design or a within-subjects design. Also, decide on the experimental conditions, such as the number of independent variables, the levels of the independent variable, and the dependent variable to be measured.
- Select Participants: Select the participants who will take part in the experiment. They should be representative of the population you are interested in studying.
- Randomly Assign Participants to Groups: If you are using a between-subjects design, randomly assign participants to groups to control for individual differences.
- Conduct the Experiment : Conduct the experiment by manipulating the independent variable(s) and measuring the dependent variable(s) across the different conditions.
- Analyze the Data: Analyze the data using appropriate statistical methods to determine if there is a significant effect of the independent variable(s) on the dependent variable(s).
- Draw Conclusions: Based on the data analysis, draw conclusions about the relationship between the independent and dependent variables. If the results support the hypothesis, then it is accepted. If the results do not support the hypothesis, then it is rejected.
- Communicate the Results: Finally, communicate the results of the experiment through a research report or presentation. Include the purpose of the study, the methods used, the results obtained, and the conclusions drawn.
Purpose of Experimental Design
The purpose of experimental design is to control and manipulate one or more independent variables to determine their effect on a dependent variable. Experimental design allows researchers to systematically investigate causal relationships between variables, and to establish cause-and-effect relationships between the independent and dependent variables. Through experimental design, researchers can test hypotheses and make inferences about the population from which the sample was drawn.
Experimental design provides a structured approach to designing and conducting experiments, ensuring that the results are reliable and valid. By carefully controlling for extraneous variables that may affect the outcome of the study, experimental design allows researchers to isolate the effect of the independent variable(s) on the dependent variable(s), and to minimize the influence of other factors that may confound the results.
Experimental design also allows researchers to generalize their findings to the larger population from which the sample was drawn. By randomly selecting participants and using statistical techniques to analyze the data, researchers can make inferences about the larger population with a high degree of confidence.
Overall, the purpose of experimental design is to provide a rigorous, systematic, and scientific method for testing hypotheses and establishing cause-and-effect relationships between variables. Experimental design is a powerful tool for advancing scientific knowledge and informing evidence-based practice in various fields, including psychology, biology, medicine, engineering, and social sciences.
Advantages of Experimental Design
Experimental design offers several advantages in research. Here are some of the main advantages:
- Control over extraneous variables: Experimental design allows researchers to control for extraneous variables that may affect the outcome of the study. By manipulating the independent variable and holding all other variables constant, researchers can isolate the effect of the independent variable on the dependent variable.
- Establishing causality: Experimental design allows researchers to establish causality by manipulating the independent variable and observing its effect on the dependent variable. This allows researchers to determine whether changes in the independent variable cause changes in the dependent variable.
- Replication : Experimental design allows researchers to replicate their experiments to ensure that the findings are consistent and reliable. Replication is important for establishing the validity and generalizability of the findings.
- Random assignment: Experimental design often involves randomly assigning participants to conditions. This helps to ensure that individual differences between participants are evenly distributed across conditions, which increases the internal validity of the study.
- Precision : Experimental design allows researchers to measure variables with precision, which can increase the accuracy and reliability of the data.
- Generalizability : If the study is well-designed, experimental design can increase the generalizability of the findings. By controlling for extraneous variables and using random assignment, researchers can increase the likelihood that the findings will apply to other populations and contexts.
Limitations of Experimental Design
Experimental design has some limitations that researchers should be aware of. Here are some of the main limitations:
- Artificiality : Experimental design often involves creating artificial situations that may not reflect real-world situations. This can limit the external validity of the findings, or the extent to which the findings can be generalized to real-world settings.
- Ethical concerns: Some experimental designs may raise ethical concerns, particularly if they involve manipulating variables that could cause harm to participants or if they involve deception.
- Participant bias : Participants in experimental studies may modify their behavior in response to the experiment, which can lead to participant bias.
- Limited generalizability: The conditions of the experiment may not reflect the complexities of real-world situations. As a result, the findings may not be applicable to all populations and contexts.
- Cost and time : Experimental design can be expensive and time-consuming, particularly if the experiment requires specialized equipment or if the sample size is large.
- Researcher bias : Researchers may unintentionally bias the results of the experiment if they have expectations or preferences for certain outcomes.
- Lack of feasibility : Experimental design may not be feasible in some cases, particularly if the research question involves variables that cannot be manipulated or controlled.
About the author
Muhammad Hassan
Researcher, Academic Writer, Web developer
You may also like

One-to-One Interview – Methods and Guide

Focus Groups – Steps, Examples and Guide

Explanatory Research – Types, Methods, Guide

Observational Research – Methods and Guide

Ethnographic Research -Types, Methods and Guide

Triangulation in Research – Types, Methods and...
- Study Protocol
- Open access
- Published: 28 September 2024
Study Protocol for a Cluster, Randomized, Controlled Community Effectiveness Trial of the Early Start Denver Model (ESDM) Compared to Community Early Behavioral Intervention (EBI) in Community Programs serving Young Autistic Children: Partnering for Autism: Learning more to improve Services (PALMS)
- Aubyn C. Stahmer 1 ,
- Sarah Dufek 1 ,
- Sally J. Rogers 1 &
- Ana-Maria Iosif 2
BMC Psychology volume 12 , Article number: 513 ( 2024 ) Cite this article
343 Accesses
Metrics details
The rising number of children identified with autism has led to exponential growth in for-profit applied behavior analysis (ABA) agencies and the use of highly structured approaches that may not be developmentally appropriate for young children. Multiple clinical trials support naturalistic developmental behavior interventions (NDBIs) that integrate ABA and developmental science and are considered best practices for young autistic children. The Early Start Denver Model (ESDM) is a comprehensive NDBI shown to improve social communication outcomes for young autistic children in several controlled efficacy studies. However, effectiveness data regarding NDBI use in community-based agencies are limited.
This study uses a community-partnered approach to test the effectiveness of ESDM compared to usual early behavioral intervention (EBI) for improving social communication and language in autistic children served by community agencies. This is a hybrid type 1 cluster-randomized controlled trial with 2 conditions: ESDM and EBI. In the intervention group, supervising providers will receive training in ESDM; in the control group, they will continue EBI as usual. We will enroll and randomize 100 supervisors (50 ESDM, 50 EBI) by region. Each supervisor enrolls 3 families of autistic children under age 5 (n = 300) and accompanying behavior technicians (n = 200). The primary outcome is child language and social communication at 6 and 12 months. Secondary outcomes include child adaptive behavior, caregiver use of ESDM strategies, and provider intervention fidelity. Child social motivation and caregiver fidelity will be tested as mediating variables. ESDM implementation determinants will be explored using mixed methods.
This study will contribute novel knowledge on ESDM effectiveness, the variables that mediate and moderate child outcomes, and engagement of its mechanisms in community use. We expect results from this trial to increase community availability of this model and access to high-quality intervention for young autistic children, especially those who depend on publicly funded intervention services. Understanding implementation determinants will aid scale-up of effective models within communities.
Trail registration
Clinicaltrials.gov identifier number NCT06005285. Registered on August 21, 2023.
Protocol version
Issue date 6 August 2024; Protocol amendment number: 02.
Peer Review reports
Autism as an ongoing significant public health concern
Autism Spectrum Disorder (autism) continues to be one of the most common forms of neurodevelopmental disability worldwide, with US estimates of 1 in 36 children [ 1 ]. Autism presents a significant public health challenge in that the average per capita lifetime costs of challenges associated with an autism diagnosis in the US exceeds $3 million. The societal costs are over $7 trillion and are projected to rise to $14 trillion by 2029 [ 2 , 3 ]. High-quality, evidence-based early intervention has the potential to improve child outcomes by reducing intellectual impairment and improving social communication and language skills [ 4 , 5 , 6 , 7 ]. In addition, research suggests that the cost of early evidence-based intervention may be offset by reduced costs of special education and other intervention across the lifespan [ 2 , 8 , 9 ]. The most frequently used early intervention for autistic children under age 5 is behavioral therapy [ 10 ]. A recent survey reports approximately 40% of autistic children in the US receive intensive behavior therapy (17–67% depending upon region) [ 11 ]. However, current data come primarily from controlled efficacy studies with strict inclusion criteria, highly trained providers and limited sample diversity [ 12 ].
Because of increasing demand due to rising prevalence, consumer knowledge, and improved insurance coverage, the US has seen a proliferation in the number of for-profit autism community-based agencies (CBAs) offering intervention. Since the Affordable Care Act (2010), 47 states have mandates for insurance funding of autism interventions based on Applied Behavior Analysis (ABA). For a variety of reasons, including initial studies from several decades ago, structured interventions based on ABA are most often used in CBAs [ 13 ]. CBAs are estimated to serve over 50,000 autistic people and generate $1.07 billion in revenues annually. They serve many historically underrepresented autistic children, including those living in low-income communities. However, the fast growth in number of CBAs belies the lack of effectiveness research for their services. This lack of the necessary evidence-base to support current community practice raises serious public health concerns about the cost, effectiveness, and quality of community early autism intervention. This is especially true for children of color [ 14 ].
Need for improvement in community-based early intervention services for autistic children
Systematic reviews and meta-analyses of randomized efficacy trials find positive effects of both highly-structured, ABA-based early interventions, and naturalistic developmental behavioral interventions (NDBIs) on developmental outcomes for young children with autism [ 15 , 16 , 17 ]. Discrete Trial Teaching (DTT), a highly-structured intervention based on ABA principles, was one of the first identified early interventions for autism [ 18 ]. DTT has a clear, structured curriculum and highly scripted teaching strategies. It is adult-directed and uses massed trial-based learning and external motivation to teach skills across domains. DTT is the primary comprehensive strategy taught in a majority of BCBA and technician training programs and therefore represents the primary strategy provided by CBAs [ 19 ]. Most effectiveness studies for the DTT model are either case–control studies or quasi-experimental with a few randomized controlled design studies [ 20 , 21 , 22 , 23 ]. Structured ABA programs often demonstrate better outcomes for children than eclectic models or waitlist controls; however, results are inconsistent [ 24 , 25 ] and effect sizes are consistently smaller than in efficacy trials [ 26 , 27 , 28 ].
In the past 25 years since the initial non-randomized DTT efficacy trial [ 18 ], intervention sciences has evolved to bring developmental science into early intervention via the NDBIs [ 29 ]. NDBIs combine developmental science with ABA principles to include developmentally appropriate learning targets and teaching strategies, including those that integrate child learning into daily activities to build well-generalized child learning. Additionally, these developmentally appropriate practices respect young children’s interests, choices, and initiative, and focus on children’s own motivations to support child-directed learning. The NDBI evidence base includes multiple randomized trials testing NDBIs [ 30 , 31 , 32 , 33 ], which are established best practice for young autistic children [ 34 ], supported by systematic reviews and meta-analyses reporting positive child outcomes [ 7 , 35 , 36 ].
In addition to being effective, NDBIs facilitate inclusion through their use of typical, developmentally appropriate practices [ 37 , 38 ]. Additionally, autistic adults have raised concerns regarding the ethics of traditional ABA approaches that focus on compliance, suppression of characteristically autistic behaviors, and “curing” autism, fearing that such approaches pathologize autism and may cause harm to autistic people [ 39 ]. While views vary widely, many neurodiversity advocates support person-centered, respectful intervention focusing on skill building and improving quality of life [ 40 ]. NDBIs use a strengths-based approach focused on child choices and preferences to support child learning, especially in social communication and language. NDBIs have potential to better align early intervention with the goals of autistic individuals [ 41 ]. However, NDBIs have a paucity of effectiveness trials in CBAs with the few existing studies flawed by lack of experimental designs [ 42 ], a focus on school settings [ 43 , 44 , 45 ], or low-intensity, parent-implemented studies [ 46 , 47 ].
Currently, CBAs rarely implement NDBI models, instead using highly structured DTT strategies not developmentally appropriate for young children, [ 48 , 49 ] due in part to lack of knowledge and quality training. A recent survey of behavior therapists found that few recognize or understand how to use NDBIs in practice [ 19 ]. Given the strengths of NDBI, the large numbers of CBAs serving young autistic children, and their lack of NDBI knowledge, there is a clear need for effectiveness testing with diverse children in community care to determine if NDBIs support child learning and progress and family use and satisfaction.
Need for effectiveness testing of NDBI to meet this need
One NDBI developed for autistic children under age 5 is the Early Start Denver Model (ESDM) [ 29 ]. ESDM is a comprehensive model that aims to increase children’s social motivation and social learning opportunities, decrease their developmental delays, and enhance social communication. ESDM uses a data-based approach and empirically supported ABA and developmental teaching practices embedded in everyday activities. ESDM integrates ABA with developmental, relationship- and play-based practices to create an integrated approach that is individualized while also being standardized and manualized. ESDM fits within the seven dimensions that define ABA practice [ 50 , 51 , 52 ] and meets the criteria of the Professional and Ethical Compliance Code for Behavior Analysts [ 53 ] and additional parameters in the ABA Treatment of ASD Practice Guidelines [ 54 ].
ESDM is one of the very few comprehensive early interventions validated and replicated in multiple, randomized trials [ 30 , 33 , 55 ]. A recent meta-analysis found significant effects of ESDM on cognition and language compared to usual care [ 4 ]. ESDM is effective for autistic children across a wide range of learning styles and abilities and is flexible enough to be used in many contexts by different types of providers and caregivers. While the evidence-base is strong, in these studies ESDM has been delivered by highly trained providers at university-based research sites. Exclusion criteria in some trials eliminated participants based on severe caregiver mental health conditions, geographic location, limited English, and child characteristics (e.g., IQ < 35, genetic comorbidities) [ 56 ].
To date, there have been no effectiveness trials of the comprehensive ESDM protocol in community settings. However, a recent review found that ESDM has more evidence to support its use with participants from culturally and linguistically diverse backgrounds than any other NDBI [ 57 ]. Two feasibility studies suggest community effectiveness when treatment is provided by ESDM-certified staff [ 58 , 59 ]. A recent randomized feasibility trial of an adapted model (Community ESDM; C-ESDM) in low resource settings demonstrated the feasibility of training community providers to coach caregivers in ESDM and found significant skill gains in provider and caregiver use of ESDM strategies. However, perhaps due to very low intensity, there were no significant differences in the amount of child gain between groups [ 46 ]. A recent randomized trial with a broad inclusion criteria that examined the use of C-ESDM in 16 community agencies in Canada involving 49 children found that families receiving C-ESDM reported higher quality of life, intervention satisfaction, and self-efficacy than the comparison group. Children in the C-ESDM group made greater gains in receptive language and faster gains in joint attention and language with greater effect sizes than the comparison group [ 56 ]. These feasibility studies show the promise of ESDM for the community and highlight the need for a full-scale effectiveness trial.
Advancing the science of intervention mechanisms through an ESDM effectiveness trial
Child social motivation and Systematic Caregiver Coaching are the main variables in the hypothesized model of change underlying ESDM [ 60 ]. Social motivation theory has been used to explain an underlying mechanism of social communication challenges in autism [ 61 , 62 ]. Dawson posits that a biological disruption of social motivation results in decreased social attention and social learning beginning in the first year of life, potentially contributing to the developmental delays in learning, social communication, social cognition and social interests observed in autism. Studies have identified early differences in social motivation between autistic and neurotypical individuals in behavioral manifestation, physiology and neurobiology from infancy on [ 63 , 64 , 65 ]. A recent meta-analysis showed that, in over 6000 participants, autistic individuals showed reduced social orienting compared to neurotypical peers [ 66 ]. Limited social orienting has a negative impact on language learning, [ 67 , 68 , 69 , 70 ] and early social motivation in autistic toddlers predicts language 2 years later [ 71 ]. These studies have led researchers to identify social motivation as a potential mediator of response to early intervention and an important mechanism to target in early treatment [ 60 ].
Social motivation has thus been suggested as the underlying mechanism of change in ESDM [ 72 ]. Given the plasticity of brain development in the first few years of life and its proclivity for social communication and language learning in this period, ESDM was developed to differentially support social attention, engagement, communication and social motivation to very young autistic children by pairing developmentally appropriate learning with children’s preferred people, activities, and materials. Caregivers learn to use different strategies that better support learning and social engagement for their autistic children. Thus, the ESDM intervention approach heightens the value of social engagement in a way that fits the child’s learning style and supports child social attention for learning. These additional social learning experiences are thought to stimulate further neural development and connectivity resulting in accelerated learning rates overall and improved growth and development of early social communication. This study represents the largest examination of ESDM or any other NDBI yet conducted and will provide additional data about whether ESDM activates the proposed mechanism – social motivation – to improve social communication and language outcomes in autistic children.
ESDM’s strong emphasis on systematic caregiver coaching facilitates child outcomes by allowing caregivers, with whom children may have the highest social motivation, to embed ESDM strategies throughout daily family routines. Coaching caregivers in strategies that increase social motivation can increase learning opportunities and child engagement beyond the treatment session. Inclusion of caregivers in intervention is best practice [ 73 ], and fidelity to intervention practices predicts child outcomes in programs with both provider- and caregiver-implemented components [ 74 , 75 ]. CBA programs typically include some coaching related to behavior concerns rather than teaching intervention strategies [ 76 ]. Therefore, having a systematic method of teaching caregivers to use ESDM strategies may increase access to intervention further activating the social motivation mechanism and improve child outcomes The importance of caregiver coaching is further supported by the positive relationship between caregiver fidelity and child outcomes across multiple studies [ 74 , 77 ]. Therefore, we aim to understand the mediating role of caregiver NDBI fidelity and child outcomes across both groups in this diverse community sample.
