G-STA-PHD - Statistical Science - PhD
Degree designation.
The Department of Statistical Science at Duke University offers graduate study leading to PhD and MS degrees in statistical science. The PhD program offers thorough preparation in the theory and methods of statistics, with major emphases on modern, model-based statistical science, Bayesian and classical approaches to inference, computational statistics, and machine learning. A hallmark of the program is the integration of interdisciplinary applications into teaching and research, reflecting the department’s broad and deep engagements in leadership and innovation in statistical science and its intersections with many other areas, including the biomedical sciences, computational sciences, data and information sciences, economic and policy sciences, environmental sciences, engineering, machine learning, physical sciences, and social sciences. The rich opportunities for students in interdisciplinary statistical research at Duke are complemented by opportunities for engagement in research in summer projects with nonprofit agencies, industry, and academia.
For an up-to-date faculty list and description of graduate programs in statistical science visit the website at stat.duke.edu .

Bulletin Links
- Divinity School
- Fuqua School of Business
- Graduate School
- School of Law
- School of Medicine
- Nicholas School of the Environment
- School of Nursing
- Pratt School of Engineering
- Sanford School of Public Policy
- Undergraduate Instruction
- Archived Bulletins
2023-2024 Graduate School Bulletin. Download PDF here.
Following the people and events that make up the research community at Duke

What does it take to be a successful PhD student? Two grad students in statistics weigh in
By Meghna Datta
On May 10, 2023
In Mathematics , Statistics , Students
With so many different career options in life, how do you know that you’ve found the right one for you?
Graduate students Edric Tam and Andrew McCormack , when asked what they hope to be doing in ten years, said they’d choose to do exactly the kind of work they’re doing right now – so clearly, they’ve found the right path. Tam, who obtained his undergraduate degree in Biomedical Engineering , Neuroscience and Applied Mathematics from Johns Hopkins University, and McCormack, who came from the University of Toronto with a degree in Statistics, are now 5 th -year PhD students in the Department of Statistical Sciences. Tam works with Professor David Dunson , while McCormack works with Professor Peter Hoff , and both hope to pursue research careers in statistics.

Research interests
For the past five years, McCormack has been doing more theoretical research, looking at how geometry can lend insights into statistical models. The example he gives is of the Fisher information matrix, a statistical model that many undergraduate statistics majors learn in their third or fourth year.
Tam, meanwhile, looks at data with unique graphical and connectivity structures that aren’t quite linear or easily modeled, such as a brain connectome or a social network. In doing so, he works on answering two questions – how can you model data like this, and how can you leverage the unique structure of the data in the process?
What both Tam and McCormack like about the field of statistics is that, as Tam puts it, “you get to play in everyone’s backyard.” Moreover, as McCormack says, the beauty of theoretical research is that, while it’s certainly more time-consuming and incremental, it is often timeless, giving insight into something previously unknown.
On walking the research path
What does it take to be a successful PhD student? Both McCormack and Tam agree that a PhD is just a degree – anyone can get one if you work hard. But what sustains both through a career in research is a passion for what they do. Tam says that “you need inherent motivation, curiosity, passion, and drive.” McCormack adds that it helps if you work on problems that are interesting to you.
Tam, who spent some time in a biomedical engineering lab during his undergraduate years, remembers reading about math and statistics the entire time he was there, which signaled to him that maybe, biomedical engineering wasn’t for him. McCormack’s defining moment occurred in the proof-based classes he took while as an undergraduate. He initially wanted to pursue a career in finance, but he quickly became enamored by the elegant precision of mathematical proofs – “even if all you’re proving is that 1+1=2!”
“You need inherent motivation, curiosity, passion, and drive.” Edric tam, on what it takes to pursue a career in research
Even with passion for what you do, however, research can have its ups and downs. McCormack describes the rollercoaster of coming up with a new idea, convinced that “this is a paper right here”, and then a day later, after he’s had time to think about the idea, realizing that it isn’t quite up to the mark. Tam, who considers himself a pretty laidback person, sometimes finds the Type A personalities in research, as in any career field, too intense. Both McCormack and Tam prefer to not take themselves too seriously, and both exude a love for – and a trust of – the process.

Reflections on the past and the future
Upon graduation, McCormack will move to Germany to pursue a post-doc before beginning a job as Assistant Professor in Statistics at the University of Alberta. Tam will continue his research at Duke before applying to post-doc programs. In reflecting on their paths that have brought them till now, both feel content with the journey they’ve taken.
Tam sees the future in front of him – from PhD to post-doc to professorship – as “just a change in the title, with more responsibility”, and is excited to embark on his post-doc, where he gets to continue to do the research he loves. “It doesn’t get much better than this,” he laughs, and McCormack agrees. When McCormack joins the faculty at the University of Alberta, he’s looking forward to mentoring students in a much larger capacity, although he comments that the job will probably be challenging and he’s expecting to feel a little bit of imposter syndrome as he settles in.
When asked for parting thoughts, both Tam and McCormack emphasize that the best time to get into statistics and machine learning is right now. The advent of ChatGPT , for example, could replace jobs and transform education. But given their love for the field, this recommendation isn’t surprising. As Tam succinctly puts it, “given a choice between doing math and going out with friends, I would do math – unless that friend is Andy!”

When Art and Science Meet as Equals
Shifting from social comparison to “social savoring” seems to help.
Powered by WordPress & Theme by Anders Norén
Traditional features of the curriculum include parallel development of theory and applications as well as coverage of specific biostatistical topic areas and ethical issues in the conduct of statistical and medical research. The core curriculum covers the principles of epidemiologic studies in detail. Embedded throughout the curriculum are examples of conflict of interest situations faced by biostatisticians, along with principles of reproducible research and strategies for implementation.
The PhD program follows the Duke Graduate School Academic Calendar .
View the timeline for students with and without an Applicable Quantitative Master's Degree.
For students with a Master's degree in Biostatistics, some of the required 700 level courses listed below may be waived if they have taken those courses or their equivalents previously.
Required Knowledge in the Following Core Courses
This course provides a formal introduction to the basic theory and methods of probability and statistics. It covers topics in probability theory with an emphasis on those needed in statistics, including probability and sample spaces, independence, conditional probability, random variables, parametric families of distributions, and sampling distributions. Core concepts are mastered through mathematical exploration and linkage with the applied concepts studied in BIOSTAT 704. Prerequisite(s): 2 semesters of calculus or its equivalent (multivariate calculus preferred). Familiarity with linear algebras is helpful. Corequisite(s): BIOSTAT 702, BIOSTAT 703. Credits: 3
This course provides an introduction to study design, descriptive statistics, and analysis of statistical models with one or two predictor variables. Topics include principles of study design, basic study designs, descriptive statistics, sampling, contingency tables, one- and two-way analysis of variance, simple linear regression, and analysis of covariance. Both parametric and non-parametric techniques are explored. Core concepts are mastered through team-based case studies and analysis of authentic research problems encountered by program faculty and demonstrated in practicum experiences in concert with BIOSTAT 703. Computational exercises will use the R and SAS packages. Prerequisite(s): 2 semesters of calculus or its equivalent (multivariate calculus preferred). Familiarity with linear algebras is helpful. Corequisites(s): BIOSTAT 701, BIOSTAT 703, BIOSTAT 721. Credits: 3
This course provides an introduction to biology at a level suitable for practicing biostatisticians and directed practice in techniques of statistical collaboration and communication. With an emphasis on the connection between biomedical content and statistical approach, this course helps unify the statistical concepts and applications learned in BIOSTAT 701 and BIOSTAT 702. In addition to didactic sessions on biomedical issues, students are introduced to different areas of biostatistical practice at Duke University Medical Center. Biomedical topics are organized around the fundamental mechanisms of disease from both evolutionary and mechanistic perspectives, illustrated using examples from infectious disease, cancer and chronic /degenerative disease. In addition, students learn how to read and interpret research and clinical trial papers. Core concepts and skills are mastered through individual reading and class discussion of selected biomedical papers, team-based case studies and practical sessions introducing the art of collaborative statistics. Corequisite(s): BIOSTAT 701, BIOSTAT 702. Credits: 3
The lab will be an extension of the course. The lab will be run like a journal club. The lab will instruct students how to dissect a research article from a statistical and scientific perspective. The lab will also give students the opportunity to present on material covered in the co-requisite course and to practice the communication skills that are a core tenant of the program. Corequisite(s): BIOSTAT 703 or permission of the Director of Graduate Studies. Credits: 0
This course provides formal introduction to the basic theory and methods of probability and statistics. It covers topics in statistical inference, including classical and Bayesian methods, and statistical models for discrete, continuous and categorical outcomes. Core concepts are mastered through mathematical exploration, simulations, and linkage with the applied concepts studied in BIOSTAT 705. Prerequisite(s): BIOSTAT 701 or its equivalent. Corequisite(s): BIOSTAT 705, BIOSTAT 706. Credits: 3
This course provides an introduction to general linear models and the concept of experimental designs. Topics include linear regression models, analysis of variance, mixed-effects models, generalized linear models (GLM) including binary, multinomial responses and log-linear models, basic models for survival analysis and regression models for censored survival data, and model assessment, validation and prediction. Core concepts are mastered through statistical methods application and analysis of practical research problems encountered by program faculty and demonstrated in practicum experiences in concert with BIOSTAT 706. Computational examples and exercises will use the SAS and R packages. Prerequisite(s): BIOSTAT 702 or its equivalent. Corequisite(s): BIOSTAT 704, BIOSTAT 706, BIOSTAT 722. Credits: 3
This course revisits the topics covered in BIOSTAT 703 in the context of high-throughput, high-dimensional studies such as genomics and transcriptomics. The course will be based on reading of both the textbook and research papers. Students will learn the biology and technology underlying the generation of “big data,” and the computational and statistical challenges associated with the analysis of such data sets. As with BIOSTAT 703, there will be strong emphasis on the development of communication skills via written and oral presentations. Prerequisite(s): BIOSTAT 703. Corequisite(s): BIOSTAT 704, BIOSTAT 705. Credits: 3
Introduction to concepts and techniques used in the analysis of time to event data, including censoring, hazard rates, estimation of survival curves, regression techniques, applications to clinical trials. Interval censoring, informative censoring, competing risks, multiple events and multiple endpoints, time dependent covariates; nonparametric and semi-parametric methods. Prerequisite(s): BIOSTAT 701, 702, 704, 705, and 721 or 722 or their equivalents, or permission of the Director of Graduate Studies. Credits: 3
Topics include linear and nonlinear mixed models; generalized estimating equations; subject specific versus population average interpretation; and hierarchical model. Prerequisite(s): BIOSTAT 701, 702, 704, 705, and 721 or 722 or their equivalents, or permission of the Director of Graduate Studies. Credits: 3
The class introduces the concept of exponential family of distributions and link function, and their use in generalizing the standard linear regression to accommodate various outcome types. Theoretical framework will be presented but detailed practical analyses will be performed as well, including logistic regression and Poisson regression with extensions. Majority of the course will deal with the independent observations framework. However, there will be substantial discussion of longitudinal/clustered data where correlations within clusters are expected. To deal with such data the Generalized Estimating Equations and the Generalized Linear Mixed models will be introduced. An introduction to a Bayesian analysis approach will be presented, time permitting.Prerequisite(s): BIOSTAT 701, 702, 704, 705, and 721 or 722 or their equivalents, or permission of the Director of Graduate Studies. Credits: 3
Advanced seminar on topics at the research frontiers in biostatistics. Readings of current biostatistical research and presentations by faculty and advanced students of current research in their area of specialization. Instructor: O’Brien. 1 unit.
Introduction to linear models and linear inference from the coordinate-free viewpoint. Topics: identifiability and estimability, key properties of and results for finite-dimensional vector spaces, linear transformations, self-adjoint transformations, spectral theorem, properties and geometry of orthogonal projectors, Cochran's theorem, estimation and inference for normal models, distributional properties of quadratic forms, minimum variance linear unbiased estimation, Gauss-Markov theorem and estimation, calculus of differentials, analysis of variance and covariance. Prerequisite: Biostatistics 906. Instructor: Shi . 3 units.
Introduce decision theory and optimality criteria, sufficiency, methods for point estimation, confidence interval and hypothesis testing methods and theory. Prerequisite: Biostatistics 704 or equivalent. Instructor consent required. Instructor: Fang . 3 units.
Students gain a holistic view of what skills they need to be successful graduate students and academic scholars. The curriculum focuses on the unique challenges of PhD students and the tools needed for long-term success in academia or industry. Instructor: Coutts. 1 unit.
The theory for M- and Z- estimators and applications. Semiparametric models, geometry of efficient score functions and efficient influence functions, construction of semiparametric efficient estimators. Introduction to the bootstrap: consistency, inconsistency and remedy, correction for bias, and double bootstrap. U statistics and rank and permutation tests. Prerequisites: Statistical Sciences 711 and Biostatistics 906. Instructor: Li. 3 units.
The goal of this course is to provide motivated Ph.D. and master’s students with background knowledge of high-dimensional statistics/machine learning for their research, especially in their methodology and theory development. Discussions cover theory, methodology, and applications. Selected topics in this course include the basics of high-dimensional statistics, matrix and tensor modeling, concentration inequity, nonconvex optimization, applications in genomics, and biomedical informatics. Prerequisite: Knowledge in probability, inference, and basic algebra are required. Instructor: Zhang, 3 units
Students gain a holistic view of career choices and how to structure a targeted job search. The curriculum focuses on exploring different career options in academia or industry, communicating unique value to employers, and organizing a job search. May be repeated with the consent of the advisor and the Director of Graduate Studies. Instructor: Coutts. 1 unit.
This course provides an introduction to measure theoretic foundations of probability theory focusing on properties of random variables, modes of convergence and their relationships, stochastic order, law of large numbers, central limit theorems, classical expectation and concentration inequalities, and characteristic functions and conditional expectations. Students seeking a more abstract or advanced coverage of concepts introduced in this course are encouraged to register for MATH631 (Measure and Integration) or MATH641 (Probability). Instructor: Owzar. 3 units.
Introduction to probability spaces, the theory of measure and integration, random variables, and limit theorems. Distribution functions, densities, and characteristic functions; convergence of random variables and of their distributions; uniform integrability and the Lebesgue convergence theorems. Weak and strong laws of large numbers, central limit theorem. Prerequisite: elementary real analysis and elementary probability theory. Instructor: Staff. 3 units.
Elective Courses
This course surveys a number of techniques for high dimensional data analysis useful for data mining, machine learning and genomic applications, among others. Topics include principal and independent component analysis, multidimensional scaling, tree-based classifiers, clustering techniques, support vector machines and networks, and techniques for model validation. Core concepts are mastered through the analysis and interpretation of several actual high dimensional genomics datasets. Prerequisite(s): BIOSTAT 701, 702, 704, 705, and 721 or 722 or their equivalents, or permission of the Director of Graduate Studies. Credits: 3
Topics include: history/background and process for clinical trial, key concepts for good statistics practice (GSP)/good clinical practice (GCP), regulatory requirement for pharmaceutical/clinical development, basic considerations for clinical trials, designs for clinical trials, classification of clinical trials, power analysis for sample size calculation, statistical analysis for efficacy evaluation, statistical analysis for safety assessment, implementation of a clinical protocol, statistical analysis plan, data safety monitoring, adaptive design methods in clinical trials (general concepts, group sequential design, dose finding design, and phase I/II or phase II/III seamless design) and controversial issues in clinical trials. Prerequisite(s): BIOSTAT 701, 702, 704, 705, and 721 or 722 or their equivalents, or permission of the Director of Graduate Studies. Credits: 3
Methods for causal inference, including confounding and selection bias in observational or quasi-experimental research designs, propensity score methodology, instrumental variables, and methods for non-compliance in randomized clinical trials. Prerequisite(s): BIOSTAT 701, 702, 704, 705, and 721 or 722 or their equivalents, or permission of the Director of Graduate Studies. Credits: 3
Topics from current and classical methods for assessing familiality and heritability, linkage analysis of Mendelian and complex traits, family-based and population-based association studies, genetic heterogeneity, epistasis, and gene-environmental interactions. Computational methods and applications in current research areas. The course will include a simple overview of genetic data, terminology, and essential population genetic results. Topics will include sampling designs in human genetics, gene frequency estimation, segregation analysis, linkage analysis, tests of association, and detection of errors in genetic data. Prerequisite(s): BIOSTAT 701, 702, 704, 705, and 721 or 722 or their equivalents, or permission of the Director of Graduate Studies. Credits: 3
Theory and application of missing data methodology, ad hoc methods, missing data mechanism, selection models, pattern mixture models, likelihood-based methods, multiple imputation, inverse probability weighting, sensitivity analysis. Prerequisites: Statistical Science 711, 721, and 732, or consent of instructor. Instructor: Allen. 3 units.
Designed for PhD students in Biostatistics or DSS departments who may be interested in conducting methodological research in the area of Survival Data Analysis. Applications of counting process and martingale theory to right censored survival data. Applications of empirical process theory to more general and possibly more complex statistical models using nonparametric analysis of interval-censored data as illustrating examples. After completion, students are anticipated to understand the statistical method papers on survival analysis appearing in top tier statistical journals. Prerequisites: BIOSTAT 701, 704, and 713, or equivalent, or consent of instructor. Instructor: Wu. 3 units.
Introduction to diverse statistical design and analytical methods for randomized phase II clinical trials. Topics: Minimax, optimal, and admissible clinical trials Inference methods for phase II clinical trials; clinical trials with a survival endpoint; clinical trials with heterogeneous patient populations; and randomized phase II clinical trials. Instructor consent required. Instructor: Jung. 3 units.
Faculty directed statistical methodology research. Instructor consent required. Instructor: O’Brien. 1 unit.
Student gains practical experience by taking an internship in industry/government and writes a report about this experience. Requires prior consent from the student's advisor and from the Director of Graduate Studies. May be repeated with consent of the advisor and the Director of Graduate Studies. Credit/no credit grading only. Instructor: O’Brien. 1 unit.
This course provides an introduction to the basic theory and application of empirical processes. Topics include: concepts of stochastic processes, Brownian motion and Brownian bridge process, stochastic integrals, weak convergence of sequences of random elements, convergence of empirical distribution functions, general Glivenko-Cantelli theorems and Donsker theorems, functional Delta method. An emphasis is put on applications in various biostatistical problems. Pre-requisites: Stat 711. Instructor: Li. Units: 3
Introduction to probabilistic graphical models and structured prediction, with applications in genetics and genomics. Hidden Markov Models, conditional random fields, stochastic grammars, Bayesian hierarchical models, neural networks, and approaches to integrative modeling. Algorithms for exact and approximate inference. Applications in DNA/RNA analysis, phylogenetics, sequence alignment, gene expression, allelic phasing and imputation, genome/epigenome annotation, and gene regulation. Department consent required. Instructor: Majoros. 3 units. C-L: Computational Biology and Bioinformatics 914.
Introduction to concepts in robabilistic machine learning with a focus on discriminative and hierarchical generative models. Topics include directed and undirected graphical models, kernel methods, exact and approximate parameter estimation methods, and structure learning. Prerequisites: Linear algebra, Statistical Science 250 or Statistical Science 611. Instructor: Heller, Mukherjee, or Reeves. 3 units.