Using implementation science to maximize efficiency and relevance of the ESDM trial
If ESDM is effective for autistic children in the community, and acceptable to community providers and caregivers, the next target should be scaling up for broader implementation. This study will use the Exploration, Adoption/Preparation, Implementation, Sustainability (EPIS) framework [ 78 ], a multi-level, multi-phase, process and determinant framework to collect preliminary implementation data. This framework both describes the process of translating research into practice and allows for identification of factors that influence implementation outcomes. The EPIS framework is relevant for understanding the process and determinants of service implementation in public service systems for young autistic children (see Fig. 1 ). EPIS specifies both the critical role of intervention characteristics and the inner and outer context factors on implementation, while attention to client diversity and potential needs for adaptations across levels. Thus, using an implementation science framework can reduce inequities in healthcare delivery [ 79 ].
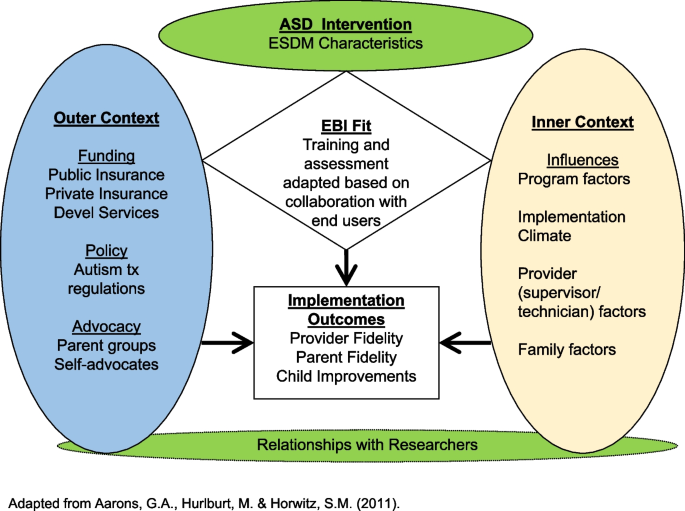
Applying the Exploration, Preparation, Implementation, Sustainment (EPIS) Conceptual Model of Implementation to ASD EBI
The current project uses a hybrid type 1 randomized controlled design to examine ESDM effectiveness and to gather data on implementation determinants. This study will test the effectiveness of ESDM for improving social communication and language (primary), adaptive behavior, goal progress and quality of life (secondary) outcomes in a diverse community sample of autistic children. Our research question include: (1) Compared to usual EBI, do children in the ESDM condition demonstrate significantly increased growth rates in social communication and language? (2) Do caregivers in the ESDM condition have greater increases in use of general NDBI strategies and greater caregiver competence than those in EBI? (3) Does ESDM engage the treatment mechanisms of child social motivation and caregiver fidelity within both treatment groups? (4) Do variable such as caregiver education, child race/ethnicity and provider adherence to ESDM fidelity moderate child progress in both groups? We will use the EPIS framework to gather data on ESDM Implementation outcomes including acceptability, feasibility, appropriateness (including for children), cultural responsivity, CBA provider ESDM fidelity, and caregiver engagement.
Design & Randomization
This study uses a parallel 2-arm, hybrid type 1 (effectiveness/implementation) cluster randomized controlled trial design. The two arms are ESDM and EBI. We will recruit a multilevel sample (Fig. 2 ), including 20 CBAs, 20 regional managers, 100 Regional Teams (program supervisor and technicians: average of 1 supervisor and 2 technicians per team) and 300 child/caregiver dyads (2–4 per team). Regional managers from participating regions will complete baseline and follow-up surveys and semi-structured interviews. We will recruit as many supervisors per region as possible with an expected mean of 5 per region. We will recruit as many technicians per region as possible, with replacement to account for high turnover, with an expected mean of 2 per supervisor.
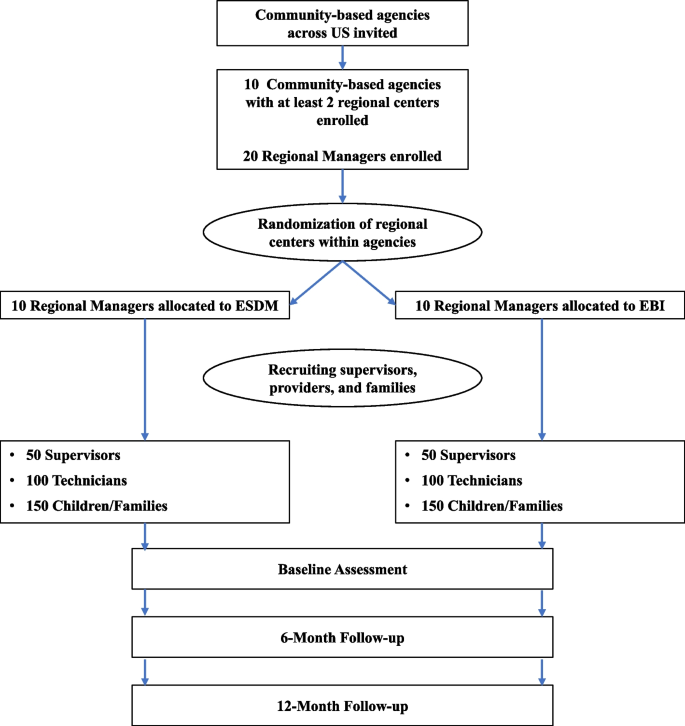
CONSORT flowchart for recruitment of community-based agencies and participants (projected)
CBAs throughout the US will be recruited, through emails, presentations at conferences and social media, to increase diversity and generalizability. Interested CBA leaders will be invited to meet with the study team. Those CBAs expressing interest and meeting inclusion criteria (see below) will be enrolled in the study. The randomization unit is the region. Within each CBA, regions will be randomized to either receive training in ESDM or continue usual early behavioral intervention (EBI). We chose to randomize at the region level to prevent potential contamination across providers and children, as our community partners indicated that children often receive treatment from multiple providers within a region. Using covariate constrained randomization, regions will be randomized so each CBA is represented in both ESDM and EBI. The variables considered in the constrained randomization include insurance mix (proportion of clients with Medicaid < 0.5 or ≥ 0.5) and size (number of autistic children under age 5 < 20 or ≥ 20). The study statistician will generate the randomization scheme before the enrollment of the first CBA and reveal the random assignments to the appropriate members of the study team. Members of the study team involved in assessments will remain unaware of the intervention assignment.
A cascading recruitment strategy will be used to first recruit agencies and then supervisors within participating regions. Supervisors will then recruit children and families and the technicians working with those children. Recruitment at each level will be facilitated through videos and handouts explaining the study and the processes. The research team will present to interested supervisors at team meetings. Supervisors will receive a handout and link to a video to share with technicians and families. Interested technicians and families will set up a time to talk with the research team about the study to determine interest.
This study was approved by the institutional review board at the University of California, Davis, protocol number 203076–2. This study is funded by the National Institute of Mental Health (NIMH; R01MH131703 and supported by the MIND Institute Intellectual and Developmental Disabilities Research Center (IDDRC) funded by the National Institute of Child Health and Human Development (NICHD; P50 HD103526).
Participants
Community-based agencies.
Eligibility criteria for autism CBA include: (a) serve at least 10 children on the autism spectrum under age 5 annually; (2) have at least 2 regions that can be randomized, and (3) accept Medicaid or equivalent payment (e.g., funding for low-income families through public service systems).
Supervisor participants
Supervisors will be recruited from enrolled agencies. To be eligible, supervisors must plan to be employed by the agency for at least 12 months, supervise programs for autistic children under age 5, supervise at least two technicians, and have not had previous ESDM training.
Technician participants
Technicians supervised by a participating supervisor and working with an enrolled child/family will be recruited. Inclusion criteria include planning to be employed at the agency for at least 12 months and have not had previous ESDM training.
Child / family participants
All child clients meeting eligibility criteria with a participating supervisor will be referred to the study and randomly selected for recruitment by the research team, with an expected average of 3 (range 2–4) per supervisor. Inclusion criteria include being under age 4 at program entry, having a current diagnosis of autism or being served by the agency due to high likelihood of autism. The family must speak English or Spanish and plan to receive intervention for at least 7 months. We will confirm autism diagnosis through record review. Payors typically require that children enter treatment with a cognitive assessment and an Autism Diagnostic Observation Scale (ADOS-2) [ 80 ]. For children under three who do not have a confirmed autism diagnosis we will complete the Telemedicine-based Autism Evaluation Tool for Toddlers and Young Children (TELE-ASD-PEDS) [ 81 ] found to be feasible and effective at assessing autism over telehealth [ 82 , 83 ].
Clinical intervention and community training
Clinical intervention.
Treatment will be conducted in the community context of the CBAs serving autistic children under age 5. They accept payment through insurance (public or private) or contracts with public agencies (e.g., Department of Developmental Services). CBA structure typically involves treatment teams that include a supervising clinician with a Master’s degree and credentials such as a BCBA, and 2–10 technicians. Supervisors conduct assessments, develop, and monitor treatment programs, provide caregiver coaching, and train and supervise technicians. Technicians have approximately 40 h of training in autism treatment and standardized supervision based on payor and board requirements; they conduct 1:1 intervention sessions with the child. Treatment intensity varies based on child need, family preference and payor requirements; however, most agencies provide 10–30 h per child per week of intervention which has been shown to be effective for this age group [ 55 ].
Early Start Denver Model (ESDM)
The Early Start Denver Model [ 30 , 72 ] focuses on teaching inside children’s play and care activities, carried out within a joint activity structure [ 84 ]. Adults follow children’s leads into activities, embed teaching objectives inside the activity, use the play or child’s activity goal as the reward, and build targeted skills by applying ESDM teaching strategies from developmental science and ABA principles. ESDM uses a developmental curriculum that defines the skills to be taught in each area of development based on each child’s strengths and needs. Core features of ESDM include child preferred materials and activities, use of both developmental strategies and naturalistic ABA strategies, a focus on teaching developmentally appropriate, well-generalized functional skills, caregiver involvement, and a focus on positive social interactions embedded within everyday activities. ESDM uses decision trees to determine when and how to vary the primary, child-centered teaching practices to assure child progress. ESDM Fidelity Tools measure the quality of implementation (see below). Providers in regions randomized to ESDM will receive ESDM training as described below.
Usual early behavioral intervention (EBI)
Treatment as usual will vary based on the agency. However, a majority of CBAs use Discrete Trial Teaching (DTT) based on the Lovaas model [ 18 ]. DTT involves 10 components described in numerous research publications [ 85 ]: capturing child physical and visual attention, adult presentation of the stimuli and instruction (antecedent), child behavior, adult reinforcement, correction procedures, 3–5 s interstimulus interval between trials, behavior-specific praise, and data recording. The use of DTT and NDBI strategies will be measured across both groups (see below) to characterize interventions delivered. Providers in regions randomized to continue EBI will continue service as usual.
Caregiver participation
Most CBAs include caregivers in some way because caregiver involvement is required by most funders. Providers in the EBI group will work with parents as usual. Providers in the ESDM condition will receive training in ESDM caregiver coaching strategies and will be asked to conduct caregiver coaching in the strategies at least monthly. Providers in the ESDM group will have training in use of “Help is in Your Hands” (HIIYH; www.helpisinyourhands.org ), an online program for parents that includes 4 modules focused on video examples of families using the strategies during daily routines. Modules cover: (1) Increasing Children’s Attention to People; (2) Increasing Children’s Communication; (3) Creating Joint Activity Routines; (4) ABCs of learning. HIIYH includes the core elements of ESDM which align with the 11 essential common elements shared across NDBIs.
CBA provider training
Working with our CBA partners, we determined that the best training approach for this trial would be using our experienced ESDM trainers to train CBA supervisors using a combination of synchronous and asynchronous methods. Trainer fidelity to the training model will be tracked. Technicians will receive asynchronous didactic trainings combined with coaching and feedback from their CBA supervisors (who will receive support from the project ESDM team).
Supervisor training
Training will begin with a series of asynchronous, interactive (e.g., quizzes and activities), web-based lessons, followed by online coaching of supervisors by the project team through video review of their ESDM implementation and both technician and caregiver coaching. Supervisors will be trained to fidelity in all aspects of the ESDM model: assessment, goal development, data collection and intervention strategies. They will be trained to fidelity in ESDM coaching strategies to be used with both caregivers and technicians. Supervisors will use on-line ESDM parent training videos, Help is in Your Hands (HIIYH), and the ESDM caregiver manual [ 86 ] for caregiver coaching. After reaching ESDM fidelity with their trainer, supervisors will attend several web-based monthly peer supervision meetings with other participating supervisors that will include ongoing fidelity checks, to assure their continued development of ESDM delivery skills (see Table 1 ).
Technician training
Technicians will complete asynchronous didactic training that includes an introduction to ESDM principles, strategies, and data collection. Supervisors will coach them in use of ESDM strategies using their agencies’ supervision model. Technicians will also view just-in-time (JIT) microlearning modules: specific 3 to 5-min lessons featuring a child of similar age, skill level and goals, just prior to an intervention session. Using JIT microlearning is an effective way to teach complex strategies [ 87 , 88 ]. JIT learning provides immediate information when it is needed by delivering content in manageable units that fit technicians’ clinical schedules. Each JIT microlearning provides ideas for learning activities to teach a specific goal and brief information about how autistic children learn. A library of JIT videos will be made available and assigned to technicians by their supervisor. See Table 1 for the technician training plan.
Training materials
Supervisors will receive three ESDM manuals: the core treatment manual [ 72 ], a manual written for caregivers [ 86 ], and a manual on coaching caregivers in ESDM [ 86 ]. They will also receive access to HIIYH videos, caregiver coaching materials, a fidelity checklist for technicians, access to an ESDM goal bank, data collection tools, and access to JIT modules.
Fidelity to the ESDM training model
To assess fidelity to the ESDM training model, we will measure three training variables: (1) supervisor and technician completion of online training modules, JIT modules, and training activities will be tracked via the web-based training system; (2) supervisor participation in coaching and supervision activities, including receiving feedback and fidelity ratings from project staff for the curriculum assessment administration and scoring, goal development, ESDM implementation, caregiver coaching and technician coaching; and (3) trainer ESDM fidelity scores based on 25% of their ESDM Trainer coaching and supervision sessions coded by project staff. Supervisors who do not meet fidelity standards will receive supervision until they meet fidelity standards.
Treatment fidelity measures
We will assess supervisor ESDM fidelity at multiple levels: child skill assessment and goal development, ESDM strategy use, data practices, and coaching others. Supervisors and technicians will be coded on ESDM Strategy Use. Scoring sheets and the fidelity measures are available from the first author.
ESDM progress tracking and goal development
Supervisors will be scored on assessment and goal fidelity (curriculum checklist described below) on the ESDM Certification Rating System (CRS). Once using ESDM, they will submit curriculum checklists and objectives for each child enrolled in the study.
Caregiver and technician coaching
A modified version of the Coaching Practices Rating Scale (CPRS) [ 89 ] will evaluate supervisors’ fidelity to coaching strategies. Supervisors in both groups will submit one caregiver session and one technician supervision video per month for the duration of the study to examine fidelity. Each of the 13 fidelity items will be rated on a binary scale of present or absent, and these scores will be summed for a total of 13 possible points. Intraclass correlation coefficients in prior studies indicated high reliability: ICC = 0.92 (CI: 0.71–0.98).
ESDM strategy use fidelity
The ESDM Fidelity Checklist [ 72 ] will assess use of ESDM practices. The ESDM Fidelity Checklist consists of 13 items: (a) management of child attention; (b) ABC teaching format; (c) instructional techniques; (d) modulating child affect/arousal; (e) management of unwanted behavior; (f) use of turn-taking/dyadic engagement; (g) child motivation is optimized; (h) adult use of positive affect; (i) adult sensitivity and responsivity; (j) multiple varied communicative functions; (k) adult language; (l) joint activity and elaboration; and (m) transition between activities.
Use of NDBI strategies
To understand treatment differentiation between the ESDM and EBI groups we will code the use of NDBI strategies across groups. To examine differentiation between the interventions in a more valid and unbiased manner than simply using ESDM codes across conditions we will use the eight-item NDBI-Fi measure [ 90 ] developed to capture common elements across NDBI interventions. This measure has adequate reliability, sensitivity to change, and concurrent, convergent, and discriminative validity. We will use the total score and examine differences by strategy type, responsiveness, and directives, consistent with recent studies [ 91 ].
Use of Discrete Trial Teaching (DTT) strategies
To understand the quality of intervention in the EBI condition we will use a fidelity tool from Rogers et al., 2021 to measure correct implementation of typical EBI teaching using discrete trial strategies. The fidelity tool measures the correct implementation of 9 components using a 5-point Likert scale applied to randomly selected 20-min sections of recorded treatment sessions (Yoder P, McEachin J, Wallace E, Leaf R, 2014, unpublished). Discrete Trial Training Fidelity of Treatment Rating). During instruction, children typically have blocks of teaching trials interspersed with short breaks that include therapist interactions. Treatment blocks will be coded with the DTT and NDBI tools. Breaks will be coded using the NDBI tool.
Providers will upload intervention and coaching videos throughout participation in the study which will be coded for the above fidelity by trained research team members naïve to study arm.