Principles of data analysis and modern statistical modeling. Exploratory data analysis. Introduction to Bayesian inference, prior and posterior distributions, predictive distributions, hierarchical models, model checking and selection, missing data, introduction to stochastic simulation by Markov Chain Monte Carlo using a higher level statistical language such as R or Matlab. Applications drawn from various disciplines. Not open to students with credit for Statistical Science 360. Prerequisite: Statistical Science 210, 230 and 250, or close equivalents. Instructor: Clyde, Dunson, Reiter, or Volfovsky. 3 units.
Statistical issues in causality and methods for estimating causal effects. Randomized designs and alternative designs and methods for when randomization is infeasible: matching methods, propensity scores, longitudinal treatments, regression discontinuity, instrumental variables, and principal stratification. Methods are motivated by examples from social sciences, policy and health sciences. Instructor: Li or VolfovskyStatistical issues in causality and methods for estimating causal effects. Randomized designs and alternative designs and methods for when randomization is infeasible: matching methods, propensity scores, longitudinal treatments, regression discontinuity, instrumental variables, and principal stratification. Methods are motivated by examples from social sciences, policy and health sciences. Instructor: Li or Volfovsky
Statistical modeling and machine learning involving large data sets and challenging computation. Data pipelines and data bases, big data tools, sequential algorithms and subsampling methods for massive data sets, efficient programming for multi-core and cluster machines, including topics drawn from GPU programming, cloud computing, Map/Reduce and general tools of distributed computing environments. Intense use of statistical and data manipulation software will be required. Data from areas such as astronomy, genomics, finance, social media, networks, neuroscience. Instructor consent required. Prerequisites: Statistics 521L, 523L; Statistics 531, 532 (or co-registration). (3 units)

Home >> Courses
Current Courses | Teaching Resources | Previous Semesters
Course Links
- Spring, 2012
- Summer, 2011
- Spring, 2011
- Spring, 2010
- Spring, 2009
- Spring, 2008
- Spring, 2007
For information, questions, or comments about Undergraduate courses, please email [email protected] ; for information about the Graduate program, email [email protected] .
- Current course homepages
- STORM interface to student administration system
- Available seats for current courses
- Duke course listings , etc.
- Teaching Resources
- Student Resources
- Duke Statistics courses from previous semesters
- Duke Statistics Undergraduate Course Descriptions
- Duke Statistics Graduate Course Descriptions
- Duke Math Department
- NC State Statistics
- UNC Biostats
- UNC Statistics
Course Renumbering Information
Course Schedule & Course Descriptions
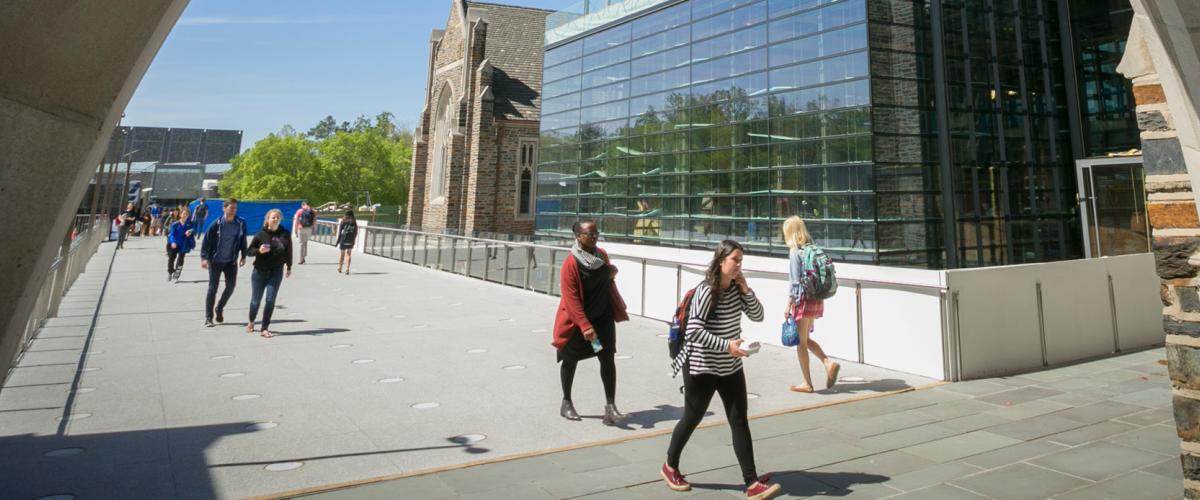
In their first two years, students in the Duke PhD in Population Health Sciences program take classes in population health, statistical methods and programming, research methods, and more. In the remaining years, students focus their efforts on their dissertation.
Year 1 |
|
|
---|---|---|
SAS Programming Primer (optional -- online, self-paced) | ||
POPHS 901: Population Health Sciences Theories (3 credits) | POPHS 910: Fundamentals of Qualitative Measurement (3 credits) | |
POPHS 905: Population Health Research Methods and Systematic Literature Reviews (3 credits) | POPHS 912: Population Health Sciences Professional Development II (1 credit) | |
POPHS 907: Population Health Sciences Professional Development I (1 credit) | POPHS 921: Analytic Methods II: Causal Inference (3 credits) | |
POPHS 920: Analytic Methods I (3 credits) | POPHS 923: Principles of Health Measurement (3 credits) | |
BIOTRAIN 750: Introduction to RCR Concepts (1-day event during orientation prior to classes) (4 RCR credits) | BIOTRAIN 751: The Responsible Scientist I (4 RCR credits) |
|
|
|
---|---|---|
POPHS 903: Analytic Methods III: Advanced Regression Methods (3 credits) | POPHS 904: Analytic Methods IV: Non-randomized designs (3 credits) | |
*BIOTRAIN 753 can be completed during either year 2 or year 3* | BIOTRAIN 753: Data Management and Quality for Biomedical PhD Students (self-paced, online) (2 RCR credits) | POPHS 930: Grant Writing in Population Health Sciences (2 credits) |
Elective (3 credits) | Elective (3 credits) | |
|
|
|
---|---|---|
Dissertation (3 credits) | Dissertation (3 credits) | |
BIOTRAIN 754: The Responsible Scientist II (4 RCR credits) | ||
Year 4 |
|
|
---|---|---|
Dissertation (3 credits) | Dissertation (3 credits) | |
Year 5 (if applicable) |
|
|
---|---|---|
Dissertation (3 credits) | Dissertation (3 credits) | |
(2 RCR credit hours each) |
Course Descriptions
Please note: Course instructors and descriptions are subject to change.
POPHS 901: Population Health Science Theories & Evidence-Based Approaches Instructor: Jennifer Gierisch
This class will describe and discuss the theories and application of theories to address population health problems. This will include theories from multiple disciplines including health behavior and education, medicine, implementation, and related fields. Emphasis on research related to social determinants of health and strategies and techniques used by health professionals to improve the health of populations.
POPHS 903: Analytic Methods III: Instructors: Megan Shepherd-Banigan & Brystana Kaufman
In this 2-semester course we will study the econometric tools which are used to support causal inference and apply these tools to real world data and draw causal conclusions based on those data. At the end of this course, students will be able to apply modern econometric methods to population health and health policy questions and to evaluate the approach and conclusions of econometric studies. Methods will address analyzing non-linear regression models and longitudinal and panel data. The 2-semseter course will culminate in a paper through which students will independently apply methods learned during the year to observational data to address a problem of their choice in population health
POPHS 905: Population Health Research Design and Systematic Literature Review Instructor: Connor Drake
This course explores the nature and process of scientific inquiry in the field of population health. Specifically, the course will establish a foundation for methodically identifying, exploring, and evaluating literature relevant to students areas of research interest.
POPHS 907: Population Health Sciences Professional Development I Instructor: Hayden Bosworth
The seminar will engage presenters from the Duke School of Medicine and University to address resources and professional development activities; leading multidisciplinary research teams, best practices for presenting and publishing research, career opportunities including building an academic CV and preparing for a career in population health.
POPHS 920: Analytic Methods I: Design, Data, and Descriptive Statistics Instructor: Emily O'Brien
This course will address designing observational and interventional studies with attention to defining, identifying, and describing cohorts using a variety of data sources. This course will address forms of bias, right-censoring of data, and describing risks. Special emphasis will be placed on data originating from real-world settings, such as electronic health records and claim data.
POPHS 904: Analytic Methods IV Instructors: Megan Shepherd Banigan & Brystana Kaufman This course is a continuation of POPHS 903. Students will study the econometric tools which are used to support causal inference and apply these tools to real world data and draw causal conclusions based on those data. At the end of this course, students will be able to apply modern econometric methods to population health and health policy questions and to evaluate the approach and conclusions of econometric studies. Methods will address analyzing non-linear regression models and longitudinal and panel data. The 2-semseter course will culminate in a paper through which students will independently apply methods learned during the year to observational data to address a problem of their choice in population health.
POPHS 910: Fundamentals of Qualitative Methods Instructor: Amy Corneli
This course will prepare learners for serving as an investigator of qualitative research studies. Learners will gain competency in 1) designing qualitative research studies, with an emphasis on selecting appropriate methods and analytical approaches and developing question guides; 2) providing oversight during data collection and analysis; 3) writing up study findings for peer-reviewed publications. Learners will gain competency in 1) designing rigorous qualitative studies for grant applications, 2) developing qualitative research study protocols, 3) identifying study domains and writing experiential question guides, 4) overseeing data collection and data management, 5) providing technical guidance during data analysis planning and implementation, 6) writing up study findings after analysis, and 7) preparing peer-reviewed manuscripts.
POPHS 912: Population Health Sciences Professional Development II Instructor: Hayden Bosworth
Following POPHS 907, this seminar will engage presenters from the Duke School of Medicine and University to address resources and professional development activities; leading multidisciplinary research teams, best practices for presenting and publishing research, career opportunities including building an academic CV, and preparing for a career in population health.