Procedures and measures
Child and family level outcomes will be assessed at three time points by trained assessors naïve to intervention condition: Baseline (BL), 6 months, and 12 months post BL. Outcome data will be collected by administering a brief battery of measures via distance technology that includes interview and survey and assessments with caregivers and video recordings. All assessors will be experienced MA or PhD level clinicians and supervised by a licensed clinical psychologist with over 20 years of experience in assessing young autistic children. All data will be entered directly into secure computer systems. Interviewers and video coders will be naive to group status (ESDM or EBI). The primary outcome will be child social communication and language (caregiver report and observational coding). Secondary outcomes are: (1) adaptive behavior and cognitive gains, (2) progress toward goals; (3) quality of life; (3) caregiver use of NDBI strategies; (4) increases in caregiver competence. We will assess engagement of the identified treatment mechanisms: child social motivation and caregiver use of NDBI strategies. Measures, constructs, and timing are listed in Table 2 . Commonly used measures are described briefly. Newer or less standard measures are described in more detail.
Participant retention will be facilitated by frequent contact with the research team, gift cards for measure completion, birthday cards sent to children and assessment reports. If child participants leave the agency we will still attempt to obtain measures at each timepoint.
Characterization measures
Treatment type and intensity.
Caregivers will complete an interview regarding intervention services received during the study period. In addition, we will track the number of CBA-provided treatment hours and caregiver coaching attendance via agency records.
Cognitive level
The Developmental Profile-4 (DP-4) [ 92 ]. Cognitive Scale is a standardized caregiver interview measure that produces norm-referenced scores for the cognitive domain. Test–retest reliability for the Cognitive scale is 0.83; internal consistency 0.82 to 0.94. Construct validity was verified with comparison of established measures (cognitive scale = 0.57).
Primary child outcomes: social communication and language
We will examine the effect of ESDM training on children’s social-communication and language using observational coding and caregiver report.
The Assessment of Phase of Preschool Language (APPL) [ 93 ] operationalizes research-based language development stages [ 94 ]. Language phases are derived from spoken language or augmentative communication systems and standardized assessments. The APPL characterizes expressive language domains: phonology, vocabulary, grammar, and pragmatics. For each domain, the APPL outlines the range of demonstrated skills that could meet criteria for each phase: Phase 1: Preverbal; Phase 2: First Words; Phase 3: Word Combinations, Phase 4: Sentences, or Phase 5: Complex Language. The APPL has strong interrater reliability and good construct validity A Language samples will be obtained from transcriptions of child-caregiver interactions recorded at each timepoint (see video collection). The APPL has been used to examine change in language level in multiple autism studies.
Vineland communication domain
The Vineland Adaptive Behavior Scales-3 (VABS-3) [ 95 ] consists of four domains of adaptive behavior: communication, daily living skills, socialization, and motor skills. It has been validated with children with developmental disabilities The scales yield normative standard scores (M = 100; SD = 15) that can be used for comparison across groups. The communication domain will be used to examine overall change in communication in the natural environment. The Vineland Interview edition will be used to obtain parent report of adaptive skills.
Secondary outcomes
Adaptive behavior.
Vineland Adaptive Behavior Scales-3 (VABS-3) [ 95 ] domains of adaptive behavior: daily living skills, socialization, and motor skills will be examined as secondary outcomes.
Caregiver and child quality of life
The CarerQoL [ 96 ]assesses perceived caregiver quality of life across seven dimensions. The Pediatric Quality of Life Inventory (PedsQL) [ 97 ] assesses children’s quality of life across four domains based on caregiver report and has been validated in an autism population [ 98 ].
Brief observation of social communication change
(BOSCC; [ 99 ]. The BOSCC consists of 15 items coded based on video observations on a 6-point scale ranging from 0 (the characteristic is not present) to 5 (the characteristic is present and it significantly impairs functioning). Thus, higher scores indicate more autism characteristics. Items 1–8 focus on Social Communication (SC), while items 9–12 capture Restricted and Repetitive Behaviors (RRBs). The BOSCC results in SC (i.e., eye contact, facial expressions, gestures, vocalizations, integration of vocal and non-vocal communication, frequency/function of social overtures, frequency/quality of social responses, engagement in activities/interaction, and play with objects) and RRB domain totals (unusual sensory interests, hand/finger or other complex mannerisms, and unusually repetitive interests/stereotyped behaviors). The Core total combines the SC and RRB scores. We will not be targeting autistic characteristics in our project. We will include the BOSCC as a secondary measure of social communication to facilitate comparison across studies.
Early intervention support and processes
The Family Outcomes Survey-Revised (FOS-R) [ 100 ] is a 41-item measure uses a 5-item Likert scale to assess parents’ perceived strengths and needs as they relate to the early intervention support they receive. The FOS-R has good internal consistency in English (subscales ranging from 0.73 to0.95 for Cronbach’s alpha). [ 100 ] The Measure of Processes of Care—20 (MPOC—20) [ 101 ] measures how family-centered parents perceive their child’s intervention services. The 20-item scale asks parents to rate how much people who work with their child (a) enable partnership, (b) provide general information, (c) provide specific information about their child, (d) coordinate comprehensive care for the child and family, and (e) are respectful and supportive. The scale has good internal consistency with coefficients ranging from 0.83 to 0.90 [ 101 ].
Intervention side effects & harm
Emotion Dysregulation Inventory-Young Children (EDI-YC) [ 102 ] short form measures emotion dysregulation with two scales, reactivity and dysphoria. Reactivity is characterized by rapidly escalating, intense, labile negative affect and difficulty downregulating that affect. Dysphoria is characterized by poor up regulation of positive emotion. This 14-item scale has been used with children on the autism spectrum. The measure has good validity and is supported by expert review. If children have > 1sd of change in this measure over time or providers or parents report regression the research team and data safety and monitoring board will assess for discontinuing or modifying the intervention and/or study participation.
Treatment mechanisms variables
Social motivation and caregiver ndbi fidelity.
Social motivation will be measured in two ways and those assessments will be used to examine proximal and distal changes to the intervention mechanism and its role as a moderator.
Pervasive Developmental Disorder Behavior Inventory (PDDBI) [ 103 ] examines characteristics of autism in children between 18 months and 12.5 years through caregiver reports. It has high internal consistency (0.84—0.97), inter-rater reliability (ICC = 0.75—0.93), and good construct validity. The Social Approach subscale will provide a distal measures of social motivation (treatment mechanism) and includes 36 items representing all three behavioral manifestations of social motivation. Studies using the Social Approach subscale report good consistency (α = 0.94) and test–retest reliability of 0.93 [ 104 , 105 ].
Joint Engagement Rating Inventory (JERI) [ 106 ] will be a proximal, objectively rated measure of child social motivation during adult/child interactions. The JERI is widely used to examine child behavior in autism studies and has high validity and reliability. One score per code will be assigned to each Communication Play Protocol observation (see below) and averaged across the 3 activities for analyses.
Caregiver NDBI fidelity
Caregiver-child interaction videos (see below) will be coded using the NDBI-Fi Checklist (see Fidelity measures and video collection).
Video data collection
Video data will be collected for outcomes at three time points using the Communication Play Protocol (CPP) [ 107 ]. The CPP produces video records of three 5-min semi-structured scenes that focus on requesting, social interacting, and shared commenting. We will collect two CPP videos at each time point, one with the caregiver and one with a provider that does not know the child and is naïve to condition. Video data will be coded using the (1) APPL for children language outcomes; (2) JERI to assess social motivation; and (3) and NDBI-Fi to examine caregiver use of ESDM/NDBI strategies.
Video coding procedures
Trained coders naïve to group, timepoint, and study aims will code video measures to avoid bias. Each coder will be trained in one scoring system to reliability (80% agreement over 3 videos). For each measure, a random sample of 20% of sessions will be double coded for inter-rater reliability throughout coding. If agreement drops below 80%, training will be provided until agreement is achieved.
Analytic plan
In this trial, there are several levels of clustering: repeated observations are nested within the child/caregiver, the child/caregiver is nested within the team, teams are nested within the region, and regions are nested within CBAs. Therefore, we will use a modeling strategy that includes random intercepts for region and/or CBA, team, and random child/caregiver effects (intercept, slopes, as appropriate). All primary analyses will be conducted on an intent-to-treat basis using a generalized linear mixed-effects models framework [ 103 ], which can accommodate continuous, binary, and count outcomes through an appropriate choice of link function. Preliminary analyses will involve examining the outcomes and covariates to verify their appropriateness, identifying patterns of missing data, and conducting a multivariate outlier analysis. Model validation will be carried out using both analytical and graphical techniques to check core assumptions such as linearity, distribution, and homoscedasticity. Transformations of outcome variables will be considered if suggested by the model validation analyses. All analyses will include available relevant biological variables, including child or caregiver sex and age and baseline characteristics if there is any evidence of randomization imbalance in them. Randomization should produce intervention and control groups that are comparable and balanced. As a first-order check on confounding, we will examine the success of randomization by comparing baseline characteristics of children, caregivers, and providers assigned to the two study arms. Where clinically significant differences are apparent, child-, caregiver-, and provider-specific covariates will be added to the statistical models as fixed predictors to examine whether the intervention effect is robust in their presence.
Primary and secondary outcomes
The analytic approach for each primary and secondary outcome measure will follow the same general model-building strategy. For outcomes assessed at baseline, 6 months, and 12 months, the models will include fixed effects for time (baseline, 6-, and 12-months), group, and their interaction, as well as covariates (e.g., child/caregiver sex, age, etc.) and random effects for child/caregiver, team, or region to account for clustering. The interaction between time and group will directly test the hypothesis that participants in the ESDM group show greater improvement than those in the EBI group. In all models, we will consider adding relevant covariates related to child/caregiver or provider-level characteristics if randomization at the region level did not ensure comparability between the two groups [ 108 ].
Moderation analyses
Moderation analyses will explore the differential effectiveness of the two interventions by maternal level of education (as a proxy for SES), child race/ethnicity, and technician ESDM fidelity. We will build upon the primary models with treatment group by time effects by incorporating interaction terms for moderators of interest and conducting sub-group analyses. For each target moderator (e.g., maternal education), we will add the 3-way treatment group by time by moderator interaction term (and all lower-order 2-way and main effects) to determine whether differences between treatment groups in change over time for a given outcome variable are modified by target moderators. A significant 3-way interaction effect will indicate the presence of treatment effect heterogeneity between subgroups. Following this, we will conduct simple effect analysis to estimate treatment effect differences (i.e., difference in changes over time between arms) within each subgroup. For adherence to ESDM fidelity at the technician level, we expect substantial differences between treatment groups, and plan to investigate this as a moderator of all child outcomes.
Mediation analyses
Conceptually, social motivation (measured by PDDBI and JERI) and caregiver NDBI fidelity can be viewed as an intermediated outcome (mediator, M). The intervention may affect the primary outcomes indirectly through a pathway of the mediator (M). To test the mediated effect (or mechanism of change), we will conduct a mediation analysis by extending the generalized mixed effects models specified for assessing treatment group differences in primary outcome variables by adding continuous ratings of social motivation and parent fidelity, respectively, as predictors to the model describing language improvement. The mediation analysis will follow a standard series of steps: (1) Test for the direct effect of the treatment group on the primary outcome variable as represented by the time by treatment group interaction effects (i.e., the primary model); (2) Test for the time by treatment group interaction effect using the measure of social motivation (or parent fidelity, respectively) as the dependent variable in an analogous mixed effects model to assess the coefficient for group differences in change over time in the target mediator; (3) Return to the model in step 1 and add the main effect of time-varying social motivation scores (or parent fidelity, respectively) in predicting outcome scores to assess the direct relationship between the target mediator and outcome while controlling for the time by treatment group effect on outcome; (4) Calculate the degree and significance of the indirect effect using Monte Carlo simulations of the estimated coefficients and their respective standard errors.
Missing data
Our protocols include numerous provisions to minimize the amount of missing data, and our team has achieved high retention rates in previous work. However, some data will inevitably be missing. We will use standard methods to evaluate missing data assumptions and to determine alternative analytic strategies if needed. One of three approaches will be used: First, if the proportion of missing data is small and there is evidence that data are missing at random (MAR), all available data will be analyzed using the maximum-likelihood estimation procedures described above. Second, if the proportion of missing data is nontrivial with evidence that data are MAR, multiple imputations for repeated measurements will be used to generate complete data. Third, if there is evidence of a non-MAR mechanism for missing data, pattern mixture models will be used to evaluate and control for the missing data pattern.
Power considerations
Given that the proposed analyses for primary outcomes will employ mixed effects modeling of clustered data to assess differences in changes from baseline between treatment groups, power analyses were conducted using Monte Carlo simulations of multi-level models in SAS (SAS Institute Inc., Cary, NC). Expected fixed effect values for effects of interest (e.g., treatment group by time interactions) were obtained from prior research on ESDM treatments and developmental change [ 109 ]. We assumed a range of plausible intraclass correlation coefficient (ICC) values for the random effects of child/caregiver dyad (0.3 to 0.5), team (0.1 to 0.25), and region (0.05 to 0.1) based on previous community intervention studies and pilot data and accounted for a 10% dropout rate. We used a type I error level of 5%.
Under each scenario, our proposed sample size of 300 children/caregivers, 100 teams, and 20 centers would provide at least 80% power to detect a standardized improvement in children’s social communication and language of d = 0.6. We assumed that 10% of children would not contribute any data; however, the participants who dropped out would have provided some data and will contribute to the analyses. Therefore, our calculations are conservative.
Procedures and measures addressing our exploratory implementation aim
We will measure implementation during the three EPIS phases: adoption (recruitment), implementation (ESDM training and delivery), and predicted sustainment (after the research study). We will measure acceptability, appropriateness, and feasibility of the intervention, and provider, family, and organizational characteristics to identify determinants of ESDM implementation. We will use a combination of surveys and structured interviews (see Table 3 ).
Acceptability of Intervention Measure (AIM), Intervention Appropriateness Measure (IAM), & Feasibility of Intervention Measure (FIM) [ 110 ] determine the extent to which a participant believes an intervention is acceptable, appropriate, and feasible and have strong internal consistency (AIM α = 0.89; IAM α = 0.87; FIM α = 0.89). All participating providers and caregivers will complete these scales every six months during participation. Total score on each scale will be used.
Adaptations to Evidence-Based Practices Scale (AES) [ 111 ] is a 6-item scale assessing provider adaptations to EBPs delivered. Providers rate six items using a 5-point Likert scale (0 “not at all,” 4 “a very great extent”) to indicate the extent to which they made each type of adaptation when delivering a specified EBP, including (a) modifying the presentation of EBP strategies, (b) shortening or condensing the pacing of the EBP, (c) lengthening or extending the pacing of the EBP, (d) integrating supplemental content or strategies, (e) removing or skipping components, and (d) adjusting the order of sessions or components.
Provider Report of Sustainment Scale (PRESS) [ 112 ] captures provider report of continued use of an intervention. The PRESS has good psychometric properties across multiple interventions and service systems and strong construct validity.
Autism Self-Efficacy Scale for Teachers (ASSET) [ 113 ] is a 30-item self-report measure of providers’ beliefs about their ability to implement appropriate teaching strategies when working with autistic children. We adapted the measure for use with community providers who rate their efficacy in carrying out several different assessment, intervention, and evidence-based practices relevant to autism early intervention. Providers rate their self-efficacy using a scale from 0 ( cannot do at all ) to 100 ( highly certain can do ). The total score is calculated as the mean score across the 30 items. Scale internal consistency is 0.96.
The Implementation Climate Scale (ICS) [ 114 , 115 ] measures employees’ shared perceptions of the policies, practices, procedures, and behaviors that are expected, rewarded, and supported to facilitate effective EBI implementation. The ICS has good psychometric properties across several settings including good internal consistency and good construct validity.
Implementation Interview: Semi-structured interviews will be conducted with regional managers, supervisors, technicians, and caregivers to gather additional information on ESDM feasibility, usability, acceptability, fit (including cultural fit with family needs) and plans for sustainment. We will conduct interviews with a subset of participants until we reach saturation (approximately 30 in each group). Facilitators will follow a semi-structured interview guide [ 116 , 117 ].
Data analysis (exploratory)
Descriptive data regarding feasibility, acceptability and fit and qualitative interview data will be examined every 6 months. These data will be used iteratively through the implementation phase of the trial to make culturally relevant adaptations to the intervention. Adaptations will be carefully logged and tracked and resulting outcomes monitored using recommended methods. We will explore descriptive statistics for the various measures of organizational and provider characteristics and participation and will use predictive models (with multi-level modeling as above) to understand appropriateness, feasibility, and acceptability in the ESDM treatment group. Given that measures of implementation (e.g., provider fidelity) are important for understanding the feasibility of scaling ESDM to CBAs, we will analyze such implementation measures as dependent variables and examine other variables in Table 3 (e.g., organization characteristics, perceived fit) as predictors of individual provider variability in fidelity.
Qualitative Data analysis. NVivo QSR 11 [ 118 ] will be used for qualitative analyses. A framework-driven analytic approach will guide the coding process [ 44 , 119 ]. Coders will use an iterative coding and review process informed by grounded theory [ 120 ].
Integration of qualitative and quantitative analyses
A sequential Quan > QUAL mixed method design will be employed [ 121 ]. The primary functions of the mixed-methods analyses will be convergence and expansion.