POPHS 921: Analytic Methods II: Causal Inference Instructor: Alan Brookhart
This course will consider drawing interference about causal effects in a variety of settings using the potential outcomes framework. Topics covered include casual interference in randomized experiments and observational studies, bounds and sensitivity analysis, propensity scores, graphical models, survival analysis, handling missing data, and other areas.
POPHS 923: Principles of Health Measurement Instructors: Theresa Coles & Christy Zigler
This course covers the development and initial evaluation of clinical outcomes assessments (COAs). We will begin by reviewing the attributes of a quality COA and classical test theory and discuss modern validity theory. The primary focus of the course will be qualitative methods for COA development including concept elicitation, item generation/writing, cognitive testing, and coding. The course will also cover the COA development process from COA-specific literature reviews to determine if a new COA (or modifications) are needed to measure key concepts.
*All POPHS electives are 3 credits
Students can choose electives offered through DPHS or courses housed in other departments. Electives from outside of DPHS should be chosen in consultation with your mentor, and are approved by the Director of Graduate Studies.
DPHS Electives
POPHS 811 Pragmatic Health Policy Research Fall, Instructor: Emma Sandoe This course bridges the divide between analysis/methods courses (generating evidence) and policy courses (understanding specific policy areas, process, and stakeholders) to help students build foundational knowledge and focused skills in framing/communicating timely, policy-relevant evidence, applicable to many population health-related career paths. Students will increase knowledge and mastery of theoretical and substantive foundations of pragmatic policy analysis, specific policy areas, and issues (e.g., SNAP, Medicaid, opioid use disorder, infant mortality, etc.). Students will be able to clearly communicate policy-relevant information, orally and in writing.
POPHS 812 Quality of Care and Population Health Spring, Instructor: Staff The goal of enhancing the quality of care and services provided by healthcare and community organizations is at the heart of much of the practice, evaluation, and research in population health sciences. This course focuses on 1) defining and identifying quality goals; 2) determining measures of quality; 3) planning projects to improve quality; and 4) summarizing the impact of quality improvement efforts.
POPHS 813 Population Health Through Implementation Science Fall, Instructor: Leah Zullig Implementation science addresses the translation of evidence-based practices, programs, and policies into real-world settings. This course will include didactic lectures, with case studies, applied group work, and a culminating real-world, hands-on implementation, dissemination, de-implementation, or QI science project.
POPHS 815 Health Services Research Fall, Instructor: Hayden Bosworth Health Services Research provides an overview of methods for undertaking research and program evaluation within health services organizations and systems. In addition to methods, the course also provides "the state of the art" in research and evaluation through the review of major completed studies. This course is recommended for students who will be carrying out policy research, social science research, or program impact evaluation within health delivery systems as well as developing and implementing programs to improve healthcare outcomes.
POPHS 816 Population Health Data Fall, Instructors: Lesley Curtis & Asheley Skinner This course will provide an introduction to multiple different types of data, including national surveys, electronic health records, health insurance claims, and others-all relevant to Population Health. Additionally, the course addresses issues such as data provenance, data linkage, governance, and ethics.
POPHS 818 Fundamentals of Qualitative Research Spring, Instructors: Amy Corneli & Brian Perry This course prepares learners for serving as a research assistant on qualitative research studies. Learners will have competency in 1) coordinating qualitative studies (e.g., screening, recruitment, regulatory, scheduling), 2) conducting qualitative interviews (e.g., demonstrable skills in leading in-depth interviews and focus groups), 3) managing study data, and 4) conducting qualitative analysis (e.g., demonstrable skills in analysis steps, use of software).
POPHS 895 Population Health Internship Summer, Instructor: Asheley Skinner Student gains practical experience by taking an internship in industry/government. Requires prior consent from the student's advisor and from the Director of Graduate Studies. May be repeated with consent of the advisor and the Director of Graduate Studies. Credit/no credit grading only.
- Get Assistance
- Health & Wellness
- Get Involved
- Identity, Culture and Religion
- Arts & Perfomance
- Space Finder
- Duke Career Center
- Parents and Families
Graduate & Professional Student Day Experience: Women’s Soccer Game (2024)
2024 grad/prof student day experience – #4 women’s soccer game.
As an extension to our 2024 Summer Transition Series webinar sessions , Graduate and Professional Student Services is excited to announce six immersive in-person Day Experiences!
Day Experiences are designed:
- For first-year incoming Graduate and Professional students at Duke University.
- To foster a sense of belonging for graduate and professional students with Duke University in addition to unique academic programs.
- To facilitate a pathway for students to build interdepartmental connections with other graduate and professional students at Duke.
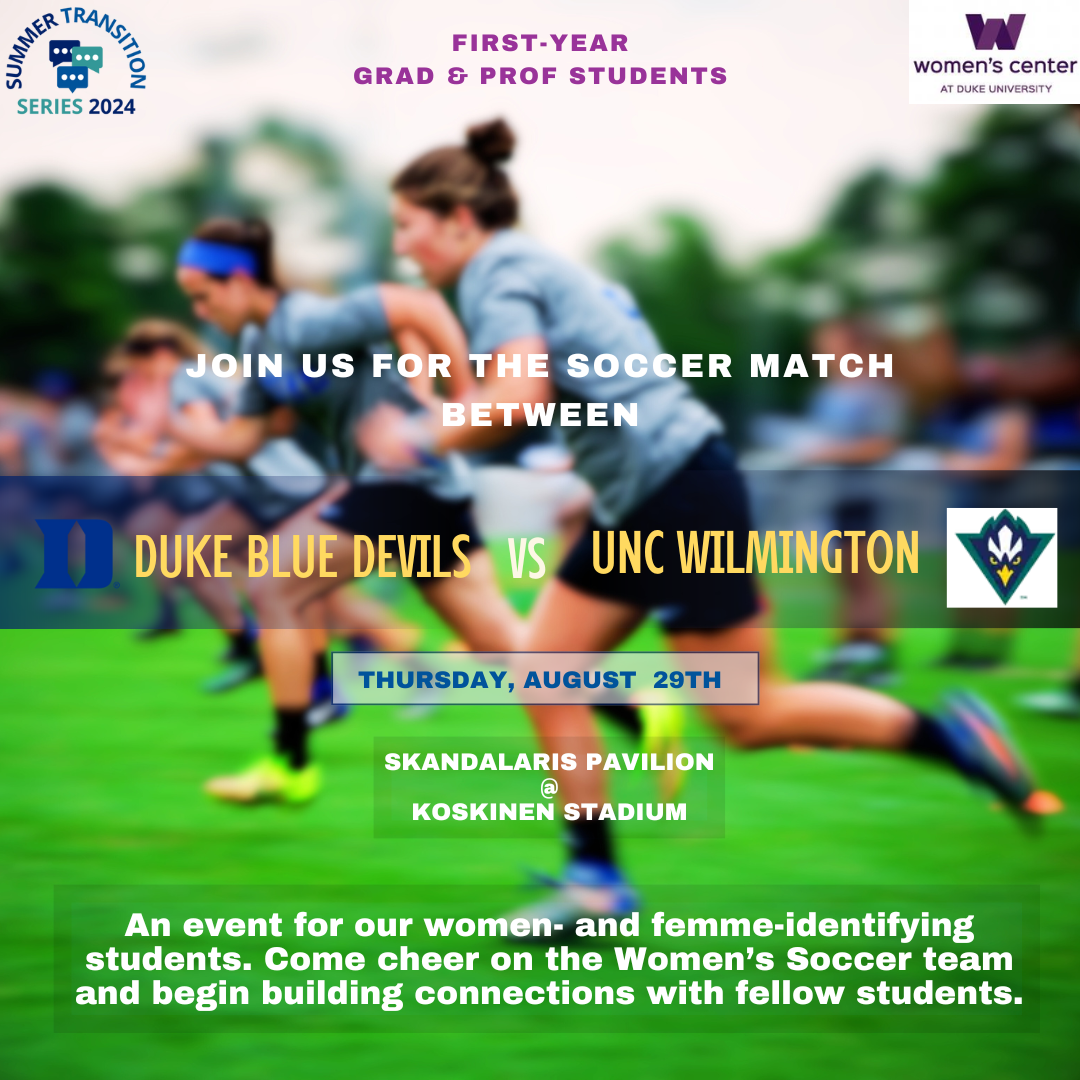
The Women’s Center is proud to host an event for our women- and femme-identifying students. Join us and cheer on the Duke Women’s Soccer team as they take on UNC Wilmington. Enjoy a fun, low-pressure environment in which you can meet other first-year students, network, and begin building a supportive sisterhood for the rest of your journey at Duke!
(Wanna get involved? Go to the Women’s Center’s Programs & Services page to join their group, register for events, access resources, and more!)
Tentative Itinerary*:
Thursday, August 29, 2024
- Location: Skandalaris Pavilion @ Koskinen Stadium
- Duke vs. UNC Wilmington.
*Itinerary and activities are subject to change
Additional information:
- There is no cost to attend. The food and beverages are all free.
- This is an in-person activity. Please only register if you can be in Durham, NC, on August 29, 2024. Space is limited.
- The venue is air-conditioned. If you tend to run cold, you may want to bring a sweater.
- Note for students who cover: There are likely to be some male staff and employees present. Please dress to your comfort level.
Communication Timeline:
- August 18: Registration will close at 11:59 PM EDT.
- August 19: Selected students will be notified (a waitlist will also be maintained).
- August 22: Confirmation and waiver collection by selected students.
- August 23: Waitlisted students will be notified.
- August 29: Duke Women’s Soccer Game event!
(Selected students will be notified by August 19 )
[Experience hosted by The Women’s Center ]
To learn about other Summer Transition Series Day Experiences, click here .
Additional Reads
2024 graduate professional student appreciation week.