This project is one of the first large-scale, randomized hybrid effectiveness trials of an autism early intervention. The large and diverse sample will allow us to examine how well ESDM conducted by CBAs activates the hypothesized mechanism of the intervention, social motivation. Understanding how social motivation works to determine response to NDBI will allow for improvement of intervention strategies that enhance social motivation to facilitate improved outcomes. Examining the role of social motivation in a diverse community sample of toddlers and young children on the autism spectrum will inform the social motivation hypothesis of autism, which, to date, has only been tested in research samples. Additionally, the relationship between caregiver fidelity and child outcomes has not been examined in a large, diverse sample that may have varying cultural values regarding intervention delivery.
The impact of the study is likely to be greater because the project utilizes partnerships with family members, autistic adults, and CBAs to develop both the proposal and to carry out the project, assuring relevance of the research questions to the community and increase the potential for uptake of the intervention [ 122 ]. Our community partners assure us that this adapted ESDM training and intervention model fits with the current CBA intervention and financing structure. By engaging an extensive network of CBAs supporting autistic children as the intervention deliverers, positive findings can readily generalize to other CBAs and increase access to diverse regions and children.
The proposal is responsive to the neurodiversity perspective. When used correctly, ESDM builds in respect for children’s interests and preferences with a strengths-based approach. ESDM emphasizes the importance of responsive, sensitive relationships between adults and children and on outcomes associated with development, quality of life, and adaptation instead of reduction of unwanted behaviors and “normalization”. The developmentally and culturally appropriate naturalistic interactions of ESDM have the potential to increase the acceptability of early intervention and increase adoption by families concerned about the long-term effects of ABA on their child’s emotional well-being.
The effectiveness trial methodology will be harnessed to examine implementation determinants to facilitate scale up. Hybrid Type 1 trials allow implementation determinants to be identified more comprehensively and earlier than in a sequential model [ 123 ]. This study uses an established implementation framework, EPIS, to facilitate effective prospective design to support future scale-up studies with diverse populations. Implementation data can be used iteratively through the implementation phase of the trial to make culturally relevant adaptations to the intervention. Adaptations, which will be carefully logged and tracked, and resulting outcomes monitored using recommended methods [ 124 ] will increase the scalability of the intervention.
Results of the project will be disseminated to several different audiences using methods specifically designed to reach each of them. Target audiences are community-based agencies, researchers and their students, state and community program administrators and providers, and parents and funders. A project website will describe the project and provide tools and information for all audiences and a method for requesting more information about the study for interested community agencies. The website will include infographics and lay abstracts of study presentation and publications. Data will be presented through conference presentations, social media, journal articles, lay publications and presentations to policy makers and funders. All publications will be made publicly available through the University of California and PubMed Central.
Availability of data and materials
Data will be available through the NIMH Data Archive. Descriptive/raw data will be submitted semi-annually, and additional data will be submitted at the time of publication. In addition, all datasets developed for the study will be available from the corresponding author upon reasonable request.
Abbreviations
Community Based Agency
Early Behavioral Intervention
Early Start Denver Model
Naturalistic Developmental Behavior Intervention
Autism Prevalence Higher, According to Data from 11 ADDM Communities | CDC Online Newsroom | CDC [Internet]. [cited 2024 Jul 28]. Available from: https://www.cdc.gov/media/releases/2023/p0323-autism.html .
Rogge N, Janssen J. The Economic Costs of Autism Spectrum Disorder: A Literature Review. J Autism Dev Disord. 2019;49:2873–900.
Article PubMed Google Scholar
Cakir J, Frye RE, Walker SJ. The lifetime social cost of autism: 1990–2029. Res Autism Spectr Disord. 2020;72: 01502.
Article Google Scholar
Fuller EA, Oliver K, Vejnoska SF, Rogers SJ. The Effects of the Early Start Denver Model for Children with Autism Spectrum Disorder: A Meta-Analysis. Brain Sciences 2020, Vol 10, Page 368. 2020;10:368.
Fuller EA, Kaiser AP. The Effects of Early Intervention on Social Communication Outcomes for Children with Autism Spectrum Disorder: A Meta-analysis. Journal of Autism and Developmental Disorders 2019 50:5. 2019;50:1683–700.
Hampton LH, Kaiser AP. Intervention effects on spoken-language outcomes for children with autism: a systematic review and meta-analysis. J Intellect Disabil Res. 2016;60:444–63.
Sandbank M, Bottema-Beutel K, Crowley S, Cassidy M, Dunham K, Feldman JI, et al. Project AIM: Autism intervention meta-analysis for studies of young children. Psychol Bull. 2020;146:1–29.
Chasson GS, Harris GE, Neely WJ. Cost comparison of early intensive behavioral intervention and special education for children with autism. J Child Fam Stud. 2007;16:401–13.
Butter EM, Wynn J, Mulick JA. Early intervention: Critical to autism treatment. Pediatr Ann. 2003;32:677–84.
Payakachat N, Tilford JM, Kuhlthau KA. Parent-Reported Use of Interventions by Toddlers and Preschoolers With Autism Spectrum Disorder. Psychiatr Serv. 2018;69:186–94.
Mire SS, Hughes KR, Manis JK, Goin-Kochel RP. Autism Treatment: Families’ Use Varies Across U.S. Regions: https://doi.org/10.1177/1044207318766597 . 2018;29:97–107.
Smith KA, Gehricke JG, Iadarola S, Wolfe A, Kuhlthau KA. Disparities in service use among children with autism: A systematic review. Pediatrics. American Academy of Pediatrics; 2020.
Williams ME, Harley EK, Quebles I, Poulsen MK. Policy and Practice Barriers to Early Identification of Autism Spectrum Disorder in the California Early Intervention System. J Autism Dev Disord. 2021;51:3423–31.
Smith KA, Gehricke JG, Iadarola S, Wolfe A, Kuhlthau KA. Disparities in service use among children with autism: A systematic review [Internet]. Pediatrics. American Academy of Pediatrics; 2020 [cited 2021 Jan 28]. Available from: https://pubmed.ncbi.nlm.nih.gov/32238530/ .
Murza KA, Schwartz JB, Hahs-Vaughn DL, Nye C. Review Joint attention interventions for children with autism spectrum disorder: a systematic review and meta-analysis. 51:236–51.
Reichow B. Overview of Meta-Analyses on Early Intensive Behavioral Intervention for Young Children with Autism Spectrum Disorders.
Sandbank M, Bottema-Beutel K, Woynaroski T. Intervention Recommendations for Children with Autism in Light of a Changing Evidence Base. JAMA Pediatr. American Medical Association; 2021. p. 341–2.
Lovaas OI. Behavioral treatment and normal educational and intellectual functioning of young autistic children. J Consult Clin Psychol. 1987;55:3–9.
Hampton LH, Sandbank MP. Keeping up with the evidence base: Survey of behavior professionals about Naturalistic Developmental Behavioral Interventions: https://doi.org/10.1177/13623613211035233 . 2021.
Sheinkopf SJ, Siegel B. Home based behavioral treatment of young children with autism. J Autism Dev Disord. 1998;28:15–23.
Luiselli JK, Cannon BO, Ellis JT, Sisson RW. Home-Based Behavioral Interventions for Young Children With Autism/Pervasive Developmental Disorder: a Preliminary Evaluation of Outcome in Relation to Child Age and Intensity of Service Delivery. Autism. 2000;4:426–38.
Bibby P, Eikeseth S, Martin NT, Mudford OC, Reeves D. Progress and outcomes for children with autism receiving parent-managed intensive interventions. Res Dev Disabil. 2002;23:81–104.
Smith T, Buch GA, Gamby TE. Parent-directed, intensive early intervention for children with pervasive developmental disorder. Res Dev Disabil. 2000;21:297–309.
Magiati I, Charman T, Howlin P. A two-year prospective follow-up study of community-based early intensive behavioural intervention and specialist nursery provision for children with autism spectrum disorders. J Child Psychol Psychiatry. 2007;48:803–12.
Magiati I, Moss J, Charman T, Howlin P. Patterns of change in children with Autism Spectrum Disorders who received community based comprehensive interventions in their pre-school years: a seven year follow up study. Res Autism Spectr Disord. 2011;5:1016–27.
Flanagan HE, Perry A, Freeman NL. Effectiveness of large-scale community-based Intensive Behavioral Intervention: A waitlist comparison study exploring outcomes and predictors. Res Autism Spectr Disord. 2012;6:673–82.
Cohen H, Amerine-Dickens M, Smith T. Early intensive behavioral treatment: Replication of the UCLA Model in a community setting. J Dev Behav Pediatr. 2006;27:S145–55.
Waters CF, Dickens MA, Thurston SW, Lu X, Smith T. Sustainability of Early Intensive Behavioral Intervention for Children With Autism Spectrum Disorder in a Community Setting. Behav Modif. 2020;44:3–26.
Schreibman L, Dawson G, Stahmer AC, Landa R, Rogers SJ, McGee GG, et al. Naturalistic Developmental Behavioral Interventions: Empirically Validated Treatments for Autism Spectrum Disorder. J Autism Dev Disord. 2015;45.
Dawson G, Rogers S, Munson J, Smith M, Winter J, Greenson J, et al. Randomized, controlled trial of an intervention for toddlers with autism: The Early Start Denver Model. Pediatrics. 2010;125:e17-23.
Gengoux GW, Abrams DA, Schuck R, Millan ME, Libove R, Ardel CM, et al. A pivotal response treatment package for children with autism spectrum disorder: An RCT. Pediatrics. 2019;144.
Kasari C, Lawton K, Shih W, Barker TV, Landa R, Lord C, et al. Caregiver-mediated intervention for low-resourced preschoolers with autism: an RCT. Pediatrics. 2014;134:e72–9.
Article PubMed PubMed Central Google Scholar
Rogers S, Estes A, Lord C, al. et. A multisite randomized controlled two-phase trial of the Early Start Denver Model compared to treatment as usual. J Am Acad Child Adolesc Psychiatry. 2019;58:853–65.
Zwaigenbaum L, Bauman ML, Choueiri R, Kasari C, Carter A, Granpeesheh D, et al. Early Intervention for Children With Autism Spectrum Disorder Under 3 Years of Age: Recommendations for Practice and Research. Pediatrics. 2015;136:S60-81.
Tiede G, Walton KM. Meta-analysis of naturalistic developmental behavioral interventions for young children with autism spectrum disorder: https://doi.org/10.1177/1362361319836371 . 2019;23:2080–95.
Song J, Reilly M, Reichow B. Overview of Meta-Analyses on Naturalistic Developmental Behavioral Interventions for Children with Autism Spectrum Disorder. J Autism Dev Disord. 2024 [cited 2024 Jul 28]; Available from: https://pubmed.ncbi.nlm.nih.gov/38170431/ .
Robinson SE. Teaching paraprofessionals of students with autism to implement pivotal response treatment in inclusive school settings using a brief video feedback training package. Focus Autism Other Dev Disabl. 2011;26:105–18.
Hardan AY, Gengoux GW, Berquist KL, Libove RA, Ardel CM, Phillips J, et al. A randomized controlled trial of Pivotal Response Treatment Group for parents of children with autism. J Child Psychol Psychiatry. 2015.
Chapman R. Neurodiversity and the Social Ecology of Mental Functions. Perspectives on Psychological Science. 2021.
den Houting J. Neurodiversity: An insider’s perspective. Autism. 2019;23:271–3.
Schuck RK, Tagavi DM, Baiden KMP, Dwyer P, Williams ZJ, Osuna A, et al. Neurodiversity and Autism Intervention: Reconciling Perspectives Through a Naturalistic Developmental Behavioral Intervention Framework. J Autism Dev Disord. 2021;2021:1–21.
Google Scholar
Smith IM, Flanagan HE, Ungar WJ, D’entremont B, Garon N, Den Otter J, et al. Comparing the 1-Year Impact of Preschool Autism Intervention Programs in Two Canadian Provinces. Autism Res. 2019;12:667–81.
Mandell D, Shin S, Stahmer AC, Xie M, Marcus SE. A randomized comparative effectiveness trial fo two school-base autism interventions. Autism.
Shire SY, Chang Y-C, Shih W, Bracaglia S, Kodjoe M, Kasari C. Hybrid implementation model of community-partnered early intervention for toddlers with autism: a randomized trial. 2016.
Vernon H, Proctor D, Bakalovski D, Moreton J. Simulation tests for cervical nonorganic signs: a study of face validity. J Manipulative Physiol Ther. 2010/02/02. 33:20–8.
Rogers SJ, Stahmer UCDavis Meagan Talbott UCDavis Gregory Young UCDavis Elizabeth Fuller UCDavis A. Feasibility of Delivering Parent-Implemented NDBI Interventions in Low Resource Regions: A Pilot Randomized Controlled Study. 2020.
Stahmer AC, Rieth SR, Dickson KS, Feder J, Burgeson M, Searcy K, et al. Project ImPACT for Toddlers: Pilot outcomes of a community adaptation of an intervention for autism risk. Autism. 2020;24.
Pickard K, Meza R, Drahota A, Brikho B. They’re Doing What? A Brief Paper on Service Use and Attitudes in ASD Community-Based Agencies. J Ment Health Res Intellect Disabil. 2018;11:111.
Schwartz IS, Sandall SR, McBride BJ, Boulware G-L. Project DATA (Developmentally appropriate treatment for autism): An inclusive school based approach to educating young children with autism. Topics Early Child Spec Educ. 2004;24:156–68.
Vivanti G, Stahmer A. Early intervention for autism: Are we prioritizing feasibility at the expenses of effectiveness? A cautionary note Autism. 2018;22:770–3.
PubMed Google Scholar
Professional Behavior Analysts Association of. 2017. Available from: www.APBAHome.net .
Association of Professional Behavior Analysts. Identifying Applied Behavior Analysis Interventions. 2017. Report No.: www.apbahome.net.
Behavior Analyst Certification Board. Professional and ethical compliance code for behavior analysts. Littleton, CO; 2014.
Council of Autism Service Providers. Applied behavior analysis treatment of autism spectrum disorder: Practice guidelines for healthcare funders, 2nd Ed and managers. 2020.
Rogers SJ, Yoder P, Estes A, Warren Z, McEachin J, Munson J, et al. A Multisite Randomized Controlled Trial Comparing the Effects of Intervention Intensity and Intervention Style on Outcomes for Young Children With Autism. J Am Acad Child Adolesc Psychiatry. 2021;60:710–22.
Mirenda P, Colozzo P, Smith V, Kroc E, Kalynchuk K, Rogers SJ, et al. A Randomized, Community-Based Feasibility Trial of Modified ESDM for Toddlers with Suspected Autism. J Autism Dev Disord. 2022.
Hampton LH. Improving preemptive intervention approaches for children with a high likelihood for autism. Conference for Research Innovations in Early Intervention. San Diego, CA.
Holzinger D, Laister D, Vivanti G, Barbaresi WJ, Fellinger J. Feasibility and Outcomes of the Early Start Denver Model Implemented with Low Intensity in a Community Setting in Austria. J Dev Behav Pediatr. 2019;40:354–63.
Vivanti G, Dissanayake C, Duncan E, Feary J, Capes K, Upson S, et al. Outcomes of children receiving Group-Early Start Denver Model in an inclusive versus autism-specific setting: A pilot randomized controlled trial. Autism. 2019;23:1165–75.
Dawson G, Bernier R, Ring RH. Social attention: A possible early indicator of efficacy in autism clinical trials. J Neurodev Disord. 2012;4:1–12.
Mundy P. Joint attention and social-emotional approach behavior in children with autism. Dev Psychopathol. 1995;7:63–82.
Chevallier C, Kohls G, Troiani V, Brodkin ES, Schultz RT. The social motivation theory of autism. Trends Cogn Sci. 2012;16:231–9.
Osterling JA, Dawson G, Munson JA. Early recognition of 1-year-old infants with autism spectrum disorder versus mental retardation. Dev Psychopathol. 2002;14:239–51.
Geurts HM, Luman M, Van Meel CS. What’s in a game: the effect of social motivation on interference control in boys with ADHD and autism spectrum disorders. J Child Psychol Psychiatry. 2008;49:848–57.
Kohls G, Chevallier C, Troiani V, Schultz RT. Social ‘wanting’ dysfunction in autism: neurobiological underpinnings and treatment implications. Journal of Neurodevelopmental Disorders. 2012;4:1–20.
Hedger N, Dubey I, Chakrabarti B. Social orienting and social seeking behaviors in ASD A meta analytic investigation. Neurosci Biobehav Rev. 2020;119:376–95.
Camarata S, Yoder P. Language transactions during development and intervention: theoretical implications for developmental neuroscience. Int J Dev Neurosci. 2002;20:459–65.
Kim H, Ahn J, Lee H, Ha S, Cheon KA. Differences in Language Ability and Emotional-Behavioral Problems according to Symptom Severity in Children with Autism Spectrum Disorder. Yonsei Med J. 2020;61:880.
Kuhl PK, Coffey-Corina S, Padden D, Dawson G. Links between social and linguistic processing of speech in preschool children with autism: behavioral and electrophysiological measures. Dev Sci. 2005;8:F1-12.
Dawson G, Toth K, Abbott R, Osterling J, Munson J, Estes A, et al. Early Social Attention Impairments in Autism: Social Orienting, Joint Attention, and Attention to Distress. Dev Psychol. 2004;40:271–83.
Su PL, Rogers SJ, Estes A, Yoder P. The role of early social motivation in explaining variability in functional language in toddlers with autism spectrum disorder. Autism. 2021 [cited 2022 Jan 11];25:244–57. Available from: https://doi.org/10.1177/1362361320953260 .