Graduate Professional Student Appreciation Week (GPSAW) events at Duke are an excellent opportunity for students to come together and engage in activities that promote social…
Aaron Dinin to Deliver Keynote Address at 2024 Duke Jewish Baccalaureate Ceremony
Jewish Life at Duke is delighted to announce that Professor Aaron P Dinin will deliver the 2024 Duke Jewish Baccalaureate Ceremony keynote address.
Graduate & Professional Student Day Experience: Duke Gardens & Nasher Museum (2024)
2024 Grad/Prof Student Day Experience – #2 Duke Gardens & Nasher Museum As an extension to our 2024 Summer Transition Series webinar sessions, Graduate and Professional…
Two School of Medicine Students Named Tillman Scholars
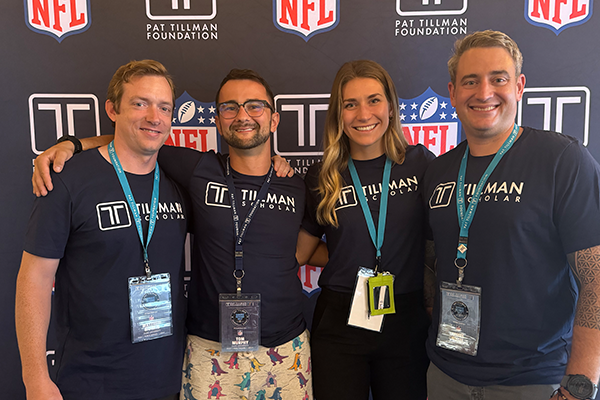
Two Duke University School of Medicine students have been named 2024 Tillman Scholars by the Pat Tillman Foundation, which recognizes exceptional military service members, veterans, and spouses and empowers them with academic scholarships, leadership development opportunities, and a global community of mentors and peers.
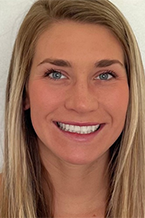
Elle Hepburn Reason, a third-year MD student, and James White, a first-year MD student, were named to this year’s class of Tillman Scholars. A total of 60 Tillman Scholars, including 17 in medical fields, were selected from among thousands of candidates nationwide.
Also named a 2024 Tillman Scholar from Duke was Matthew Morris, a dual degree candidate in the Master of Environmental Management and Master of Business Administration programs.
In all, six School of Medicine students have been named Tillman Scholars since the program began in 2009.
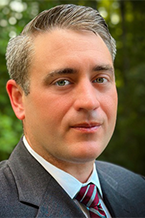
Reason, in addition to her clinical responsibilities, is conducting research on treatment options for a rare type of breast cancer and participates in mentorship activities for high school students from underrepresented minority groups in the Durham community. Her husband, Ross, is an infantry officer at Fort Liberty.
White, a West Point graduate who served six deployments in Afghanistan, Africa, and the Middle East, aspires to use his military and biomedical engineering experience to systematically identify and reduce medical errors while improving patient safety in clinical and pre-hospital health care environments.
The Pat Tillman Foundation was established by the family of Pat Tillman, an NFL football player who left the sport to join the U.S. Army after the 9/11 attacks and died during combat operations in Afghanistan in 2004.
College of Arts and Sciences
Mathematics, m.s. in statistics and data science.
The discipline of statistical sciences is concerned with the art of developing techniques to gain information and make decisions from data in the presence of uncertainty. The techniques are based on the theory and tools of the various branches of mathematics, especially probability.
A graduate education in the statistical sciences allows one an entry into many areas of physical and social sciences, medicine, business and government. There are more than 130 universities in the US that offer graduate programs in the statistical sciences. In spite of the declining graduate enrollment in most disciplines across the country, the enrollment in statistics has remained steady over the last fifteen years. An average of 1000 M.S. degrees in Statistics and Biostatistics are awarded every year. A recent study by the NSF and DOE (NSF publication 80-78) concludes that during the 90's "the supply of scientists and engineers at all degree levels will likely be more than adequate to meet demand in all fields except computer professions, statistics and some fields of engineering".
The Program
The prerequisite for admission to the M.S. degree in Statistics and Data Science is an undergraduate degree that includes at least 9 semester-hours of calculus. Students who have not had any course in linear algebra, complex variables and advanced calculus are advised to take Lehigh's Math 205 (or 244), Math 208 (or 316) and Math 301 at the earliest opportunity.
The M.S. in Statistics and Data Science requires 30 credit hours of graduate courses with at least 18 hours of 400-level courses. The choice of the courses must be approved by the graduate advisor. Up to 6 hours of coursework may be replaced with a masters thesis. All students in the program must also pass a comprehensive examination on basic probability, statistics and linear algebra.
The M.S. program in Statistics and Data Science has two tracks, Statistics and Stochastic Modeling. The following is a guide for courses and electives in the two tracks.
Please see Lehigh's catalog for course descriptions
Statistics Track
First year recommended course sequence
- MATH 309 Probability with Applications and Simulation
- MATH 312 Statistical Computing and Applications
- STAT 342 Applied Linear Algebra
- STAT 410 (Random Processes and Applications)
- STAT 434 (Mathematical Statistics)
- STAT 438 (Linear Models in Statistics with Applications)
Second Year
Select at least 4 electives from the following (not all courses are offered every year):
- STAT 412 Advanced Applied Statistics
- STAT 462 Modern Nonparametric Methods in Statistics
- STAT 461 Topics in Mathematical Statistics
- STAT 439 Time Series and Forecasting
- MATH 450 Special topics - Programming in SAS
- STAT 465 Statistical Machine Learning
- STAT 471 Topics in Statistical Learning and Computing
- STAT 474 Statistical Practice
Stochastic Modeling Track
Recommended Courses
- Math 309 Theory of Probability
- STAT 410 Random Processes
- STAT 463 Advanced Probability
- Math 401 Real Analysis I
- STAT 434 Mathematical Statistics
- STAT 438 Linear Models in Statistics with Applications
- STAT 464 Advanced Stochastic Processes
- Math 341 Mathematical Models and Their Formulation
Select one other possible elective from the following:
- STAT 408 Seminar in Statistics and Probability (Spring)
- STAT 409 Seminar in Statistics and Probability (Fall)
- Math 320 Ordinary Differential Equations
- Math 340 Design and Analysis of Algorithms
- Math 402. Real Analysis II
- Math 407 Theory and Techniques of Optimization
- Math 430 Numerical Analysis
- Math 467 Financial Calculus I
- Math 468 Financial Calculus II
- Eco. 453 Index Numbers and Time Series Analysis
- CSE 411 Advanced Programming Techniques
- I.E. 316 Advanced Operations Research Techniques
- I.E. 339 Queuing Theory
- I.E. 409 Time Series Analysis
- I.E. 416 Dynamic Programming
- I.E. 439 Applications of Stochastic Processes
Additional Information
Current Math courses to be cross-listed in the catalog as STAT courses are as follows. We have elevated some 300 level Math courses to the level of 400 STAT courses in order to facilitate meeting the 18 credit hour requirement for M.S. in Statistics and Data Science.
- STAT 410 = Current Math 310
- STAT 434 = Current Math 334
- STAT 438 = Current Math 338
- STAT 462 = Current Math 462
- STAT 461 = Current Math 461
- STAT 463 = Current Math 463
- STAT 464 = Current Math 464
An undergraduate student will receive 4 hours of credit if enrolled in Math 310, 334, 312 or 338 but 3 hours of credit if enrolled in STAT 410, 434, 412 or 438.
For more information, please send e-mail to [email protected] .
Program Requirements

Financial Technology - Master of Engineering
Become a Leader and Driver of Innovation Technologies like AI (machine learning) and blockchain are driving advances across the financial sector. The Duke Master of Engineering in Financial Technology (FinTech) will prepare you with the technical, financial and management skills to lead the next generation of financial innovation.
Financial Technology – Master of Engineering
Is duke’s fintech master’s right for you, consider applying if you are an:.
- Engineering, computer science, physics, math, statistics or econometrics graduate of an accredited institution who wants to create solutions that automate transactions, create revenue, strengthen security and add value
- Economics or business graduate who wants to learn about emerging technologies in the finance space
- Ambitious working professional looking for an online or accelerated degree that will advance your career trajectory
- Undergraduate at Duke who is interested in FinTech and the 4+1: Bachelor’s + Master’s program
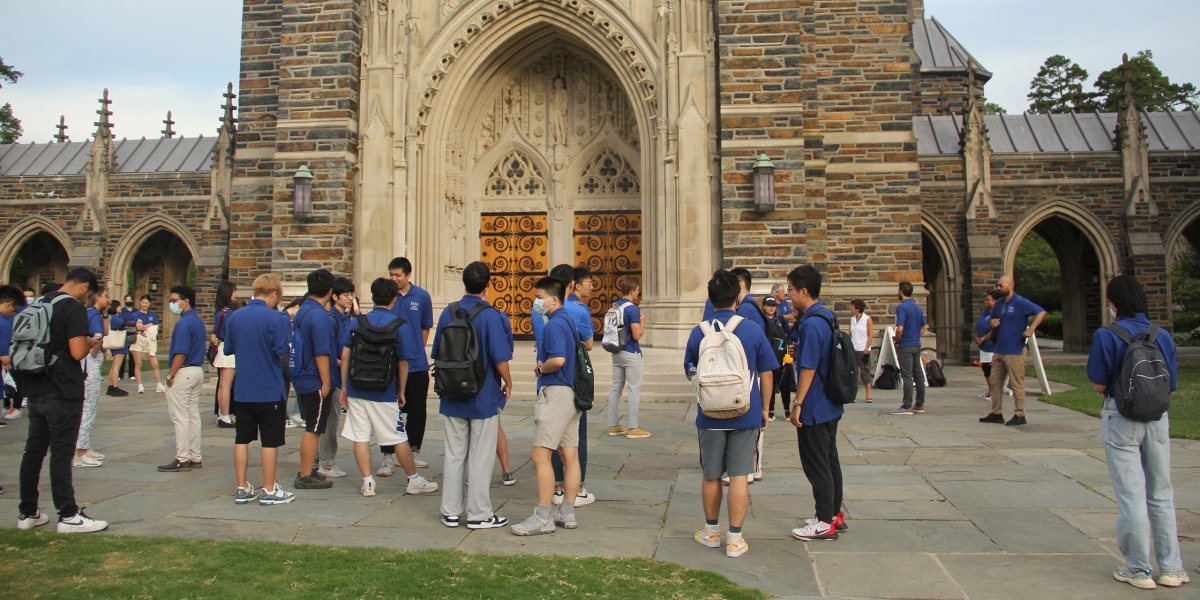
Digital Assets @ Duke Conference January 30-31, 2025
Join key industry players in the digital assets space, regulatory experts and select researchers for two days of rigorous debate, discussions and education.