Rogers SJ, Dawson G. Early start denver model for young children with autism: Promoting language, learning, and engagement. Guildford Press; 2010.
Zwaigenbaum L, Bauman ML, Stone WL, Yirmiya N, Estes A, Hansen RL, et al. Early Identification of Autism Spectrum Disorder: Recommendations for Practice and Research. Pediatrics. 2015 [cited 2024 Aug 5];136 Suppl 1:S10–40. Available from: https://pubmed.ncbi.nlm.nih.gov/26430168/ .
Zitter A, Rinn H, Szapuova Z, Avila-Pons VM, Kirsty ·, Coulter L, et al. Does Treatment Fidelity of the Early Start Denver Model Impact Skill Acquisition in Young Children with Autism? J Autism Dev Disord. Available from: https://doi.org/10.1007/s10803-021-05371-4 .
Rogers SJ, Estes A, Lord C, Vismara L, Winter J, Fitzpatrick A, et al. Effects of a brief Early Start Denver Model (ESDM)–based parent intervention on toddlers at risk for autism spectrum disorders: a randomized controlled trial. J Am Acad Child Adolesc Psychiatry. 2012;51:1052–65.
Ingersoll BR. Teaching Social Communication A Comparison of Naturalistic Behavioral and Development, Social Pragmatic Approaches for Children With Autism Spectrum Disorders. 2010; Available from: http://jpbi.sagepub.com .
Rogers SJ, Estes A, Vismara L, Munson J, Zierhut C, Greenson J, et al. Enhancing Low-Intensity Coaching in Parent Implemented Early Start Denver Model Intervention for Early Autism: A Randomized Comparison Treatment Trial. J Autism Dev Disord. 2019 [cited 2024 Aug 5];49:632–46. Available from: https://pubmed.ncbi.nlm.nih.gov/30203308/ .
Aarons G, Hurlburt M, Horwitz SM. Advancing a conceptual model of evidnece-based practice implementation in public service sectors. Administration and Policy in Mental Health and Mental Health Services. 2011;38:4–23.
Baumann AA, Cabassa LJ. Reframing implementation science to address inequities in healthcare delivery. BMC Health Serv Res. 2020;20.
Lord C, Rutter M, DiLavore PC, Risi S, Gotham K, Bishop SL, et al. Autism diagnostic observation schedule–2nd edition (ADOS-2). Los Angeles, CA: Western Psychological Services; 2012.
Corona LL, Weitlauf AS, Hine J, Berman A, Miceli A, Nicholson A, et al. Parent Perceptions of Caregiver-Mediated Telemedicine Tools for Assessing Autism Risk in Toddlers. J Autism Dev Disord. 2020;51:476–86.
Article PubMed Central Google Scholar
Stainbrook JA, Weitlauf AS, Juárez AP, Taylor JL, Hine J, Broderick N, et al. Measuring the service system impact of a novel telediagnostic service program for young children with autism spectrum disorder. Autism. 2019;23:1051–6.
Juárez AP, Weitlauf AS, Nicholson A, Pasternak A,Broderick · Neill, Hine J, et al. Early Identification of ASD Through Telemedicine: Potential Value for Underserved Populations. 2018;48:2601–10.
Ratner N, Bruner J. Games, social exchange and the acquisition of language. J Child Lang. 1978;5:391–401.
Sarokoff RA, Sturmey P. The effects of behavioral skills training on staff implementation of discrete-trial teaching. J Appl Behav Anal. 2004;37:535–8.
Rogers SJ, Dawson Geraldine, Vismara LA. An early start for your child with autism : using everyday activities to help kids connect, communicate, and learn. 2012;342.
Zhang J, West RE. Designing Microlearning Instruction for ProfessionalDevelopment Through a Competency Based Approach. TechTrends. 2020;64:310–8.
Javorcik T, Polasek R. Comparing the Effectiveness of Microlearning and eLearning Courses in the Education of Future Teachers; Comparing the Effectiveness of Microlearning and eLearning Courses in the Education of Future Teachers. 2019 17th International Conference on Emerging eLearning Technologies and Applications (ICETA). 2019.
Rush M’ DD, Shelden LL. Insights into Early Childhood and Family Support Practices Validity of the Coaching Practices Rating Scale CASE inPoint. 2006.
Frost KM, Brian J, Gengoux GW, Hardan A, Rieth SR, Stahmer A, et al. Identifying and measuring the common elements of naturalistic developmental behavioral interventions for autism spectrum disorder: Development of the NDBI-Fi. Autism. 2020;24:2285–97.
Sone BJ, Kaat AJ, Roberts MY. Measuring parent strategy use in early intervention: Reliability and validity of the Naturalistic Developmental Behavioral Intervention Fidelity Rating Scale across strategy types. Autism. 2021;25:2101–11.
Alpern GD. (DP TM -4) Developmental Profile 4. 2020.
Flanagan HE, Smith IM, Davidson F. The Assessment of Phase of Preschool Language: Applying the language benchmarks framework to characterize language profiles and change in four- to five-year-olds with autism spectrum disorder: https://doi.org/10.1177/2396941519864084 . 2019;4:239694151986408.
Tager-Flusberg H, Rogers S, Cooper J, Landa R, Lord C, Paul R, et al. Defining Spoken Language Benchmarks and Selecting Measures of Expressive Language Development for Young Children With Autism Spectrum Disorders. J Speech Lang Hear Res. 2009;52:643–52.
Vineland Adaptive Behavior Scales | Third Edition. [cited 2022 Feb 7]. Available from: https://www.pearsonassessments.com/store/usassessments/en/Store/Professional-Assessments/Behavior/Adaptive/Vineland-Adaptive-Behavior-Scales-%7C-Third-Edition/p/100001622.html .
Brouwer WBF, Van Exel NJA, Van Gorp B, Redekop WK. The CarerQol instrument: A new instrument to measure care-related quality of life of informal caregivers for use in economic evaluations.
Varni J. Pediatric Quality of Life Inventory (PedsQL). 2022.
Katsiana A, Strimpakos N, Ioannis V, Kapreli E, Sofologi M, Bonti E, et al. Health-related Quality of Life in Children with Autism Spectrum Disorder and Children with Down Syndrome. Mater Sociomed. 2020 [cited 2024 Jul 28];32:93–8. Available from: https://pubmed.ncbi.nlm.nih.gov/32843854/ .
Lord C, GR, HA, KSH, MK, BK, CT, CC, & DS. Brief Observation of Social Communication Change (BOSCC). Copyright in progress. 2020.
Bailey DB, Raspa M, Olmsted MG, Novak SP, Sam AM, Humphreys BP, et al. Development and Psychometric Validation of the Family Outcomes Survey-Revised. 2011 [cited 2024 Jul 29];33:6–23. Available from: https://doi.org/10.1177/1053815111399441 .
King S, King G, Rosenbaum P. Evaluating Health Service Delivery to Children With Chronic Conditions and Their Families: Development of a Refined Measure of Processes of Care (MPOC−20). Children’s Health Care. 2004 [cited 2024 Jul 29];33:35–57. Available from: https://doi.org/10.1207/s15326888chc3301_3 .
Day TN, Mazefsky CA, Yu L, Zeglen KN, Neece CL, Pilkonis PA. The Emotion Dysregulation Inventory-Young Child: Psychometric Properties and Item Response Theory Calibration in 2- to 5-Year-Olds. J Am Acad Child Adolesc Psychiatry. 2024 [cited 2024 Jul 29];63:52–64. Available from: https://pubmed.ncbi.nlm.nih.gov/37422108/ .
Cohen IL, Schmidt-Lackner S, Romanczyk R, Sudhalter V. The PDD Behavior Inventory: A rating scale for assessing response to intervention in children with pervasive developmental disorder. J Autism Dev Disord. 2003;33:31–45.
Cohen IL. Criterion-related validity of the PDD Behavior Inventory. J Autism Dev Disord. 2003;33:47–53.
Cohen IL, Gomez TR, Gonzalez MG, Lennon EM, Karmel BZ, Gardner JM. Parent PDD behavior inventory profiles of young children classified according to autism diagnostic observation schedule-generic and autism diagnostic interview-revised criteria. J Autism Dev Disord. 2010;40:246–54.
Adamson LB, Bakeman R, Suma K, Nelson B, Rutherford PK©, Adamson B. The Joint Engagement Rating Inventory (JERI). 2020.
Adamson LB, Bakeman R. The Communication Play Protocol: Capturing Variations in Language Development. Perspect ASHA Spec Interest Groups. 2016;1:164–71.
McCullagh P, Nelder JA. Generalized Linear Models. 2nd ed. Chapman and Hall; 1989.
Stahmer AC, Suhrheinrich J, Rieth SR, Roesch S, Vejnoska S, Chan J, et al. A Waitlist Randomized Implementation Trial of Classroom Pivotal Response Teaching for Students with Autism. Focus on autism and other developmental disorders. 2022.
Weiner BJ, Lewis CC, Stanick C, Powell BJ, Dorsey CN, Clary AS, et al. Psychometric assessment of three newly developed implementation outcome measures. Implement Sci. 2017;12:1–12.
Lau A, Barnett M, Stadnick N, Saifan D, Regan J, Wiltsey Stirman S, et al. Therapist report of adaptations to delivery of evidence-based practices within a system-driven reform of publicly funded childrens mental health services. J Consult Clin Psychol. 2017;85:664–75.
Moullin JC, Sklar M, Ehrhart MG, Green A, Aarons GA. Provider REport of Sustainment Scale (PRESS): development and validation of a brief measure of inner context sustainment. Implementation Science. 2021 [cited 2022 Feb 6];16:1–10. Available from: https://doi.org/10.1186/s13012-021-01152-w .
Ruble LA, Toland MD, Birdwhistell JL, McGrew JH, Usher EL. Preliminary Study of the Autism Self-Efficacy Scale for Teachers (ASSET). Res Autism Spectr Disord. 2013;7:1151–9.
Ehrhart MG, Aarons GA, Farahnak LR. Assessing the organizational context for EBP implementation: the development and validity testing of the Implementation Climate Scale (ICS). Implement Sci. 2014;9:157.
Ehrhart MG, Aarons GA, Farahnak LR. Going above and beyond for implementation: the development and validity testing of the Implementation Citizenship Behavior Scale (ICBS). Implement Sci. 2015;10:65.
Gibbs A. Focus groups. Social Research Update. 1997;19.
Merton RK, Kendall PL. The focused interview. Am J Sociol. 1946;51:541–57.
Qualitative Data Analysis Software | NVivo. [cited 2022 Feb 9]. Available from: https://www.qsrinternational.com/nvivo-qualitative-data-analysis-software/home .
Hamilton AB, Finley EP. Qualitative methods in implementation research: An introduction. Psychiatry Res. 2019;280:112516.
Glaser B, Strauss A. The discovery of grounded theory: Strategies for qualitative research. Chicago: Aldine; 1967.
Palinkas LA, Aarons GA, Horwitz S, Chamberlain P, Hurlburt M, Landsverk J. Mixed method designs in implementation research. Adm Policy Ment Health. 2011;38:44–53.
Wells KB, Staunton A, Norris KC, Bluthenthal R, Chung B, Gelberg L, et al. Building an academic-community partnered network for clinical services research: the Community Health Improvement Collaborative (CHIC). Ethn Dis. 2006;16:S3-17.
Curran GM, Bauer M, Mittman B, Pyne JM, Stetler C. Med Care. 2012;50:217–26.
Wiltsey Stirman S, Miller CJ, Toder K, Calloway A. Development of a framework and coding system for modifications and adaptations of evidence-based interventions. [cited 2018 Jan 1]; Available from: http://www.implementationscience.com/content/8/1/65 .
Download references
Acknowledgements
We thank our community program collaborators Jocelyn Thompson and Melissa Willa for their input into the project processes and training protocol to ensure feasibility in community systems. Thank you to our community advisory committee for supporting development of the final measures and protocol to support neurodiversity affirming procedures. Thank you to the MIND ESDM training team who developed the intervention training protocol for community providers including Dr. Marie Rocha, Rachel Balitsky, and Vanessa Avilla-Pons. Thank you to Amanda Castillas, Walter Cervantes, Julia Sherman, and Dr. Sarah Vejnoska for supporting development of study protocol, training materials recruitment tools and assessment procedures. Special thanks to Deeniece Hatten for providing overall administrative management of the project and developing recruitment procedures to support the needs of diverse families.
This study includes a community advisory board who reviews study protocol, recruitment material and processes, study processes etc., and make recommendations to the research team to support culturally responsive and family-centered research protocol. This study includes a data safety and monitoring board who reviews study enrollment, procedures, protocol deviations and adverse events bi-annual and reports to the institutional review board and funder. A complete list of committee members and activities can be obtained from the PI.
This study is funded by the National Institute of Mental Health (NIMH; R01MH131703 and supported by the MIND Institute Intellectual and Developmental Disabilities Research Center (IDDRC) funded by the National Institute of Child Health and Human Development (NICHD; P50 HD103526). The protocol underwent peer review as part of the funding process. These funding sources were not involved in the design of this study and will not participate in its execution, analysis, data interpretation, or decision to submit the results.
Author information
Authors and affiliations.
UC Davis Health, MIND Institute, University of California, 2825 50th St., Sacramento, CA, 95819, USA
Aubyn C. Stahmer, Sarah Dufek & Sally J. Rogers
UC Davis Health, Department of Public Health Sciences, University of California, One Shields Ave., Davis, CA, 95616, USA
Ana-Maria Iosif
You can also search for this author in PubMed Google Scholar
Contributions
ACS, SD, and SJR conceived the study, participated in its design and coordination, and drafted the manuscript. AMI participated in the design of the study and developed the statistical analysis plan. All authors reviewed and edited the manuscript. All authors read and approved the final manuscript.
Corresponding author
Correspondence to Aubyn C. Stahmer .
Ethics declarations
Ethics approval and consent to participate.
This study was approved by the institutional review board at the University of California, Davis, protocol number 203076–2. Specific strategies for consent and confidentiality can be obtained from the PI upon request.
Consent for publication
Not applicable.
Competing interests
Aubyn Stahmer, Sarah Dufek and Ana-Maria Iosif declare no competing interests. Conflicts of interest for Sally Rogers involve royalties from publications, training programs, and lectures related to the Early Start Denver Model.
Additional information
Publisher’s note.
Springer Nature remains neutral with regard to jurisdictional claims in published maps and institutional affiliations.
Rights and permissions
Open Access This article is licensed under a Creative Commons Attribution 4.0 International License, which permits use, sharing, adaptation, distribution and reproduction in any medium or format, as long as you give appropriate credit to the original author(s) and the source, provide a link to the Creative Commons licence, and indicate if changes were made. The images or other third party material in this article are included in the article's Creative Commons licence, unless indicated otherwise in a credit line to the material. If material is not included in the article's Creative Commons licence and your intended use is not permitted by statutory regulation or exceeds the permitted use, you will need to obtain permission directly from the copyright holder. To view a copy of this licence, visit http://creativecommons.org/licenses/by/4.0/ .
Reprints and permissions
About this article
Cite this article.
Stahmer, A.C., Dufek, S., Rogers, S.J. et al. Study Protocol for a Cluster, Randomized, Controlled Community Effectiveness Trial of the Early Start Denver Model (ESDM) Compared to Community Early Behavioral Intervention (EBI) in Community Programs serving Young Autistic Children: Partnering for Autism: Learning more to improve Services (PALMS). BMC Psychol 12 , 513 (2024). https://doi.org/10.1186/s40359-024-02020-0
Download citation
Received : 06 August 2024
Accepted : 20 September 2024
Published : 28 September 2024
DOI : https://doi.org/10.1186/s40359-024-02020-0
Share this article
Anyone you share the following link with will be able to read this content:
Sorry, a shareable link is not currently available for this article.
Provided by the Springer Nature SharedIt content-sharing initiative
- Early intervention
- Early start Denver model
- Community implementation
BMC Psychology
ISSN: 2050-7283
- General enquiries: [email protected]
Dynamic Response Analyses and Experimental Research into Deep-Sea Mining Systems Based on Flexible Risers
- Research Article
- Published: 01 October 2024
Cite this article
- Jianyu Xiao 1 , 2 ,
- Zhuang Kang 1 ,
- Ming Chen 2 ,
- Yijun Shen 3 ,
- Yanlian Du 3 &
- Jing Leng 2
Explore all metrics
The deep seabed is known for its abundant reserves of various mineral resources. Notably, the Clarion Clipperton (C–C) mining area in the northeast Pacific Ocean, where China holds exploration rights, is particularly rich in deep-sea polymetallic nodules. These nodules, which are nodular and unevenly distributed in seafloor sediments, have significant industrial exploitation value. Over the decades, the deep-sea mining industry has increasingly adopted systems that combine rigid and flexible risers supported by large surface mining vessels. However, current systems face economic and structural stability challenges, hindering the development of deep-sea mining technology. This paper proposes a new structural design for a deep-sea mining system based on flexible risers, validated through numerical simulations and experimental research. The system composition, function and operational characteristics are comprehensively introduced. Detailed calculations determine the production capacity of the deep-sea mining system and the dimensions of the seabed mining subsystem. Finite element numerical simulations analyze the morphological changes of flexible risers and the stress conditions at key connection points under different ocean current incident angles. Experimental research verifies the feasibility of collaborative movement between two tethered underwater devices. The proposed deep-sea mining system, utilizing flexible risers, significantly advances the establishment of a commercial deep-sea mining system. The production calculations and parameter determinations provide essential references for the system’s future detailed design. Furthermore, the finite element simulation model established in this paper provides a research basis, and the method established in this paper offers a foundation for subsequent research under more complex ocean conditions. The control strategy for the collaborative movement between two tethered underwater devices provides an effective solution for deep-sea mining control systems.