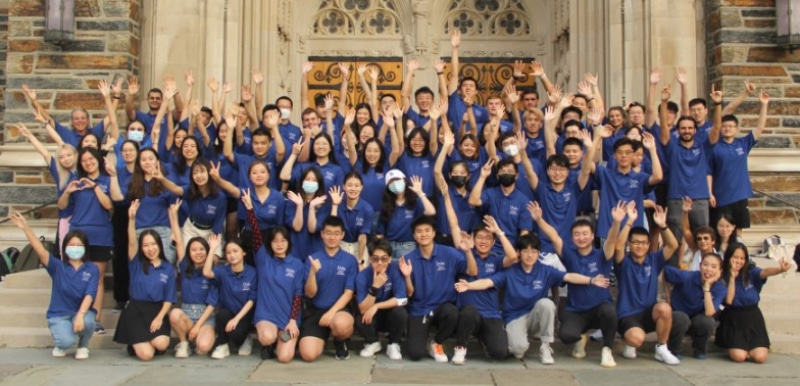
Study On Campus or Online
30-credit degree program | 10 courses.
- Study online or on-campus
- Business courses, plus project or internship
- Complete in 12 months to 29 months
12-29 months
to complete on-campus or online

7 technical courses
provide in-demand knowledge and skills
2 business courses
develop leadership and management skills
1 capstone project
builds your real-world work portfolio
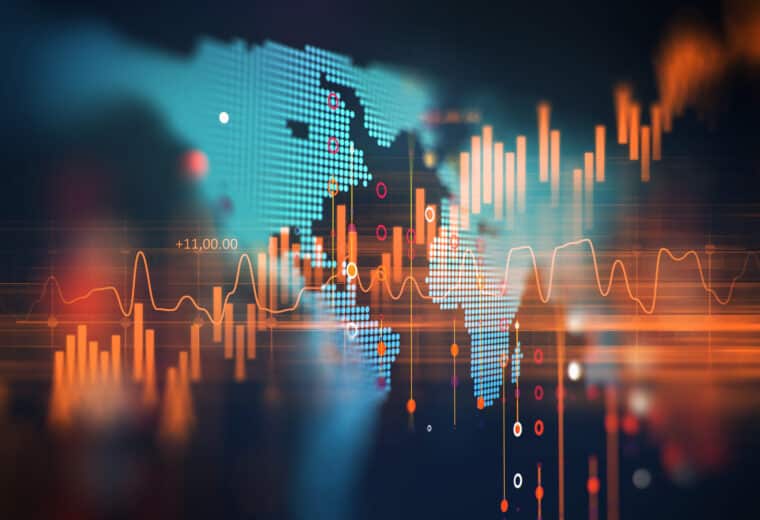
Duke Engineering Announces a 12-Month Master of Engineering in Financial Technology
Duke’s Master of Engineering in FinTech now offers a 12-month program for professionals with work experience, starting in Summer 2024. Applications open this fall.
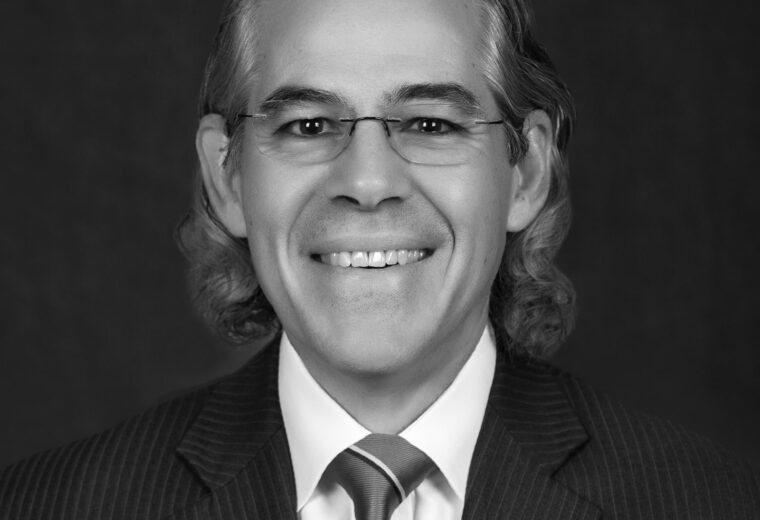
Pratt Trailblazers: Jimmie Lenz
Jimmie Lenz embraced the transformative potential of blockchain technology and digital assets early on—a vision that ultimately reshaped his career trajectory and brought him to Duke University
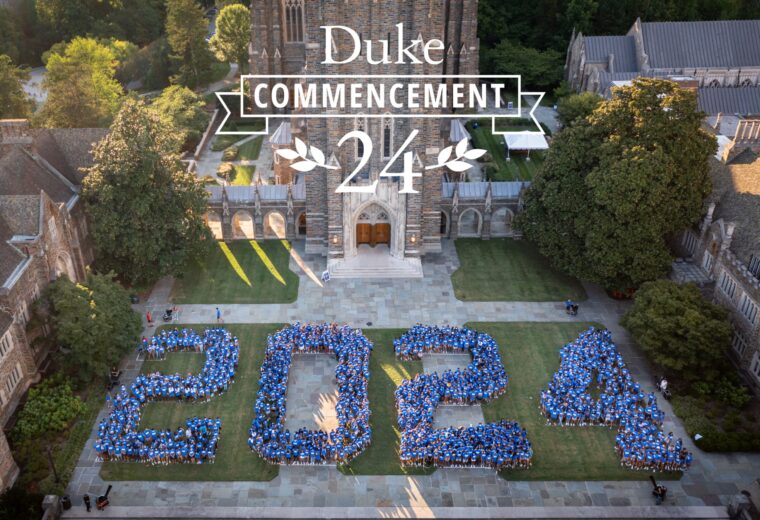
Duke Engineering Celebrates the Class of 2024
On Sunday, May 12, 2024, Duke Engineering celebrated about 1,100 graduates from its various programs
More episodes »
Fall semester classes begin (8:30 AM); Drop/Add continues
Aug 26 8:30 am-8:30 am
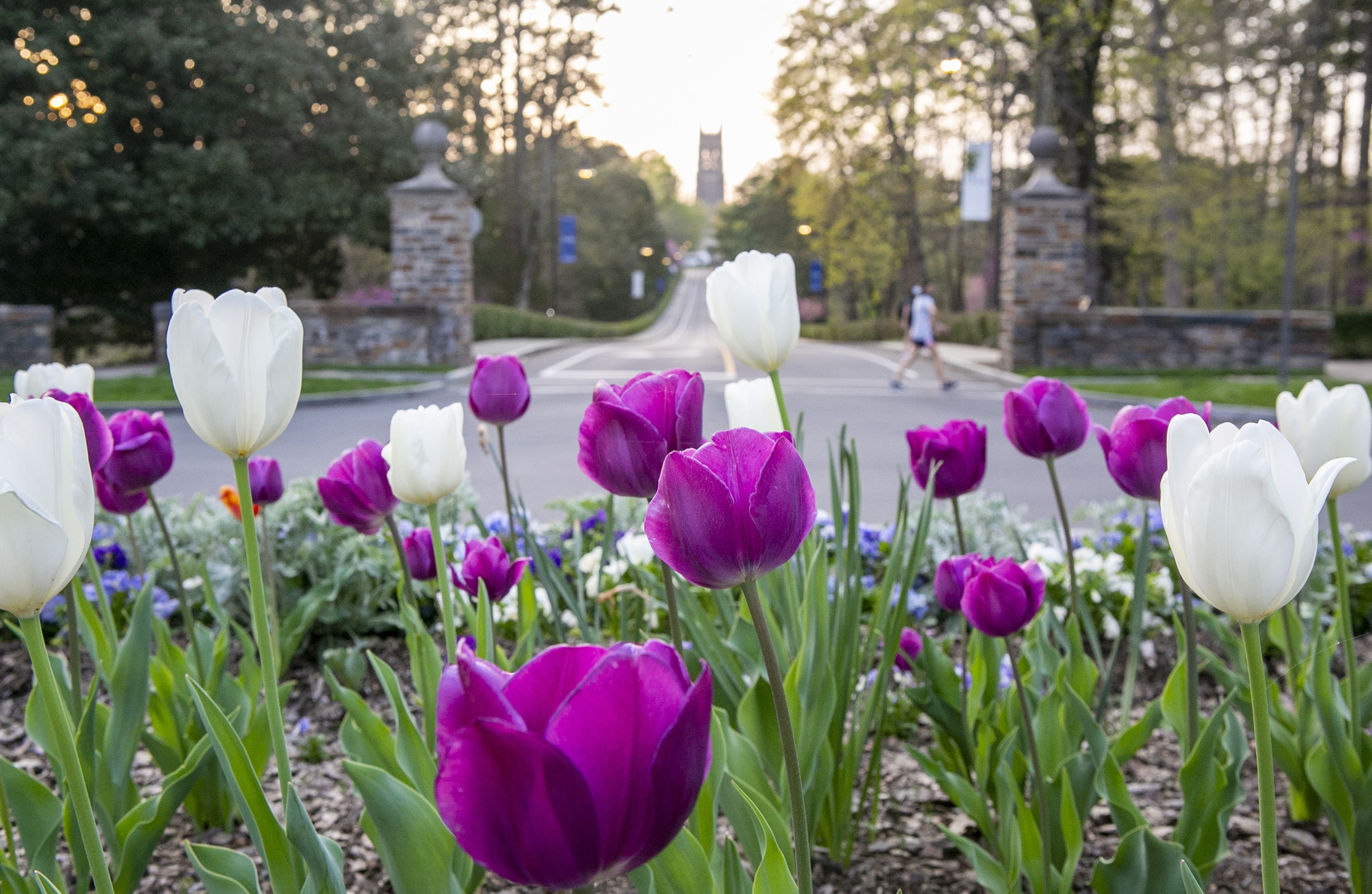
3-Minute Thesis Competition
PhD students from the School of Medicine and the Pratt School of Engineering will compete in the 3-Minute Thesis final round. Come cheer them on.
3:45 pm Fitzpatrick Center Schiciano Auditorium
3-Minute Thesis Final Competition
PhD candidates in the Pratt School of Engineering and the School of Medicine will share their research to a non-expert audience in 3 minutes. Judges will select 3 winners for […]
Summer Term 2 Classes End
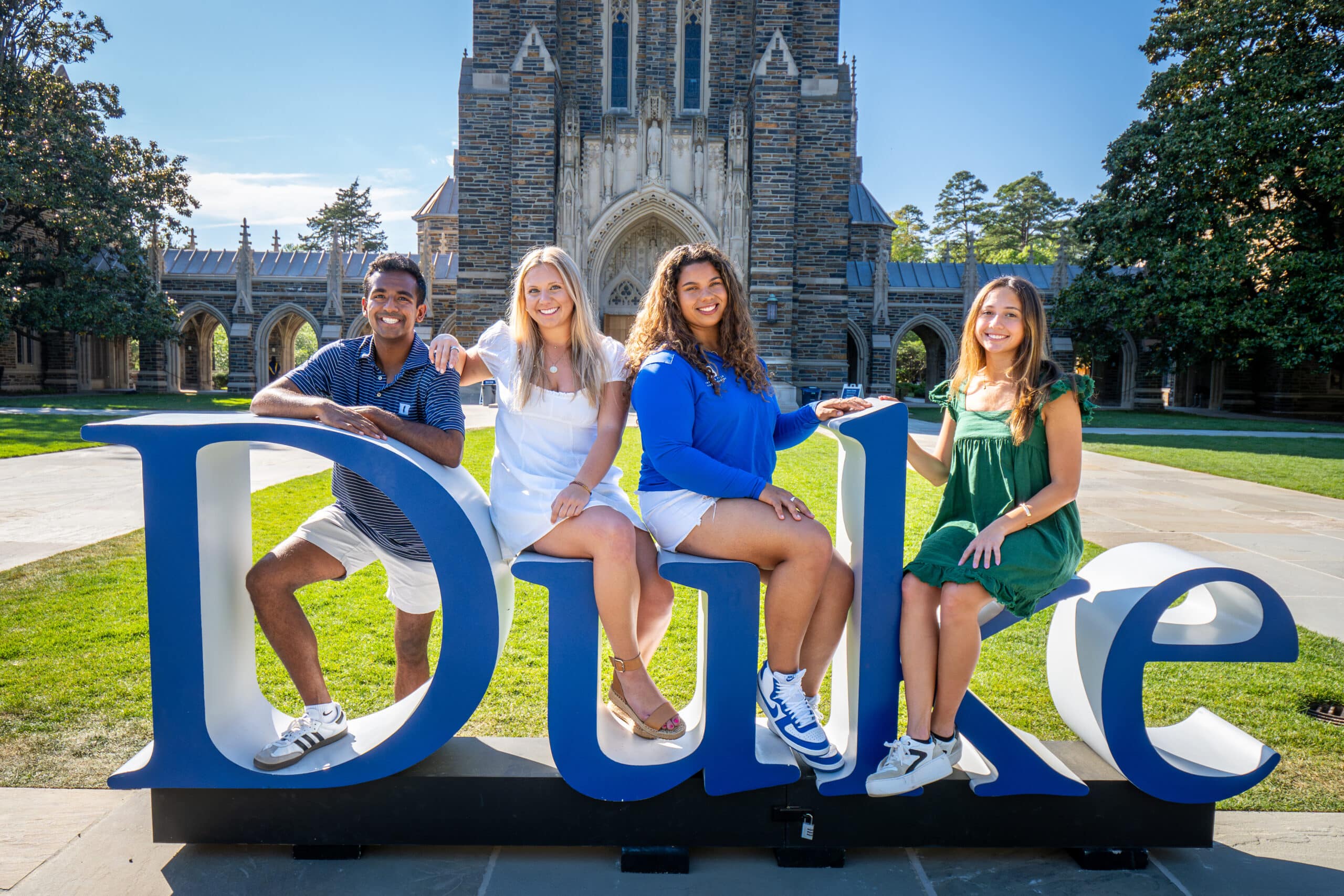
All Departments: PhD and Master's Admissions and Enrollment Statistics
- Honors Programs
- Online Learning
- Graduate Programs
- Penn State Law
- Dickinson Law
- College of Medicine
- Undergraduate Campuses
- Residence Life
- Clubs and Organizations
- Well-Being and Safety
- Diversity and Belonging
- Academic Credit
- Resources and FAQ
- How to Apply
- Scholarships
- Student Employment
- Federal Work-Study
- Prospective Students
- Current Students
- Transfer Students
- Military and Veteran Students
- Graduate and Professional Students
- Understanding Your Offer
- Paying Your Bill
- Why Aid is On Hold
- Special or Unusual Circumstances
- Accepting or Declining Aid
- Nittany Lion Shrine
- Board of Trustees
- President's Office
- University Faculty Senate
- Stories of Impact
- Undergraduate
- Graduate and Professional Studies
- Online Degrees
- Academic Colleges
- Campus Locations
- Global Education
- Academic Calendar
- Explore Penn State
- Continuing Education
- Professional Schools
- Costs & Aid
- How Aid Works
- Types of Aid
- Apply for Aid
- Managing Your Aid
- Cost of Attendance
- Latest News
- Our Research
- Campus Life
- Administration
- Arts and Entertainment
- College and Campus News
- Mission and Values
- Facts and Rankings
- Health Care
Tuition and Costs
We know costs are an important factor in any decision to invest in a college education. We want to help you to make an informed choice.
Penn State Tuition
Below are the most recent tuition rates with room and board estimates for an academic year (fall and spring semesters). These costs are set by the Board of Trustees each year in July. We encourage students and families to budget for additional cost including travel, personal expenses, books, and supplies. Penn State's tuition rates vary by campus, program, student level, and residency.
Please visit the Office of Summer Session website to see an estimate of the costs for Penn State's summer session.
All World Campus students pay the same tuition rate, regardless of residency. Visit the World Campus website for tuition details.
Penn State provides several tools to help you estimate the costs associated with earning a degree. We encourage students to use them in order to have a better understanding of the true cost of attendance.
College Cost Calculator
Estimate essential costs of attending Penn State, then customize for a more accurate reflection of your individual expenses.
Tuition Website
Use this tool to calculate tuition based on campus, program, and residency, and see specific rates for individual students.
Housing and Food Services
Research room and meal rates for each campus to understand the costs associated with your living options.
Penn State Global
Find detailed financial information for international students interested in attending Penn State, including costs and aid options.
Penn State Student Aid
Penn State’s Office of Student Aid handles loans, grants, work-study, and scholarships, and is your go-to for financial questions.
Residency Information
Since Penn State is a state-affiliated institution, tuition rates are different for in-state and out-of-state students. Learn more about the policy and the process if you have to appeal residency.
Generally speaking, a student needs to live in Pennsylvania for non-academic reasons for a year prior to enrollment to be considered a resident for tuition purposes. More details on Penn State’s residency policy and residency FAQs can be found on the Office of the Bursar website .
Residency Appeal plus icon
When students apply to Penn State, most times residency is set automatically based on the location of a student’s high school. If this is the case when you apply, and your high school no longer reflects your state of residency, or you believe you have been incorrectly classified as an out-of-state student, you will need to submit a residency appeal . Details about this process are available on the Office of the Bursar website.
For residency appeals prior to enrollment, please send the following documentation to the Undergraduate Admissions Office by mail or fax. Be sure to include your full name and Penn State ID or MyPennState user ID with your appeal. Appeals should include a written explanation, as well as the following items:
Students 24 and Under
Copy of your parents' lease, deed, or mortgage
Copy of your parents’ first page of the Federal and Pennsylvania income tax returns for the most recent year (you may block out financial information)
Copy of your parents’ current Pennsylvania driver's license or state-issued photo identification card
Adult Students
Copy of your lease, deed, or mortgage
Copy of the first page of your Federal and Pennsylvania income tax returns for the most recent year (you may block out financial information)
Copy of your current Pennsylvania driver's license or state-issued photo identification card
Residency Information for Active Military Personnel or Dependents
Veterans using any chapter of the GI Bill®, and dependents receiving Chapter 33, Chapter 35 or Fry Scholarship benefits will be eligible for in-state tuition regardless of residency. If you are a veteran and you are not using GI bill® benefits, please contact your campus Certifying Official to determine requirements to establish eligibility for the in-state rate. All active duty military and DOD employees (their spouse and dependent children) are eligible for consideration to receive in-state tuition provided they are assigned to an active duty station in Pennsylvania and reside in Pennsylvania. Active duty military and DOD employees (their spouse and dependent children) enrolled in Penn State's World Campus will receive in-state tuition.