This is a preview of subscription content, log in via an institution to check access.
Access this article
Subscribe and save.
- Get 10 units per month
- Download Article/Chapter or eBook
- 1 Unit = 1 Article or 1 Chapter
- Cancel anytime
Price includes VAT (Russian Federation)
Instant access to the full article PDF.
Rent this article via DeepDyve
Institutional subscriptions
Similar content being viewed by others
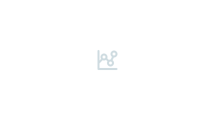
An Overview of Structural Design, Analysis and Common Monitoring Technologies for Floating Platform and Flexible Cable and Riser
Design of full-ocean-depth self-floating sampler and analysis of factors affecting core penetration depth.
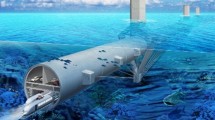
Feasibility Study of Submerged Floating Tunnels Moored by an Inclined Tendon System
Ahmad S, Datta TK (1989) Dynamic response of marine risers. Engineering Structures 11(3): 179–188. https://doi.org/10.1016/0141-0296(89)90005-9
Article Google Scholar
Amaechi CV, Chesterton C, Butler HO, Gillet N, Wang C, Ja’e IA, Reda A, Odijie AC (2022a) Review of composite marine risers for deep-water applications: Design, development and mechanics. Journal of Composites Science 6(3): 96. https://doi.org/10.3390/jcs6030096
Amaechi CV, Ja E IA, Reda A, Ju X (2022b) Scientometric review and thematic areas for the research trends on marine hoses. Energies 15(20): 7723. https://doi.org/10.3390/en15207723
Amaechi CV, Reda A, Ja’e IA, Wang C, An C (2022c) Guidelines on composite flexible risers: Monitoring techniques and design approaches. Energies 15(14): 4982. https://doi.org/10.3390/en15144982
Bath AR (1989) Deep sea mining technology: Recent developments and future projects. Offshore Technology Conference, Houston, Texas, 5998. https://doi.org/10.4043/5998-MS
Google Scholar
Bernard J, Bath A, Greger B (1987) Analysis and comparison of nodule hydraulic transport systems. Offshore Technology Conference, Houston, Texas, 5476. https://doi.org/10.4043/5476-MS
Bruyne KD, Stoffers H, Flamen S, Beuf HD, Taymans C, Smith S, Nijen KV (2022) A precautionary approach to developing nodule collector technology. Perspectives On Deep-Sea Mining 6: 137–165. https://doi.org/10.1007/978-3-030-87982-26
Burns JQ, Suh SL (1979) Design and analysis of hydraulic lift systems for deep ocean mining. Offshore Technology Conference, Houston, Texas, 3366. https://doi.org/10.4043/3366-MS
Chang YJ, Chen GM, Sun YY, Xu LB, Peng P (2008) Nonlinear dynamic analysis of deepwater drilling risers subjected to random loads. China Ocean Engineering. 22(4): 683–691
Chen XM (2006) Development of deep-sea mining technology in China (in Chinese). Mining Research and Development (S1): 40–48. https://doi.org/10.13827/j.cnki.kyyk.2006.s1.010
Chen YX, Yang N, Jin X (2014) Simulation of flexible multibody dynamics for deep-sea lifting system (in Chinese). Mining and Metallurgical Engineering 34(5): 6–9. https://doi.org/10.3969/j.issn.0253-6099.2014.05.002
Dai Y, Liu SJ (2013) Researches on deep ocean mining robots: status and development (in Chinese). Robot. 35(3): 363–375. https://doi.org/10.3724/SP.J.1218.2013.00363
Dai Y, Liu SJ (2012) Establishment of the dynamic model of the total deep ocean mining system and fast simulation of its integrated operation process. Journal of Mechanical Engineering 48(9): 79–88. https://doi.org/10.3901/JME.2012.09.079
Du XG, Guan LQ, Zhou WX (2016) Development status of deep-sea mining and demand analysis of deep-sea mining vessels in China (in Chinese). Straits Science 12: 62–67
Ge T, Zhu JM, Yao BH (2010) Distributed deep-sea local test mining system. C.N. Patent CN1807836 A
Guo F, Liu Y, Wu Y, Luo F (2018) Observer-based backstepping boundary control for a flexible riser system. Mechanical Systems & Signal Processing 111: 314–330. https://doi.org/10.1016/j.ymssp.2018.03.058
Han QJ, Liu SJ (2013) Dynamic Analysis and Path Tracking Control of Deep-sea Tracked Miner. Advanced Materials Research 753: 1161–1168. https://doi.org/10.4028/AMR.753-755.1161
Handschuh R, Grebe H, Panthel J, Schulte E, Ravindran M (2001) Innovative deep ocean mining concept based on flexible riser and self-propelled mining machines. The Fourth ISOPE Ocean Mining Symposium, Szczecin, Poland, 17
He W, Ge SS, How BVE, Choo YS, Hong KS (2011) Robust adaptive boundary control of a flexible marine riser with vessel dynamics. Automatica 47(4): 722–732. https://doi.org/10.1016/j.automatica.2011.01.064
Article MathSciNet Google Scholar
Heine OR, Suh SL (1978) An experimental nodule collection vehicle design and testing. The Offshore Technology Conference, Houston, Texas, 3138. https://doi.org/10.4043/3138-MS
Herrouin G, Lenoble J, Charles C, Mauviel F, Taine B (1989) A manganese nodule industrial venture would be profitable: Summary of a 4-year study in France. The Offshore Technology Conference, Houston, Texas, 5997. https://doi.org/10.4043/5997-MS
Huera-Huarte FJ, Bearman PW (2009) Wake structures and vortex-induced vibrations of a long flexible cylinder—part 1: Dynamic response. Journal of Fluids and Structures 25(6): 969–990. https://doi.org/10.1016/j.jfluidstructs.2009.03.007
Kaufman R, Latimer JP, Tolefson DC, Senni S (1985) The design and operation of a Pacific Ocean deep-ocean mining test ship: R/V Deepsea Minner 2. The Offshore Technology Conference, Houston, Texas, 4901. https://doi.org/10.4043/4901-MS
Kirk CL, Etok EU (1979) Wave induced random oscillations of pipelines during laying. Applied Ocean Research. 1(1): 51–60. https://doi.org/10.1016/0141-1187(79)90008-7
Kotlinski R, Stoyanova V, Hamrak H, Avramov A (2008) An overview of the interoceanmetal (IOM) deep-sea technology development (Mining and Processing). Proceedings of the Workshop on Polymetallic Nodule Mining Technology-Current Status and Challenges Ahead, Chennai, India, 18–22
Krolikowski LP, Gay TA (1980) An improved linearization technique for frequency domain riser analysis. The Offshore Technology Conference, Houston, Texas, 3777. https://doi.org/10.4043/3777-MS
Li JB, Wang YJ, Liu L, Xu XW (2022) Current status and prospect of deep-sea mining technology (in Chinese). Science and Technology Foresight 02(1): 92–102. https://doi.org/10.3981/j.issn.2097-0781.2022.02.007
Liu J, Mao JL, Liu BY, Ling S (2001) Dynamic analysis for the lateral movement of a lifting pipe in deep sea mining (in Chinese). Engineering Science 3(11): 74–79
Liu JH, Yang Q (2012) Analysis on lateral motion response of deep-sea mining riser (in Chinese). Mining & Processing Equipment 40(02): 5–9. https://doi.org/10.16816/j.cnki.ksjx.2012.02.002
Liu SJ, Liu C, Dai Y (2014) Status and progress on researches and developments of deep ocean mining equipments (in Chinese). Journal of Mechanical Engineering 02(50): 8–18. https://doi.org/10.3901/JME.2014.02.008
Masuda Y, Cruickshank MJ, Mero JL (1971) Continuous bucket-line dredging at 12, 000 feet. The Offshore Technology Conference, Houston, Texas, 1410. https://doi.org/10.4043/1410-MS
Morkookaza CK, Coelho FM, Shiguemoto DA, Franciss R, Matt C (2006) Dynamic behavior of a top tensioned riser in frequency and time domain. ISOPE International Ocean and Polar Engineering Conference, San Francisco, California, USA, 2: 31–36
Reda A, Elgazzar MA, Sultan IA, Shahin MA, McKee KK (2021) Failure analysis of articulated paddings at crossing interface between crossing cable and crossed pipeline. Applied Ocean Research. 115: 102850. https://doi.org/10.1016/j.apor.2021.102850
Reda A, Howard IM, Forbes GL, Sultan IA, McKee KK (2017) Design and installation of subsea cable, pipeline and umbilical crossing interfaces. Engineering Failure Analysis 81: 193–203. https://doi.org/10.1016/j.engfailanal.2017.07.001
Saito T, Usami T, Yamazaki T, Tomishima Y, Kiyono F (1989) Lifting characteristics of manganese nodules by air-lift-pump on 200m vertical test plant. Proceedings OCEANS, Seattle, 48–53. https://doi.org/10.1109/OCEANS.1989.592812
Chapter Google Scholar
Sterk R, Stein JK (2015) Seabed mineral resources: A review of current mineral resources and future developments. Deep Sea Mining Summit, Aberdeen, Scotland, 126: 9–10. https://doi.org/10.1016/j.oregeorev.2020.103699
Sup H, Hyung-Woo K, Jong-Su C, Tae-Kyung Y, Seong-Jae P (2008) A way to accomplish the mining technology for polymetallic nodules. Proceedings of the Workshop on Polymetallic Nodule Mining Technology-Current Status and Challenges Ahead, Chennai, India, 18–22
Tang DS, Yang N, Jin X (2013) Hydraulic lifting technique with vertical pipe for deep-sea coarse mineral particles (in Chinese), Mining and Metallurgical Engineering 33(5): 1–8. https://doi.org/10.3969/j.issn.0253-6099.2013.05.001
Wang YJ, Yang N, Jin X (2011) Current situation and developing trend of exploitation and utilization technology of deep-sea mineral resources (in Chinese). Proceedings of 2011 China Mining Technology Conference, Xi’an, Shaanxi, China, 11–14
Welling CG (1981) An advanced design deep sea mining system. The Offshore Technology Conference, Houston, Texas, 4094. https://doi.org/10.4043/4094-MS
William T, Bin TL, Jaiman RK, Tay TE, Tan VBC (2018) A comprehensive study on composite risers: Material solution, local end fitting design and global response. Marine Structures 61: 155–169. https://doi.org/10.1016/j.marstruc.2018.05.005
Xiao JY, Sup H, Yang N, Xiong H, Liu J, Ou W, Parianos J, Chen YX (2019) Model test of skip lifting system for deep-sea mining. The 29th International Ocean and Polar Engineering Conference, Honolulu, Hawaii, USA, 296
Xiao YX, Yang LB, Cao L, Wang ZW (2014) Distribution of marine mineral resource and advances of deep-sea lifting pump technology. Journal of Drainage and Irrigation Machinery Engineering 32(4): 319–326. https://doi.org/10.3969/j.issn.1674-8530.13.1064
Yang N, Chen GG (2010) Status quo and development tendency of deep-sea minerals mining technology (in Chinese). Mining & Processing Equipment 1:12–18. https://doi.org/10.16816/j.cnki.ksjx.2010.10.002
Yang N, Xia JX (2000) Development techniques for international sea-floor resources and their future trend (in Chinese). Mining and Metallurgical Engineering 1(20): 1–4
Yoon GS, Park JH, Lee SH, Park JH, Jeong YN (2005) Collecting and lifting methods of manganese nodule and mining device. K. R. Patent WO/2005/093215
Yu Y, Wang CJ, Liu DH (2023) Norwegian deep sea resource exploitation strategic analysis and suggestion for China (in Chinese). Ocean Development and Management 40(1): 3–11. https://doi.org/10.20016/j.cnki.hykfygl.20230202.001
Zou L, Sun JZ, Sun Z (2023) Research status and prospect of core technology of deep-sea mineral resources exploitation in China. Journal of Harbin Engineering University 5(44): 708–716
Download references
Funding Supported by Finance Science and Technology Project of Hainan Province under Grant No. ZDKJ2021027 and the National Natural Science Foundation of China under Grant No. 52231012.
Author information
Authors and affiliations.
College of Shipbuilding Engineering, Harbin Engineering University, Harbin, 150000, China
Jianyu Xiao & Zhuang Kang
Deep Sea Engineering Division, Institute of Deep-sea Science and Engineering, Chinese Academy of Sciences, Sanya, 572000, China
Jianyu Xiao, Ming Chen & Jing Leng
State Key Laboratory of Marine Resources Utilization in South China Sea, Hainan University, Haikou, 570228, China
Yijun Shen & Yanlian Du
You can also search for this author in PubMed Google Scholar
Corresponding author
Correspondence to Zhuang Kang .
Ethics declarations
Competing interest The authors have no competing interests to declare that are relevant to the content of this article.
Additional information
Article highlights.
• To address the weak economic and structural stability of the existing systems, a new structural form of deep-sea mining system based on flexible risers has been proposed.
• Detailed calculations have been performed to study the production capacity and dimension parameters of this deep-sea mining system based on flexible risers.
• A finite element simulation model has been developed to analyze the morphological changes of flexible risers and the stress conditions at key connection points under different ocean current incident angles.
• An experimental test of collaborative movement was conducted in a 20-m-deep water tank, verifying its feasibility and proposing an effective control strategy.
Rights and permissions
Reprints and permissions
About this article
Xiao, J., Kang, Z., Chen, M. et al. Dynamic Response Analyses and Experimental Research into Deep-Sea Mining Systems Based on Flexible Risers. J. Marine. Sci. Appl. (2024). https://doi.org/10.1007/s11804-024-00491-6
Download citation
Received : 07 March 2024
Accepted : 25 April 2024
Published : 01 October 2024
DOI : https://doi.org/10.1007/s11804-024-00491-6
Share this article
Anyone you share the following link with will be able to read this content:
Sorry, a shareable link is not currently available for this article.
Provided by the Springer Nature SharedIt content-sharing initiative
- Deep-sea mining
- Flexible riser
- Underwater mineral resources
- Dynamic response analyses
- Collaborative movement
- Find a journal
- Publish with us
- Track your research
Module 1: Lifespan Development
Developmental research designs, learning outcomes.
- Compare advantages and disadvantages of developmental research designs (cross-sectional, longitudinal, and sequential)
Now you know about some tools used to conduct research about human development. Remember, research methods are tools that are used to collect information. But it is easy to confuse research methods and research design. Research design is the strategy or blueprint for deciding how to collect and analyze information. Research design dictates which methods are used and how. Developmental research designs are techniques used particularly in lifespan development research. When we are trying to describe development and change, the research designs become especially important because we are interested in what changes and what stays the same with age. These techniques try to examine how age, cohort, gender, and social class impact development.
Cross-sectional designs
The majority of developmental studies use cross-sectional designs because they are less time-consuming and less expensive than other developmental designs. Cross-sectional research designs are used to examine behavior in participants of different ages who are tested at the same point in time. Let’s suppose that researchers are interested in the relationship between intelligence and aging. They might have a hypothesis (an educated guess, based on theory or observations) that intelligence declines as people get older. The researchers might choose to give a certain intelligence test to individuals who are 20 years old, individuals who are 50 years old, and individuals who are 80 years old at the same time and compare the data from each age group. This research is cross-sectional in design because the researchers plan to examine the intelligence scores of individuals of different ages within the same study at the same time; they are taking a “cross-section” of people at one point in time. Let’s say that the comparisons find that the 80-year-old adults score lower on the intelligence test than the 50-year-old adults, and the 50-year-old adults score lower on the intelligence test than the 20-year-old adults. Based on these data, the researchers might conclude that individuals become less intelligent as they get older. Would that be a valid (accurate) interpretation of the results?
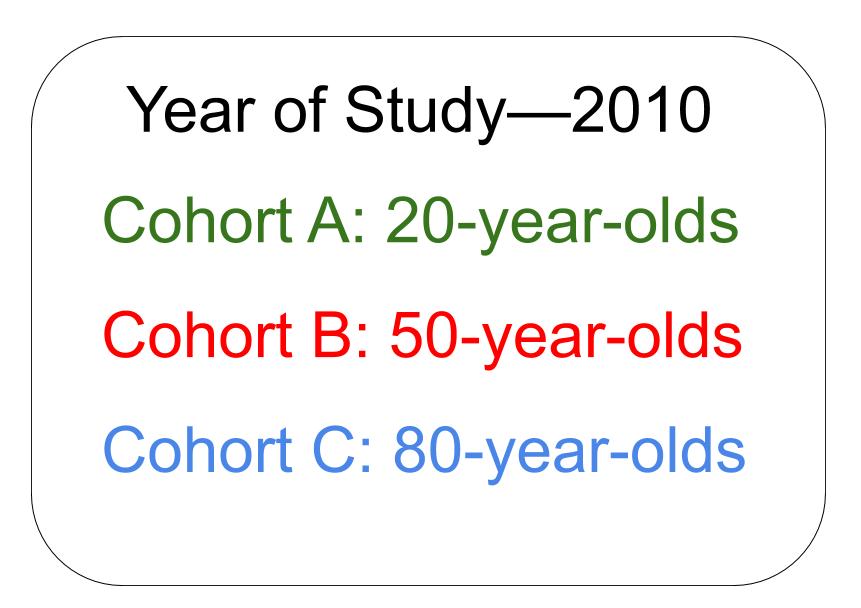
Figure 1 . Example of cross-sectional research design
No, that would not be a valid conclusion because the researchers did not follow individuals as they aged from 20 to 50 to 80 years old. One of the primary limitations of cross-sectional research is that the results yield information about age differences not necessarily changes with age or over time. That is, although the study described above can show that in 2010, the 80-year-olds scored lower on the intelligence test than the 50-year-olds, and the 50-year-olds scored lower on the intelligence test than the 20-year-olds, the data used to come up with this conclusion were collected from different individuals (or groups of individuals). It could be, for instance, that when these 20-year-olds get older (50 and eventually 80), they will still score just as high on the intelligence test as they did at age 20. In a similar way, maybe the 80-year-olds would have scored relatively low on the intelligence test even at ages 50 and 20; the researchers don’t know for certain because they did not follow the same individuals as they got older.