GI Bill® is a registered trademark of the U.S. Department of Veterans Affairs (VA). More information about education benefits offered by VA is available at the official U.S. government website at www.benefits.va.gov/gibill .
Scholarships and Awards
Scholarships and awards are a form of financial aid that does not need to be repaid. Penn State offers several sources for possible scholarships funding. While not a requirement for all scholarships, Penn State recommends that all students who are offered admission submit a Free Application for Federal Student Aid (FAFSA) to maximize their aid options.
T he Office of Student Aid is just one unit that offers scholarships and awards at Penn State. Additionally, scholarships and awards can be offered from academic colleges, campuses, and other administrative units, some of which will require separate applications. In addition to the scholarships and awards outlined on the Office of Student Aid website , Penn State offers the following awards to incoming first-year and transfer students through Undergraduate Admissions:
Penn State Discover Award
The Discover Award is awarded to first-time, first-year domestic students who enroll at a Penn State campus other than Penn State University Park or Penn State World Campus for both the fall and spring semester immediately following their high school graduation. Transfer students who enroll in a degree program that can be completed at a Penn State campus other than Penn State University Park or Penn State World campus are also eligible.
Penn State Provost's Award
The Provost's Award is a four-year award that is available to first-time, first-year students with offers of admission to any of Penn State’s twenty undergraduate campuses, excluding Penn State World Campus. Both Pennsylvania and non-Pennsylvania residents are considered for this award, as well as international students, and it is very competitive. This award is not available for transfer students to Penn State.
The Pennsylvania State University 201 Old Main University Park, PA 16802
- instagram icon
- facebook icon
- linkedIn icon
- youtube icon
Student Support
Quick links, stay connected.
- Privacy Statements
- Non Discrimination
- Accessibility
- Equal Opportunity
- Legal Statements

IMAGES
VIDEO
COMMENTS
Statistical Science at Duke is the world's leading graduate research and educational environment for Bayesian statistics, emphasizing the major themes of 21st century statistical science: foundational concepts of statistics, theory and methods of complex stochastic modeling, interdisciplinary applications of statistics, computational statistics, big data analytics, and machine learning. Life ...
All students are encouraged to register for STA 701S Statistical Science Graduate Student Seminar each semester. Every student in their 3rd year or higher must give (at least) one presentation/talk in STA 701S each year; this is a primary opportunity for practicing research presentations and presenting progress in research to committee members ...
Statistical Science. 214 Old Chemistry Box 90251 Durham, NC 27708-0251 (919) 684-4210
Merlise Clyde Director of Graduate Studies Department of Statistical Science Duke University Box 90251 Durham, NC 27708-0251 Phone: (919) 684-8029. Contact: Director of Graduate Studies (for PhD) [email protected]. PhD Program: https://stat.duke.edu/phd.
Statistics. In an effort to provide comprehensive information for all interested individuals, The Duke University Graduate School posts summary data on its Ph.D. and master's programs. These data include information such as total applications, admissions, matriculations, demographics, median GRE and GPA scores, and career outcomes.
Graduating students are extremely well-prepared and competitive for graduate study in statistics and allied disciplines, and for early careers in business, finance, law, medicine or other fields. The department offers Interdepartmental Majors in Data Science (Statistical Science + Computer Science) or in Statistical Science + another field of ...
The PhD program offers thorough preparation in the theory and methods of statistics, with major emphases on modern, model-based statistical science, Bayesian and classical approaches to inference, computational statistics, and machine learning. A hallmark of the program is the integration of interdisciplinary applications into teaching and ...
The Department of Biostatistics and Bioinformatics offers a Ph.D. degree in Biostatistics through the Duke University Graduate School. A distinguishing feature of the program is its integration within the world-class biomedical research enterprise at Duke University and the Duke School of Medicine. The goal of the program is to train students ...
The most important requirement for the Ph.D. degree is the writing of a satisfactory Ph.D. Dissertation. Your dissertation must be approved, first by the Advisor and then by the entire Dissertation Committee. You and your advisor should be fully aware of all details of the Graduate School requirements for thesis format and submission. (You can also find macros for thesis production at this ...
M.S. Program. Master's in Statistical Science (MSS) program is a rigorous two-year graduate experience, where you'll delve into the very core of statistical theory, methods, computation, and their real-world applications. This is the pathway to unlock a world of professional opportunities in industry, business, and government.
Graduate students Edric Tam and Andrew McCormack, ... and McCormack, who came from the University of Toronto with a degree in Statistics, are now 5 th-year PhD students in the Department of Statistical Sciences. ... Tam will continue his research at Duke before applying to post-doc programs. In reflecting on their paths that have brought them ...
All Duke biomedical PhD students are required to complete 18 contact hours of Responsible Conduct in Research (RCR) training. RCR training is provided by the Office of Biomedical Graduate Education (OBGE). The required curriculum differs for students matriculating in Fall 2020 or later and those who matriculated prior to Fall 2020.
The PhD program follows the Duke Graduate School Academic Calendar. View the timeline for students with and without an Applicable Quantitative Master's Degree. For students with a Master's degree in Biostatistics, some of the required 700 level courses listed below may be waived if they have taken those courses or their equivalents previously.
Why Choose Duke for a PhD in Population Health Sciences? The department, which is part of the Duke University School of Medicine, offers Ph.D. candidates a unique and rich setting in which to acquire that foundation and then use it to complete their studies and dissertation research. Ph.D. students can:
Current PhD Students Current PhD Students Current PhD Students. David Anderson. PhD Student. Maryam Aziz. PhD Student. Lindsay Ballengee, PT, DPT. PhD Student. Colleen Burke . PhD Student. Tina Cheng . PhD Student. January Cornelius . ... @2024 Duke University and Duke University Health System.
General information for students taking a first statistics course at Duke. For information, questions, or comments about Undergraduate courses, please email [email protected] ; for information about the Graduate program, email [email protected] . Current course homepages.
All Departments: PhD Admissions and Enrollment Statistics - The Graduate School
Duke Student Medical Insurance: 3282.12: 3,461.64: Duke Student Dental Insurance: 329.00: 329.00 Recommended Stipends for Ph.D. Students (All Position Types) The stipend rates listed below are the amounts recommended by The Graduate School. They apply to Ph.D. students in programs housed in Trinity, Nicholas, Pratt, Nursing, Medicine, and ...
In their first two years, students in the Duke PhD in Population Health Sciences program take classes in population health, statistical methods and programming, research methods, and more. In the remaining years, students focus their efforts on their dissertation. Year 1 Fall: 10 credits (14 with RCR training) Spring: 10 credits (14 with RCR training)
Are you interested in pursuing a PhD in computer science at Duke University? Find out the admission and enrollment statistics for this program, such as the number of applicants, acceptances, enrollments, and degrees awarded. Compare the data by gender, ethnicity, citizenship, and funding sources. Learn more about the computer science department and its research areas at Duke.
2024 Grad/Prof Student Day Experience - #4 Women's Soccer Game As an extension to our 2024 Summer Transition Series webinar sessions, Graduate and Professional Student Services is excited to announce six immersive in-person Day Experiences! Day Experiences are designed: The Women's Center is proud to host an event for our women- and femme-identifying students. Enjoy a fun, low-pressure ...
Graduate School students in Ph.D., A.M., M.S., M.F.A. and M.A.T. degree programs are invited to attend this general information and orientation session. Who Should Atte
Two Duke University School of Medicine students have been named 2024 Tillman Scholars by the Pat Tillman Foundation, which recognizes exceptional military service members, veterans, and spouses and empowers them with academic scholarships, leadership development opportunities, and a global community of mentors and peers.
Responsible Conduct of Research training is a formal requirement for every PhD student enrolled in The Graduate School. This orientation is a requirement for incoming, new students, but it is open to all graduate students for RCR credit. The orientation is being held entirely online via Zoom on Canvas, Duke's learning management platform.
Students who have not had any course in linear algebra, complex variables and advanced calculus are advised to take Lehigh's Math 205 (or 244), Math 208 (or 316) and Math 301 at the earliest opportunity. The M.S. in Statistics and Data Science requires 30 credit hours of graduate courses with at least 18 hours of 400-level courses.
PhD student Braden Scherting won the best poster award at the 2024 International Statistical Ecology Conference in Swansea, Wales for his presentation "Interpretable ecological community modeling for observed abundance." Braden worked with Professor David Dunson to introduce a new class of joint species distribution model (JSDM) for abundance data based on sparse, nonnegative matrix ...
Engineering, computer science, physics, math, statistics or econometrics graduate of an accredited institution who wants to create solutions that automate transactions, create revenue, strengthen security and add value ... Undergraduate at Duke who is interested in FinTech and the 4+1: Bachelor's + Master's program ... PhD students from the ...
Further information regarding the application process and requirements is available at the Graduate School website above or by mail: Graduate School Admissions Office. 2127 Campus Drive. Duke University. Box 90065. Durham, NC 27708-0065. phone/fax: (919) 684-3913 /2277. Additionally, we expect all students to have multivariate calculus, linear ...
All Departments: PhD and Master's Admissions and Enrollment Statistics. All Departments: PhD and Master's Admissions and Enrollment Statistics. More Statistics. The Graduate School. The Graduate School 2127 Campus Drive Durham, NC 27708. (919) 681-3257. Contact Us Make A Gift.
When students apply to Penn State, most times residency is set automatically based on the location of a student's high school. If this is the case when you apply, and your high school no longer reflects your state of residency, or you believe you have been incorrectly classified as an out-of-state student, you will need to submit a residency appeal.