It is also possible that the differences found between the age groups are not due to age, per se, but due to cohort effects. The 80-year-olds in this 2010 research grew up during a particular time and experienced certain events as a group. They were born in 1930 and are part of the Traditional or Silent Generation. The 50-year-olds were born in 1960 and are members of the Baby Boomer cohort. The 20-year-olds were born in 1990 and are part of the Millennial or Gen Y Generation. What kinds of things did each of these cohorts experience that the others did not experience or at least not in the same ways?
You may have come up with many differences between these cohorts’ experiences, such as living through certain wars, political and social movements, economic conditions, advances in technology, changes in health and nutrition standards, etc. There may be particular cohort differences that could especially influence their performance on intelligence tests, such as education level and use of computers. That is, many of those born in 1930 probably did not complete high school; those born in 1960 may have high school degrees, on average, but the majority did not attain college degrees; the young adults are probably current college students. And this is not even considering additional factors such as gender, race, or socioeconomic status. The young adults are used to taking tests on computers, but the members of the other two cohorts did not grow up with computers and may not be as comfortable if the intelligence test is administered on computers. These factors could have been a factor in the research results.
Another disadvantage of cross-sectional research is that it is limited to one time of measurement. Data are collected at one point in time and it’s possible that something could have happened in that year in history that affected all of the participants, although possibly each cohort may have been affected differently. Just think about the mindsets of participants in research that was conducted in the United States right after the terrorist attacks on September 11, 2001.
Longitudinal research designs
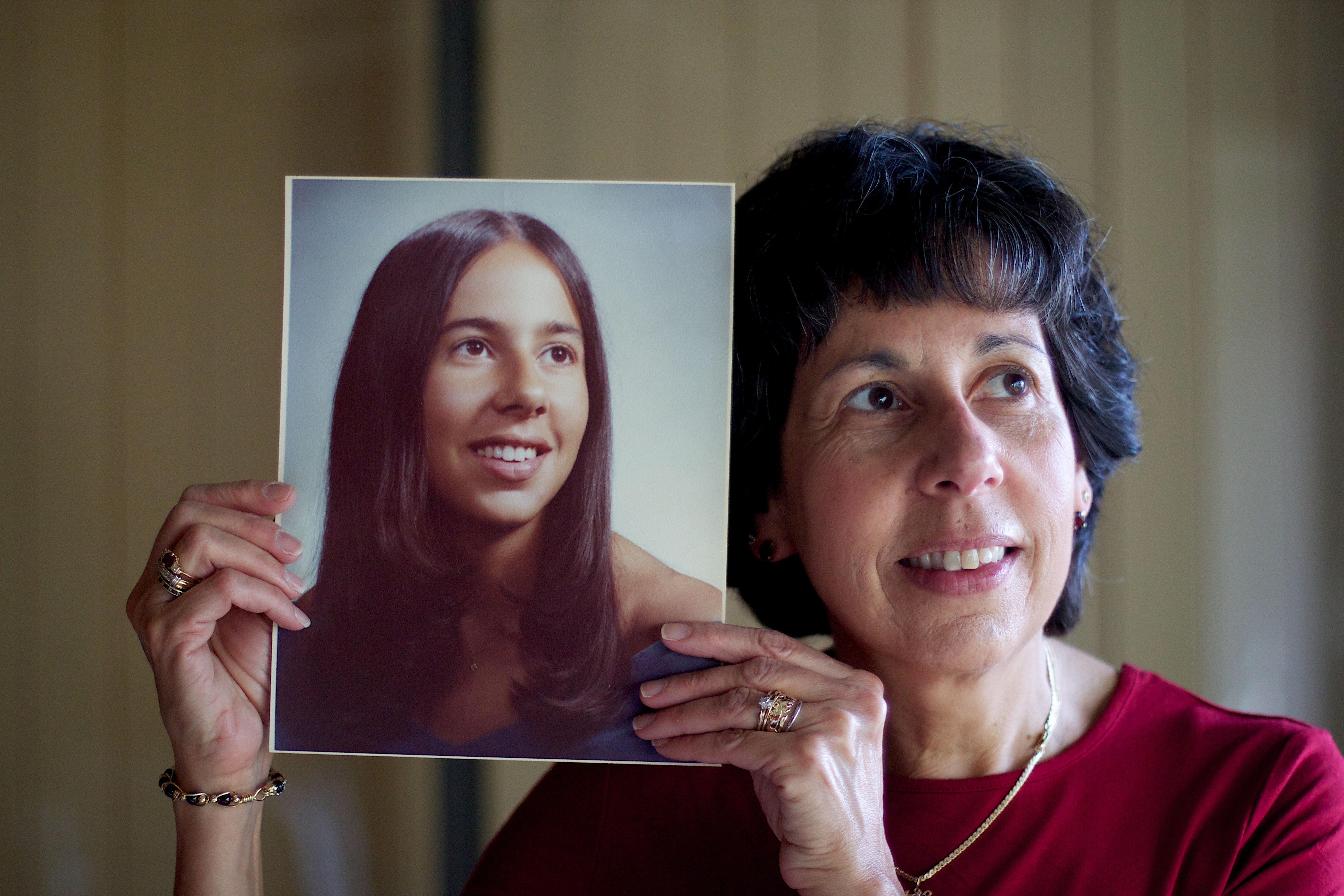
Figure 2 . Longitudinal research studies the same person or group of people over an extended period of time.
Longitudinal research involves beginning with a group of people who may be of the same age and background (cohort) and measuring them repeatedly over a long period of time. One of the benefits of this type of research is that people can be followed through time and be compared with themselves when they were younger; therefore changes with age over time are measured. What would be the advantages and disadvantages of longitudinal research? Problems with this type of research include being expensive, taking a long time, and participants dropping out over time. Think about the film, 63 Up , part of the Up Series mentioned earlier, which is an example of following individuals over time. In the videos, filmed every seven years, you see how people change physically, emotionally, and socially through time; and some remain the same in certain ways, too. But many of the participants really disliked being part of the project and repeatedly threatened to quit; one disappeared for several years; another died before her 63rd year. Would you want to be interviewed every seven years? Would you want to have it made public for all to watch?
Longitudinal research designs are used to examine behavior in the same individuals over time. For instance, with our example of studying intelligence and aging, a researcher might conduct a longitudinal study to examine whether 20-year-olds become less intelligent with age over time. To this end, a researcher might give an intelligence test to individuals when they are 20 years old, again when they are 50 years old, and then again when they are 80 years old. This study is longitudinal in nature because the researcher plans to study the same individuals as they age. Based on these data, the pattern of intelligence and age might look different than from the cross-sectional research; it might be found that participants’ intelligence scores are higher at age 50 than at age 20 and then remain stable or decline a little by age 80. How can that be when cross-sectional research revealed declines in intelligence with age?

Figure 3 . Example of a longitudinal research design
Since longitudinal research happens over a period of time (which could be short term, as in months, but is often longer, as in years), there is a risk of attrition. Attrition occurs when participants fail to complete all portions of a study. Participants may move, change their phone numbers, die, or simply become disinterested in participating over time. Researchers should account for the possibility of attrition by enrolling a larger sample into their study initially, as some participants will likely drop out over time. There is also something known as selective attrition— this means that certain groups of individuals may tend to drop out. It is often the least healthy, least educated, and lower socioeconomic participants who tend to drop out over time. That means that the remaining participants may no longer be representative of the whole population, as they are, in general, healthier, better educated, and have more money. This could be a factor in why our hypothetical research found a more optimistic picture of intelligence and aging as the years went by. What can researchers do about selective attrition? At each time of testing, they could randomly recruit more participants from the same cohort as the original members, to replace those who have dropped out.
The results from longitudinal studies may also be impacted by repeated assessments. Consider how well you would do on a math test if you were given the exact same exam every day for a week. Your performance would likely improve over time, not necessarily because you developed better math abilities, but because you were continuously practicing the same math problems. This phenomenon is known as a practice effect. Practice effects occur when participants become better at a task over time because they have done it again and again (not due to natural psychological development). So our participants may have become familiar with the intelligence test each time (and with the computerized testing administration).
Another limitation of longitudinal research is that the data are limited to only one cohort. As an example, think about how comfortable the participants in the 2010 cohort of 20-year-olds are with computers. Since only one cohort is being studied, there is no way to know if findings would be different from other cohorts. In addition, changes that are found as individuals age over time could be due to age or to time of measurement effects. That is, the participants are tested at different periods in history, so the variables of age and time of measurement could be confounded (mixed up). For example, what if there is a major shift in workplace training and education between 2020 and 2040 and many of the participants experience a lot more formal education in adulthood, which positively impacts their intelligence scores in 2040? Researchers wouldn’t know if the intelligence scores increased due to growing older or due to a more educated workforce over time between measurements.
Sequential research designs
Sequential research designs include elements of both longitudinal and cross-sectional research designs. Similar to longitudinal designs, sequential research features participants who are followed over time; similar to cross-sectional designs, sequential research includes participants of different ages. This research design is also distinct from those that have been discussed previously in that individuals of different ages are enrolled into a study at various points in time to examine age-related changes, development within the same individuals as they age, and to account for the possibility of cohort and/or time of measurement effects. In 1965, K. Warner Schaie [1] (a leading theorist and researcher on intelligence and aging), described particular sequential designs: cross-sequential, cohort sequential, and time-sequential. The differences between them depended on which variables were focused on for analyses of the data (data could be viewed in terms of multiple cross-sectional designs or multiple longitudinal designs or multiple cohort designs). Ideally, by comparing results from the different types of analyses, the effects of age, cohort, and time in history could be separated out.
Consider, once again, our example of intelligence and aging. In a study with a sequential design, a researcher might recruit three separate groups of participants (Groups A, B, and C). Group A would be recruited when they are 20 years old in 2010 and would be tested again when they are 50 and 80 years old in 2040 and 2070, respectively (similar in design to the longitudinal study described previously). Group B would be recruited when they are 20 years old in 2040 and would be tested again when they are 50 years old in 2070. Group C would be recruited when they are 20 years old in 2070 and so on.
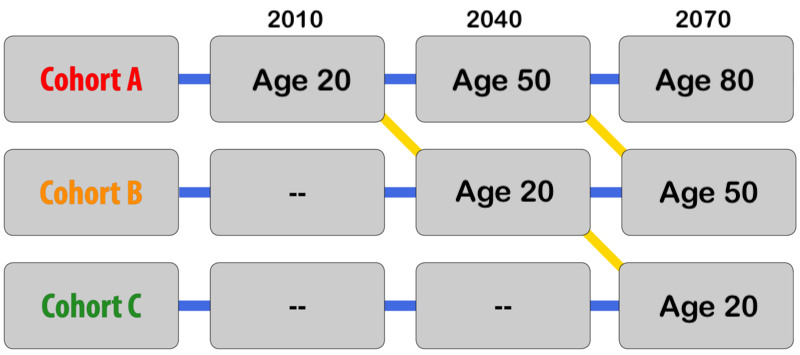
Figure 4. Example of sequential research design
Studies with sequential designs are powerful because they allow for both longitudinal and cross-sectional comparisons—changes and/or stability with age over time can be measured and compared with differences between age and cohort groups. This research design also allows for the examination of cohort and time of measurement effects. For example, the researcher could examine the intelligence scores of 20-year-olds in different times in history and different cohorts (follow the yellow diagonal lines in figure 3). This might be examined by researchers who are interested in sociocultural and historical changes (because we know that lifespan development is multidisciplinary). One way of looking at the usefulness of the various developmental research designs was described by Schaie and Baltes (1975) [2] : cross-sectional and longitudinal designs might reveal change patterns while sequential designs might identify developmental origins for the observed change patterns.
Since they include elements of longitudinal and cross-sectional designs, sequential research has many of the same strengths and limitations as these other approaches. For example, sequential work may require less time and effort than longitudinal research (if data are collected more frequently than over the 30-year spans in our example) but more time and effort than cross-sectional research. Although practice effects may be an issue if participants are asked to complete the same tasks or assessments over time, attrition may be less problematic than what is commonly experienced in longitudinal research since participants may not have to remain involved in the study for such a long period of time.
When considering the best research design to use in their research, scientists think about their main research question and the best way to come up with an answer. A table of advantages and disadvantages for each of the described research designs is provided here to help you as you consider what sorts of studies would be best conducted using each of these different approaches.
Research Design | Advantages | Disadvantages |
---|---|---|
Cross-Sectional | ||
Longitudinal | ||
Sequential |
- Schaie, K.W. (1965). A general model for the study of developmental problems. Psychological Bulletin, 64(2), 92-107. ↵
- Schaie, K.W. & Baltes, B.P. (1975). On sequential strategies in developmental research: Description or Explanation. Human Development, 18: 384-390. ↵
- Modification, adaptation, and original content. Authored by : Margaret Clark-Plaskie for Lumen Learning. Provided by : Lumen Learning. License : CC BY-NC-SA: Attribution-NonCommercial-ShareAlike
- Research Methods in Developmental Psychology. Authored by : Angela Lukowski and Helen Milojevich. Provided by : University of Calfornia, Irvine. Located at : https://nobaproject.com/modules/research-methods-in-developmental-psychology?r=LDcyNTg0 . Project : The Noba Project. License : CC BY-NC-SA: Attribution-NonCommercial-ShareAlike
- Woman holding own photograph. Provided by : Pxhere. Located at : https://pxhere.com/en/photo/221167 . License : CC0: No Rights Reserved
Information
- Author Services
Initiatives
You are accessing a machine-readable page. In order to be human-readable, please install an RSS reader.
All articles published by MDPI are made immediately available worldwide under an open access license. No special permission is required to reuse all or part of the article published by MDPI, including figures and tables. For articles published under an open access Creative Common CC BY license, any part of the article may be reused without permission provided that the original article is clearly cited. For more information, please refer to https://www.mdpi.com/openaccess .
Feature papers represent the most advanced research with significant potential for high impact in the field. A Feature Paper should be a substantial original Article that involves several techniques or approaches, provides an outlook for future research directions and describes possible research applications.
Feature papers are submitted upon individual invitation or recommendation by the scientific editors and must receive positive feedback from the reviewers.
Editor’s Choice articles are based on recommendations by the scientific editors of MDPI journals from around the world. Editors select a small number of articles recently published in the journal that they believe will be particularly interesting to readers, or important in the respective research area. The aim is to provide a snapshot of some of the most exciting work published in the various research areas of the journal.
Original Submission Date Received: .
- Active Journals
- Find a Journal
- Journal Proposal
- Proceedings Series
- For Authors
- For Reviewers
- For Editors
- For Librarians
- For Publishers
- For Societies
- For Conference Organizers
- Open Access Policy
- Institutional Open Access Program
- Special Issues Guidelines
- Editorial Process
- Research and Publication Ethics
- Article Processing Charges
- Testimonials
- Preprints.org
- SciProfiles
- Encyclopedia
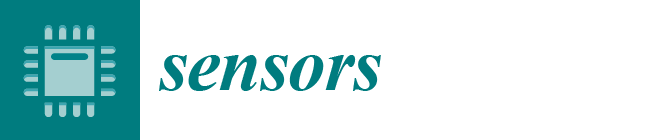
Article Menu

- Subscribe SciFeed
- Recommended Articles
- Google Scholar
- on Google Scholar
- Table of Contents
Find support for a specific problem in the support section of our website.
Please let us know what you think of our products and services.
Visit our dedicated information section to learn more about MDPI.
JSmol Viewer
Design and experimental test of rope-driven force sensing flexible gripper.
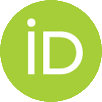
1. Introduction
2. structure design and prototype development, 3. force sensing strategy, 3.1. relationship matrix, 3.2. determination of contact force, 4. experimental study, 4.1. experimental system, 4.2. results analysis, 5. conclusions, author contributions, institutional review board statement, informed consent statement, data availability statement, conflicts of interest.
- Zhao, Y.X.; Wan, X.F.; Duo, H.X. Review of rigid fruit and vegetable picking robots. Int. J. Agr. Biol. Eng. 2023 , 16 , 1–11. [ Google Scholar ] [ CrossRef ]
- Wang, Z.H.; Xun, Y.; Wang, Y.K.; Yang, Q.H. Review of smart robots for fruit and vegetable picking in agriculture. Int. J. Agric. Biol. Eng. 2022 , 15 , 33–54. [ Google Scholar ] [ CrossRef ]
- Navas, E.; Shamshiri, R.R.; Dworak, V.; Weltzien, C.; Fernandez, R. Soft gripper for small fruits harvesting and pick and place operations. Front. Robot. AI 2024 , 10 , 1330496. [ Google Scholar ] [ CrossRef ]
- Chen, M.Y.; Chen, Z.X.; Luo, L.F.; Tang, Y.C.; Cheng, J.B.; Wei, H.L.; Wang, J.H. Dynamic visual servo control methods for continuous operation of a fruit harvesting robot working throughout an orchard. Comput. Electron. Agric. 2024 , 219 , 108774. [ Google Scholar ] [ CrossRef ]
- Chen, X.Y.; Sun, Y.L.; Zhang, Q.J.; Dai, X.S.; Tian, S.; Guo, Y.X. Three-dimension object detection and forward-looking control strategy for non-destructive grasp of thin-skinned fruits. Appl. Soft. Comput. 2024 , 150 , 111082. [ Google Scholar ] [ CrossRef ]
- Tang, Y.C.; Chen, M.Y.; Wang, C.L.; Luo, L.F.; Li, J.H.; Lian, G.P.; Zou, X.J. Recognition and Localization Methods for Vision-Based Fruit Picking Robots: A Review. Front. Plant. Sci. 2020 , 11 , 510. [ Google Scholar ] [ CrossRef ]
- Wei, Y.Y.; Cai, L.H.; Fang, H.F.; Chen, H.Y. Fruit recognition and classification based on tactile information of flexible hand. Sens. Actuators A-Phys. 2024 , 370 , 115224. [ Google Scholar ] [ CrossRef ]
- He, Z.Y.; Lian, B.B.; Song, Y.M. Rigid-Soft Coupled Robotic Gripper for Adaptable Grasping. J. Bionic. Eng. 2023 , 20 , 2601–2618. [ Google Scholar ] [ CrossRef ]
- Zhu, Y.L.; Wang, T.; Gong, W.Z.; Feng, K.; Wang, X.; Xi, S. Design and motion analysis of soft robotic arm with pneumatic-network structure. Smart Mater. Struct. 2024 , 33 , 095038. [ Google Scholar ] [ CrossRef ]
- Tang, Z.L.; Xu, L.J.; Wang, Y.C.; Kang, Z.L.; Xie, H. Collision-Free Motion Planning of a Six-Link Manipulator Used in a Citrus Picking Robot. Appl. Sci. 2021 , 11 , 11336. [ Google Scholar ] [ CrossRef ]
- Deng, L.X.; Liu, T.Y.; Jiang, P.; Qi, A.L.; He, Y.C.; Li, Y.J.; Yang, M.Q.; Deng, X. Design and Testing of Bionic-Feature-Based 3D-Printed Flexible End-Effectors for Picking Horn Peppers. Agronomy 2023 , 13 , 2231. [ Google Scholar ] [ CrossRef ]
- Pi, J.; Liu, J.; Zhou, K.H.; Qian, M.Y. An Octopus-Inspired Bionic Flexible Gripper for Apple Grasping. Agriculture 2021 , 11 , 1014. [ Google Scholar ] [ CrossRef ]
- Liu, J.; Chen, Z.J.; Wen, G.L.; He, J.F.; Wang, H.X.; Xue, L.; Long, K.; Xie, Y.M. Origami Chomper-Based Flexible Gripper with Superior Gripping Performances. Adv. Intell. 2023 , 5 , 2300238. [ Google Scholar ] [ CrossRef ]
- Wang, R.G.; Li, X.P.; Huang, H.B. Design of thick panels origami-inspired flexible grasper with anti-interference ability. Mech. Mach. Theory 2023 , 189 , 105431. [ Google Scholar ] [ CrossRef ]
- Li, H.L.; Yao, J.T.; Wei, C.J.; Zhou, P.; Xu, Y.D.; Zhao, Y.S. An untethered soft robotic gripper with high payload-to-weight ratio. Mech. Mach. Theory 2021 , 158 , 104226. [ Google Scholar ] [ CrossRef ]
- Liu, P.B.; Qian, J.W.; Yan, P.; Liu, Y. Design, analysis and evaluation of a self-lockable constant-force compliant gripper. Sens. Actuators A-Phys. 2022 , 335 , 113354. [ Google Scholar ] [ CrossRef ]
- Hao, T.Z.; Xiao, H.P.; Liu, Y.B.; Pang, D.Z.; Liu, S.H. Gripping performance of soft grippers with fingerprint-like surface texture for objects with slippery surfaces. Tribol. Int. 2023 , 189 , 108992. [ Google Scholar ] [ CrossRef ]
- Wang, B.Z.; Yu, T.T. Numerical investigation of novel 3D-SPA for gripping analysis in multi-environment. Int. J. Mech. Sci. 2023 , 240 , 107916. [ Google Scholar ] [ CrossRef ]
- Fan, Q.; Miao, J.L.; Tian, M.W.; Zhao, H.T.; Zhu, S.F.; Liu, X.H.; Ma, Y.L.; Qu, L.J. Low-voltage driven flexible double-layer electrothermal actuator for smart human-machine interactions. Sens. Actuators A-Phys. 2020 , 315 , 112352. [ Google Scholar ] [ CrossRef ]
- Ji, H.; Zhang, L.; Nie, S.; Huo, L.F.; Nie, S.L.; Wu, Z.W. Optimization design and experiment study on a water hydraulic flexible actuator integrating flexible inner skeleton and soft external skin used for underwater flexible gripper. Sens. Actuators A-Phys. 2024 , 366 , 114957. [ Google Scholar ] [ CrossRef ]
- Nie, S.L.; Huo, L.F.; Ji, H.; Lan, Y.; Wu, Z.W. Bending deformation characteristics of high-pressure soft actuator driven by water-hydraulics for underwater manipulator. Sens. Actuators A-Phys. 2022 , 344 , 113736. [ Google Scholar ] [ CrossRef ]
- Jin, L.Y.; Wang, Z.P.; Tian, S.J.; Feng, J.T.; An, C.Q.; Xu, H.R. Grasping perception and prediction model of kiwifruit firmness based on flexible sensing claw. Comput. Electron. Agric. 2023 , 215 , 108389. [ Google Scholar ] [ CrossRef ]
- Chen, Y.; Guo, S.F.; Li, C.F.; Yang, H.; Hao, L.N. Size recognition and adaptive grasping using an integration of actuating and sensing soft pneumatic gripper. Robot. Auton. Syst. 2018 , 104 , 14–24. [ Google Scholar ] [ CrossRef ]
- Ren, J.; Wu, F.; Shang, E.R.; Li, D.Y.; Liu, Y. 3D printed smart elastomeric foam with force sensing and its integration with robotic gripper. Sens. Actuators A-Phys. 2023 , 349 , 113998. [ Google Scholar ] [ CrossRef ]
- Dilibal, S.; Sahin, H.; Danquah, J.O.; Emon, M.O.F.; Choi, J.W. Additively Manufactured Custom Soft Gripper with Embedded Soft Force Sensors for an Industrial Robot. Int. J. Precis. Eng. Manuf. 2021 , 22 , 709–718. [ Google Scholar ] [ CrossRef ]
- Xu, J.S.; Xie, Z.J.; Yue, H.H.; Lu, Y.F.; Yang, F. A triboelectric multifunctional sensor based on the controlled buckling structure for motion monitoring and bionic tactile of soft robots. Nano Energy 2022 , 104 Pt A , 107845. [ Google Scholar ] [ CrossRef ]
- Sun, Z.D.; Zhu, M.L.; Zhang, Z.X.; Chen, Z.C.; Shi, Q.F.; Shan, X.C.; Yeow, R.C.H.; Lee, C.K. Artificial Intelligence of Things (AIoT) Enabled Virtual Shop Applications Using Self-Powered Sensor Enhanced Soft Robotic Manipulator. Adv. Sci. 2021 , 8 , 14. [ Google Scholar ] [ CrossRef ]
- Wang, H.J.; Zhou, C.; Li, H.Z.; Chen, J.M.; Yu, P.; Chen, C.G.; Zhang, Y.Z. A soft robot tactile fingertip for grasping surface posture detection. Smart Mater. Struct. 2024 , 33 , 065014. [ Google Scholar ] [ CrossRef ]
- Lan, C.C.; Lin, C.M.; Fan, C.H. A Self-Sensing Microgripper Module With Wide Handling Ranges. IEEE-ASME Trans. Mechatron. 2011 , 16 , 141–150. [ Google Scholar ] [ CrossRef ]
- Wang, W.; Tang, Y.X.; Li, C. Controlling bending deformation of a shape memory alloy-based soft planar gripper to grip deformable objects. Int. J. Mech. Sci. 2021 , 193 , 106181. [ Google Scholar ] [ CrossRef ]
- Xie, Y.X.; Zhang, B.H.; Zhou, J.; Bai, Y.H.; Zhang, M. An Integrated Multi-Sensor Network for Adaptive Grasping of Fragile Fruits: Design and Feasibility Tests. Sensors 2020 , 20 , 4973. [ Google Scholar ] [ CrossRef ] [ PubMed ]
- Wang, Y.S.; Wu, H.Y.; Zhu, Z.K.; Ye, Y.K.; Qian, M.B. Continuous picking of yellow peaches with recognition and collision-free path. Comput. Electron. Agric. 2023 , 214 , 108273. [ Google Scholar ] [ CrossRef ]
- Wang, C.L.; Li, C.J.; Han, Q.Y.; Wu, F.Y.; Zou, X.J.; Zhang, B.H. A Performance Analysis of a Litchi Picking Robot System for Actively Removing Obstructions, Using an Artificial Intelligence Algorithm. Agronomy 2023 , 13 , 2795. [ Google Scholar ] [ CrossRef ]
- Zhang, Z.; Zhou, J.; Yi, B.Y.; Zhang, B.H.; Wang, K. A flexible swallowing gripper for harvesting apples and its grasping force sensing model. Comput. Electron. Agric. 2023 , 204 , 107489. [ Google Scholar ] [ CrossRef ]
- Xu, W.F.; Zhang, H.; Yuan, H.; Liang, B. A Compliant Adaptive Gripper and Its Intrinsic Force Sensing Method. IEEE Trans. Robot. 2021 , 37 , 1584–1603. [ Google Scholar ] [ CrossRef ]
- Yao, J.Q.; Fang, Y.F.; Yang, X.H.; Wang, P.Y.; Li, L.Q. Design optimization of soft robotic fingers biologically inspired by the fin ray effect with intrinsic force sensing. Mech. Mach. Theory 2024 , 191 , 105472. [ Google Scholar ] [ CrossRef ]
- Shan, X.W.; Birglen, L. Modeling and analysis of soft robotic fingers using the fin ray effect. Int. J. Robot. Res. 2020 , 39 , 1686–1705. [ Google Scholar ] [ CrossRef ]
Click here to enlarge figure
The statements, opinions and data contained in all publications are solely those of the individual author(s) and contributor(s) and not of MDPI and/or the editor(s). MDPI and/or the editor(s) disclaim responsibility for any injury to people or property resulting from any ideas, methods, instructions or products referred to in the content. |
Share and Cite
Zhu, Z.; Liu, Y.; Ju, J.; Lu, E. Design and Experimental Test of Rope-Driven Force Sensing Flexible Gripper. Sensors 2024 , 24 , 6407. https://doi.org/10.3390/s24196407
Zhu Z, Liu Y, Ju J, Lu E. Design and Experimental Test of Rope-Driven Force Sensing Flexible Gripper. Sensors . 2024; 24(19):6407. https://doi.org/10.3390/s24196407
Zhu, Zuhao, Yufei Liu, Jinyong Ju, and En Lu. 2024. "Design and Experimental Test of Rope-Driven Force Sensing Flexible Gripper" Sensors 24, no. 19: 6407. https://doi.org/10.3390/s24196407
Article Metrics
Article access statistics, further information, mdpi initiatives, follow mdpi.
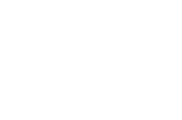
Subscribe to receive issue release notifications and newsletters from MDPI journals

COMMENTS
Lifespan development is a fascinating field of study - but care must be taken to ensure that researchers use appropriate methods to examine human behavior, use the correct experimental design to answer their questions, and be aware of the special challenges that are part-and-parcel of developmental research.
Sometimes, especially in developmental research, the researcher is interested in examining changes over time and will need to consider a research design that will capture these changes. Remember, research methods are tools that are used to collect information, while r esearch design is the strategy or blueprint for deciding how to collect and ...
Research design dictates which methods are used and how. Developmental research designs are techniques used particularly in lifespan development research. When we are trying to describe development and change, the research designs become especially important because we are interested in what changes and what stays the same with age.
Video 2.11 Introduction to Experimental Design introduces fundamental elements for experimental research design. Independent and Dependent Variables. In a research experiment, we strive to study whether changes in one thing cause changes in another. To achieve this, we must pay attention to two important variables, or things that can be changed ...
Sometimes, especially in developmental research, the researcher is interested in examining changes over time and will need to consider a research design that will capture these changes. Remember, research methods are tools that are used to collect information, while r esearch design is the strategy or blueprint for deciding how to collect and ...
Experimental research design is a framework of protocols and procedures created to conduct experimental research with a scientific approach using two sets of variables. Herein, the first set of variables acts as a constant, used to measure the differences of the second set. ... President of the EASE, Development Editor of Reproductive ...
There are many different developmental psychology research methods, including cross-sectional, longitudinal, correlational, and experimental. Each has its own specific advantages and disadvantages. The one that a scientist chooses depends largely on the aim of the study and the nature of the phenomenon being studied.
A key component in all research designs, variables can be broadly conceptualized as being in two different classes: predictor, or independent, variables (often represented as X) and criterion, or dependent, variables (often represented as Y).Independent variables are what researchers either control or use to categorize their subjects, and dependent variables are the measurements taken to ...
In experimental design research, these discussions are nascent and form a major reason for the development of this book. Core to this endeavour is the realisation that experimental design research concerns human beings and thus faces a set of challenges not fully reflected by discussions of experimentation in the natural sciences (Radder 2003 ...
Guide to Experimental Design | Overview, 5 steps & Examples. Published on December 3, 2019 by Rebecca Bevans.Revised on June 21, 2023. Experiments are used to study causal relationships.You manipulate one or more independent variables and measure their effect on one or more dependent variables.. Experimental design create a set of procedures to systematically test a hypothesis.
This stage of pre-experimentation research design consists of three steps: one-shot case study, one-group pre and post-tests, and static-group comparison (Figure 7).
The design of research is fraught with complicated and crucial decisions. Researchers must decide which research questions to address, which theoretical perspective will guide the research, how to measure key constructs reliably and accurately, who or what to sample and observe, how many people/places/things need to be sampled in order to achieve adequate statistical power, and which data ...
Remember, research methods are tools that are used to collect information, while research design is the strategy or blueprint for deciding how to collect and analyze information. Research design dictates which methods are used and how. There are three types of developmental research designs: cross-sectional, longitudinal, and sequential.
Developmental designs are techniques used in life span research (and other areas as well). These techniques try to examine how age, cohort, gender, and social class impact development. Cross-sectional research involves beginning with a sample that represents a cross-section of the population. Respondents who vary in age, gender, ethnicity, and ...
PDF | Chapter to appear in Handbook of Research Methods in Developmental Science (2nd edition). (edited by D. Teti, B. Cleveland, and K. Rulison) | Find, read and cite all the research you need on ...
The two main kinds of developmental research designs are cross-sectional study and longitudinal study. ... Experimental research allows the researcher to manipulate one or more variables to ...
developmental research where the object of such research is clearly not simply knowledge, but knowledge that practitioners can use. ... design, development, and formative evaluation of several prototypes, and summative evaluation. ... Typically, literature reviews in experimental studies concentrate
Qualitative research designs tend to be more flexible and inductive, allowing you to adjust your approach based on what you find throughout the research process.. Qualitative research example If you want to generate new ideas for online teaching strategies, a qualitative approach would make the most sense. You can use this type of research to explore exactly what teachers and students struggle ...
When to use Experimental Research Design . Experimental research design should be used when a researcher wants to establish a cause-and-effect relationship between variables. It is particularly useful when studying the impact of an intervention or treatment on a particular outcome. Here are some situations where experimental research design may ...
The rising number of children identified with autism has led to exponential growth in for-profit applied behavior analysis (ABA) agencies and the use of highly structured approaches that may not be developmentally appropriate for young children. Multiple clinical trials support naturalistic developmental behavior interventions (NDBIs) that integrate ABA and developmental science and are ...
However, current systems face economic and structural stability challenges, hindering the development of deep-sea mining technology. This paper proposes a new structural design for a deep-sea mining system based on flexible risers, validated through numerical simulations and experimental research.
Sometimes, especially in developmental research, the researcher is interested in examining changes over time and will need to consider a research design that will capture these changes. Remember, research methods are tools that are used to collect information, while r esearch design is the strategy or blueprint for deciding how to collect and ...
Developmental research designs are techniques used particularly in lifespan development research. When we are trying to describe development and change, the research designs become especially important because we are interested in what changes and what stays the same with age. These techniques try to examine how age, cohort, gender, and social ...
Robotic grasping is a common operation scenario in industry and agriculture, in which the force sensing function is a significant factor to achieve reliable grasping. Existing force sensing methods of flexible grippers require intelligent materials or force sensors embedded in the flexible gripper, which causes such problems of higher manufacturing requirements and contact surface properties ...